Training a Mini-GPT to Learn Two-Digit Addition#
Motivation#
Generative Pre-trained Transformer (GPT) are well known to perform bad on arithmetic tasks such as addition. This should not come as a surprise since GPT is a language model and not a math model. It is designed to train on a large corpus of text and learn the patterns and structure of natural language. While we do encounter many arithmetic operations in corpus, the encoding of these operations are often in a form that is in the text sense, not in the mathematical sense. After all, what GPT does best is to predict the next token over the entire vocabulary distribution.
In one of the examples provided from the repository minGPT, Karpathy demonstrates training a GPT model to learn the addition of two numbers presented as strings. This is a simple task designed to illustrate how a decoder-only model can be trained to learn “addition”. Thus, the input is a sequence of characters representing an addition operation (like “12 + 35”) and the output is the sequence of characters representing the result of the addition (like “47”).
To this end, we replicate his example, which serves as a proof-of-concept to show that decoder only models, which are often used for language-related tasks, can learn other patterns or “languages,” such as the “language” of arithmetic.
from __future__ import annotations
from tqdm.auto import tqdm
import inspect
import math
import os
import sys
import time
from dataclasses import dataclass
from pathlib import Path
from typing import Any, Callable, Dict, List, Optional, Tuple, Union
import matplotlib.pyplot as plt
import rich
import seaborn as sns
import torch
from omegaconf import OmegaConf as om
from rich.pretty import pprint
from torch import nn
from torch.optim import Optimizer
from torch.optim.lr_scheduler import LRScheduler
from torch.utils.data import DataLoader, Dataset, Subset, random_split
def find_root_dir(current_path: Path | None = None, marker: str = '.git') -> Path | None:
"""
Find the root directory by searching for a directory or file that serves as a
marker.
Parameters
----------
current_path : Path | None
The starting path to search from. If None, the current working directory
`Path.cwd()` is used.
marker : str
The name of the file or directory that signifies the root.
Returns
-------
Path | None
The path to the root directory. Returns None if the marker is not found.
"""
if not current_path:
current_path = Path.cwd()
current_path = current_path.resolve()
for parent in [current_path, *current_path.parents]:
if (parent / marker).exists():
return parent
return None
current_file_path = Path(os.getcwd())
root_dir = find_root_dir(current_file_path, marker='omnivault')
if root_dir is not None:
sys.path.append(str(root_dir))
from omnivault._types._alias import Accuracy, Loss
from omnivault.core.logger import RichLogger
from omnivault.transformer.config.composer import Composer, DataConfig
from omnivault.transformer.config.constants import MaybeConstant
from omnivault.transformer.config.decoder import (
AddNormConfig,
DecoderBlockConfig,
DecoderConfig,
MultiHeadedAttentionConfig,
PositionwiseFeedForwardConfig,
)
from omnivault.transformer.config.generator import GeneratorConfig
from omnivault.transformer.config.global_ import MaybeGlobal
from omnivault.transformer.config.optim import OPTIMIZER_REGISTRY, AdamConfig, OptimizerConfig
from omnivault.transformer.config.scheduler import SCHEDULER_REGISTRY, LambdaLRConfig
from omnivault.transformer.config.trainer import TrainerConfig
from omnivault.transformer.core.callbacks import save_state
from omnivault.transformer.core.dataset import (
AdderDataset,
construct_dummy_batch_future_masks,
construct_dummy_batch_target_padding_masks,
create_loader,
split_dataset,
)
from omnivault.transformer.core.optim import apply_weight_decay_to_different_param_groups
from omnivault.transformer.core.tokenizer import AdderTokenizer
from omnivault.transformer.core.trainer import Trainer, TrainerEvent
from omnivault.transformer.core.vocabulary import AdderVocabulary
from omnivault.transformer.decoder.core import GPTDecoder
from omnivault.transformer.modules.attention.core import ScaledDotProductAttention
from omnivault.transformer.projects.adder.main import evaluate_and_generate_on_valid_epoch_end
from omnivault.transformer.utils.general_utils import create_directory, download_file
from omnivault.transformer.utils.visualization import show_attention_heatmaps
from omnivault.utils.config_management.omegaconf import load_yaml_config, merge_configs
from omnivault.utils.inspector.core import get_field_annotations
from omnivault.utils.reproducibility.seed import seed_all
else:
raise ImportError("Root directory not found.")
Config#
yaml_cfg = load_yaml_config(yaml_path=root_dir / "omnivault/transformer/projects/adder/config.yaml")
cfg = merge_configs(yaml_cfg, args_list=[])
om.resolve(cfg) # inplace ops
constants: MaybeConstant = MaybeConstant(
NUM_DIGITS=2,
TOKENS=[
"0",
"1",
"2",
"3",
"4",
"5",
"6",
"7",
"8",
"9",
"+",
"*",
"-",
"=",
"<BOS>",
"<EOS>",
"<PAD>",
"<UNK>",
],
)
global_config: MaybeGlobal = MaybeGlobal(seed=42, debug=True, debug_samples=100)
data_config: DataConfig = DataConfig(**cfg.data)
optimizer_config = AdamConfig(name="torch.optim.Adam", lr=0.2, betas=(0.9, 0.98), eps=1e-9)
cfg.trainer.device = "cpu"
cfg.trainer.max_epochs = 9
trainer_config = TrainerConfig(**cfg.trainer)
generate_config = GeneratorConfig(**cfg.generator)
composer = Composer(
constants=constants,
global_=global_config,
data=data_config,
optimizer=optimizer_config,
trainer=trainer_config,
generator=generate_config,
)
pprint(composer)
LOGGER = RichLogger(**composer.logger.model_dump(mode="python")).logger
Composer( │ constants=MaybeConstant( │ │ NUM_DIGITS=2, │ │ TOKENS=[ │ │ │ '0', │ │ │ '1', │ │ │ '2', │ │ │ '3', │ │ │ '4', │ │ │ '5', │ │ │ '6', │ │ │ '7', │ │ │ '8', │ │ │ '9', │ │ │ '+', │ │ │ '*', │ │ │ '-', │ │ │ '=', │ │ │ '<BOS>', │ │ │ '<EOS>', │ │ │ '<PAD>', │ │ │ '<UNK>' │ │ ] │ ), │ logger=LoggerConfig( │ │ log_file=None, │ │ module_name=None, │ │ propagate=False, │ │ log_root_dir=None, │ │ rich_handler_config={ │ │ │ 'level': 'INFO', │ │ │ 'console': MISSING, │ │ │ 'show_level': True, │ │ │ 'show_path': True, │ │ │ 'show_time': True, │ │ │ 'rich_tracebacks': True, │ │ │ 'markup': True, │ │ │ 'log_time_format': '[%Y-%m-%d %H:%M:%S]' │ │ } │ ), │ global_=MaybeGlobal(seed=42, debug=True, debug_samples=100), │ data=DataConfig( │ │ context_length=11, │ │ dataset_name='adder_dataset', │ │ dataset_size=10000, │ │ dataset_path='./data/adder/adder_dataset.txt', │ │ dataset_dir='./data/adder', │ │ dataset_url='https://raw.githubusercontent.com/gao-hongnan/omniverse/dev/omnivault/transformer/projects/adder/assets/adder_dataset.txt', │ │ split=[0.7, 0.2, 0.1], │ │ collate_fn={'batch_first': True, 'pad_token_id': 16}, │ │ train_loader={ │ │ │ 'batch_size': 32, │ │ │ 'shuffle': True, │ │ │ 'num_workers': 0, │ │ │ 'pin_memory': False, │ │ │ 'drop_last': False │ │ }, │ │ valid_loader={ │ │ │ 'batch_size': 32, │ │ │ 'shuffle': False, │ │ │ 'num_workers': 0, │ │ │ 'pin_memory': False, │ │ │ 'drop_last': False │ │ }, │ │ test_loader={ │ │ │ 'batch_size': 128, │ │ │ 'shuffle': False, │ │ │ 'num_workers': 0, │ │ │ 'pin_memory': False, │ │ │ 'drop_last': False │ │ } │ ), │ model=MISSING, │ optimizer=AdamConfig(name='torch.optim.Adam', lr=0.2, betas=(0.9, 0.98), eps=1e-09, weight_decay=0.0), │ criterion=MISSING, │ scheduler=MISSING, │ trainer=TrainerConfig( │ │ device=device(type='cpu'), │ │ max_epochs=9, │ │ log_every_n_steps=100, │ │ eval_every_n_steps=4, │ │ step_scheduler_on_batch_or_epoch='epoch', │ │ use_amp=False, │ │ autocast_config={'enabled': False, 'dtype': None, 'cache_enabled': None}, │ │ scaler_config={ │ │ │ 'enabled': False, │ │ │ 'init_scale': 65536.0, │ │ │ 'growth_factor': 2.0, │ │ │ 'backoff_factor': 0.5, │ │ │ 'growth_interval': 2000 │ │ }, │ │ gradient_accumulation_steps=1, │ │ clip_grad_norm={'max_norm': 1.0, 'norm_type': 2.0, 'error_if_nonfinite': False, 'foreach': None}, │ │ apply_weight_decay_to_different_param_groups=False, │ │ save_dir='./data/adder/checkpoints/2024-12-17_09-43-55', │ │ save_every_epoch=False, │ │ save_best_only=True, │ │ monitor='valid_this_epoch_average_loss', │ │ mode='min' │ ), │ generator=GeneratorConfig(max_tokens=4, temperature=1.0, greedy=True, top_k=None, top_p=None), │ distributed=DistributedConfig( │ │ log_dir='logs_distributed', │ │ log_level=20, │ │ log_on_master_or_all=True, │ │ master_addr='localhost', │ │ master_port='29500', │ │ nnodes=1, │ │ nproc_per_node=1, │ │ node_rank=0, │ │ world_size=1, │ │ backend='gloo', │ │ init_method='env://' │ ) )
Reproducibility#
Reproducibility in deep learning ensures that experiments can be repeated with identical results, critical for verifying research findings and deploying reliable models. Distributed training introduces complexity because it involves multiple computation units which may not synchronize their random states perfectly. If training is paused and resumed, ensuring each unit starts with the correct seed to reproduce the exact computational path becomes challenging. To address this, one can find more sophisticated examples in libraries like Composer, where the whole library’s core is built around training deep neural nets in any environment (distributed or not) with reproducibility in mind.
References
print(get_field_annotations(func_or_method = seed_all)[0])
print("\n")
print(inspect.getdoc(seed_all))
seed_all(composer.global_.seed, seed_torch=True, set_torch_deterministic=False)
[('seed', <class 'int'>, 1992), ('seed_torch', <class 'bool'>, True), ('set_torch_deterministic', <class 'bool'>, True)]
Seeds all relevant random number generators to ensure reproducible
outcomes. Optionally seeds PyTorch and activates deterministic
behavior in PyTorch based on the flags provided.
Parameters
----------
seed : int, default=1992
The seed number for reproducibility.
seed_torch : bool, default=True
If True, seeds PyTorch's RNGs.
set_torch_deterministic : bool, default=True
If True, activates deterministic mode in PyTorch.
Returns
-------
seed : int
The seed number used for reproducibility.
42
Vocabulary#
vocabulary = AdderVocabulary.from_tokens(tokens=constants.TOKENS, num_digits=constants.NUM_DIGITS) # type: ignore[attr-defined]
token_to_index = vocabulary.token_to_index
index_to_token = vocabulary.index_to_token
vocab_size = vocabulary.vocab_size
pprint(token_to_index)
pprint(index_to_token)
pprint(vocab_size)
{ │ '0': 0, │ '1': 1, │ '2': 2, │ '3': 3, │ '4': 4, │ '5': 5, │ '6': 6, │ '7': 7, │ '8': 8, │ '9': 9, │ '+': 10, │ '*': 11, │ '-': 12, │ '=': 13, │ '<BOS>': 14, │ '<EOS>': 15, │ '<PAD>': 16, │ '<UNK>': 17 }
{ │ 0: '0', │ 1: '1', │ 2: '2', │ 3: '3', │ 4: '4', │ 5: '5', │ 6: '6', │ 7: '7', │ 8: '8', │ 9: '9', │ 10: '+', │ 11: '*', │ 12: '-', │ 13: '=', │ 14: '<BOS>', │ 15: '<EOS>', │ 16: '<PAD>', │ 17: '<UNK>' }
18
Assign vocab_size
to composer.model
because we don’t want to hardcode
vocab_size
beforehand, and want to derive concrete values from the
Vocabulary
object.
try:
composer.model.vocab_size = vocab_size
except AttributeError as err:
LOGGER.error(err)
[2024-12-17 09:43:55] ERROR _Missing instances are immutable 2890644827.py:4
Ah okay haha, this is the price of writing overly complex and useless code to look fancy and you end up a mess. Anyways, we will handle this later on where we can explicitly instantiate the model config class.
Tokenization#
tokenizer = AdderTokenizer(vocabulary=vocabulary)
assert tokenizer.vocabulary.token_to_index == token_to_index
assert tokenizer.vocabulary.index_to_token == index_to_token
pprint(tokenizer.encode("1"))
[14, 1, 15]
sequence = "15+57=072"
sequences = ["15+57=072", "01+02=003"]
encoded_sentence = tokenizer.encode(sequence)
print(f"Encoded sentence: {encoded_sentence}")
decoded_sentence = tokenizer.decode(encoded_sentence)
print(f"Decoded sentence: {decoded_sentence}")
Encoded sentence: [14, 1, 5, 10, 5, 7, 13, 0, 7, 2, 15]
Decoded sentence: 15+57=072
encoded_sentences = tokenizer.encode_batch(sequences) # type: ignore[attr-defined]
print(f"Encoded sentences: {encoded_sentences}")
decoded_sentences = tokenizer.decode_batch(encoded_sentences) # type: ignore[attr-defined]
print(f"Decoded sentences: {decoded_sentences}")
Encoded sentences: [[14, 1, 5, 10, 5, 7, 13, 0, 7, 2, 15], [14, 0, 1, 10, 0, 2, 13, 0, 0, 3, 15]]
Decoded sentences: ['15+57=072', '01+02=003']
Dataset#
Create Dataset#
def pad_number(num: int, length: int) -> str:
"""
Pad numbers with zeros in front so that they have uniform length.
Note, if a + b = c and num digits allowed to add is 2, then for
a and b we always pad to length 2, but for c we always pad to length 3.
Example
-------
6 + 90 = 96 -> 06 + 90 = 096
Parameters
----------
num : int
Number to be padded.
num_digits : int
Length of the resulting padded number string.
Returns
-------
str
Padded number string.
"""
return str(num).zfill(length)
def equation_to_string(a: int, b: int, c: int, num_digits: int) -> str:
"""
Formats the addition equation as a string.
Parameters
----------
a : int
First addend.
b : int
Second addend.
c : int
Sum of a and b.
num_digits : int
Number of digits each number in the equation should have.
Returns
-------
str
Formatted equation string.
"""
padded_a = pad_number(a, num_digits)
padded_b = pad_number(b, num_digits)
padded_c = pad_number(c, num_digits + 1) # note the padding here!
return f"{padded_a}+{padded_b}={padded_c}"
def decode_equation(vocab: AdderVocabulary, equation: torch.Tensor | List[int], show_special_tokens: bool = False) -> str:
"""
Convert an equation in list format to string format.
Parameters
----------
equation : List[int]
The equation in list format.
Returns
-------
str
The equation in string format.
"""
if isinstance(equation, torch.Tensor):
equation = equation.tolist()
UNK = vocab.token_to_index[vocab.UNK]
decoded_equation = "".join([str(index_to_token.get(x, UNK)) for x in equation])
if show_special_tokens:
return decoded_equation
return decoded_equation.replace("<BOS>", "").replace("<EOS>", "").replace("<PAD>", "").replace("<UNK>", "")
def batch_decode_equation(vocab: AdderVocabulary, equations: torch.Tensor | List[List[int]]) -> List[str]:
decoded_equations = []
for equation in equations:
decoded_equation = decode_equation(vocab, equation)
decoded_equations.append(decoded_equation)
return decoded_equations
def encode_equation(vocab: AdderVocabulary, equation: str, num_digits: int, device: torch.device) -> torch.Tensor:
"""
Convert an equation (up to the equal sign in it) in string format to a list.
Parameters
----------
equation : str
The equation in string format.
num_digits : int
Number of digits each number in the equation should have.
device : torch.device
The device to which the tensor should be sent.
Returns
-------
torch.Tensor
The equation in list format as a tensor.
"""
plus_idx = equation.index(vocab.ADD)
equal_idx = equation.index(vocab.EQUAL)
BOS = vocab.token_to_index[vocab.BOS]
UNK = vocab.token_to_index[vocab.UNK]
a = pad_number(int(equation[:plus_idx]), num_digits)
b = pad_number(int(equation[plus_idx + 1:equal_idx]), num_digits)
new_equation = f"{a}+{b}="
return torch.tensor(
[BOS] + [token_to_index.get(n, UNK) for n in new_equation],
dtype=torch.int
).to(device)
def create_add_dataset(
vocab: AdderVocabulary, num_digits: int, dataset_size: int, rng_seed: int = 1337
) -> Tuple[List[torch.Tensor], List[str]]:
BOS = vocab.token_to_index[vocab.BOS]
EOS = vocab.token_to_index[vocab.EOS]
UNK = vocab.token_to_index[vocab.UNK]
rng = torch.Generator()
rng.manual_seed(rng_seed)
max_num = 10**num_digits - 1
dataset_str = []
for _ in range(dataset_size):
a = torch.randint(low=0, high=max_num + 1, size=(1,), generator=rng).item()
b = torch.randint(low=0, high=max_num + 1, size=(1,), generator=rng).item()
c = a + b
equation = equation_to_string(a, b, c, num_digits)
dataset_str.append(equation)
dataset_tensor = [
torch.tensor([BOS] + [token_to_index.get(n, UNK) for n in x] + [EOS])
for x in dataset_str
]
return dataset_tensor, dataset_str
dataset_tensor, dataset_str = create_add_dataset(vocab=vocabulary, num_digits=2, dataset_size=4)
pprint(dataset_tensor)
pprint(dataset_str)
[ │ tensor([14, 1, 5, 10, 5, 7, 13, 0, 7, 2, 15]), │ tensor([14, 9, 2, 10, 0, 0, 13, 0, 9, 2, 15]), │ tensor([14, 9, 5, 10, 5, 3, 13, 1, 4, 8, 15]), │ tensor([14, 1, 5, 10, 1, 0, 13, 0, 2, 5, 15]) ]
['15+57=072', '92+00=092', '95+53=148', '15+10=025']
print(f"Decoded equation: {decode_equation(vocabulary, dataset_tensor[0])}")
assert (
decode_equation(vocabulary, dataset_tensor[0])
== dataset_str[0]
== decode_equation(vocabulary, [15, 1, 5, 10, 5, 7, 13, 0, 7, 2, 14])
)
Decoded equation: 15+57=072
if we encode equation, we can encode up to equal sign like below.
print(f"Encoded equation: {encode_equation(vocabulary, dataset_str[0], num_digits=2, device=composer.trainer.device)}")
torch.testing.assert_close(
encode_equation(vocabulary, dataset_str[0], num_digits=2, device=composer.trainer.device),
torch.tensor([14, 1, 5, 10, 5, 7, 13], dtype=torch.int32),
)
Encoded equation: tensor([14, 1, 5, 10, 5, 7, 13], dtype=torch.int32)
Uncomment the below code to generate the dataset into a text file and yes, I am lazy to add a config variable for whether to generate the dataset or not.
# dataset, dataset_str = create_add_dataset(vocab, self.num_digits, self.dataset_size)
# write dataset_str to a file
# with open("dataset_str.txt", "w") as f:
# for item in dataset_str:
# f.write("%s\n" % item)
Encoding Strategy Overview#
Our strategy for encoding arithmetic expressions is pretty self-explanatory,
where given a string D1 + D2 = D3
, we encode it as <BOS>D1+D2=0D3<EOS>
.
However, this is verbose for clarity sake. In fact, Karpathy’s encoding strategy
simplifies arithmetic expressions by concatenating the digits of operands and
the result into a single string without explicit symbols for operations or
equality. This method relies on a fixed number of digits (num_digits
) for
operands, which streamlines the model’s interpretation of the sequence. For
example, if num_digits
is set to 2, every encoded expression is structured to
follow a predictable pattern: the first two digits represent the first operand,
the next two digits represent the second operand, and the final digits are
encoded as 3 digits because the max sum of two 2-digit numbers is 199, which is
3 digits. The digits of the result are encoded in reverse order. This
counterintuitive approach is designed to align with the GPT model’s learning
algorithm, facilitating easier learning of the addition operation by mimicking
the traditional right-to-left calculation process in addition.
To illustrate, let’s examine the encoding of arithmetic expressions with
num_digits=2
:
For the expression 6 + 39 = 45
, we have the following:
The first two digits
06
represent the number 6, zero-padded to adhere to thenum_digits=2
requirement.The next two digits
39
represent the number 39, already fitting the digit requirement.The final part
054
represents the result 45, reversed to54
and preceded by a zero to maintain the total length of \(2n + (n + 1) = 7 \) digits fornum_digits=2
.
Constructing PyTorch Dataset#
create_directory(composer.data.dataset_dir)
download_file(url=composer.data.dataset_url, output_path=composer.data.dataset_path)
% Total % Received % Xferd Average Speed Time Time Time Current
Dload Upload Total Spent Left Speed
0 0 0 0 0 0 0 0 --:--:-- --:--:-- --:--:-- 0
100 97k 100 97k 0 0 635k 0 --:--:-- --:--:-- --:--:-- 638k
with open(composer.data.dataset_path, "r") as file:
sequences = [line.strip() for line in file]
dataset = AdderDataset(data=sequences, tokenizer=tokenizer)
pprint(next(iter(dataset)))
( │ tensor([14, 1, 5, 10, 5, 7, 13, 0, 7, 2]), │ tensor([16, 16, 16, 16, 16, 16, 0, 7, 2, 15]), │ tensor([True, True, True, True, True, True, True, True, True, True]), │ tensor([[ True, False, False, False, False, False, False, False, False, False], │ │ [ True, True, False, False, False, False, False, False, False, False], │ │ [ True, True, True, False, False, False, False, False, False, False], │ │ [ True, True, True, True, False, False, False, False, False, False], │ │ [ True, True, True, True, True, False, False, False, False, False], │ │ [ True, True, True, True, True, True, False, False, False, False], │ │ [ True, True, True, True, True, True, True, False, False, False], │ │ [ True, True, True, True, True, True, True, True, False, False], │ │ [ True, True, True, True, True, True, True, True, True, False], │ │ [ True, True, True, True, True, True, True, True, True, True]]) )
Construct Batches, Collate Function and DataLoader#
We first reverse engineer what our dataset is returning. The disclaimer here is
that for decoder only models like GPT, many people often omit the padding mask
since all the samples \(\mathbf{x}\) are chunked to sequence/context length of
window size \(T\), and future masks are usually handled within the Attention
class since we will never attend to the future tokens. However, for the sake of
clarity, we will include the padding and future mask in the dataset (i.e.
actually it is for the sake of my own understanding when I started to implement
decoder from scratch).
input, target, target_padding_mask, future_mask = next(iter(dataset))
Input and Target#
I think if you’ve read my section here, then we would easily see that given an input sequence \(\mathbf{x}\), the target sequence \(\mathbf{y}\) is simply the input sequence \(\mathbf{x}\) shifted by one time step to the left.
print(f"Input : {input}")
print(f"Target: {target}")
Input : tensor([14, 1, 5, 10, 5, 7, 13, 0, 7, 2])
Target: tensor([16, 16, 16, 16, 16, 16, 0, 7, 2, 15])
Target Padding Mask#
When you’re dealing with sequences of different lengths, you pad the shorter
sequences with a special token PAD
(usually \(0\) or \(-100\)) to make them the
same length as the longest one in the batch. These paddings should not
contribute to the model’s learning, so you need to mask them out. In practice,
you’ll often see a mask argument in Attention
layers in PyTorch where if
True
, the attention scores are set to -inf
for the padded positions so that
these positions become zero after the softmax operation, thereby not
contributing to the weighted sum of the input sequence.
In a decoder-only model like GPT, the input sequence is essentially the target. The model aims to generate tokens that come after the given input, treating it as the “history” or “context” for the task of text generation. Unlike encoder-decoder models like the original Transformer, where the encoder processes a source sequence and the decoder generates a target sequence, a decoder-only model works solely with what would traditionally be considered the target sequence.
Consequently, although the terminology “target padding mask” might seem more intuitive in the context of encoder-decoder models, where the distinction between source (input) and target (output) sequences is clear. The distinction is blurred in decoder-only models like GPT as the model processes input to predict the next token in a sequence. Here, the source is essentially the target at different stages of processing: the model uses previous tokens (source) to predict the next token (target). However, during my implementation, I was mainly referring to transformer models that use encoder-decoder architecture, and the terminology therefore stemmed from that context.
The definition of a target padding mask is a binary mark that ignores pad-tokens in the source input (in decoder only model, the source is the target). And the shape is \((\mathcal{B}, T)\).
Let’s illustrate the target padding mask with an example. Suppose we have a batch of sequences with different lengths:
target_batch = [
[5, 7, 9],
[8, 6],
[3, 12, 4, 11, 17],
[2, 1, 4, 5],
]
pprint(target_batch)
[[5, 7, 9], [8, 6], [3, 12, 4, 11, 17], [2, 1, 4, 5]]
If we try to “batch” these sequences, PyTorch would throw an error indicating that you need all sequences to have the same length.
try:
target_batch = torch.tensor(target_batch, dtype=torch.int64)
except ValueError as err:
LOGGER.error(err)
ERROR expected sequence of length 3 at dim 1 (got 2) 1205213247.py:4
To address this issue, we could pad the sequences to the same length and create a mask to indicate
which positions are padded. We pad the shorter sequences with a special token PAD
to make them the same length as the longest one in the batch.
PAD = vocabulary.token_to_index[vocabulary.PAD]
max_len = max(len(seq) for seq in target_batch)
target_batch = [seq + [PAD] * (max_len - len(seq)) for seq in target_batch]
pprint(target_batch)
target_batch = torch.tensor(target_batch, dtype=torch.int64)
pprint(target_batch)
[[5, 7, 9, 16, 16], [8, 6, 16, 16, 16], [3, 12, 4, 11, 17], [2, 1, 4, 5, 16]]
tensor([[ 5, 7, 9, 16, 16], │ │ [ 8, 6, 16, 16, 16], │ │ [ 3, 12, 4, 11, 17], │ │ [ 2, 1, 4, 5, 16]])
batch_size, seq_len = target_batch.size()
target_padding_mask = target_batch != PAD
pprint(target_padding_mask)
assert target_padding_mask.size() == (batch_size, seq_len) == (4, 5)
tensor([[ True, True, True, False, False], │ │ [ True, True, False, False, False], │ │ [ True, True, True, True, True], │ │ [ True, True, True, True, False]])
Of course, we would need a batch of these masks, so we would have a shape of \((\mathcal{B}, T)\) like mentioned above. As we will see later, we will still need to broadcast the shape to \((\mathcal{B}, 1, T, T)\) to match the shape of the attention scores.
Theoretically speaking, it is possible for the sequence length \(T\) to vary
across samples \(\mathbf{x}\). However, we usually have the same length for all
samples in GPT, and in this particular case, we do know that each sample
necessarily have the same length by design. However, for the sake of
explanation, we note that in our Dataset
, it will only generate 1 single
sample data point and do not worry about different sequence length across other
samples in the dataset \(\mathcal{S}\), but in deep learning we train in
mini-batches \(\mathcal{B}\), and with different batch sizes we may encounter
issues (i.e. matrix multiplication may not work).
Future Mask#
In the decoder, each position can only attend to positions that come before it in the sequence to maintain the auto-regressive property. This is different from the encoder, where all positions can attend to all other positions.
The definition of future mask is basically a look-ahead mask to ensure that each
position only attends to positions before it in the sequence where we mask out
future positions (i.e., positions that come after the current position) so that
they don’t contribute to the current attention scores. Before the softmax
operation, we’ll mark these positions as -inf
so that they become zero after
the softmax operation - effectively zeroing out the attention scores for future
positions. What does zeroing out these masked logits actually does? Basically,
the attention mechanism can be thought of as a weighted average of all the
tokens in the input sequence. Each token is assigned a weight, with higher
weights indicating more relevance to the token under consideration. If a certain
token should not be considered at all (e.g., it’s a future token that should not
be visible to the current decoder step, or it’s a padding token), its weight
should be zero.
The shape of the future mask is \((T, T)\) for a target sequence/sample \(\mathbf{x}\) of length \(T\). Let’s see a concrete example to illustrate the future mask.
seq_len = 5
future_mask = torch.triu(torch.ones(seq_len, seq_len), diagonal=1)
future_mask = future_mask == 0
pprint(future_mask)
assert future_mask.size() == (seq_len, seq_len) == (5, 5)
tensor([[ True, False, False, False, False], │ │ [ True, True, False, False, False], │ │ [ True, True, True, False, False], │ │ [ True, True, True, True, False], │ │ [ True, True, True, True, True]])
Merge Padding and Future Masks#
We see from our decoder
implementation below, that one of the method is
creating the target masks. In other words, we are creating the target padding
masks and future masks, and merging them together.
1def create_target_masks(
2 self,
3 batch_size: int,
4 seq_len: int,
5 target_padding_masks: torch.BoolTensor | NotGiven = NOT_GIVEN,
6 future_masks: torch.BoolTensor | NotGiven = NOT_GIVEN,
7) -> torch.BoolTensor:
8 target_masks_shape = (batch_size, 1, seq_len, seq_len)
9 if target_padding_masks is NOT_GIVEN and future_masks is NOT_GIVEN:
10 target_padding_masks = cast(
11 torch.BoolTensor, construct_dummy_batch_target_padding_masks(batch_size, seq_len)
12 )
13 future_masks = cast(torch.BoolTensor, construct_dummy_batch_future_masks(batch_size, seq_len))
14
15 if target_padding_masks is NOT_GIVEN:
16 target_padding_masks = cast(
17 torch.BoolTensor, construct_dummy_batch_target_padding_masks(batch_size, seq_len)
18 )
19
20 if future_masks is NOT_GIVEN:
21 future_masks = cast(torch.BoolTensor, construct_dummy_batch_future_masks(batch_size, seq_len))
22
23 assert target_padding_masks.shape == future_masks.shape == target_masks_shape # type: ignore[union-attr]
24
25 return cast(
26 torch.BoolTensor,
27 torch.logical_and(cast(torch.Tensor, target_padding_masks), cast(torch.Tensor, future_masks)).bool(),
28 )
The purpose of applying logical_and
between target_padding_mask
and
future_mask
is to combine the constraints from both masks when calculating
self-attention scores in the transformer’s decoder. The target_padding_mask
is
designed to mask out the padding tokens in the input sequence, while the
future_mask
ensures that a given position cannot attend to future positions in
the sequence. By combining these masks, you can perform the necessary masking
for both padding and future tokens in a single step.
Here’s how it works:
target_padding_mask
: Masks out the padding tokens so that they don’t contribute to the attention calculations. True values mean “attend to this token,” and False values mean “ignore this token.”future_mask
: The future mask is created as a lower triangular matrix, where the lower triangle, including the diagonal, is filled with ones, and the upper triangle is filled with zeros. Masks out future tokens in a sequence so that a token at a given position can only attend to positions that come before it (and itself). True values mean “attend to this token,” and False values mean “ignore this token.”logical_and(target_padding_mask, future_mask)
: Combines the two masks. A True in the resulting mask means that the condition for both padding and future attention is satisfied.
By combining these two masks, the decoder obeys the autoregressive property,
ensuring it doesn’t see future tokens, while also ignoring padding tokens in the
input sequence. We may term it the target_mask
.
First Sample First Token#
target_padding_mask
has size of[4, 5]
.We zoom in to the first row (sample) which is of length 5.
This length 5 is the sequence length, which is
T, T, T, F, F
indicating the last 2 tokens being padded.
future_mask
has size of[5, 5]
.We note that this is indepedent of batch size. Each sample should have the same future mask shape of
[L, L]
.This
L=5
should necessary be same for the sequence length intarget_padding_mask
.
First, let’s consider one batch of 4 samples. What we do first is to broadcast
future_mask
to[4, 5, 5]
because we want each sample/row in the batch to have the same future mask. As shown below:
pprint(future_mask)
future_mask = future_mask.view(1, seq_len, seq_len).expand(size=(batch_size, -1, -1))
pprint(future_mask)
pprint(future_mask.shape)
tensor([[ True, False, False, False, False], │ │ [ True, True, False, False, False], │ │ [ True, True, True, False, False], │ │ [ True, True, True, True, False], │ │ [ True, True, True, True, True]])
tensor([[[ True, False, False, False, False], │ │ [ True, True, False, False, False], │ │ [ True, True, True, False, False], │ │ [ True, True, True, True, False], │ │ [ True, True, True, True, True]], │ │ │ │ [[ True, False, False, False, False], │ │ [ True, True, False, False, False], │ │ [ True, True, True, False, False], │ │ [ True, True, True, True, False], │ │ [ True, True, True, True, True]], │ │ │ │ [[ True, False, False, False, False], │ │ [ True, True, False, False, False], │ │ [ True, True, True, False, False], │ │ [ True, True, True, True, False], │ │ [ True, True, True, True, True]], │ │ │ │ [[ True, False, False, False, False], │ │ [ True, True, False, False, False], │ │ [ True, True, True, False, False], │ │ [ True, True, True, True, False], │ │ [ True, True, True, True, True]]])
torch.Size([4, 5, 5])
Now, we can zoom in to one particular sample since both
target_padding_mask
andfuture_mask
have the same first dimension of batch size.What is incomplete is that we need to broadcast
target_padding_mask
’s last dimension to have the same dimensions asfuture_mask
. This means we broadcast[4, 5]
to[4, 5, 5]
. But why?For simplicity, we slice the first same of both below.
The first row of the
future_mask
of the first sample isT, F, F, F, F
. This corresponds to what? This is the future mask of the first token in the sequence. Well, that is confusing, because it apparently have 5 elements, and has “information” of the other 4 tokens in the sequence. Let’s explain in details below:Regarding the first row of the
future_mask
in the first sample, which is[T, F, F, F, F]
, it might initially seem confusing why there are 5 elements. Each of these elements, in fact, corresponds to whether the first token can attend to other tokens at each respective position in the sequence. Here’s how to interpret it:The first element (
True
) indicates that the first token can attend to itself.The next four elements (
False
) specify that the first token should not attend to any of the future tokens in the sequence.
Consequently, what is the first token in the sequence of the
target_padding_mask
? Recall earlier we mentioned that the first sample’starget_padding_mask
isT, T, T, F, F
and therefore the first token in the sequence isT
.What do we want to achieve here? We want to make sure that the model does not attend to tokens in the sequence that are masked with
False
.In other words, the first token in the sequence of the first sample has
target_padding_mask
ofT
andfuture_masks
ofT, F, F, F, F
.We need to broadcast this
T
toT, T, T, T, T
to align withT, F, F, F, F
because? Because we need ensure that this first token in the sequence is also able to considered in relation to every other token in the sequence.So the first token is not a padded token, which is
T
, similarly, the first token needs to attend to itself at the first position, henceT
andT
giveT
. But for the secondT
in the now broadcastedtarget_padding_mask
, it is still representing the first token or?Broadcasting the first token’s
target_padding_mask
value ofT
to[T, T, T, T, T]
ensures that when this first token is being considered for attention computations, it is free to attend to any position, barring any restrictions set byfuture_mask
.Tricky: after broadcasting, each
T
in[T, T, T, T, T]
is still representing the first token. They indicate that when the first token is compared with any token in the sequence (including itself), it is not a padding token. The element-wiseAND
with thefuture_mask
then further refines this by restricting it from attending to future tokens.
pprint(target_padding_mask)
pprint(target_padding_mask[0])
target_padding_mask = target_padding_mask.view(batch_size, 1, seq_len).expand(size=(batch_size, seq_len, seq_len))
pprint(target_padding_mask)
pprint(target_padding_mask.shape)
tensor([[ True, True, True, False, False], │ │ [ True, True, False, False, False], │ │ [ True, True, True, True, True], │ │ [ True, True, True, True, False]])
tensor([ True, True, True, False, False])
tensor([[[ True, True, True, False, False], │ │ [ True, True, True, False, False], │ │ [ True, True, True, False, False], │ │ [ True, True, True, False, False], │ │ [ True, True, True, False, False]], │ │ │ │ [[ True, True, False, False, False], │ │ [ True, True, False, False, False], │ │ [ True, True, False, False, False], │ │ [ True, True, False, False, False], │ │ [ True, True, False, False, False]], │ │ │ │ [[ True, True, True, True, True], │ │ [ True, True, True, True, True], │ │ [ True, True, True, True, True], │ │ [ True, True, True, True, True], │ │ [ True, True, True, True, True]], │ │ │ │ [[ True, True, True, True, False], │ │ [ True, True, True, True, False], │ │ [ True, True, True, True, False], │ │ [ True, True, True, True, False], │ │ [ True, True, True, True, False]]])
torch.Size([4, 5, 5])
pprint(target_padding_mask[0])
pprint(future_mask[0])
pprint(target_padding_mask[0] & future_mask[0])
tensor([[ True, True, True, False, False], │ │ [ True, True, True, False, False], │ │ [ True, True, True, False, False], │ │ [ True, True, True, False, False], │ │ [ True, True, True, False, False]])
tensor([[ True, False, False, False, False], │ │ [ True, True, False, False, False], │ │ [ True, True, True, False, False], │ │ [ True, True, True, True, False], │ │ [ True, True, True, True, True]])
tensor([[ True, False, False, False, False], │ │ [ True, True, False, False, False], │ │ [ True, True, True, False, False], │ │ [ True, True, True, False, False], │ │ [ True, True, True, False, False]])
First Sample Fourth Token#
Now let’s look at another example—the 4th token in the sequence, where
target_padding_mask = [T, T, T, F, F]
and future_mask
is a lower triangular
matrix with True
s.
4th Token’s target_padding_mask: The 4th token has a value of
F
intarget_padding_mask
, indicating it’s a padding token.4th Row of future_mask: The 4th row in
future_mask
is[True, True, True, True, False]
. This means that if this token were not a padding token, it would be allowed to attend to all the previous tokens in the sequence and itself, but not to any future token.Broadcast target_padding_mask: To align
target_padding_mask
withfuture_mask
, we’d broadcastF
from thetarget_padding_mask
to[F, F, F, F, F]
. This way, when we consider the 4th token in relation to any other token in the sequence, it’s still marked as a padding token.Element-wise AND with future_mask: After broadcasting, you’d perform an element-wise AND between
[F, F, F, F, F]
and[True, True, True, True, False]
, resulting in[F, F, F, F, F]
.Interpretation: This effectively means that the 4th token won’t attend to any other token in the sequence, and no token will attend to it either, as it is a padding token.
So, the masks are doing their jobs correctly: the target_padding_mask
indicates whether each token is a padding token or not, and future_mask
dictates the “rules” of attention regarding what each token can attend to.
Combining them ensures that both conditions are met.
Further Add a Singleton Dimension in Target Masks#
Now both masks are of shape: (B, L, L)
but we need to add a singleton
dimension to the last dimension to make it (B, 1, L, L)
.
In deep learning frameworks like PyTorch, the dimensions of the tensors involved in operations like matrix multiplication or attention mechanisms often have specific semantic meanings. In the context of attention mechanisms, especially in the transformer architecture, the attention mask usually has a shape that is compatible with the attention logits for element-wise multiplication.
In the transformer model, the attention logits are often computed as a dot
product between query and key vectors, resulting in a tensor of shape
(Batch size, Num heads, Sequence length, Sequence length)
or (B, H, L, L)
.
Here, B
is the batch size, H
is the number of attention heads, and L
is
the sequence length.
To make the mask tensor compatible for element-wise operations with this 4D
tensor, it needs to have a shape that can be broadcasted to (B, H, L, L)
. A
mask of shape (B, 1, L, L)
fulfills this requirement.
The singleton dimension is added so that the mask can be easily broadcast to the
shape of the attention logits tensor during the computation. When a tensor with
shape (B, 1, L, L)
is element-wise multiplied with a tensor of shape
(B, H, L, L)
, the singleton dimension (the 1
) allows the mask to be used for
each attention head without explicitly replicating the mask H
times. This is
more memory-efficient and often faster.
Thus, adding a singleton dimension in masks is a preparatory step that allows for efficient element-wise operations later in the model’s forward pass.
target_padding_mask = target_padding_mask.unsqueeze(1)
pprint(target_padding_mask.shape)
future_mask = future_mask.unsqueeze(1)
pprint(future_mask.shape)
target_mask = target_padding_mask & future_mask
pprint(target_mask.shape)
torch.Size([4, 1, 5, 5])
torch.Size([4, 1, 5, 5])
torch.Size([4, 1, 5, 5])
Why mask our target in Adder?#
If you see the source code of how the AdderDataset
is constructed, you will
see that we masked out all the tokens before (and including) the equal sign.
For example, if our sequence is 12+97=109
, the input sequence will be
tokenized to the following:
input = [BOS, 1, 2, +, 9, 7, =, 1, 0, 9]
target = [1, 2, +, 9, 7, =, 1, 0, 9, EOS]
What our code below does is to mask out the tokens before the equal sign for the target sequence.
target = [MASK, MASK, MASK, MASK, MASK, MASK, 1, 0, 9, EOS]
def construct_target_tensor(self, input_sequence: torch.Tensor) -> torch.LongTensor:
target = input_sequence.clone()
where_equal_index = torch.where(input_sequence == self.equal_token_id)[0].item()
where_equal_index = int(where_equal_index) # to appease mypy lol
target[: where_equal_index + 1] = self.pad_token_id
return torch.LongTensor(target[1:])
Simply put, we do not care what the model predict for anything before the equal sign. By masking out (or ignoring) the tokens before the =, we are asking the model to “focus” on generating the correct answer after the equal sign.
Split to Train-Valid-Test#
batch_size = 256
composer.data.train_loader["batch_size"] = batch_size
composer.data.valid_loader["batch_size"] = batch_size
composer.data.test_loader["batch_size"] = batch_size
train_dataset, valid_dataset, test_dataset = split_dataset(
dataset=dataset, split=composer.data.split, seed=composer.global_.seed
)
train_size, valid_size, test_size = len(train_dataset), len(valid_dataset), len(test_dataset)
train_size, valid_size, test_size
(7000, 2000, 1000)
# max_seq_len is determined by 1+ num_digits + 1 + num_digits + 1 + num_digits + 1 + 1
# where the 1s represent BOS, Plus sign, Equal sign, the extra digit in the sum, EOS, respectively.
max_seq_len = 1 + 1 + 1 + 1 + 2 * composer.constants.NUM_DIGITS + (composer.constants.NUM_DIGITS + 1)
assert max_seq_len == composer.data.context_length
Create DataLoader#
train_loader = create_loader(
dataset=train_dataset,
loader_config=composer.data.train_loader,
collate_fn_config=composer.data.collate_fn,
)
valid_loader = create_loader(
dataset=valid_dataset,
loader_config=composer.data.valid_loader,
collate_fn_config=composer.data.collate_fn,
)
test_loader = create_loader(
dataset=test_dataset,
loader_config=composer.data.test_loader,
collate_fn_config=composer.data.collate_fn,
)
The collate_fn
defines how to combine these variable-length samples into a
batch. This usually involves padding the sequences in the batch to a common
length, which is typically the length of the longest sequence in the batch. Note
here the padding in collate is “redundant” since in our earlier code we ensured
that all sample has same number of characters by way of padding zeros in front.
For example, 23 + 3 =26
will become 23 + 03 = 026
. Consequently, all samples
in the mini-batch will have same length by definition.
torch.manual_seed(composer.global_.seed)
batch_index = 0
for batch in train_loader:
# Each batch is a tuple containing all elements for the batch
inputs_padded, targets_padded, padding_masks_padded_and_expanded, future_masks_expanded = batch
# Print the length of each component in the batch
print("Batch Size:", len(inputs_padded))
# Now you can print shapes or other properties of each batch element
print("Inputs Shape:", inputs_padded.shape)
print("Targets Shape:", targets_padded.shape)
# Decoding and other processing can be done here
# For example, decoding the first sequence in the batch
print("Decoded First Equation/Sample of the Batch:", decode_equation(vocabulary, inputs_padded[0].tolist()))
print("-" * 80)
batch_index += 1
if batch_index == 4: break
Batch Size: 256
Inputs Shape: torch.Size([256, 10])
Targets Shape: torch.Size([256, 10])
Decoded First Equation/Sample of the Batch: 31+04=035
--------------------------------------------------------------------------------
Batch Size: 256
Inputs Shape: torch.Size([256, 10])
Targets Shape: torch.Size([256, 10])
Decoded First Equation/Sample of the Batch: 37+49=086
--------------------------------------------------------------------------------
Batch Size: 256
Inputs Shape: torch.Size([256, 10])
Targets Shape: torch.Size([256, 10])
Decoded First Equation/Sample of the Batch: 47+26=073
--------------------------------------------------------------------------------
Batch Size: 256
Inputs Shape: torch.Size([256, 10])
Targets Shape: torch.Size([256, 10])
Decoded First Equation/Sample of the Batch: 53+05=058
--------------------------------------------------------------------------------
Model#
We have went into extensive details on the implementation of the decoder in the implementation section. We will not repeat the concepts here, instead we will just compile the model with the configurations.
# Create individual component configurations
masked_self_attention_mha_config = MultiHeadedAttentionConfig(
attention=ScaledDotProductAttention(),
d_model=128, H=4, dropout=0.1
)
feed_forward_config = PositionwiseFeedForwardConfig(
d_model=128, d_ff=256, activation=nn.GELU(approximate="tanh"), dropout=0.1, bias=True
)
add_norm_config_1 = AddNormConfig(feature_dim=128, dropout=0.1)
add_norm_config_2 = AddNormConfig(feature_dim=128, dropout=0.1)
# Create DecoderBlockConfig
decoder_block_config = DecoderBlockConfig(
masked_self_attention_mha=masked_self_attention_mha_config,
feed_forward=feed_forward_config,
add_norm_1=add_norm_config_1,
add_norm_2=add_norm_config_2,
)
# Create the overall DecoderConfig
model_config = DecoderConfig(
d_model=128,
vocab_size=vocab_size,
context_length=max_seq_len,
num_decoder_blocks=2,
dropout=0.1,
decoder_block=decoder_block_config,
)
model = GPTDecoder(model_config)
model = model.to(device=composer.trainer.device, dtype=next(model.parameters()).dtype, non_blocking=True)
model_size = model.total_trainable_parameters
print(f'model_size: {model_size}, train_set_size: {train_size}')
composer.model = model_config
model_size: 270226, train_set_size: 7000
Training Paradigm#
Here, we would list some of the training paradigms that we would be using in this project.
Optimizer#
We start off by defining the optimizer for GPT-2. A common choice used is the Adam [Kingma and Ba, 2014] or AdamW [Loshchilov and Hutter, 2017]. We conveniently take the configuration provided in Karpathy’s nanoGPT.
Furthermore, we briefly mention that Karpathy applies weight decay to different
parameter groups - which is quite a common practice. As we can see from the code
below, we define whitelisted and blacklisted modules that we want to apply
weight decay to. The whitelist modules are nn.Linear
and the blacklist modules
are nn.LayerNorm
, nn.Embedding
.
Weight decay, which is basically L2 regularization penalizes the square of the weights, encouraging smaller weight values. This can lead to a “spreading out” effect, as it discourages the model from relying too heavily on a small number of input features, promoting a more even distribution of weight values and, by extension, a more balanced consideration of input dimensions. This regularization technique is particularly beneficial for layers that perform matrix multiplication, as it helps in ensuring that the model utilizes a broader range of input features rather than becoming overly dependent on a few dominant ones. We can find more intuition in the discussion Why not perform weight decay on layernorm/embedding?, Weight decay in the optimizers is a bad idea (especially with BatchNorm) and Weight decay exclusions (Karpathy).
1def apply_weight_decay_to_different_param_groups(
2 model: nn.Module, weight_decay: float
3) -> List[Dict[Literal["params", "weight_decay"], List[torch.nn.Parameter] | float]]:
4 decay: Set[str] = set()
5 no_decay: Set[str] = set()
6 whitelist_weight_modules: Tuple[Type[nn.Module], ...] = (nn.Linear,)
7 blacklist_weight_modules: Tuple[Type[nn.Module], ...] = (nn.LayerNorm, nn.Embedding, LayerNorm)
8
9 for module_name, module in model.named_modules():
10 for parameter_name, _parameter in module.named_parameters():
11 full_parameter_name = f"{module_name}.{parameter_name}" if module_name else parameter_name
12 if parameter_name.endswith("bias"):
13 # biases of all modules are not decayed
14 no_decay.add(full_parameter_name)
15 elif parameter_name.endswith("weight") and isinstance(module, whitelist_weight_modules):
16 # weights of whitelisted modules are decayed
17 decay.add(full_parameter_name)
18 elif parameter_name.endswith("in_proj_weight"):
19 # MHA projection layer, does not exist in my implementation
20 decay.add(full_parameter_name)
21 elif parameter_name.endswith("weight") and isinstance(module, blacklist_weight_modules):
22 # weights of blacklisted modules are not decayed
23 no_decay.add(full_parameter_name)
24 elif (parameter_name.endswith("gamma") or parameter_name.endswith("beta")) and isinstance(
25 module, LayerNorm
26 ):
27 # weights of LayerNorm modules are not decayed
28 # TODO: why do I need to do this is because my custom LayerNorm has gamma and beta
29 # as their "weight" and "bias" attributes, respectively.
30 no_decay.add(full_parameter_name)
31 elif parameter_name.endswith("pos_embed"):
32 no_decay.add(full_parameter_name)
33
34 param_dict = {parameter_name: parameter for parameter_name, parameter in model.named_parameters()} # noqa: C416
35 inter_params = decay & no_decay
36 union_params = decay | no_decay
37 assert not inter_params, f"Parameters {inter_params} are in both decay and no_decay sets."
38 assert not (
39 param_dict.keys() - union_params
40 ), f"Parameters {param_dict.keys() - union_params} were not categorized."
41
42 optim_groups: List[Dict[Literal["params", "weight_decay"], List[torch.nn.Parameter] | float]] = [
43 {"params": [param_dict[parameter_name] for parameter_name in sorted(decay)], "weight_decay": weight_decay},
44 {"params": [param_dict[parameter_name] for parameter_name in sorted(no_decay)], "weight_decay": 0.0},
45 ]
46
47 return optim_groups
We won’t go into too much technical rigour on the optimizer, but note that more modern variations exist, for instance DecoupledAdamW, which furthers decouple the weight decay term \(\lambda\) from the learning rate, as well RAdam [Liu et al., 2019], which is intended to address bias correction factors leading to higher variance in the adaptive learning rate for the initial training iterations.
To this end, we create the optimizer in code as follows, noting that we would not use the exact same configuration as Karpathy, but rather use what is deemed fit for the case at hand.
pprint(composer.optimizer)
optimizer_config_cls = OPTIMIZER_REGISTRY[composer.optimizer.name]
optimizer_pydantic_config = optimizer_config_cls(**composer.optimizer.model_dump(mode="python"))
pprint(optimizer_pydantic_config)
AdamConfig(name='torch.optim.Adam', lr=0.2, betas=(0.9, 0.98), eps=1e-09, weight_decay=0.0)
AdamConfig(name='torch.optim.Adam', lr=0.2, betas=(0.9, 0.98), eps=1e-09, weight_decay=0.0)
assert hasattr(composer.optimizer, "weight_decay")
optimizer = optimizer_pydantic_config.build(
params=apply_weight_decay_to_different_param_groups(
model=model, weight_decay=composer.optimizer.weight_decay
)
)
pprint(optimizer)
Adam ( Parameter Group 0 │ amsgrad: False │ betas: (0.9, 0.98) │ capturable: False │ differentiable: False │ eps: 1e-09 │ foreach: None │ fused: None │ lr: 0.2 │ maximize: False │ weight_decay: 0.0 Parameter Group 1 │ amsgrad: False │ betas: (0.9, 0.98) │ capturable: False │ differentiable: False │ eps: 1e-09 │ foreach: None │ fused: None │ lr: 0.2 │ maximize: False │ weight_decay: 0.0 )
Learning Rate Scheduler#
Motivation#
In training deep neural networks, learning rate is definitely one of the most important parameter to tune. Optimization algorithms like Adam and SGD tell us how the weights \(\boldsymbol{\theta} \in \boldsymbol{\Theta}\) should be updated, but the learning rate \(\eta\) tells us the rate at which the weights are being updated.
Theoretically and empircally, the magnitude of the learning rate \(\eta\) can have a significant impact on the training process. If the learning rate is too large, we might experience divergence, on the other hand, if the learning rate is too small, the model might take longer to converge or might get stuck in a local minima. The condition number of the problem also impacts optimization efficiency, as discussed in the momentum section, where the concept can be understood as the ratio between the smallest and largest changes possible in response to adjustments in different directions of the parameter space, reflecting the variance in sensitivity across these directions[^1] [Zhang et al., 2023]. As we progress through the training steps, it is also equally important to apply a learning rate scheduler to adjust (may not be monotonous decay) the learning rate discriminatively.
In the paper SGDR: Stochastic Gradient Descent with Restarts by Loshchilov and Hutter, they introduced an heuristic that relies on the empirical observation that we can improve the convergence of the model (usually in ill-conditioned situations) if we want follow an annealing process over the learning rate. This means that at the beginning of training, we do not want to decrease the learning too drastically. My (potentially wrong) intuition is that this may allow the model to consider exploring a larger parameter space without too much constraints than if we were to rapidly decrease the learning rate. The authors further claim that as we progress towards the end of the training, we would want to “fine-tune” the model parameters with a very small learning rate, as it could potentially help “refine” the solution space to find a “more optimal” set of parameters [Loshchilov and Hutter, 2016]. This idea naturally lands us to using cosine function because the cosine curve starts with a gentle slope, which coincides with the idea of gradual decrease in learning rate in the beginning, and the cosine curve naturally flattens and approaches zero towards the end as it reaches the end of its cycle, which again coincides with the idea of fine-tuning the model parameters with a very small learning rate.
Consequently, a cosine decaying scheduler has the below function form for learning rates in the range \(t \in [0, T]\):
Here \(\eta_0\) is the initial learning rate, \(\eta_T\) is the target rate at time \(T\). Furthermore, for \(t>T\) we simply pin the value to \(\eta_T\) without increasing it again. \(T\) represents the end of the learning rate annealing phase rather than the absolute end of training. It’s the point in time when the learning rate reaches \(\eta_T\), the target rate, and beyond which the learning rate is maintained constant at \(\eta_T\).
During \(0 \leq t < T\): The learning rate \(\eta_t\) is actively adjusted according to the cosine annealing formula. It transitions from the initial learning rate \(\eta_0\) towards the target rate \(\eta_T\), following a half-cosine wave.
For \(t \geq T\): The learning rate is set to \(\eta_T\) and no longer changes. This doesn’t necessarily mean that training must stop at \(t = T\). Training can continue beyond \(T\) with the learning rate fixed at \(\eta_T\).
In code, we can observe the behavior of the cosine annealing scheduler as follows:
from __future__ import annotations
from typing import Any, List
import matplotlib.pyplot as plt
import torch
from torch.optim import Optimizer
from torch.optim.lr_scheduler import CosineAnnealingLR, _LRScheduler
def get_learning_rates(optimizer: Optimizer, scheduler: _LRScheduler, steps: int) -> List[float]:
lrs = []
for _ in range(steps):
lrs.append(optimizer.param_groups[0]["lr"])
optimizer.step()
scheduler.step()
return lrs
def plot_learning_rates(
lrs: List[float], title: str, marker: str = "o", ax: plt.Axes | None = None, **kwargs: Any
) -> None:
ax = ax or plt.gca()
ax.plot(lrs, label=title, marker=marker, **kwargs)
ax.set_title(title)
ax.set_xlabel("Step")
ax.set_ylabel("Learning Rate")
ax.legend()
def main() -> None:
initial_lr = 0.1
eta_min = 0
steps = 100
model = torch.nn.Linear(2, 1)
optimizer = torch.optim.SGD(model.parameters(), lr=initial_lr)
scheduler_non_cyclic = CosineAnnealingLR(optimizer, T_max=steps, eta_min=eta_min)
lrs_non_cyclic = get_learning_rates(optimizer, scheduler_non_cyclic, steps)
optimizer = torch.optim.SGD(model.parameters(), lr=initial_lr)
scheduler_cyclic = CosineAnnealingLR(optimizer, T_max=steps // 8, eta_min=eta_min)
lrs_cyclic = get_learning_rates(optimizer, scheduler_cyclic, steps)
# Plotting
fig, axes = plt.subplots(1, 2, figsize=(12, 4))
plot_learning_rates(lrs_non_cyclic, 'Non-Cyclic Cosine Annealing', ax=axes[0])
plot_learning_rates(lrs_cyclic, 'Cyclic Cosine Annealing', ax=axes[1])
plt.tight_layout()
plt.show()
main()
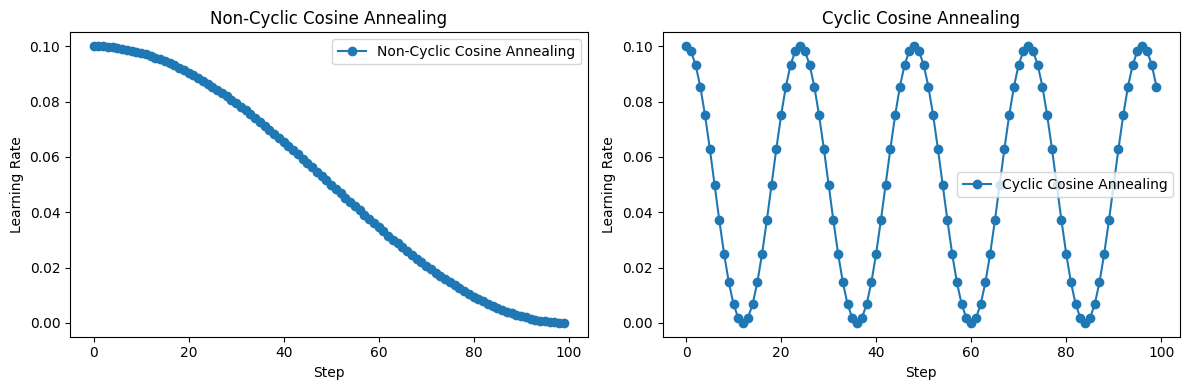
Warmup#
Our motivation would have ended here, but in practice, we often see that the cosine annealing scheduler is often combined with a warmup phase. In Fig. 18, we can see that the loss curve is relatively smooth and converges way better than the ones without warmup.
Fig. 4 Training loss v.s. # of iterations of Transformers on the De-En IWSLT’14 dataset.#
Image Credit: ON THE VARIANCE OF THE ADAPTIVE LEARNING RATE AND BEYOND
It might be worth having some intuition on why warmup works so well in practice, and in particular, in language models like Transformers.
Firstly, the RAdam paper suggests warmup works as a variance reduction technique, which overcomes the problem of bias correction factors in optimizers like Adam, where having these bias correction factors would lead to larger variance in the adaptive learning rate during the initial training iterations [Lippe, 2023]. More concretely, Adam estimates the first and second moments of the gradient to change the learning rate of each individual parameter (hence adaptive) and having high variance between adaptive learning rates may de-stablize the training. If we don’t want to swap out Adam, then this calls for a warmup phase to stabilize the learning rate and reduce the variance in the early stages of training.
Secondly, language models like Transformers use iteratively applied Layer Normalization across layers can lead to very high gradients during the first iterations, which can be solved by using Pre-Layer Normalization (similar to Pre-Activation ResNet), which applies normalization before the layer’s main operations, contributing to gradient stabilization and reducing the necessity for a warm-up phase, or replacing Layer Normalization by other techniques (Adaptive Normalization, Power Normalization) [Lippe, 2023].
However, even though there are solutions to the problem, certain setups still use the Adam optimizer, and therefore warmup is still a simple and effective technique to stabilize the learning rate in the early stages of training - solving the afforementioned problems (i.e. stabilize the bias correction factors, moving averages of gradients and squared gradients).
To this end, we end our discussion on the motivation behind 1) using cosine annealing schedulers and 2) using warmup phases, often coupled with cosine annealing schedulers. In what follows, we will provide a more formal definition of the cosine annealing scheduler with warmup, and provide a running example to illustrate the behavior of the scheduler.
Definition#
The CosineAnnealingWithWarmupScheduler
decays the learning rate \(\eta\)
according to the decreasing part of a cosine curve, with an initial warmup
\(t_{\text{warmup}}\).
This scheduler modulates \(\eta\) within defined upper and lower bounds over a predetermined interval, employing a cosine function. The formula for cosine annealing reflects the shape of a half-cosine wave, which decreases from a maximum value to a minimum and then increases back to the maximum. This cycle can repeat multiple times over the training process, depending on how the scheduler is configured. Although this approach suggests cyclic adjustments (oscillations) within the training duration, for simplicity’s sake, our specific implementation, inspired by MosaicML’s Composer’s CosineAnnealingWithWarmupScheduler, explicitly excludes considerations for such cycles/oscillations.
Definition 17 (Cosine Annealing With Warmup)
The CosineAnnealingWithWarmupScheduler
modulates the learning rate \(\eta\)
according to a two-phase process: a warmup phase followed by a
cosine annealing phase. The learning rate multiplier[^lr-multiplier]
\(\alpha_{t}\) at any given time (step) \(t\) is given by:
where we denote:
\(t\) represents the current training step or epoch.
\(\eta_{\max}\) as the maximum learning rate reached during training, and often is the initial learning rate given into an optimizer.
\(t_{\text{warmup}}\) denotes the duration of the warmup period, in terms of the number of steps or epochs, during which the learning rate linearly increases to the maximum learning rate \(\eta_{\max}\).
\(t_{\max}\) as the maximum number of training steps, or maximum number of iterations in an epoch (see here).
\(\tau_w = \frac{t - t_{\text{warmup}}}{t_{\max}}\), the fraction of post-warmup time elapsed,
\(\alpha_f\) is a scaling factor that determines the final learning rate multiplier to decay to (a value between \(0\) and \(1\)), and this is a fixed value. For example, if \(\alpha_f = 0.1\) and the initial learning rate is \(\eta_{\max} = 3e-4\), then the final learning rate will be \(\eta_{\min} = 3e-4 \times 0.1 = 3e-5\).
The actual learning rate \(\eta_{t}\) at time (step) \(t\) is then computed as:
where we emphasize again that \(\eta_{\max}\) is the maximum learning rate reached during training.
A Word on Oscillations
Note that if you set \(t_{\max}\) to the total number of training steps that is needed for the entire dataset \(\mathcal{S}\), the scheduler will only decay the learning rate after the warmup phase and not oscillate further. This configuration means that after completing the linear increase during the warmup, the learning rate will decrease following a cosine curve until it reaches the final learning rate determined by \(\alpha_f\).
Single Cycle (No Oscillation): If \(t_{\max}\) is set to cover exactly one half-cycle of the cosine function from the end of the warmup phase to the conclusion of training, the learning rate will monotonically decrease from its maximum value (at the end of warmup) to its minimum value (as determined by \(\alpha_f\)) without oscillating. This is because the scheduler’s active period only spans a single descent phase of the cosine wave.
Multiple Cycles (Oscillation): If \(t_{\max}\) is set to allow for a longer duration than what is needed for a single half-cycle descent, the cosine annealing function can complete its initial descent and then begin to ascend as part of a new cycle. This leads to oscillations in the learning rate—after decreasing, it will start to increase again, potentially multiple times, depending on the total number of cycles fitted within \(t_{\max}\). This is where the term “oscillation” comes into play; it describes the periodic increase and decrease in the learning rate according to the cosine function over multiple cycles.
True oscillation, where the learning rate decreases and then increases within a training regime, typically requires either a restart mechanism (as seen in Cosine Annealing with Warm Restarts) or an explicit multi-cycle configuration. A standard cosine annealing scheduler, especially with a warmup phase, generally only supports a monotonic decrease within a single cycle, unless it is specifically designed to handle restarts or multiple cycles.
Implementation#
from __future__ import annotations
import math
from functools import partial
from torch.optim.lr_scheduler import LambdaLR
from torch.optim.optimizer import Optimizer
def _get_cosine_schedule_with_warmup_lr_lambda(
current_step: int, *, num_warmup_steps: int, num_training_steps: int, alpha_f: float
) -> float:
"""
Helper function for calculating the learning rate using cosine annealing
with warmup.
Parameters
----------
current_step: int
The current step in the training process.
num_warmup_steps: int
The number of steps for the warmup phase.
num_training_steps: int
The total number of training steps.
alpha_f: float
The minimum learning rate at the end of the schedule.
Returns
-------
float
The calculated learning rate.
"""
if current_step < num_warmup_steps:
alpha = current_step / max(1, num_warmup_steps)
else:
tau_w = (current_step - num_warmup_steps) / num_training_steps
tau_w = min(1.0, tau_w)
alpha = alpha_f + (1 - alpha_f) * (1 + math.cos(math.pi * tau_w)) / 2
return alpha
def get_cosine_annealing_with_warmup(
optimizer: Optimizer,
num_warmup_steps: int,
num_training_steps: int,
alpha_f: float = 0.1,
last_epoch: int = -1,
verbose: bool = False,
) -> LambdaLR:
"""
Create a schedule with a learning rate that decreases following the values
of the cosine function between the initial lr set in the optimizer to 0,
after a warmup period during which it increases linearly between 0 and the
initial lr set in the optimizer.
Parameters
----------
optimizer: `~torch.optim.Optimizer`
The optimizer for which to schedule the learning rate.
num_warmup_steps: int
The number of steps for the warmup phase.
num_training_steps: int
The total number of training steps.
alpha_f: float
The minimum learning rate at the end of the schedule, by default 0.1.
last_epoch: int
The index of the last epoch when resuming training, by default -1.
verbose: bool
Whether to print the learning rate at every update, by default False.
Returns
-------
`torch.optim.lr_scheduler.LambdaLR`
The scheduler with the appropriate schedule.
Examples
--------
>>> from torch import nn
>>> from torch.optim import Adam
>>> dummy_model = nn.Linear(1, 1)
>>> optimizer = Adam(dummy_model.parameters(), lr=3e-4)
>>> scheduler = get_cosine_annealing_with_warmup(optimizer, num_warmup_steps=5, num_training_steps=10, alpha_f=0.5)
>>> assert isinstance(scheduler, LambdaLR)
"""
lr_lambda = partial(
_get_cosine_schedule_with_warmup_lr_lambda,
num_warmup_steps=num_warmup_steps,
num_training_steps=num_training_steps,
alpha_f=alpha_f,
)
return LambdaLR(optimizer, lr_lambda, last_epoch, verbose)
num_warmup_steps = 3 * len(train_loader)
num_training_steps = composer.trainer.max_epochs * (len(train_dataset) // composer.data.train_loader["batch_size"])
alpha_f = 1 # as if no decay
scheduler = get_cosine_annealing_with_warmup(optimizer, num_warmup_steps=num_warmup_steps, num_training_steps=num_training_steps, alpha_f=alpha_f)
from omnivault.transformer.core.scheduler import noam_lr_decay
warmup_steps = 3 * len(train_loader)
# lr first increases in the warmup steps, and then decays
noam = lambda step: noam_lr_decay(step, d_model=128, warmup_steps=warmup_steps) # noqa: E731
scheduler_config_cls = SCHEDULER_REGISTRY[cfg.scheduler.name]
if issubclass(scheduler_config_cls, LambdaLRConfig):
scheduler_pydantic_config = scheduler_config_cls(lr_lambda=noam, **cfg.scheduler)
else:
scheduler_pydantic_config = scheduler_config_cls(**cfg.scheduler) # type: ignore[assignment]
composer.scheduler = scheduler_pydantic_config
scheduler = scheduler_pydantic_config.build(optimizer=optimizer)
Criterion#
The Cross Entropy Loss function calculates the difference between two probability distributions - the predicted probability distribution output by the model (logits) and the actual distribution (target labels). It’s primarily used in classification tasks involving \(C\) classes.
\(\mathcal{B}\) : Denotes batch size,
\(K\) : The number of additional dimensions beyond batch and class, representing spatial or other feature dimensions in the input tensor,
\(N=\mathcal{B} \times d_1 \times \ldots \times d_K\) : Total count of individual elements across all dimensions, including batch and spatial dimensions. This value adjusts as per the dimensional complexity:
For \(K=0, N=\mathcal{B}\),
For \(K=1, N=\mathcal{B} \times d_1\),
For \(K>1, N\) scales accordingly.
\(C\) : The total number of classification categories,
\(x\) : Represents the input logits tensor,
\(y\) : Denotes the target tensor,
\(w\) : An optional tensor assigning weights to each class,
\(\mathcal{L}\) : Symbolizes the aggregate loss prior to any reduction,
\(l_b\) : The loss corresponding to the \(b\) th element within the batch, ranging over \(b=1\) to \(\mathcal{B}\).
Inputs and Targets#
Inputs (Logits): The function expects unnormalized logits for each class per input. These logits do not necessarily need to be positive values nor sum to 1. The shape of the input tensor can be:
For unbatched input: \((C)\),
For batched input: \((\mathcal{B}, C)\),
For \(K\)-dimensional input: \((\mathcal{B}, C, d_1, d_2, \ldots, d_K)\), suitable for tasks like pixel-wise classification in images where \(K \geq 1\).
Targets: When configuring the targets for the Cross Entropy Loss function, their expected shapes vary based on the nature of the targets (class indices vs. probabilities) and the dimensionality of the input:
For Class Indices as Targets:
Unbatched input: The shape should be a scalar representing a single class index in \([0, C)\).
Batched input: The shape should be \((\mathcal{B},)\), where each element is a class index for the corresponding input in the batch.
\(K\)-dimensional input: The shape should be \((\mathcal{B}, d_1, d_2, \ldots, d_K)\) for the \(K\)-dimensional case, with each element representing a class index for the corresponding spatial location.
For Probabilities as Targets (applicable in advanced scenarios like label smoothing or multi-label classification):
The shape of the targets must match the shape of the input logits tensor: \((\mathcal{B}, C)\) for batched input or \((\mathcal{B}, C, d_1, d_2, \ldots, d_K)\) for \(K\)-dimensional input. Each element in this tensor should be a probability corresponding to the likelihood of the class, with values in \([0, 1]\).
Loss Computation#
For Class Indices as Targets:
The loss for each element \(n\), accurately spanning across all considered dimensions, is calculated as:
\[ \ell(x, y) = \mathcal{L} = \{l_1, \ldots, l_{N}\}^{\top}, \quad l_n = -w_{y_n} \cdot \log \left( \frac{\exp(x_{n, y_n})}{\sum_{c=1}^{C} \exp(x_{n, c})} \right) \cdot \mathbb{1}\{y_n \neq \text{ignore_index}\} \]Here, \(N\) correctly reflects the aggregate count of elements when considering \(\mathcal{B}\) and the \(K\)-dimensional context. Consequently, if \(K=0\), \(N\) reduces to \(\mathcal{B}\).
For Probabilities as Targets:
In cases where the targets are probabilities, the calculation over each element \(n\), aligning with \(N\)’s definition, should be:
\[ \ell(x, y) = \mathcal{L} = \{l_1, \ldots, l_{N}\}^{\top}, \quad l_n = -\sum_{c=1}^{C} w_c \cdot y_{n, c} \cdot \log \left( \frac{\exp(x_{n, c})}{\sum_{i=1}^{C} \exp(x_{n, i})} \right) \]
Reduction#
No Reduction (
reduction='none'
):When the reduction is set to ‘none’, the loss computation preserves the original dimensionality of the input, effectively returning a tensor that maps directly to each input element’s loss. This tensor has the shape \((\mathcal{B}, d_1, \ldots, d_K)\), where each element \(l_{n}\) within this tensor represents the computed loss for the corresponding input element across all dimensions, including the batch and any \(K\)-dimensional space:
\[ \mathcal{L} = \{l_1, \ldots, l_N\} \]This preserves the granularity of loss across the dataset, allowing for detailed analysis or custom reduction post hoc.
Mean Reduction (
reduction='mean'
):For the ‘mean’ reduction, the losses across all elements are averaged to yield a single scalar value. This operation accounts for the total count of elements (\(N\)), including those spanning batch and additional dimensions, and is not merely an average over the batch size \(\mathcal{B}\), but over all \(N\) elements:
\[ \mathcal{L}_{mean} = \frac{1}{N} \sum_{n=1}^{N} l_n \]Here, traditionally we think of \(N\) as just the number of elements in the batch, but in the implementation, it spans all elements across the batch and \(K\)-dimensional spaces.
Sum Reduction (
reduction='sum'
):With ‘sum’ reduction, the losses for all elements are aggregated into a single scalar through summation, without averaging. This sums the losses across all elements, including those across the batch and \(K\)-dimensional spaces:
\[ \mathcal{L}_{sum} = \sum_{n=1}^{N} l_n \]This scalar represents the total loss accumulated across the entire input set, providing a measure of overall loss magnitude without normalization by the number of elements.
Simple Binary Classification Example#
nn.CrossEntropyLoss
in PyTorch expects the input logits to be of shape[N, C, d1, d2, ..., dK]
(whereN
is the batch size,C
is the number of classes, andd1
todK
are optional additional dimensions) and the target to be of shape[N, d1, d2, ..., dK]
.Let’s look a simplified example in image classification. The target is a single integer representing the class label, and the input logits are a vector of length
C
(the number of classes).More concretely, in the below example, we have \(\mathcal{B} = 4\) (batch size), \(C = 2\) (number of classes), \(K = 0\) (no additional dimensions), and \(N = 4\) (total number of elements across all dimensions).
Our inputs (logits) are of shape \((\mathcal{B}, C) = (4, 2)\).
Our targets are of shape \((\mathcal{B}) = (4)\).
rng = torch.Generator().manual_seed(composer.global_.seed)
criterion = nn.CrossEntropyLoss(reduction="mean")
B, C = 4, 2
targets = torch.tensor([1, 0, 0, 0]) # indicating sample 1 is class 1 and sample 2 is class 0
logits = torch.tensor([[0.1, 0.9], [0.9, 0.1], [0.8, 0.2], [0.3, 0.7]])
assert logits.size() == (B, C)
loss = criterion(logits, targets)
pprint(loss)
tensor(0.5232)
GPT Example#
First we set up the criterion for the model. We use the nn.CrossEntropyLoss
criterion, which is commonly used for classification tasks.
criterion = nn.CrossEntropyLoss(reduction="mean", ignore_index=vocabulary.token_to_index[vocabulary.PAD])
In scenarios involving classification tasks, targets and inputs (logits) usually align in a straightforward manner where each target is a single integer that signifies the class label, and the corresponding input logits form a vector of length \(C\) (the number of classes).
However, complexity arises when dealing with sequences, such as in decoder outputs, this is because we are predicting a sequence of class labels for each token in the sequence. So if a sequence \(\mathbf{x}\) has \(10\) tokens \(x_1, x_2, \ldots, x_{10}\), the target is a sequence of class labels \(y_1, y_2, \ldots, y_{10}\). While the target shape is still \((\mathcal{B}, T)\), and for each sample, you can think of it as \(10\) samples embedded within, each with a single class label corresponding to the token at that step. Consequently, the logits shape becomes \((\mathcal{B}, T, \mathcal{V})\), where \(\mathcal{V}\) aligns with \(\mathcal{C}\), the number of classes. In other words, this counter-intuitive structure can be easily reduced to our normal classification problem if we remove the batch dimension to have \((T, )\) and \((T, \mathcal{V})\) respectively for the target and logits - in which case we can treat \(T\) as the batch size \(\mathcal{B}\) and proceed as usual. To this end, if we want to introduce the batch dimension back, then a simple idea is to flatten the target and logits to \((\mathcal{B} \times T, )\) and \((\mathcal{B} \times T, \mathcal{V})\) respectively. This way, say we have a batch size of \(2\) and a sequence length of \(3\), we can easily think of it as \(6\) samples in total, each with a single class label. Why this idea isn’t obvious to me at first is because not having the fundamentals from earlier models such as RNNs and LSTMs.
Let’s consider the example below, with batch size \(\mathcal{B} = 2\), sequence length \(T = 10\), and number of classes \(V = 18\).
rng = torch.Generator().manual_seed(composer.global_.seed)
input_tokens, targets, _, _ = next(iter(train_loader))
B, T, V = 2, 10, 18
# get only first two samples
input_tokens = input_tokens[0:B, :]
targets = targets[0:B, :]
assert input_tokens.size() == (B, T)
assert targets.size() == (B, T)
# get logits
logits = model(input_tokens)
assert logits.size() == (B, T, V) # 2, 10, 18
logits.view(-1, logits.size(-1)).size(), targets.view(-1).size()
(torch.Size([20, 18]), torch.Size([20]))
logits_flattened = logits.view(-1, logits.size(-1))
targets_flattened = targets.view(-1)
assert logits_flattened.size() == (B * T, V)
loss = criterion(logits_flattened, targets_flattened)
pprint(loss)
tensor(2.8830, grad_fn=<NllLossBackward0>)
The second way we will use is essentially the same as the first way, but more implicit.
logits.permute(0, 2, 1).contiguous().shape
torch.Size([2, 18, 10])
We first permute the logits tensor to have the shape
\((\mathcal{B} \times \mathcal{V}, T)\), why so? Because recall our earlier
definition in PyTorch’s nn.CrossEntropyLoss
where the input logits should be
of shape [B, C, d1, d2, ..., dK]
(where B
is the batch size, C
is the
number of classes, and d1
to dK
are optional additional dimensions) and the
target to be of shape [B, d1, d2, ..., dK]
. In our case, we have
\(\mathcal{B} = 2\) (batch size), \(C = 18\) (number of classes), \(K = 1\)
(additional dimension), but however we are in the wrong order of dimension
because PyTorch expects the first dimension to be of batch size, which is fine,
but the second dimension must be the class dimension, which in our case is not
because our second dimension is the sequence length \(T\). So we swap the second
and third dimension to have the shape \((\mathcal{B}, \mathcal{V}, T)\), and then
PyTorch’s nn.CrossEntropyLoss
would then reshape the logits to
\((\mathcal{B} \times T, \mathcal{V})\) and the targets to
\((\mathcal{B} \times T, )\) - coinciding with our earlier discussion.
loss = criterion(logits.permute(0, 2, 1).contiguous(), targets.contiguous())
pprint(loss) # same
tensor(2.8830, grad_fn=<NllLoss2DBackward0>)
In a GPT-like model, each token in the sequence is used to predict the next token. The model takes a sequence of tokens and produces a new sequence of the same length where each new token is predicted based on all the preceding tokens in the input sequence. The loss is then computed between the predicted sequence and the target sequence.
Let’s take a closer look at an example:
The original tensor:
[15, 9, 0, 10, 3, 8, 13, 1, 2, 8, 14]
which corresponds to<BOS>90+38=128<EOS>
Input tensor:
[15, 9, 0, 10, 3, 8, 13, 1, 2, 8]
, which corresponds to<BOS>90+38=128
withoutEOS
Target tensor:
[9, 0, 10, 3, 8, 13, 1, 2, 8, 14]
[16, 16, 16, 16, 16, 16, 1, 2, 8, 14]
During training:
First Timestep: The model takes
[15]
(or[<BOS>]
if 15 is your BOS token) and tries to predict the next token. Ideally, it should predict9
. But here, your target sequence starts with masked tokens (16
, if 16 is your masking token). So the loss is computed between the predicted token and the masked token16
. But sinceCrossEntropyLoss
has anignore_index
(now you know what they are right!), you can set it to say16
or (default-1
but you would need to change padding number) and tell the model that whenever the ground truth is16
, the loss is zeroed out so it is not counted? This allows the model to focus on learning from the relevant parts of the sequence while ignoring the masked portions.Second Timestep: The model takes
[15, 9]
and predicts the next token, which should be0
. Again, the target is a masked token16
.…
Eighth Timestep: The model takes
[15, 9, 0, 10, 3, 8, 13]
(which is90+38=
) and predicts the next token. Now the target is1
, so the loss is computed between the predicted token and1
. There is no mask anymore here, so the loss will be computed.Ninth Timestep: The model takes
[15, 9, 0, 10, 3, 8, 13, 1]
(which is90+38=1
) and predicts the next token. Now the target is2
, so the loss is computed between the predicted token and2
.Here’s an important thing for beginners (me), In a typical GPT-like architecture used for sequence-to-sequence tasks like this one, the model doesn’t use its own predictions as input during training. Instead, it uses the original, ground-truth input sequence. This is known as “teacher forcing.” In teacher forcing, even if the model predicts a wrong token at some timestep, it doesn’t affect the input sequence for subsequent timesteps. The model continues to get the original input sequence for the entire training epoch.
So if model predicts a
3
during the eighth timestep, where the ground trut is1
, the model would simply incur a higher loss for that prediction. However, the input for the ninth timestep would still be the ground truth sequence up to that point, regardless of what the model predicted at the eighth timestep.But it is noted that this behaviour is still autoregressive.
Tenth: The model takes
[15, 9, 0, 10, 3, 8, 13, 1, 2]
and predicts the next token which is8
.Last: The model takes
[15, 9, 0, 10, 3, 8, 13, 1, 2, 8]
and predicts the next token which is14
theEOS
.The reason you need to predict
EOS
is simple intuitively, consider the case where there’s no need forEOS
, then the model will not know when to stop.
This goes on until the entire sequence is processed. Note that the model never actually “sees” the target tokens during the prediction. It is solely relying on the tokens that came before the current token in the input sequence. After the model makes its prediction, then the predicted tokens are compared to the target tokens to compute the loss, which is then backpropagated to update the model weights.
A Smaller Example for Illustration#
The above example has too big of a dimension, let’s scale down \(T=10\) to \(T=3\) and \(V=18\) to \(V=4\).
# fmt: off
rng = torch.Generator().manual_seed(composer.global_.seed)
B, T, V = 2, 3, 4 # Assuming we have B = batch size, T = sequence length, V = vocab size
logits = torch.randn(B, T, V, generator=rng) # logits from the head
targets = torch.randint(low=0, high=V, size=(B, T), generator=rng) # targets are the labels
# fmt: on
pprint(logits)
pprint(targets)
pprint(logits[0]) # logits for the first sequence [T=3, V=4]
pprint(targets[0]) # target for the first sequence [T=3]
tensor([[[ 1.9269, 1.4873, 0.9007, -2.1055], │ │ [ 0.6784, -1.2345, -0.0431, -1.6047], │ │ [ 0.3559, -0.6866, -0.4934, 0.2415]], │ │ │ │ [[-1.1109, 0.0915, -2.3169, -0.2168], │ │ [-0.3097, -0.3957, 0.8034, -0.6216], │ │ [-0.5920, -0.0631, -0.8286, 0.3309]]])
tensor([[2, 2, 1], │ │ [3, 3, 3]])
tensor([[ 1.9269, 1.4873, 0.9007, -2.1055], │ │ [ 0.6784, -1.2345, -0.0431, -1.6047], │ │ [ 0.3559, -0.6866, -0.4934, 0.2415]])
tensor([2, 2, 1])
We establish some conceptual understanding first:
Each sample in the batch has the following characteristics:
Denote
target
andlogit
as the target and logits for a particular sample in the batch.The
target
is of shape[T] = [3]
and each element is the class/vocab label for each token in the sequence.The
logit
is of shape[T, V] = [3, 4]
and each row is the logits for each token in the sequence.Therefore, we want to compare each row in
logit
with each element intarget
to compute the loss.We can think of each row in
logit
as the prediction for each token in the sequence, and each element intarget
as the ground truth for each token in the sequence.Intuitively this means that within each sample, there are many “sub-samples” where each sub-sample is a token in the sequence. If you can visualize this, then there should be no confusion.
In code, we can do so with the following manner:
Calculate loss for each token in each sample individually and then sum them up.
Reduction by mean will mean we need to divide our
total_loss
by the total number of samples in the batch. But remember that even though technically we have 2 samples in the batch, we are actually treating each token in each sample as a sub-sample, so the total samples isB * T
whereB
is the batch size andT
is the sequence length.
criterion = nn.CrossEntropyLoss(reduction="mean")
total_loss = 0
for b in range(B):
for t in range(T):
logit = logits[b, t].unsqueeze(0)
target = targets[b, t].unsqueeze(0)
total_loss += criterion(logit, target)
pprint(total_loss)
total_loss = total_loss / (B * T)
pprint(total_loss)
tensor(9.0105)
tensor(1.5017)
In PyTorch however, if you have a logits tensor of shape [B, S, V]
, you need
to permute it to [B, V, S]
to align with the format that CrossEntropyLoss
wants, so that V
(vocab size) is treated as C
(number of classes), and S
(sequence length) is treated as one of the additional dimensions
d1, d2, ..., dK
.
But all in all, if you understood the previous loop to calculate the loss for each token in each sample individually and then sum them up, then dividing to fulfill reduction of mean, then you should be fine.
Masking and Ignore Index#
# fmt: off
rng = torch.Generator().manual_seed(composer.global_.seed)
B, T, V = 2, 3, 4 # Assuming we have B = batch size, L = sequence length, V = vocab size
logits = torch.randn(B, T, V, generator=rng) # logits from the head
targets = torch.randint(low=0, high=V, size=(B, T), generator=rng) # targets are the labels
# fmt: on
pprint(logits)
pprint(targets)
pprint(logits[0]) # logits for the first sequence [L=10, V=18]
pprint(targets[0]) # target for the first sequence [L=10]
tensor([[[ 1.9269, 1.4873, 0.9007, -2.1055], │ │ [ 0.6784, -1.2345, -0.0431, -1.6047], │ │ [ 0.3559, -0.6866, -0.4934, 0.2415]], │ │ │ │ [[-1.1109, 0.0915, -2.3169, -0.2168], │ │ [-0.3097, -0.3957, 0.8034, -0.6216], │ │ [-0.5920, -0.0631, -0.8286, 0.3309]]])
tensor([[2, 2, 1], │ │ [3, 3, 3]])
tensor([[ 1.9269, 1.4873, 0.9007, -2.1055], │ │ [ 0.6784, -1.2345, -0.0431, -1.6047], │ │ [ 0.3559, -0.6866, -0.4934, 0.2415]])
tensor([2, 2, 1])
targets[:, 0] = -123
PAD_ = -123
criterion = nn.CrossEntropyLoss(reduction="mean", ignore_index=PAD_)
NON_IGNORE_COUNT = 0
total_loss = 0
for b in range(B):
for t in range(T):
logit = logits[b, t].unsqueeze(0)
target = targets[b, t].unsqueeze(0)
if target == torch.tensor([PAD_]):
continue
total_loss += criterion(logit, target)
NON_IGNORE_COUNT += 1
pprint(total_loss)
total_loss = total_loss / NON_IGNORE_COUNT
pprint(total_loss)
tensor(6.2188)
tensor(1.5547)
NOTE: NON_IGNORE_COUNT
is used instead of BxT
, why? Cause we are averaging
over all non-ignored guys!
# Permute logits to shape [B, V, S]
logits_permuted = logits.permute(0, 2, 1)
# Instantiate the CrossEntropyLoss
# By default, it reduces by averaging the losses over each observation in the input
criterion = nn.CrossEntropyLoss(reduction="mean", ignore_index=PAD_)
loss = criterion(logits_permuted, targets)
pprint(loss)
tensor(1.5547)
# Permute logits to shape [B, V, S]
logits_permuted = logits.permute(0, 2, 1)
# Instantiate the CrossEntropyLoss
# By default, it reduces by averaging the losses over each observation in the input
criterion = nn.CrossEntropyLoss(reduction="mean", ignore_index=PAD_)
loss = criterion(logits_permuted, targets)
pprint(loss)
tensor(1.5547)
Initializing Criterion With Composer#
from omnivault.transformer.config.criterion import CRITERION_REGISTRY
criterion_config_cls = CRITERION_REGISTRY[cfg.criterion.name]
criterion_pydantic_config = criterion_config_cls(**cfg.criterion)
criterion = criterion_pydantic_config.create_instance()
assert criterion.ignore_index == vocabulary.token_to_index[vocabulary.PAD]
pprint(criterion.ignore_index)
pprint(criterion.reduction)
16
'mean'
State#
from omnivault.transformer.core.state import State
state = State(
model=model,
criterion=criterion,
optimizer=optimizer,
scheduler=scheduler,
vocabulary=vocabulary,
tokenizer=tokenizer,
)
state.pretty_print()
State( │ model=GPTDecoder( (tok_embed): Embedding(18, 128) (decoder_blocks): ModuleList( │ (0-1): 2 x GPTDecoderBlock( │ (masked_self_attention_mha): MultiHeadedAttention( │ │ (W_Q): Linear(in_features=128, out_features=128, bias=False) │ │ (W_K): Linear(in_features=128, out_features=128, bias=False) │ │ (W_V): Linear(in_features=128, out_features=128, bias=False) │ │ (W_O): Linear(in_features=128, out_features=128, bias=False) │ │ (attention): ScaledDotProductAttention( │ │ (dropout): Dropout(p=0.0, inplace=False) │ │ ) │ │ (dropout): Dropout(p=0.1, inplace=False) │ ) │ (feed_forward): PositionwiseFeedForward( │ │ (ffn): ModuleDict( │ │ (context_fc): Linear(in_features=128, out_features=256, bias=True) │ │ (activation): GELU(approximate='tanh') │ │ (context_projection): Linear(in_features=256, out_features=128, bias=True) │ │ (dropout): Dropout(p=0.1, inplace=False) │ │ ) │ ) │ (add_norm_1): AddNorm( │ │ (dropout): Dropout(p=0.1, inplace=False) │ │ (layer_norm): LayerNorm((128,), eps=1e-05, elementwise_affine=True) │ ) │ (add_norm_2): AddNorm( │ │ (dropout): Dropout(p=0.1, inplace=False) │ │ (layer_norm): LayerNorm((128,), eps=1e-05, elementwise_affine=True) │ ) │ ) ) (dropout): Dropout(p=0.1, inplace=False) (layer_norm): LayerNorm((128,), eps=1e-05, elementwise_affine=True) (head): Linear(in_features=128, out_features=18, bias=True) ), │ criterion=CrossEntropyLoss(), │ optimizer=Adam ( Parameter Group 0 │ amsgrad: False │ betas: (0.9, 0.98) │ capturable: False │ differentiable: False │ eps: 1e-09 │ foreach: None │ fused: None │ initial_lr: 0.2 │ lr: 2.2961808030073203e-05 │ maximize: False │ weight_decay: 0.0 Parameter Group 1 │ amsgrad: False │ betas: (0.9, 0.98) │ capturable: False │ differentiable: False │ eps: 1e-09 │ foreach: None │ fused: None │ initial_lr: 0.2 │ lr: 2.2961808030073203e-05 │ maximize: False │ weight_decay: 0.0 ), │ scheduler=<torch.optim.lr_scheduler.LambdaLR object at 0x7f6af036ef70>, │ epoch_index=0, │ train_batch_index=0, │ step_index=0, │ history={}, │ vocabulary=<omnivault.transformer.core.vocabulary.AdderVocabulary object at 0x7f6a286850d0>, │ tokenizer=<omnivault.transformer.core.tokenizer.AdderTokenizer object at 0x7f6a28685400>, │ tokens_per_iter=None )
Trainer#
trainer = Trainer(
state=state,
composer=composer,
# logger=LOGGER,
device=composer.trainer.device, # type: ignore[arg-type]
)
trainer.remove_callback(event=TrainerEvent.ON_VALID_EPOCH_END.value, callback=save_state)
trainer.add_callback(
TrainerEvent.ON_VALID_EPOCH_END.value,
lambda trainer: evaluate_and_generate_on_valid_epoch_end(trainer, num_batches_to_eval=None),
)
_trained_state = trainer.fit(train_loader=train_loader, valid_loader=valid_loader, test_loader=test_loader)
# _trained_state.pretty_print()
history = _trained_state.history
/home/runner/work/omniverse/omniverse/omnivault/transformer/core/trainer.py:172: FutureWarning: `torch.cuda.amp.GradScaler(args...)` is deprecated. Please use `torch.amp.GradScaler('cuda', args...)` instead.
self.scaler = torch.cuda.amp.GradScaler(**self.scaler_config)
2024-12-17 09:43:57,370 - root - INFO - Total Parameters: 270226
2024-12-17 09:43:57,370 - root - INFO - Trainable Parameters: 270226
2024-12-17 09:43:57,371 - root - INFO - Vocabulary Size: 18
2024-12-17 09:43:57,371 - root - INFO - Context Length: 11
2024-12-17 09:43:57,372 - root - INFO - Device: cpu
2024-12-17 09:43:57,372 - root - INFO - Tokens per Iteration: 2816
2024-12-17 09:43:57,373 - root - INFO - Total Tokens Consumed In Training: 126720
2024-12-17 09:43:57,373 - root - INFO - Initial Learning Rate(s): 0.000022962, 0.000022962
2024-12-17 09:43:57,374 - root - INFO -
2024-12-17 09:43:57,376 - root - INFO - ====================================================== Starting Train Epoch: 1/9 ======================================================
2024-12-17 09:43:57,377 - root - INFO - Learning rates for each parameter group: 0.00002296180803007320, 0.00002296180803007320
0%| | 0/28 [00:00<?, ?it/s]
Epoch: 1, Step: 1: 0%| | 0/28 [00:00<?, ?it/s]
Epoch: 1, Step: 1: 0%| | 0/28 [00:00<?, ?it/s, total_batch_loss=747.79352, average_batch_loss=2.92107, average_batch_perplexity=18.56111, lr=0.000022962, 0.000022962]
Epoch: 1, Step: 1: 4%|▎ | 1/28 [00:00<00:02, 9.27it/s, total_batch_loss=747.79352, average_batch_loss=2.92107, average_batch_perplexity=18.56111, lr=0.000022962, 0.000022962]
Epoch: 1, Step: 2: 4%|▎ | 1/28 [00:00<00:02, 9.27it/s, total_batch_loss=747.79352, average_batch_loss=2.92107, average_batch_perplexity=18.56111, lr=0.000022962, 0.000022962]
Epoch: 1, Step: 2: 4%|▎ | 1/28 [00:00<00:02, 9.27it/s, total_batch_loss=739.30005, average_batch_loss=2.88789, average_batch_perplexity=17.95540, lr=0.000045924, 0.000045924]
Epoch: 1, Step: 2: 7%|▋ | 2/28 [00:00<00:02, 9.35it/s, total_batch_loss=739.30005, average_batch_loss=2.88789, average_batch_perplexity=17.95540, lr=0.000045924, 0.000045924]
Epoch: 1, Step: 3: 7%|▋ | 2/28 [00:00<00:02, 9.35it/s, total_batch_loss=739.30005, average_batch_loss=2.88789, average_batch_perplexity=17.95540, lr=0.000045924, 0.000045924]
Epoch: 1, Step: 3: 7%|▋ | 2/28 [00:00<00:02, 9.35it/s, total_batch_loss=720.04199, average_batch_loss=2.81266, average_batch_perplexity=16.65422, lr=0.000068885, 0.000068885]
Epoch: 1, Step: 4: 7%|▋ | 2/28 [00:00<00:02, 9.35it/s, total_batch_loss=720.04199, average_batch_loss=2.81266, average_batch_perplexity=16.65422, lr=0.000068885, 0.000068885]
Epoch: 1, Step: 4: 7%|▋ | 2/28 [00:00<00:02, 9.35it/s, total_batch_loss=695.30609, average_batch_loss=2.71604, average_batch_perplexity=15.12032, lr=0.000091847, 0.000091847]
Epoch: 1, Step: 4: 14%|█▍ | 4/28 [00:00<00:03, 7.46it/s, total_batch_loss=695.30609, average_batch_loss=2.71604, average_batch_perplexity=15.12032, lr=0.000091847, 0.000091847]
Epoch: 1, Step: 5: 14%|█▍ | 4/28 [00:00<00:03, 7.46it/s, total_batch_loss=695.30609, average_batch_loss=2.71604, average_batch_perplexity=15.12032, lr=0.000091847, 0.000091847]
Epoch: 1, Step: 5: 14%|█▍ | 4/28 [00:00<00:03, 7.46it/s, total_batch_loss=662.83459, average_batch_loss=2.58920, average_batch_perplexity=13.31908, lr=0.000114809, 0.000114809]
Epoch: 1, Step: 6: 14%|█▍ | 4/28 [00:00<00:03, 7.46it/s, total_batch_loss=662.83459, average_batch_loss=2.58920, average_batch_perplexity=13.31908, lr=0.000114809, 0.000114809]
Epoch: 1, Step: 6: 14%|█▍ | 4/28 [00:00<00:03, 7.46it/s, total_batch_loss=639.73022, average_batch_loss=2.49895, average_batch_perplexity=12.16967, lr=0.000137771, 0.000137771]
Epoch: 1, Step: 6: 21%|██▏ | 6/28 [00:00<00:02, 9.21it/s, total_batch_loss=639.73022, average_batch_loss=2.49895, average_batch_perplexity=12.16967, lr=0.000137771, 0.000137771]
Epoch: 1, Step: 7: 21%|██▏ | 6/28 [00:00<00:02, 9.21it/s, total_batch_loss=639.73022, average_batch_loss=2.49895, average_batch_perplexity=12.16967, lr=0.000137771, 0.000137771]
Epoch: 1, Step: 7: 21%|██▏ | 6/28 [00:00<00:02, 9.21it/s, total_batch_loss=617.32874, average_batch_loss=2.41144, average_batch_perplexity=11.15001, lr=0.000160733, 0.000160733]
Epoch: 1, Step: 8: 21%|██▏ | 6/28 [00:00<00:02, 9.21it/s, total_batch_loss=617.32874, average_batch_loss=2.41144, average_batch_perplexity=11.15001, lr=0.000160733, 0.000160733]
Epoch: 1, Step: 8: 21%|██▏ | 6/28 [00:00<00:02, 9.21it/s, total_batch_loss=597.33167, average_batch_loss=2.33333, average_batch_perplexity=10.31219, lr=0.000183694, 0.000183694]
Epoch: 1, Step: 8: 29%|██▊ | 8/28 [00:00<00:01, 10.35it/s, total_batch_loss=597.33167, average_batch_loss=2.33333, average_batch_perplexity=10.31219, lr=0.000183694, 0.000183694]
Epoch: 1, Step: 9: 29%|██▊ | 8/28 [00:00<00:01, 10.35it/s, total_batch_loss=597.33167, average_batch_loss=2.33333, average_batch_perplexity=10.31219, lr=0.000183694, 0.000183694]
Epoch: 1, Step: 9: 29%|██▊ | 8/28 [00:00<00:01, 10.35it/s, total_batch_loss=581.93115, average_batch_loss=2.27317, average_batch_perplexity=9.71012, lr=0.000206656, 0.000206656]
Epoch: 1, Step: 10: 29%|██▊ | 8/28 [00:00<00:01, 10.35it/s, total_batch_loss=581.93115, average_batch_loss=2.27317, average_batch_perplexity=9.71012, lr=0.000206656, 0.000206656]
Epoch: 1, Step: 10: 29%|██▊ | 8/28 [00:00<00:01, 10.35it/s, total_batch_loss=566.71552, average_batch_loss=2.21373, average_batch_perplexity=9.14981, lr=0.000229618, 0.000229618]
Epoch: 1, Step: 10: 36%|███▌ | 10/28 [00:00<00:01, 11.03it/s, total_batch_loss=566.71552, average_batch_loss=2.21373, average_batch_perplexity=9.14981, lr=0.000229618, 0.000229618]
Epoch: 1, Step: 11: 36%|███▌ | 10/28 [00:01<00:01, 11.03it/s, total_batch_loss=566.71552, average_batch_loss=2.21373, average_batch_perplexity=9.14981, lr=0.000229618, 0.000229618]
Epoch: 1, Step: 11: 36%|███▌ | 10/28 [00:01<00:01, 11.03it/s, total_batch_loss=552.24475, average_batch_loss=2.15721, average_batch_perplexity=8.64694, lr=0.000252580, 0.000252580]
Epoch: 1, Step: 12: 36%|███▌ | 10/28 [00:01<00:01, 11.03it/s, total_batch_loss=552.24475, average_batch_loss=2.15721, average_batch_perplexity=8.64694, lr=0.000252580, 0.000252580]
Epoch: 1, Step: 12: 36%|███▌ | 10/28 [00:01<00:01, 11.03it/s, total_batch_loss=543.99951, average_batch_loss=2.12500, average_batch_perplexity=8.37288, lr=0.000275542, 0.000275542]
Epoch: 1, Step: 12: 43%|████▎ | 12/28 [00:01<00:01, 11.60it/s, total_batch_loss=543.99951, average_batch_loss=2.12500, average_batch_perplexity=8.37288, lr=0.000275542, 0.000275542]
Epoch: 1, Step: 13: 43%|████▎ | 12/28 [00:01<00:01, 11.60it/s, total_batch_loss=543.99951, average_batch_loss=2.12500, average_batch_perplexity=8.37288, lr=0.000275542, 0.000275542]
Epoch: 1, Step: 13: 43%|████▎ | 12/28 [00:01<00:01, 11.60it/s, total_batch_loss=530.47626, average_batch_loss=2.07217, average_batch_perplexity=7.94206, lr=0.000298504, 0.000298504]
Epoch: 1, Step: 14: 43%|████▎ | 12/28 [00:01<00:01, 11.60it/s, total_batch_loss=530.47626, average_batch_loss=2.07217, average_batch_perplexity=7.94206, lr=0.000298504, 0.000298504]
Epoch: 1, Step: 14: 43%|████▎ | 12/28 [00:01<00:01, 11.60it/s, total_batch_loss=522.51752, average_batch_loss=2.04108, average_batch_perplexity=7.69895, lr=0.000321465, 0.000321465]
Epoch: 1, Step: 14: 50%|█████ | 14/28 [00:01<00:01, 11.96it/s, total_batch_loss=522.51752, average_batch_loss=2.04108, average_batch_perplexity=7.69895, lr=0.000321465, 0.000321465]
Epoch: 1, Step: 15: 50%|█████ | 14/28 [00:01<00:01, 11.96it/s, total_batch_loss=522.51752, average_batch_loss=2.04108, average_batch_perplexity=7.69895, lr=0.000321465, 0.000321465]
Epoch: 1, Step: 15: 50%|█████ | 14/28 [00:01<00:01, 11.96it/s, total_batch_loss=515.61969, average_batch_loss=2.01414, average_batch_perplexity=7.49428, lr=0.000344427, 0.000344427]
Epoch: 1, Step: 16: 50%|█████ | 14/28 [00:01<00:01, 11.96it/s, total_batch_loss=515.61969, average_batch_loss=2.01414, average_batch_perplexity=7.49428, lr=0.000344427, 0.000344427]
Epoch: 1, Step: 16: 50%|█████ | 14/28 [00:01<00:01, 11.96it/s, total_batch_loss=504.18988, average_batch_loss=1.96949, average_batch_perplexity=7.16703, lr=0.000367389, 0.000367389]
Epoch: 1, Step: 16: 57%|█████▋ | 16/28 [00:01<00:00, 12.27it/s, total_batch_loss=504.18988, average_batch_loss=1.96949, average_batch_perplexity=7.16703, lr=0.000367389, 0.000367389]
Epoch: 1, Step: 17: 57%|█████▋ | 16/28 [00:01<00:00, 12.27it/s, total_batch_loss=504.18988, average_batch_loss=1.96949, average_batch_perplexity=7.16703, lr=0.000367389, 0.000367389]
Epoch: 1, Step: 17: 57%|█████▋ | 16/28 [00:01<00:00, 12.27it/s, total_batch_loss=500.77026, average_batch_loss=1.95613, average_batch_perplexity=7.07193, lr=0.000390351, 0.000390351]
Epoch: 1, Step: 18: 57%|█████▋ | 16/28 [00:01<00:00, 12.27it/s, total_batch_loss=500.77026, average_batch_loss=1.95613, average_batch_perplexity=7.07193, lr=0.000390351, 0.000390351]
Epoch: 1, Step: 18: 57%|█████▋ | 16/28 [00:01<00:00, 12.27it/s, total_batch_loss=489.87445, average_batch_loss=1.91357, average_batch_perplexity=6.77726, lr=0.000413313, 0.000413313]
Epoch: 1, Step: 18: 64%|██████▍ | 18/28 [00:01<00:00, 12.26it/s, total_batch_loss=489.87445, average_batch_loss=1.91357, average_batch_perplexity=6.77726, lr=0.000413313, 0.000413313]
Epoch: 1, Step: 19: 64%|██████▍ | 18/28 [00:01<00:00, 12.26it/s, total_batch_loss=489.87445, average_batch_loss=1.91357, average_batch_perplexity=6.77726, lr=0.000413313, 0.000413313]
Epoch: 1, Step: 19: 64%|██████▍ | 18/28 [00:01<00:00, 12.26it/s, total_batch_loss=484.02982, average_batch_loss=1.89074, average_batch_perplexity=6.62428, lr=0.000436274, 0.000436274]
Epoch: 1, Step: 20: 64%|██████▍ | 18/28 [00:01<00:00, 12.26it/s, total_batch_loss=484.02982, average_batch_loss=1.89074, average_batch_perplexity=6.62428, lr=0.000436274, 0.000436274]
Epoch: 1, Step: 20: 64%|██████▍ | 18/28 [00:01<00:00, 12.26it/s, total_batch_loss=479.82721, average_batch_loss=1.87433, average_batch_perplexity=6.51642, lr=0.000459236, 0.000459236]
Epoch: 1, Step: 20: 71%|███████▏ | 20/28 [00:01<00:00, 12.44it/s, total_batch_loss=479.82721, average_batch_loss=1.87433, average_batch_perplexity=6.51642, lr=0.000459236, 0.000459236]
Epoch: 1, Step: 21: 71%|███████▏ | 20/28 [00:01<00:00, 12.44it/s, total_batch_loss=479.82721, average_batch_loss=1.87433, average_batch_perplexity=6.51642, lr=0.000459236, 0.000459236]
Epoch: 1, Step: 21: 71%|███████▏ | 20/28 [00:01<00:00, 12.44it/s, total_batch_loss=467.47736, average_batch_loss=1.82608, average_batch_perplexity=6.20952, lr=0.000482198, 0.000482198]
Epoch: 1, Step: 22: 71%|███████▏ | 20/28 [00:01<00:00, 12.44it/s, total_batch_loss=467.47736, average_batch_loss=1.82608, average_batch_perplexity=6.20952, lr=0.000482198, 0.000482198]
Epoch: 1, Step: 22: 71%|███████▏ | 20/28 [00:01<00:00, 12.44it/s, total_batch_loss=458.11777, average_batch_loss=1.78952, average_batch_perplexity=5.98659, lr=0.000505160, 0.000505160]
Epoch: 1, Step: 22: 79%|███████▊ | 22/28 [00:01<00:00, 12.45it/s, total_batch_loss=458.11777, average_batch_loss=1.78952, average_batch_perplexity=5.98659, lr=0.000505160, 0.000505160]
Epoch: 1, Step: 23: 79%|███████▊ | 22/28 [00:02<00:00, 12.45it/s, total_batch_loss=458.11777, average_batch_loss=1.78952, average_batch_perplexity=5.98659, lr=0.000505160, 0.000505160]
Epoch: 1, Step: 23: 79%|███████▊ | 22/28 [00:02<00:00, 12.45it/s, total_batch_loss=454.91241, average_batch_loss=1.77700, average_batch_perplexity=5.91210, lr=0.000528122, 0.000528122]
Epoch: 1, Step: 24: 79%|███████▊ | 22/28 [00:02<00:00, 12.45it/s, total_batch_loss=454.91241, average_batch_loss=1.77700, average_batch_perplexity=5.91210, lr=0.000528122, 0.000528122]
Epoch: 1, Step: 24: 79%|███████▊ | 22/28 [00:02<00:00, 12.45it/s, total_batch_loss=440.47971, average_batch_loss=1.72062, average_batch_perplexity=5.58801, lr=0.000551083, 0.000551083]
Epoch: 1, Step: 24: 86%|████████▌ | 24/28 [00:02<00:00, 12.54it/s, total_batch_loss=440.47971, average_batch_loss=1.72062, average_batch_perplexity=5.58801, lr=0.000551083, 0.000551083]
Epoch: 1, Step: 25: 86%|████████▌ | 24/28 [00:02<00:00, 12.54it/s, total_batch_loss=440.47971, average_batch_loss=1.72062, average_batch_perplexity=5.58801, lr=0.000551083, 0.000551083]
Epoch: 1, Step: 25: 86%|████████▌ | 24/28 [00:02<00:00, 12.54it/s, total_batch_loss=433.07043, average_batch_loss=1.69168, average_batch_perplexity=5.42860, lr=0.000574045, 0.000574045]
Epoch: 1, Step: 26: 86%|████████▌ | 24/28 [00:02<00:00, 12.54it/s, total_batch_loss=433.07043, average_batch_loss=1.69168, average_batch_perplexity=5.42860, lr=0.000574045, 0.000574045]
Epoch: 1, Step: 26: 86%|████████▌ | 24/28 [00:02<00:00, 12.54it/s, total_batch_loss=419.93542, average_batch_loss=1.64037, average_batch_perplexity=5.15709, lr=0.000597007, 0.000597007]
Epoch: 1, Step: 26: 93%|█████████▎| 26/28 [00:02<00:00, 12.26it/s, total_batch_loss=419.93542, average_batch_loss=1.64037, average_batch_perplexity=5.15709, lr=0.000597007, 0.000597007]
Epoch: 1, Step: 27: 93%|█████████▎| 26/28 [00:02<00:00, 12.26it/s, total_batch_loss=419.93542, average_batch_loss=1.64037, average_batch_perplexity=5.15709, lr=0.000597007, 0.000597007]
Epoch: 1, Step: 27: 93%|█████████▎| 26/28 [00:02<00:00, 12.26it/s, total_batch_loss=411.37436, average_batch_loss=1.60693, average_batch_perplexity=4.98748, lr=0.000619969, 0.000619969]
Epoch: 1, Step: 28: 93%|█████████▎| 26/28 [00:02<00:00, 12.26it/s, total_batch_loss=411.37436, average_batch_loss=1.60693, average_batch_perplexity=4.98748, lr=0.000619969, 0.000619969]
Epoch: 1, Step: 28: 93%|█████████▎| 26/28 [00:02<00:00, 12.26it/s, total_batch_loss=136.92018, average_batch_loss=1.55591, average_batch_perplexity=4.73940, lr=0.000642931, 0.000642931]
Epoch: 1, Step: 28: 100%|██████████| 28/28 [00:02<00:00, 13.50it/s, total_batch_loss=136.92018, average_batch_loss=1.55591, average_batch_perplexity=4.73940, lr=0.000642931, 0.000642931]
2024-12-17 09:43:59,762 - root - INFO - Total Samples: 7000
2024-12-17 09:43:59,762 - root - INFO - Total Batches: 28
2024-12-17 09:43:59,763 - root - INFO - Average Epoch Train Loss: 2.13059
2024-12-17 09:43:59,763 - root - INFO - Average Epoch Train Perplexity: 8.41986
2024-12-17 09:43:59,764 - root - INFO -
2024-12-17 09:43:59,765 - root - INFO - ====================================================== Starting Valid Epoch: 1/9 ======================================================
0%| | 0/8 [00:00<?, ?it/s]
Epoch: 1, Step: 1: 0%| | 0/8 [00:00<?, ?it/s]
Epoch: 1, Step: 1: 0%| | 0/8 [00:00<?, ?it/s, total_batch_loss=381.24884, average_batch_loss=1.48925, average_batch_perplexity=4.43378]
Epoch: 1, Step: 2: 0%| | 0/8 [00:00<?, ?it/s, total_batch_loss=381.24884, average_batch_loss=1.48925, average_batch_perplexity=4.43378]
Epoch: 1, Step: 2: 0%| | 0/8 [00:00<?, ?it/s, total_batch_loss=382.19833, average_batch_loss=1.49296, average_batch_perplexity=4.45026]
Epoch: 1, Step: 3: 0%| | 0/8 [00:00<?, ?it/s, total_batch_loss=382.19833, average_batch_loss=1.49296, average_batch_perplexity=4.45026]
Epoch: 1, Step: 3: 0%| | 0/8 [00:00<?, ?it/s, total_batch_loss=385.25818, average_batch_loss=1.50491, average_batch_perplexity=4.50377]
Epoch: 1, Step: 3: 38%|███▊ | 3/8 [00:00<00:00, 23.42it/s, total_batch_loss=385.25818, average_batch_loss=1.50491, average_batch_perplexity=4.50377]
Epoch: 1, Step: 4: 38%|███▊ | 3/8 [00:00<00:00, 23.42it/s, total_batch_loss=385.25818, average_batch_loss=1.50491, average_batch_perplexity=4.50377]
Epoch: 1, Step: 4: 38%|███▊ | 3/8 [00:00<00:00, 23.42it/s, total_batch_loss=384.94681, average_batch_loss=1.50370, average_batch_perplexity=4.49829]
Epoch: 1, Step: 5: 38%|███▊ | 3/8 [00:00<00:00, 23.42it/s, total_batch_loss=384.94681, average_batch_loss=1.50370, average_batch_perplexity=4.49829]
Epoch: 1, Step: 5: 38%|███▊ | 3/8 [00:00<00:00, 23.42it/s, total_batch_loss=387.60138, average_batch_loss=1.51407, average_batch_perplexity=4.54518]
Epoch: 1, Step: 6: 38%|███▊ | 3/8 [00:00<00:00, 23.42it/s, total_batch_loss=387.60138, average_batch_loss=1.51407, average_batch_perplexity=4.54518]
Epoch: 1, Step: 6: 38%|███▊ | 3/8 [00:00<00:00, 23.42it/s, total_batch_loss=382.18872, average_batch_loss=1.49292, average_batch_perplexity=4.45009]
Epoch: 1, Step: 6: 75%|███████▌ | 6/8 [00:00<00:00, 23.92it/s, total_batch_loss=382.18872, average_batch_loss=1.49292, average_batch_perplexity=4.45009]
Epoch: 1, Step: 7: 75%|███████▌ | 6/8 [00:00<00:00, 23.92it/s, total_batch_loss=382.18872, average_batch_loss=1.49292, average_batch_perplexity=4.45009]
Epoch: 1, Step: 7: 75%|███████▌ | 6/8 [00:00<00:00, 23.92it/s, total_batch_loss=380.99832, average_batch_loss=1.48827, average_batch_perplexity=4.42945]
Epoch: 1, Step: 8: 75%|███████▌ | 6/8 [00:00<00:00, 23.92it/s, total_batch_loss=380.99832, average_batch_loss=1.48827, average_batch_perplexity=4.42945]
Epoch: 1, Step: 8: 75%|███████▌ | 6/8 [00:00<00:00, 23.92it/s, total_batch_loss=309.65563, average_batch_loss=1.48873, average_batch_perplexity=4.43146]
2024-12-17 09:44:00,096 - root - INFO - Total Samples: 2000
2024-12-17 09:44:00,097 - root - INFO - Total Batches: 8
2024-12-17 09:44:00,097 - root - INFO - Average Epoch Valid Loss: 1.49705
2024-12-17 09:44:00,098 - root - INFO - Average Epoch Valid Perplexity: 4.46848
2024-12-17 09:44:00,098 - root - INFO -
Evaluating and Generation.: 0%| | 0/4 [00:00<?, ?it/s]
Evaluating and Generation.: 0%| | 0/4 [00:00<?, ?it/s, accuracy: 0.0117]
Evaluating and Generation.: 0%| | 0/4 [00:00<?, ?it/s, accuracy: 0.0078]
Evaluating and Generation.: 50%|█████ | 2/4 [00:00<00:00, 10.72it/s, accuracy: 0.0078]
Evaluating and Generation.: 50%|█████ | 2/4 [00:00<00:00, 10.72it/s, accuracy: 0.0078]
Evaluating and Generation.: 50%|█████ | 2/4 [00:00<00:00, 10.72it/s, accuracy: 0.0216]
Evaluating and Generation.: 100%|██████████| 4/4 [00:00<00:00, 10.97it/s, accuracy: 0.0216]
2024-12-17 09:44:00,470 - root - INFO - Correct/Total Samples: 12/1000
2024-12-17 09:44:00,470 - root - INFO - Eval Accuracy: 0.012
2024-12-17 09:44:00,490 - root - INFO -
epoch batch_index sample_index equation generated correct
1 1 0 13+48=061 13+48=090 False
1 1 1 16+55=071 16+55=090 False
1 1 2 79+34=113 79+34=111 False
1 1 3 35+44=079 35+44=091 False
1 1 4 16+50=066 16+50=090 False
1 1 5 28+47=075 28+47=109 False
1 1 6 00+74=074 00+74=090 False
1 1 7 15+20=035 15+20=090 False
1 1 8 72+60=132 72+60=091 False
1 1 9 63+68=131 63+68=111 False
1 1 10 29+45=074 29+45=109 False
1 1 11 34+60=094 34+60=091 False
1 1 12 53+70=123 53+70=091 False
1 1 13 70+50=120 70+50=091 False
1 1 14 11+84=095 11+84=090 False
1 1 15 42+71=113 42+71=090 False
1 1 16 98+22=120 98+22=112 False
1 1 17 02+02=004 02+02=090 False
1 1 18 15+85=100 15+85=091 False
1 1 19 21+78=099 21+78=091 False
1 1 20 61+79=140 61+79=110 False
1 1 21 25+99=124 25+99=110 False
1 1 22 09+85=094 09+85=121 False
1 1 23 60+91=151 60+91=091 False
1 1 24 35+30=065 35+30=090 False
1 1 25 24+51=075 24+51=090 False
1 1 26 93+91=184 93+91=112 False
1 1 27 39+96=135 39+96=111 False
1 1 28 64+35=099 64+35=091 False
1 1 29 36+22=058 36+22=090 False
1 1 30 68+45=113 68+45=111 False
1 1 31 16+84=100 16+84=091 False
1 1 32 91+52=143 91+52=091 False
1 1 33 97+36=133 97+36=111 False
1 1 34 27+37=064 27+37=091 False
1 1 35 99+82=181 99+82=111 False
1 1 36 03+42=045 03+42=090 False
1 1 37 18+38=056 18+38=091 False
1 1 38 32+20=052 32+20=090 False
1 1 39 38+13=051 38+13=091 False
1 1 40 68+42=110 68+42=110 True
1 1 41 64+00=064 64+00=091 False
1 1 42 48+94=142 48+94=111 False
1 1 43 58+36=094 58+36=111 False
1 1 44 41+22=063 41+22=090 False
1 1 45 23+58=081 23+58=091 False
1 1 46 67+46=113 67+46=111 False
1 1 47 40+78=118 40+78=091 False
1 1 48 90+38=128 90+38=121 False
1 1 49 89+52=141 89+52=111 False
1 1 50 37+77=114 37+77=111 False
1 1 51 29+76=105 29+76=112 False
1 1 52 42+90=132 42+90=091 False
1 1 53 45+82=127 45+82=109 False
1 1 54 35+95=130 35+95=110 False
1 1 55 92+98=190 92+98=111 False
1 1 56 73+91=164 73+91=112 False
1 1 57 53+97=150 53+97=111 False
1 1 58 98+69=167 98+69=111 False
1 1 59 20+46=066 20+46=090 False
1 1 60 48+69=117 48+69=111 False
1 1 61 62+31=093 62+31=090 False
1 1 62 80+59=139 80+59=121 False
1 1 63 58+12=070 58+12=091 False
1 1 64 08+96=104 08+96=121 False
1 1 65 67+06=073 67+06=110 False
1 1 66 22+04=026 22+04=090 False
1 1 67 61+87=148 61+87=110 False
1 1 68 95+27=122 95+27=111 False
1 1 69 49+83=132 49+83=111 False
1 1 70 43+00=043 43+00=090 False
1 1 71 01+85=086 01+85=090 False
1 1 72 11+68=079 11+68=090 False
1 1 73 80+03=083 80+03=091 False
1 1 74 54+83=137 54+83=110 False
1 1 75 73+47=120 73+47=110 False
1 1 76 99+93=192 99+93=111 False
1 1 77 99+13=112 99+13=112 True
1 1 78 92+66=158 92+66=111 False
1 1 79 90+31=121 90+31=091 False
1 1 80 25+69=094 25+69=109 False
1 1 81 25+44=069 25+44=090 False
1 1 82 00+93=093 00+93=090 False
1 1 83 88+87=175 88+87=111 False
1 1 84 47+56=103 47+56=110 False
1 1 85 43+59=102 43+59=109 False
1 1 86 22+00=022 22+00=090 False
1 1 87 34+04=038 34+04=090 False
1 1 88 65+13=078 65+13=091 False
1 1 89 39+82=121 39+82=112 False
1 1 90 66+83=149 66+83=111 False
1 1 91 51+69=120 51+69=091 False
1 1 92 80+21=101 80+21=091 False
1 1 93 36+79=115 36+79=111 False
1 1 94 21+68=089 21+68=090 False
1 1 95 11+66=077 11+66=090 False
1 1 96 55+19=074 55+19=091 False
1 1 97 51+61=112 51+61=090 False
1 1 98 38+88=126 38+88=111 False
1 1 99 37+27=064 37+27=109 False
1 1 100 18+63=081 18+63=091 False
1 1 101 48+11=059 48+11=090 False
1 1 102 72+68=140 72+68=112 False
1 1 103 37+39=076 37+39=112 False
1 1 104 64+95=159 64+95=111 False
1 1 105 49+75=124 49+75=111 False
1 1 106 45+66=111 45+66=109 False
1 1 107 34+87=121 34+87=110 False
1 1 108 02+84=086 02+84=090 False
1 1 109 95+00=095 95+00=091 False
1 1 110 09+56=065 09+56=121 False
1 1 111 22+66=088 22+66=090 False
1 1 112 43+18=061 43+18=091 False
1 1 113 61+35=096 61+35=091 False
1 1 114 13+73=086 13+73=090 False
1 1 115 25+95=120 25+95=109 False
1 1 116 73+96=169 73+96=111 False
1 1 117 03+96=099 03+96=091 False
1 1 118 97+82=179 97+82=111 False
1 1 119 18+42=060 18+42=090 False
1 1 120 29+98=127 29+98=111 False
1 1 121 61+00=061 61+00=090 False
1 1 122 22+98=120 22+98=109 False
1 1 123 12+50=062 12+50=090 False
1 1 124 02+58=060 02+58=090 False
1 1 125 75+86=161 75+86=111 False
1 1 126 31+57=088 31+57=090 False
1 1 127 49+82=131 49+82=112 False
1 1 128 15+33=048 15+33=090 False
1 1 129 49+57=106 49+57=111 False
1 1 130 61+70=131 61+70=091 False
1 1 131 91+51=142 91+51=091 False
1 1 132 50+05=055 50+05=090 False
1 1 133 44+16=060 44+16=090 False
1 1 134 92+01=093 92+01=091 False
1 1 135 85+82=167 85+82=111 False
1 1 136 07+41=048 07+41=090 False
1 1 137 40+06=046 40+06=090 False
1 1 138 79+62=141 79+62=111 False
1 1 139 95+62=157 95+62=111 False
1 1 140 42+93=135 42+93=091 False
1 1 141 32+73=105 32+73=091 False
1 1 142 47+09=056 47+09=121 False
1 1 143 59+50=109 59+50=113 False
1 1 144 61+77=138 61+77=109 False
1 1 145 64+06=070 64+06=091 False
1 1 146 35+10=045 35+10=090 False
1 1 147 32+88=120 32+88=110 False
1 1 148 03+95=098 03+95=091 False
1 1 149 11+38=049 11+38=090 False
1 1 150 21+67=088 21+67=090 False
1 1 151 33+25=058 33+25=090 False
1 1 152 63+45=108 63+45=091 False
1 1 153 56+12=068 56+12=090 False
1 1 154 19+79=098 19+79=112 False
1 1 155 60+43=103 60+43=091 False
1 1 156 07+61=068 07+61=090 False
1 1 157 58+03=061 58+03=091 False
1 1 158 11+10=021 11+10=090 False
1 1 159 49+89=138 49+89=111 False
1 1 160 37+58=095 37+58=111 False
1 1 161 59+78=137 59+78=111 False
1 1 162 11+21=032 11+21=090 False
1 1 163 37+43=080 37+43=091 False
1 1 164 44+21=065 44+21=090 False
1 1 165 22+97=119 22+97=091 False
1 1 166 65+35=100 65+35=109 False
1 1 167 06+51=057 06+51=090 False
1 1 168 65+25=090 65+25=091 False
1 1 169 74+94=168 74+94=111 False
1 1 170 87+55=142 87+55=111 False
1 1 171 90+67=157 90+67=121 False
1 1 172 11+02=013 11+02=090 False
1 1 173 01+66=067 01+66=090 False
1 1 174 56+00=056 56+00=091 False
1 1 175 58+52=110 58+52=110 True
1 1 176 24+99=123 24+99=110 False
1 1 177 97+13=110 97+13=112 False
1 1 178 42+94=136 42+94=091 False
1 1 179 60+15=075 60+15=090 False
1 1 180 20+46=066 20+46=090 False
1 1 181 40+70=110 40+70=090 False
1 1 182 95+45=140 95+45=111 False
1 1 183 96+95=191 96+95=111 False
1 1 184 98+20=118 98+20=126 False
1 1 185 43+19=062 43+19=091 False
1 1 186 50+69=119 50+69=121 False
1 1 187 27+53=080 27+53=091 False
1 1 188 24+25=049 24+25=090 False
1 1 189 65+92=157 65+92=110 False
1 1 190 28+14=042 28+14=090 False
1 1 191 20+57=077 20+57=090 False
1 1 192 59+97=156 59+97=111 False
1 1 193 98+32=130 98+32=112 False
1 1 194 55+84=139 55+84=110 False
1 1 195 20+39=059 20+39=090 False
1 1 196 86+47=133 86+47=111 False
1 1 197 92+36=128 92+36=110 False
1 1 198 05+38=043 05+38=091 False
1 1 199 77+36=113 77+36=111 False
1 1 200 41+64=105 41+64=090 False
1 1 201 74+51=125 74+51=091 False
1 1 202 74+55=129 74+55=110 False
1 1 203 64+64=128 64+64=109 False
1 1 204 60+19=079 60+19=091 False
1 1 205 77+96=173 77+96=111 False
1 1 206 22+30=052 22+30=090 False
1 1 207 82+49=131 82+49=112 False
1 1 208 39+67=106 39+67=111 False
1 1 209 62+40=102 62+40=090 False
1 1 210 28+71=099 28+71=091 False
1 1 211 47+26=073 47+26=091 False
1 1 212 98+54=152 98+54=111 False
1 1 213 38+70=108 38+70=121 False
1 1 214 63+40=103 63+40=091 False
1 1 215 86+62=148 86+62=111 False
1 1 216 22+65=087 22+65=090 False
1 1 217 41+17=058 41+17=090 False
1 1 218 68+88=156 68+88=111 False
1 1 219 96+70=166 96+70=113 False
1 1 220 99+29=128 99+29=111 False
1 1 221 83+39=122 83+39=111 False
1 1 222 26+55=081 26+55=091 False
1 1 223 53+70=123 53+70=091 False
1 1 224 94+12=106 94+12=091 False
1 1 225 00+37=037 00+37=090 False
1 1 226 36+94=130 36+94=110 False
1 1 227 40+58=098 40+58=091 False
1 1 228 19+80=099 19+80=091 False
1 1 229 49+44=093 49+44=110 False
1 1 230 70+27=097 70+27=091 False
1 1 231 52+80=132 52+80=091 False
1 1 232 77+90=167 77+90=113 False
1 1 233 13+92=105 13+92=090 False
1 1 234 59+09=068 59+09=112 False
1 1 235 33+55=088 33+55=091 False
1 1 236 85+16=101 85+16=110 False
1 1 237 25+65=090 25+65=091 False
1 1 238 46+20=066 46+20=090 False
1 1 239 29+52=081 29+52=091 False
1 1 240 32+36=068 32+36=090 False
1 1 241 47+08=055 47+08=121 False
1 1 242 21+84=105 21+84=090 False
1 1 243 24+45=069 24+45=090 False
1 1 244 29+15=044 29+15=091 False
1 1 245 83+03=086 83+03=091 False
1 1 246 83+36=119 83+36=111 False
1 1 247 58+95=153 58+95=111 False
1 1 248 76+79=155 76+79=111 False
1 1 249 63+30=093 63+30=091 False
1 1 250 38+24=062 38+24=091 False
1 1 251 19+46=065 19+46=091 False
1 1 252 99+66=165 99+66=111 False
1 1 253 95+73=168 95+73=111 False
1 1 254 65+27=092 65+27=109 False
1 1 255 91+83=174 91+83=112 False
1 2 0 65+49=114 65+49=111 False
1 2 1 03+08=011 03+08=090 False
1 2 2 67+81=148 67+81=111 False
1 2 3 47+23=070 47+23=091 False
1 2 4 43+91=134 43+91=091 False
1 2 5 41+67=108 41+67=091 False
1 2 6 02+33=035 02+33=090 False
1 2 7 64+84=148 64+84=110 False
1 2 8 81+64=145 81+64=091 False
1 2 9 80+11=091 80+11=090 False
1 2 10 78+01=079 78+01=091 False
1 2 11 89+18=107 89+18=112 False
1 2 12 45+52=097 45+52=091 False
1 2 13 35+30=065 35+30=090 False
1 2 14 53+32=085 53+32=091 False
1 2 15 49+90=139 49+90=121 False
1 2 16 41+37=078 41+37=090 False
1 2 17 35+14=049 35+14=090 False
1 2 18 92+50=142 92+50=123 False
1 2 19 37+60=097 37+60=091 False
1 2 20 91+61=152 91+61=091 False
1 2 21 80+77=157 80+77=121 False
1 2 22 66+24=090 66+24=091 False
1 2 23 81+07=088 81+07=091 False
1 2 24 85+59=144 85+59=111 False
1 2 25 19+69=088 19+69=112 False
1 2 26 91+44=135 91+44=091 False
1 2 27 25+29=054 25+29=091 False
1 2 28 27+08=035 27+08=091 False
1 2 29 66+14=080 66+14=091 False
1 2 30 95+11=106 95+11=091 False
1 2 31 13+97=110 13+97=091 False
1 2 32 94+40=134 94+40=121 False
1 2 33 74+31=105 74+31=091 False
1 2 34 49+00=049 49+00=091 False
1 2 35 59+18=077 59+18=112 False
1 2 36 07+65=072 07+65=091 False
1 2 37 83+55=138 83+55=111 False
1 2 38 49+80=129 49+80=121 False
1 2 39 64+17=081 64+17=091 False
1 2 40 48+83=131 48+83=111 False
1 2 41 95+44=139 95+44=111 False
1 2 42 71+26=097 71+26=091 False
1 2 43 06+74=080 06+74=091 False
1 2 44 34+24=058 34+24=090 False
1 2 45 59+71=130 59+71=112 False
1 2 46 68+32=100 68+32=110 False
1 2 47 38+81=119 38+81=110 False
1 2 48 29+56=085 29+56=110 False
1 2 49 54+55=109 54+55=091 False
1 2 50 31+27=058 31+27=090 False
1 2 51 97+89=186 97+89=111 False
1 2 52 48+09=057 48+09=121 False
1 2 53 86+76=162 86+76=111 False
1 2 54 82+59=141 82+59=111 False
1 2 55 01+67=068 01+67=090 False
1 2 56 26+06=032 26+06=090 False
1 2 57 22+46=068 22+46=090 False
1 2 58 85+16=101 85+16=110 False
1 2 59 29+08=037 29+08=123 False
1 2 60 73+94=167 73+94=111 False
1 2 61 19+62=081 19+62=091 False
1 2 62 86+62=148 86+62=111 False
1 2 63 38+99=137 38+99=111 False
1 2 64 64+25=089 64+25=091 False
1 2 65 61+72=133 61+72=091 False
1 2 66 78+88=166 78+88=111 False
1 2 67 43+66=109 43+66=091 False
1 2 68 69+35=104 69+35=111 False
1 2 69 33+77=110 33+77=110 True
1 2 70 37+37=074 37+37=110 False
1 2 71 87+54=141 87+54=111 False
1 2 72 68+90=158 68+90=113 False
1 2 73 83+44=127 83+44=110 False
1 2 74 41+09=050 41+09=090 False
1 2 75 13+48=061 13+48=090 False
1 2 76 01+41=042 01+41=090 False
1 2 77 19+74=093 19+74=109 False
1 2 78 15+05=020 15+05=090 False
1 2 79 55+46=101 55+46=109 False
1 2 80 68+33=101 68+33=111 False
1 2 81 44+40=084 44+40=090 False
1 2 82 88+03=091 88+03=121 False
1 2 83 81+79=160 81+79=112 False
1 2 84 18+98=116 18+98=112 False
1 2 85 70+64=134 70+64=091 False
1 2 86 26+44=070 26+44=090 False
1 2 87 98+87=185 98+87=111 False
1 2 88 18+74=092 18+74=091 False
1 2 89 50+68=118 50+68=091 False
1 2 90 13+51=064 13+51=090 False
1 2 91 90+89=179 90+89=113 False
1 2 92 47+78=125 47+78=111 False
1 2 93 81+57=138 81+57=110 False
1 2 94 34+47=081 34+47=091 False
1 2 95 94+23=117 94+23=110 False
1 2 96 07+70=077 07+70=096 False
1 2 97 56+33=089 56+33=091 False
1 2 98 33+04=037 33+04=090 False
1 2 99 26+09=035 26+09=091 False
1 2 100 14+92=106 14+92=090 False
1 2 101 78+54=132 78+54=111 False
1 2 102 36+76=112 36+76=110 False
1 2 103 17+47=064 17+47=091 False
1 2 104 28+18=046 28+18=091 False
1 2 105 78+54=132 78+54=111 False
1 2 106 84+72=156 84+72=111 False
1 2 107 00+44=044 00+44=090 False
1 2 108 50+41=091 50+41=090 False
1 2 109 87+88=175 87+88=111 False
1 2 110 11+66=077 11+66=090 False
1 2 111 80+60=140 80+60=091 False
1 2 112 78+76=154 78+76=111 False
1 2 113 24+74=098 24+74=091 False
1 2 114 88+48=136 88+48=111 False
1 2 115 38+31=069 38+31=091 False
1 2 116 29+27=056 29+27=109 False
1 2 117 08+45=053 08+45=091 False
1 2 118 28+13=041 28+13=090 False
1 2 119 53+99=152 53+99=111 False
1 2 120 47+92=139 47+92=110 False
1 2 121 76+21=097 76+21=091 False
1 2 122 53+96=149 53+96=111 False
1 2 123 93+91=184 93+91=112 False
1 2 124 97+33=130 97+33=111 False
1 2 125 67+78=145 67+78=111 False
1 2 126 58+05=063 58+05=121 False
1 2 127 00+16=016 00+16=090 False
1 2 128 80+19=099 80+19=091 False
1 2 129 98+22=120 98+22=112 False
1 2 130 09+62=071 09+62=091 False
1 2 131 06+23=029 06+23=090 False
1 2 132 32+99=131 32+99=110 False
1 2 133 17+02=019 17+02=090 False
1 2 134 64+35=099 64+35=091 False
1 2 135 35+83=118 35+83=110 False
1 2 136 71+36=107 71+36=091 False
1 2 137 75+06=081 75+06=091 False
1 2 138 88+95=183 88+95=111 False
1 2 139 19+98=117 19+98=112 False
1 2 140 28+89=117 28+89=111 False
1 2 141 33+11=044 33+11=090 False
1 2 142 34+49=083 34+49=109 False
1 2 143 90+35=125 90+35=121 False
1 2 144 22+90=112 22+90=090 False
1 2 145 98+89=187 98+89=111 False
1 2 146 88+47=135 88+47=111 False
1 2 147 30+86=116 30+86=091 False
1 2 148 31+48=079 31+48=090 False
1 2 149 39+21=060 39+21=091 False
1 2 150 19+17=036 19+17=091 False
1 2 151 27+60=087 27+60=091 False
1 2 152 12+16=028 12+16=090 False
1 2 153 51+75=126 51+75=091 False
1 2 154 10+74=084 10+74=090 False
1 2 155 42+63=105 42+63=090 False
1 2 156 40+14=054 40+14=090 False
1 2 157 23+93=116 23+93=091 False
1 2 158 85+26=111 85+26=110 False
1 2 159 28+46=074 28+46=109 False
1 2 160 28+33=061 28+33=091 False
1 2 161 43+30=073 43+30=090 False
1 2 162 89+72=161 89+72=111 False
1 2 163 52+21=073 52+21=090 False
1 2 164 21+54=075 21+54=090 False
1 2 165 69+13=082 69+13=109 False
1 2 166 07+60=067 07+60=091 False
1 2 167 63+83=146 63+83=110 False
1 2 168 80+69=149 80+69=121 False
1 2 169 27+28=055 27+28=091 False
1 2 170 42+31=073 42+31=090 False
1 2 171 51+99=150 51+99=110 False
1 2 172 28+75=103 28+75=110 False
1 2 173 38+57=095 38+57=111 False
1 2 174 83+16=099 83+16=091 False
1 2 175 92+94=186 92+94=111 False
1 2 176 55+75=130 55+75=110 False
1 2 177 59+51=110 59+51=110 True
1 2 178 33+09=042 33+09=091 False
1 2 179 53+13=066 53+13=090 False
1 2 180 05+70=075 05+70=090 False
1 2 181 12+20=032 12+20=090 False
1 2 182 11+49=060 11+49=090 False
1 2 183 63+45=108 63+45=091 False
1 2 184 92+23=115 92+23=091 False
1 2 185 82+45=127 82+45=109 False
1 2 186 23+41=064 23+41=090 False
1 2 187 64+26=090 64+26=091 False
1 2 188 91+24=115 91+24=091 False
1 2 189 20+32=052 20+32=090 False
1 2 190 83+21=104 83+21=091 False
1 2 191 07+20=027 07+20=090 False
1 2 192 94+14=108 94+14=109 False
1 2 193 96+89=185 96+89=111 False
1 2 194 13+08=021 13+08=090 False
1 2 195 32+05=037 32+05=090 False
1 2 196 09+51=060 09+51=091 False
1 2 197 26+29=055 26+29=091 False
1 2 198 49+65=114 49+65=111 False
1 2 199 32+66=098 32+66=091 False
1 2 200 41+08=049 41+08=090 False
1 2 201 26+79=105 26+79=110 False
1 2 202 29+91=120 29+91=109 False
1 2 203 51+00=051 51+00=090 False
1 2 204 61+60=121 61+60=090 False
1 2 205 45+78=123 45+78=111 False
1 2 206 56+16=072 56+16=091 False
1 2 207 66+68=134 66+68=111 False
1 2 208 32+16=048 32+16=090 False
1 2 209 84+49=133 84+49=111 False
1 2 210 45+09=054 45+09=091 False
1 2 211 96+78=174 96+78=111 False
1 2 212 10+02=012 10+02=090 False
1 2 213 36+60=096 36+60=091 False
1 2 214 44+36=080 44+36=091 False
1 2 215 12+86=098 12+86=090 False
1 2 216 94+54=148 94+54=111 False
1 2 217 64+73=137 64+73=110 False
1 2 218 73+10=083 73+10=091 False
1 2 219 14+62=076 14+62=090 False
1 2 220 25+22=047 25+22=090 False
1 2 221 94+22=116 94+22=109 False
1 2 222 41+76=117 41+76=091 False
1 2 223 38+46=084 38+46=110 False
1 2 224 71+72=143 71+72=091 False
1 2 225 74+79=153 74+79=111 False
1 2 226 99+67=166 99+67=111 False
1 2 227 78+71=149 78+71=112 False
1 2 228 23+19=042 23+19=090 False
1 2 229 51+65=116 51+65=091 False
1 2 230 94+86=180 94+86=111 False
1 2 231 09+79=088 09+79=126 False
1 2 232 69+39=108 69+39=111 False
1 2 233 84+13=097 84+13=091 False
1 2 234 36+59=095 36+59=110 False
1 2 235 87+47=134 87+47=111 False
1 2 236 50+00=050 50+00=090 False
1 2 237 76+96=172 76+96=111 False
1 2 238 12+18=030 12+18=090 False
1 2 239 99+95=194 99+95=111 False
1 2 240 22+00=022 22+00=090 False
1 2 241 96+18=114 96+18=112 False
1 2 242 51+20=071 51+20=090 False
1 2 243 66+81=147 66+81=110 False
1 2 244 78+18=096 78+18=112 False
1 2 245 09+78=087 09+78=126 False
1 2 246 24+20=044 24+20=090 False
1 2 247 76+13=089 76+13=091 False
1 2 248 05+10=015 05+10=090 False
1 2 249 97+14=111 97+14=112 False
1 2 250 92+38=130 92+38=112 False
1 2 251 77+13=090 77+13=109 False
1 2 252 70+19=089 70+19=091 False
1 2 253 90+45=135 90+45=121 False
1 2 254 50+09=059 50+09=091 False
1 2 255 78+06=084 78+06=121 False
1 3 0 03+25=028 03+25=090 False
1 3 1 48+43=091 48+43=110 False
1 3 2 39+47=086 39+47=111 False
1 3 3 48+19=067 48+19=110 False
1 3 4 07+22=029 07+22=090 False
1 3 5 73+68=141 73+68=111 False
1 3 6 14+56=070 14+56=090 False
1 3 7 96+95=191 96+95=111 False
1 3 8 96+28=124 96+28=111 False
1 3 9 82+05=087 82+05=091 False
1 3 10 27+94=121 27+94=110 False
1 3 11 87+86=173 87+86=111 False
1 3 12 00+68=068 00+68=091 False
1 3 13 11+37=048 11+37=090 False
1 3 14 95+93=188 95+93=111 False
1 3 15 75+82=157 75+82=111 False
1 3 16 41+71=112 41+71=090 False
1 3 17 60+14=074 60+14=090 False
1 3 18 77+77=154 77+77=111 False
1 3 19 31+84=115 31+84=091 False
1 3 20 31+57=088 31+57=090 False
1 3 21 27+87=114 27+87=112 False
1 3 22 31+89=120 31+89=109 False
1 3 23 22+18=040 22+18=090 False
1 3 24 38+25=063 38+25=109 False
1 3 25 64+54=118 64+54=109 False
1 3 26 85+60=145 85+60=121 False
1 3 27 14+71=085 14+71=090 False
1 3 28 06+16=022 06+16=090 False
1 3 29 78+61=139 78+61=112 False
1 3 30 65+75=140 65+75=111 False
1 3 31 13+83=096 13+83=090 False
1 3 32 75+49=124 75+49=111 False
1 3 33 05+78=083 05+78=091 False
1 3 34 66+55=121 66+55=110 False
1 3 35 03+05=008 03+05=090 False
1 3 36 69+99=168 69+99=111 False
1 3 37 52+82=134 52+82=091 False
1 3 38 45+97=142 45+97=111 False
1 3 39 66+17=083 66+17=091 False
1 3 40 36+17=053 36+17=091 False
1 3 41 92+74=166 92+74=112 False
1 3 42 48+44=092 48+44=110 False
1 3 43 34+17=051 34+17=090 False
1 3 44 56+11=067 56+11=090 False
1 3 45 77+23=100 77+23=110 False
1 3 46 10+11=021 10+11=090 False
1 3 47 32+65=097 32+65=091 False
1 3 48 53+49=102 53+49=110 False
1 3 49 68+86=154 68+86=111 False
1 3 50 52+94=146 52+94=109 False
1 3 51 97+71=168 97+71=112 False
1 3 52 05+37=042 05+37=091 False
1 3 53 58+75=133 58+75=111 False
1 3 54 06+24=030 06+24=090 False
1 3 55 15+44=059 15+44=090 False
1 3 56 90+49=139 90+49=121 False
1 3 57 50+37=087 50+37=091 False
1 3 58 88+61=149 88+61=111 False
1 3 59 21+57=078 21+57=090 False
1 3 60 24+85=109 24+85=091 False
1 3 61 01+66=067 01+66=090 False
1 3 62 50+46=096 50+46=091 False
1 3 63 76+65=141 76+65=111 False
1 3 64 23+74=097 23+74=091 False
1 3 65 76+16=092 76+16=109 False
1 3 66 06+08=014 06+08=091 False
1 3 67 69+25=094 69+25=111 False
1 3 68 15+23=038 15+23=090 False
1 3 69 41+02=043 41+02=090 False
1 3 70 16+66=082 16+66=091 False
1 3 71 59+94=153 59+94=111 False
1 3 72 32+88=120 32+88=110 False
1 3 73 46+21=067 46+21=090 False
1 3 74 57+28=085 57+28=110 False
1 3 75 00+31=031 00+31=090 False
1 3 76 77+07=084 77+07=126 False
1 3 77 28+70=098 28+70=091 False
1 3 78 05+61=066 05+61=090 False
1 3 79 22+09=031 22+09=090 False
1 3 80 08+94=102 08+94=121 False
1 3 81 40+11=051 40+11=090 False
1 3 82 10+48=058 10+48=090 False
1 3 83 27+56=083 27+56=091 False
1 3 84 42+16=058 42+16=090 False
1 3 85 69+43=112 69+43=111 False
1 3 86 57+69=126 57+69=111 False
1 3 87 18+86=104 18+86=110 False
1 3 88 86+80=166 86+80=113 False
1 3 89 30+85=115 30+85=091 False
1 3 90 77+66=143 77+66=111 False
1 3 91 39+64=103 39+64=110 False
1 3 92 76+61=137 76+61=110 False
1 3 93 42+61=103 42+61=090 False
1 3 94 07+30=037 07+30=090 False
1 3 95 35+93=128 35+93=110 False
1 3 96 40+90=130 40+90=091 False
1 3 97 08+91=099 08+91=091 False
1 3 98 62+34=096 62+34=091 False
1 3 99 86+49=135 86+49=111 False
1 3 100 73+23=096 73+23=091 False
1 3 101 87+35=122 87+35=111 False
1 3 102 35+31=066 35+31=090 False
1 3 103 07+13=020 07+13=090 False
1 3 104 39+41=080 39+41=091 False
1 3 105 44+63=107 44+63=091 False
1 3 106 94+66=160 94+66=111 False
1 3 107 49+54=103 49+54=110 False
1 3 108 79+46=125 79+46=111 False
1 3 109 53+12=065 53+12=090 False
1 3 110 60+92=152 60+92=091 False
1 3 111 25+60=085 25+60=090 False
1 3 112 64+53=117 64+53=091 False
1 3 113 41+02=043 41+02=090 False
1 3 114 00+97=097 00+97=096 False
1 3 115 12+52=064 12+52=090 False
1 3 116 39+50=089 39+50=121 False
1 3 117 87+21=108 87+21=110 False
1 3 118 04+99=103 04+99=123 False
1 3 119 19+75=094 19+75=109 False
1 3 120 90+05=095 90+05=091 False
1 3 121 54+39=093 54+39=110 False
1 3 122 29+26=055 29+26=091 False
1 3 123 82+95=177 82+95=111 False
1 3 124 55+09=064 55+09=109 False
1 3 125 02+62=064 02+62=090 False
1 3 126 68+30=098 68+30=121 False
1 3 127 99+16=115 99+16=112 False
1 3 128 63+11=074 63+11=090 False
1 3 129 42+92=134 42+92=091 False
1 3 130 99+16=115 99+16=112 False
1 3 131 50+31=081 50+31=090 False
1 3 132 23+46=069 23+46=090 False
1 3 133 45+73=118 45+73=109 False
1 3 134 89+77=166 89+77=111 False
1 3 135 45+78=123 45+78=111 False
1 3 136 96+60=156 96+60=113 False
1 3 137 74+61=135 74+61=091 False
1 3 138 87+01=088 87+01=091 False
1 3 139 63+88=151 63+88=111 False
1 3 140 59+72=131 59+72=111 False
1 3 141 17+96=113 17+96=110 False
1 3 142 89+77=166 89+77=111 False
1 3 143 24+69=093 24+69=091 False
1 3 144 75+83=158 75+83=111 False
1 3 145 50+54=104 50+54=091 False
1 3 146 93+47=140 93+47=111 False
1 3 147 20+55=075 20+55=090 False
1 3 148 91+79=170 91+79=112 False
1 3 149 15+13=028 15+13=090 False
1 3 150 86+09=095 86+09=113 False
1 3 151 29+58=087 29+58=112 False
1 3 152 01+29=030 01+29=090 False
1 3 153 65+48=113 65+48=111 False
1 3 154 96+45=141 96+45=111 False
1 3 155 58+69=127 58+69=111 False
1 3 156 84+43=127 84+43=110 False
1 3 157 90+38=128 90+38=121 False
1 3 158 39+97=136 39+97=111 False
1 3 159 74+84=158 74+84=111 False
1 3 160 86+22=108 86+22=110 False
1 3 161 01+86=087 01+86=090 False
1 3 162 81+63=144 81+63=091 False
1 3 163 80+94=174 80+94=121 False
1 3 164 44+42=086 44+42=090 False
1 3 165 72+60=132 72+60=091 False
1 3 166 28+07=035 28+07=091 False
1 3 167 69+54=123 69+54=111 False
1 3 168 68+77=145 68+77=111 False
1 3 169 90+16=106 90+16=091 False
1 3 170 64+50=114 64+50=091 False
1 3 171 46+88=134 46+88=111 False
1 3 172 55+99=154 55+99=111 False
1 3 173 31+97=128 31+97=109 False
1 3 174 79+28=107 79+28=111 False
1 3 175 81+43=124 81+43=091 False
1 3 176 41+15=056 41+15=090 False
1 3 177 38+77=115 38+77=111 False
1 3 178 25+06=031 25+06=090 False
1 3 179 01+93=094 01+93=090 False
1 3 180 97+22=119 97+22=112 False
1 3 181 71+84=155 71+84=109 False
1 3 182 26+36=062 26+36=091 False
1 3 183 60+92=152 60+92=091 False
1 3 184 02+94=096 02+94=090 False
1 3 185 31+58=089 31+58=091 False
1 3 186 70+52=122 70+52=091 False
1 3 187 19+42=061 19+42=090 False
1 3 188 95+73=168 95+73=111 False
1 3 189 21+25=046 21+25=090 False
1 3 190 13+58=071 13+58=090 False
1 3 191 62+28=090 62+28=091 False
1 3 192 38+14=052 38+14=091 False
1 3 193 66+75=141 66+75=111 False
1 3 194 24+59=083 24+59=091 False
1 3 195 97+66=163 97+66=111 False
1 3 196 76+70=146 76+70=113 False
1 3 197 08+40=048 08+40=091 False
1 3 198 84+00=084 84+00=091 False
1 3 199 54+73=127 54+73=109 False
1 3 200 16+88=104 16+88=110 False
1 3 201 99+47=146 99+47=111 False
1 3 202 31+95=126 31+95=091 False
1 3 203 01+79=080 01+79=091 False
1 3 204 03+68=071 03+68=091 False
1 3 205 10+05=015 10+05=090 False
1 3 206 98+90=188 98+90=113 False
1 3 207 58+53=111 58+53=111 True
1 3 208 34+87=121 34+87=110 False
1 3 209 07+31=038 07+31=090 False
1 3 210 59+08=067 59+08=121 False
1 3 211 51+38=089 51+38=091 False
1 3 212 62+62=124 62+62=091 False
1 3 213 80+32=112 80+32=091 False
1 3 214 69+16=085 69+16=110 False
1 3 215 01+17=018 01+17=090 False
1 3 216 74+41=115 74+41=091 False
1 3 217 20+89=109 20+89=091 False
1 3 218 53+50=103 53+50=091 False
1 3 219 82+85=167 82+85=111 False
1 3 220 34+47=081 34+47=091 False
1 3 221 34+45=079 34+45=091 False
1 3 222 77+34=111 77+34=111 True
1 3 223 56+33=089 56+33=091 False
1 3 224 97+56=153 97+56=111 False
1 3 225 29+06=035 29+06=091 False
1 3 226 78+96=174 78+96=111 False
1 3 227 28+65=093 28+65=109 False
1 3 228 61+64=125 61+64=091 False
1 3 229 32+64=096 32+64=090 False
1 3 230 98+32=130 98+32=112 False
1 3 231 25+35=060 25+35=090 False
1 3 232 05+08=013 05+08=091 False
1 3 233 05+26=031 05+26=090 False
1 3 234 84+71=155 84+71=110 False
1 3 235 33+10=043 33+10=090 False
1 3 236 98+35=133 98+35=111 False
1 3 237 68+98=166 68+98=111 False
1 3 238 03+63=066 03+63=090 False
1 3 239 12+96=108 12+96=090 False
1 3 240 02+81=083 02+81=090 False
1 3 241 83+13=096 83+13=091 False
1 3 242 55+92=147 55+92=110 False
1 3 243 96+09=105 96+09=113 False
1 3 244 61+08=069 61+08=091 False
1 3 245 39+75=114 39+75=111 False
1 3 246 40+74=114 40+74=091 False
1 3 247 39+80=119 39+80=121 False
1 3 248 57+95=152 57+95=111 False
1 3 249 92+97=189 92+97=111 False
1 3 250 33+03=036 33+03=090 False
1 3 251 74+92=166 74+92=111 False
1 3 252 99+09=108 99+09=113 False
1 3 253 98+10=108 98+10=123 False
1 3 254 46+77=123 46+77=111 False
1 3 255 85+78=163 85+78=111 False
1 4 0 41+21=062 41+21=090 False
1 4 1 49+13=062 49+13=091 False
1 4 2 59+07=066 59+07=121 False
1 4 3 31+11=042 31+11=090 False
1 4 4 74+16=090 74+16=091 False
1 4 5 43+38=081 43+38=091 False
1 4 6 08+67=075 08+67=121 False
1 4 7 31+66=097 31+66=090 False
1 4 8 10+31=041 10+31=090 False
1 4 9 34+59=093 34+59=109 False
1 4 10 78+42=120 78+42=111 False
1 4 11 13+41=054 13+41=090 False
1 4 12 97+89=186 97+89=111 False
1 4 13 15+62=077 15+62=090 False
1 4 14 39+36=075 39+36=110 False
1 4 15 21+25=046 21+25=090 False
1 4 16 74+56=130 74+56=110 False
1 4 17 85+47=132 85+47=111 False
1 4 18 47+32=079 47+32=091 False
1 4 19 37+66=103 37+66=110 False
1 4 20 16+29=045 16+29=090 False
1 4 21 86+77=163 86+77=111 False
1 4 22 80+07=087 80+07=091 False
1 4 23 87+05=092 87+05=121 False
1 4 24 58+16=074 58+16=109 False
1 4 25 52+79=131 52+79=110 False
1 4 26 91+08=099 91+08=123 False
1 4 27 47+78=125 47+78=111 False
1 4 28 86+96=182 86+96=111 False
1 4 29 90+22=112 90+22=091 False
1 4 30 31+18=049 31+18=090 False
1 4 31 86+15=101 86+15=110 False
1 4 32 15+95=110 15+95=091 False
1 4 33 42+11=053 42+11=090 False
1 4 34 65+99=164 65+99=111 False
1 4 35 89+29=118 89+29=111 False
1 4 36 35+11=046 35+11=090 False
1 4 37 71+41=112 71+41=090 False
1 4 38 16+24=040 16+24=090 False
1 4 39 77+82=159 77+82=111 False
1 4 40 55+89=144 55+89=111 False
1 4 41 17+88=105 17+88=112 False
1 4 42 54+72=126 54+72=091 False
1 4 43 34+98=132 34+98=112 False
1 4 44 09+97=106 09+97=126 False
1 4 45 91+07=098 91+07=091 False
1 4 46 55+94=149 55+94=110 False
1 4 47 22+58=080 22+58=090 False
1 4 48 91+37=128 91+37=110 False
1 4 49 16+10=026 16+10=090 False
1 4 50 96+32=128 96+32=111 False
1 4 51 35+75=110 35+75=109 False
1 4 52 88+73=161 88+73=111 False
1 4 53 35+18=053 35+18=091 False
1 4 54 33+10=043 33+10=090 False
1 4 55 08+50=058 08+50=091 False
1 4 56 22+62=084 22+62=090 False
1 4 57 26+37=063 26+37=091 False
1 4 58 80+27=107 80+27=091 False
1 4 59 68+28=096 68+28=111 False
1 4 60 48+03=051 48+03=091 False
1 4 61 40+18=058 40+18=090 False
1 4 62 16+59=075 16+59=091 False
1 4 63 02+19=021 02+19=090 False
1 4 64 01+09=010 01+09=090 False
1 4 65 62+68=130 62+68=110 False
1 4 66 09+71=080 09+71=091 False
1 4 67 00+58=058 00+58=090 False
1 4 68 16+45=061 16+45=090 False
1 4 69 24+98=122 24+98=110 False
1 4 70 47+92=139 47+92=110 False
1 4 71 94+84=178 94+84=111 False
1 4 72 21+32=053 21+32=090 False
1 4 73 29+82=111 29+82=110 False
1 4 74 32+79=111 32+79=109 False
1 4 75 13+98=111 13+98=109 False
1 4 76 41+94=135 41+94=091 False
1 4 77 51+84=135 51+84=091 False
1 4 78 42+05=047 42+05=090 False
1 4 79 39+03=042 39+03=091 False
1 4 80 02+92=094 02+92=090 False
1 4 81 99+81=180 99+81=111 False
1 4 82 32+68=100 32+68=091 False
1 4 83 52+17=069 52+17=090 False
1 4 84 56+58=114 56+58=111 False
1 4 85 21+48=069 21+48=090 False
1 4 86 61+71=132 61+71=090 False
1 4 87 17+01=018 17+01=090 False
1 4 88 68+23=091 68+23=110 False
1 4 89 00+37=037 00+37=090 False
1 4 90 94+88=182 94+88=111 False
1 4 91 06+31=037 06+31=090 False
1 4 92 27+18=045 27+18=091 False
1 4 93 41+81=122 41+81=090 False
1 4 94 15+86=101 15+86=091 False
1 4 95 36+87=123 36+87=111 False
1 4 96 17+37=054 17+37=091 False
1 4 97 13+86=099 13+86=091 False
1 4 98 29+69=098 29+69=112 False
1 4 99 31+99=130 31+99=109 False
1 4 100 47+29=076 47+29=110 False
1 4 101 08+81=089 08+81=091 False
1 4 102 72+82=154 72+82=110 False
1 4 103 46+91=137 46+91=109 False
1 4 104 70+35=105 70+35=091 False
1 4 105 90+55=145 90+55=121 False
1 4 106 99+99=198 99+99=111 False
1 4 107 60+97=157 60+97=121 False
1 4 108 03+40=043 03+40=090 False
1 4 109 35+49=084 35+49=109 False
1 4 110 32+02=034 32+02=090 False
1 4 111 70+18=088 70+18=091 False
1 4 112 99+05=104 99+05=113 False
1 4 113 78+73=151 78+73=111 False
1 4 114 03+02=005 03+02=090 False
1 4 115 50+14=064 50+14=090 False
1 4 116 62+02=064 62+02=090 False
1 4 117 16+74=090 16+74=091 False
1 4 118 68+65=133 68+65=111 False
1 4 119 74+81=155 74+81=110 False
1 4 120 37+48=085 37+48=110 False
1 4 121 63+04=067 63+04=091 False
1 4 122 06+62=068 06+62=090 False
1 4 123 95+75=170 95+75=111 False
1 4 124 92+37=129 92+37=112 False
1 4 125 81+32=113 81+32=091 False
1 4 126 53+28=081 53+28=091 False
1 4 127 52+42=094 52+42=090 False
1 4 128 66+97=163 66+97=111 False
1 4 129 00+48=048 00+48=090 False
1 4 130 65+32=097 65+32=091 False
1 4 131 60+89=149 60+89=112 False
1 4 132 71+61=132 71+61=091 False
1 4 133 98+50=148 98+50=113 False
1 4 134 90+96=186 90+96=113 False
1 4 135 02+96=098 02+96=091 False
1 4 136 62+75=137 62+75=109 False
1 4 137 41+28=069 41+28=090 False
1 4 138 95+79=174 95+79=111 False
1 4 139 48+41=089 48+41=091 False
1 4 140 87+95=182 87+95=111 False
1 4 141 75+38=113 75+38=111 False
1 4 142 31+55=086 31+55=090 False
1 4 143 54+63=117 54+63=091 False
1 4 144 75+82=157 75+82=111 False
1 4 145 46+45=091 46+45=091 True
1 4 146 13+08=021 13+08=090 False
1 4 147 77+97=174 77+97=111 False
1 4 148 37+35=072 37+35=109 False
1 4 149 21+89=110 21+89=091 False
1 4 150 58+51=109 58+51=109 True
1 4 151 91+48=139 91+48=112 False
1 4 152 33+23=056 33+23=090 False
1 4 153 80+96=176 80+96=121 False
1 4 154 78+02=080 78+02=123 False
1 4 155 38+95=133 38+95=111 False
1 4 156 99+25=124 99+25=111 False
1 4 157 30+76=106 30+76=091 False
1 4 158 42+40=082 42+40=090 False
1 4 159 85+58=143 85+58=111 False
1 4 160 44+46=090 44+46=091 False
1 4 161 06+41=047 06+41=090 False
1 4 162 65+90=155 65+90=112 False
1 4 163 43+83=126 43+83=109 False
1 4 164 36+61=097 36+61=091 False
1 4 165 61+51=112 61+51=090 False
1 4 166 38+09=047 38+09=121 False
1 4 167 21+97=118 21+97=091 False
1 4 168 83+30=113 83+30=091 False
1 4 169 11+79=090 11+79=090 True
1 4 170 14+29=043 14+29=090 False
1 4 171 21+11=032 21+11=090 False
1 4 172 43+53=096 43+53=091 False
1 4 173 02+58=060 02+58=090 False
1 4 174 78+82=160 78+82=111 False
1 4 175 91+11=102 91+11=090 False
1 4 176 58+54=112 58+54=111 False
1 4 177 00+15=015 00+15=090 False
1 4 178 83+51=134 83+51=091 False
1 4 179 44+72=116 44+72=091 False
1 4 180 71+20=091 71+20=090 False
1 4 181 24+99=123 24+99=110 False
1 4 182 46+30=076 46+30=091 False
1 4 183 08+67=075 08+67=121 False
1 4 184 47+42=089 47+42=091 False
1 4 185 95+67=162 95+67=111 False
1 4 186 40+56=096 40+56=091 False
1 4 187 17+95=112 17+95=109 False
1 4 188 94+66=160 94+66=111 False
1 4 189 14+58=072 14+58=090 False
1 4 190 56+05=061 56+05=091 False
1 4 191 70+01=071 70+01=090 False
1 4 192 97+59=156 97+59=111 False
1 4 193 94+67=161 94+67=111 False
1 4 194 13+41=054 13+41=090 False
1 4 195 85+15=100 85+15=109 False
1 4 196 48+53=101 48+53=110 False
1 4 197 62+75=137 62+75=109 False
1 4 198 87+47=134 87+47=111 False
1 4 199 31+88=119 31+88=091 False
1 4 200 97+16=113 97+16=112 False
1 4 201 48+45=093 48+45=110 False
1 4 202 99+00=099 99+00=136 False
1 4 203 15+01=016 15+01=090 False
1 4 204 28+96=124 28+96=112 False
1 4 205 20+11=031 20+11=090 False
1 4 206 07+56=063 07+56=091 False
1 4 207 06+08=014 06+08=091 False
1 4 208 45+46=091 45+46=091 True
1 4 209 48+85=133 48+85=111 False
1 4 210 62+14=076 62+14=090 False
1 4 211 82+31=113 82+31=091 False
1 4 212 85+88=173 85+88=111 False
1 4 213 77+08=085 77+08=126 False
1 4 214 16+64=080 16+64=090 False
1 4 215 00+27=027 00+27=090 False
1 4 216 36+75=111 36+75=110 False
1 4 217 38+38=076 38+38=112 False
1 4 218 88+32=120 88+32=112 False
1 4 219 09+88=097 09+88=126 False
1 4 220 96+87=183 96+87=111 False
1 4 221 71+29=100 71+29=091 False
1 4 222 99+13=112 99+13=112 True
1 4 223 03+13=016 03+13=090 False
1 4 224 67+23=090 67+23=109 False
1 4 225 15+98=113 15+98=109 False
1 4 226 10+08=018 10+08=090 False
1 4 227 46+24=070 46+24=091 False
1 4 228 55+63=118 55+63=109 False
1 4 229 28+06=034 28+06=091 False
1 4 230 43+87=130 43+87=110 False
1 4 231 34+05=039 34+05=090 False
2024-12-17 09:44:00,491 - root - WARNING - The method `test_one_epoch` is not implemented. Please override this method in a subclass or use a custom callback.
2024-12-17 09:44:00,492 - root - INFO - ====================================================== Starting Train Epoch: 2/9 ======================================================
2024-12-17 09:44:00,493 - root - INFO - Learning rates for each parameter group: 0.00066589243287212298, 0.00066589243287212298
0%| | 0/28 [00:00<?, ?it/s]
Epoch: 2, Step: 1: 0%| | 0/28 [00:00<?, ?it/s]
Epoch: 2, Step: 1: 0%| | 0/28 [00:00<?, ?it/s, total_batch_loss=387.97672, average_batch_loss=1.51553, average_batch_perplexity=4.55185, lr=0.000665892, 0.000665892]
Epoch: 2, Step: 2: 0%| | 0/28 [00:00<?, ?it/s, total_batch_loss=387.97672, average_batch_loss=1.51553, average_batch_perplexity=4.55185, lr=0.000665892, 0.000665892]
Epoch: 2, Step: 2: 0%| | 0/28 [00:00<?, ?it/s, total_batch_loss=386.84814, average_batch_loss=1.51113, average_batch_perplexity=4.53183, lr=0.000688854, 0.000688854]
Epoch: 2, Step: 2: 7%|▋ | 2/28 [00:00<00:02, 11.08it/s, total_batch_loss=386.84814, average_batch_loss=1.51113, average_batch_perplexity=4.53183, lr=0.000688854, 0.000688854]
Epoch: 2, Step: 3: 7%|▋ | 2/28 [00:00<00:02, 11.08it/s, total_batch_loss=386.84814, average_batch_loss=1.51113, average_batch_perplexity=4.53183, lr=0.000688854, 0.000688854]
Epoch: 2, Step: 3: 7%|▋ | 2/28 [00:00<00:02, 11.08it/s, total_batch_loss=379.06183, average_batch_loss=1.48071, average_batch_perplexity=4.39607, lr=0.000711816, 0.000711816]
Epoch: 2, Step: 4: 7%|▋ | 2/28 [00:00<00:02, 11.08it/s, total_batch_loss=379.06183, average_batch_loss=1.48071, average_batch_perplexity=4.39607, lr=0.000711816, 0.000711816]
Epoch: 2, Step: 4: 7%|▋ | 2/28 [00:00<00:02, 11.08it/s, total_batch_loss=372.22934, average_batch_loss=1.45402, average_batch_perplexity=4.28029, lr=0.000734778, 0.000734778]
Epoch: 2, Step: 4: 14%|█▍ | 4/28 [00:00<00:01, 12.05it/s, total_batch_loss=372.22934, average_batch_loss=1.45402, average_batch_perplexity=4.28029, lr=0.000734778, 0.000734778]
Epoch: 2, Step: 5: 14%|█▍ | 4/28 [00:00<00:01, 12.05it/s, total_batch_loss=372.22934, average_batch_loss=1.45402, average_batch_perplexity=4.28029, lr=0.000734778, 0.000734778]
Epoch: 2, Step: 5: 14%|█▍ | 4/28 [00:00<00:01, 12.05it/s, total_batch_loss=369.99042, average_batch_loss=1.44528, average_batch_perplexity=4.24302, lr=0.000757740, 0.000757740]
Epoch: 2, Step: 6: 14%|█▍ | 4/28 [00:00<00:01, 12.05it/s, total_batch_loss=369.99042, average_batch_loss=1.44528, average_batch_perplexity=4.24302, lr=0.000757740, 0.000757740]
Epoch: 2, Step: 6: 14%|█▍ | 4/28 [00:00<00:01, 12.05it/s, total_batch_loss=359.53345, average_batch_loss=1.40443, average_batch_perplexity=4.07319, lr=0.000780701, 0.000780701]
Epoch: 2, Step: 6: 21%|██▏ | 6/28 [00:00<00:01, 12.17it/s, total_batch_loss=359.53345, average_batch_loss=1.40443, average_batch_perplexity=4.07319, lr=0.000780701, 0.000780701]
Epoch: 2, Step: 7: 21%|██▏ | 6/28 [00:00<00:01, 12.17it/s, total_batch_loss=359.53345, average_batch_loss=1.40443, average_batch_perplexity=4.07319, lr=0.000780701, 0.000780701]
Epoch: 2, Step: 7: 21%|██▏ | 6/28 [00:00<00:01, 12.17it/s, total_batch_loss=357.81116, average_batch_loss=1.39770, average_batch_perplexity=4.04588, lr=0.000803663, 0.000803663]
Epoch: 2, Step: 8: 21%|██▏ | 6/28 [00:00<00:01, 12.17it/s, total_batch_loss=357.81116, average_batch_loss=1.39770, average_batch_perplexity=4.04588, lr=0.000803663, 0.000803663]
Epoch: 2, Step: 8: 21%|██▏ | 6/28 [00:00<00:01, 12.17it/s, total_batch_loss=350.02820, average_batch_loss=1.36730, average_batch_perplexity=3.92473, lr=0.000826625, 0.000826625]
Epoch: 2, Step: 8: 29%|██▊ | 8/28 [00:00<00:01, 12.47it/s, total_batch_loss=350.02820, average_batch_loss=1.36730, average_batch_perplexity=3.92473, lr=0.000826625, 0.000826625]
Epoch: 2, Step: 9: 29%|██▊ | 8/28 [00:00<00:01, 12.47it/s, total_batch_loss=350.02820, average_batch_loss=1.36730, average_batch_perplexity=3.92473, lr=0.000826625, 0.000826625]
Epoch: 2, Step: 9: 29%|██▊ | 8/28 [00:00<00:01, 12.47it/s, total_batch_loss=346.96631, average_batch_loss=1.35534, average_batch_perplexity=3.87807, lr=0.000849587, 0.000849587]
Epoch: 2, Step: 10: 29%|██▊ | 8/28 [00:00<00:01, 12.47it/s, total_batch_loss=346.96631, average_batch_loss=1.35534, average_batch_perplexity=3.87807, lr=0.000849587, 0.000849587]
Epoch: 2, Step: 10: 29%|██▊ | 8/28 [00:00<00:01, 12.47it/s, total_batch_loss=338.66644, average_batch_loss=1.32292, average_batch_perplexity=3.75435, lr=0.000872549, 0.000872549]
Epoch: 2, Step: 10: 36%|███▌ | 10/28 [00:00<00:01, 12.56it/s, total_batch_loss=338.66644, average_batch_loss=1.32292, average_batch_perplexity=3.75435, lr=0.000872549, 0.000872549]
Epoch: 2, Step: 11: 36%|███▌ | 10/28 [00:00<00:01, 12.56it/s, total_batch_loss=338.66644, average_batch_loss=1.32292, average_batch_perplexity=3.75435, lr=0.000872549, 0.000872549]
Epoch: 2, Step: 11: 36%|███▌ | 10/28 [00:00<00:01, 12.56it/s, total_batch_loss=334.35333, average_batch_loss=1.30607, average_batch_perplexity=3.69163, lr=0.000895511, 0.000895511]
Epoch: 2, Step: 12: 36%|███▌ | 10/28 [00:00<00:01, 12.56it/s, total_batch_loss=334.35333, average_batch_loss=1.30607, average_batch_perplexity=3.69163, lr=0.000895511, 0.000895511]
Epoch: 2, Step: 12: 36%|███▌ | 10/28 [00:00<00:01, 12.56it/s, total_batch_loss=331.53091, average_batch_loss=1.29504, average_batch_perplexity=3.65115, lr=0.000918472, 0.000918472]
Epoch: 2, Step: 12: 43%|████▎ | 12/28 [00:00<00:01, 12.38it/s, total_batch_loss=331.53091, average_batch_loss=1.29504, average_batch_perplexity=3.65115, lr=0.000918472, 0.000918472]
Epoch: 2, Step: 13: 43%|████▎ | 12/28 [00:01<00:01, 12.38it/s, total_batch_loss=331.53091, average_batch_loss=1.29504, average_batch_perplexity=3.65115, lr=0.000918472, 0.000918472]
Epoch: 2, Step: 13: 43%|████▎ | 12/28 [00:01<00:01, 12.38it/s, total_batch_loss=327.52557, average_batch_loss=1.27940, average_batch_perplexity=3.59447, lr=0.000941434, 0.000941434]
Epoch: 2, Step: 14: 43%|████▎ | 12/28 [00:01<00:01, 12.38it/s, total_batch_loss=327.52557, average_batch_loss=1.27940, average_batch_perplexity=3.59447, lr=0.000941434, 0.000941434]
Epoch: 2, Step: 14: 43%|████▎ | 12/28 [00:01<00:01, 12.38it/s, total_batch_loss=324.04407, average_batch_loss=1.26580, average_batch_perplexity=3.54592, lr=0.000964396, 0.000964396]
Epoch: 2, Step: 14: 50%|█████ | 14/28 [00:01<00:01, 12.35it/s, total_batch_loss=324.04407, average_batch_loss=1.26580, average_batch_perplexity=3.54592, lr=0.000964396, 0.000964396]
Epoch: 2, Step: 15: 50%|█████ | 14/28 [00:01<00:01, 12.35it/s, total_batch_loss=324.04407, average_batch_loss=1.26580, average_batch_perplexity=3.54592, lr=0.000964396, 0.000964396]
Epoch: 2, Step: 15: 50%|█████ | 14/28 [00:01<00:01, 12.35it/s, total_batch_loss=313.24115, average_batch_loss=1.22360, average_batch_perplexity=3.39940, lr=0.000987358, 0.000987358]
Epoch: 2, Step: 16: 50%|█████ | 14/28 [00:01<00:01, 12.35it/s, total_batch_loss=313.24115, average_batch_loss=1.22360, average_batch_perplexity=3.39940, lr=0.000987358, 0.000987358]
Epoch: 2, Step: 16: 50%|█████ | 14/28 [00:01<00:01, 12.35it/s, total_batch_loss=315.94284, average_batch_loss=1.23415, average_batch_perplexity=3.43546, lr=0.001010320, 0.001010320]
Epoch: 2, Step: 16: 57%|█████▋ | 16/28 [00:01<00:00, 12.28it/s, total_batch_loss=315.94284, average_batch_loss=1.23415, average_batch_perplexity=3.43546, lr=0.001010320, 0.001010320]
Epoch: 2, Step: 17: 57%|█████▋ | 16/28 [00:01<00:00, 12.28it/s, total_batch_loss=315.94284, average_batch_loss=1.23415, average_batch_perplexity=3.43546, lr=0.001010320, 0.001010320]
Epoch: 2, Step: 17: 57%|█████▋ | 16/28 [00:01<00:00, 12.28it/s, total_batch_loss=305.40536, average_batch_loss=1.19299, average_batch_perplexity=3.29692, lr=0.001033281, 0.001033281]
Epoch: 2, Step: 18: 57%|█████▋ | 16/28 [00:01<00:00, 12.28it/s, total_batch_loss=305.40536, average_batch_loss=1.19299, average_batch_perplexity=3.29692, lr=0.001033281, 0.001033281]
Epoch: 2, Step: 18: 57%|█████▋ | 16/28 [00:01<00:00, 12.28it/s, total_batch_loss=301.18091, average_batch_loss=1.17649, average_batch_perplexity=3.24296, lr=0.001056243, 0.001056243]
Epoch: 2, Step: 18: 64%|██████▍ | 18/28 [00:01<00:00, 12.32it/s, total_batch_loss=301.18091, average_batch_loss=1.17649, average_batch_perplexity=3.24296, lr=0.001056243, 0.001056243]
Epoch: 2, Step: 19: 64%|██████▍ | 18/28 [00:01<00:00, 12.32it/s, total_batch_loss=301.18091, average_batch_loss=1.17649, average_batch_perplexity=3.24296, lr=0.001056243, 0.001056243]
Epoch: 2, Step: 19: 64%|██████▍ | 18/28 [00:01<00:00, 12.32it/s, total_batch_loss=299.07318, average_batch_loss=1.16825, average_batch_perplexity=3.21637, lr=0.001079205, 0.001079205]
Epoch: 2, Step: 20: 64%|██████▍ | 18/28 [00:01<00:00, 12.32it/s, total_batch_loss=299.07318, average_batch_loss=1.16825, average_batch_perplexity=3.21637, lr=0.001079205, 0.001079205]
Epoch: 2, Step: 20: 64%|██████▍ | 18/28 [00:01<00:00, 12.32it/s, total_batch_loss=304.44934, average_batch_loss=1.18926, average_batch_perplexity=3.28463, lr=0.001102167, 0.001102167]
Epoch: 2, Step: 20: 71%|███████▏ | 20/28 [00:01<00:00, 12.41it/s, total_batch_loss=304.44934, average_batch_loss=1.18926, average_batch_perplexity=3.28463, lr=0.001102167, 0.001102167]
Epoch: 2, Step: 21: 71%|███████▏ | 20/28 [00:01<00:00, 12.41it/s, total_batch_loss=304.44934, average_batch_loss=1.18926, average_batch_perplexity=3.28463, lr=0.001102167, 0.001102167]
Epoch: 2, Step: 21: 71%|███████▏ | 20/28 [00:01<00:00, 12.41it/s, total_batch_loss=292.82129, average_batch_loss=1.14383, average_batch_perplexity=3.13878, lr=0.001125129, 0.001125129]
Epoch: 2, Step: 22: 71%|███████▏ | 20/28 [00:01<00:00, 12.41it/s, total_batch_loss=292.82129, average_batch_loss=1.14383, average_batch_perplexity=3.13878, lr=0.001125129, 0.001125129]
Epoch: 2, Step: 22: 71%|███████▏ | 20/28 [00:01<00:00, 12.41it/s, total_batch_loss=289.08966, average_batch_loss=1.12926, average_batch_perplexity=3.09336, lr=0.001148090, 0.001148090]
Epoch: 2, Step: 22: 79%|███████▊ | 22/28 [00:01<00:00, 12.53it/s, total_batch_loss=289.08966, average_batch_loss=1.12926, average_batch_perplexity=3.09336, lr=0.001148090, 0.001148090]
Epoch: 2, Step: 23: 79%|███████▊ | 22/28 [00:01<00:00, 12.53it/s, total_batch_loss=289.08966, average_batch_loss=1.12926, average_batch_perplexity=3.09336, lr=0.001148090, 0.001148090]
Epoch: 2, Step: 23: 79%|███████▊ | 22/28 [00:01<00:00, 12.53it/s, total_batch_loss=285.48624, average_batch_loss=1.11518, average_batch_perplexity=3.05012, lr=0.001171052, 0.001171052]
Epoch: 2, Step: 24: 79%|███████▊ | 22/28 [00:01<00:00, 12.53it/s, total_batch_loss=285.48624, average_batch_loss=1.11518, average_batch_perplexity=3.05012, lr=0.001171052, 0.001171052]
Epoch: 2, Step: 24: 79%|███████▊ | 22/28 [00:01<00:00, 12.53it/s, total_batch_loss=277.67117, average_batch_loss=1.08465, average_batch_perplexity=2.95841, lr=0.001194014, 0.001194014]
Epoch: 2, Step: 24: 86%|████████▌ | 24/28 [00:01<00:00, 12.62it/s, total_batch_loss=277.67117, average_batch_loss=1.08465, average_batch_perplexity=2.95841, lr=0.001194014, 0.001194014]
Epoch: 2, Step: 25: 86%|████████▌ | 24/28 [00:02<00:00, 12.62it/s, total_batch_loss=277.67117, average_batch_loss=1.08465, average_batch_perplexity=2.95841, lr=0.001194014, 0.001194014]
Epoch: 2, Step: 25: 86%|████████▌ | 24/28 [00:02<00:00, 12.62it/s, total_batch_loss=282.78699, average_batch_loss=1.10464, average_batch_perplexity=3.01813, lr=0.001216976, 0.001216976]
Epoch: 2, Step: 26: 86%|████████▌ | 24/28 [00:02<00:00, 12.62it/s, total_batch_loss=282.78699, average_batch_loss=1.10464, average_batch_perplexity=3.01813, lr=0.001216976, 0.001216976]
Epoch: 2, Step: 26: 86%|████████▌ | 24/28 [00:02<00:00, 12.62it/s, total_batch_loss=276.07965, average_batch_loss=1.07844, average_batch_perplexity=2.94008, lr=0.001239938, 0.001239938]
Epoch: 2, Step: 26: 93%|█████████▎| 26/28 [00:02<00:00, 12.59it/s, total_batch_loss=276.07965, average_batch_loss=1.07844, average_batch_perplexity=2.94008, lr=0.001239938, 0.001239938]
Epoch: 2, Step: 27: 93%|█████████▎| 26/28 [00:02<00:00, 12.59it/s, total_batch_loss=276.07965, average_batch_loss=1.07844, average_batch_perplexity=2.94008, lr=0.001239938, 0.001239938]
Epoch: 2, Step: 27: 93%|█████████▎| 26/28 [00:02<00:00, 12.59it/s, total_batch_loss=276.06375, average_batch_loss=1.07837, average_batch_perplexity=2.93990, lr=0.001262899, 0.001262899]
Epoch: 2, Step: 28: 93%|█████████▎| 26/28 [00:02<00:00, 12.59it/s, total_batch_loss=276.06375, average_batch_loss=1.07837, average_batch_perplexity=2.93990, lr=0.001262899, 0.001262899]
Epoch: 2, Step: 28: 93%|█████████▎| 26/28 [00:02<00:00, 12.59it/s, total_batch_loss=92.09214, average_batch_loss=1.04650, average_batch_perplexity=2.84767, lr=0.001285861, 0.001285861]
Epoch: 2, Step: 28: 100%|██████████| 28/28 [00:02<00:00, 13.58it/s, total_batch_loss=92.09214, average_batch_loss=1.04650, average_batch_perplexity=2.84767, lr=0.001285861, 0.001285861]
2024-12-17 09:44:02,712 - root - INFO - Total Samples: 7000
2024-12-17 09:44:02,713 - root - INFO - Total Batches: 28
2024-12-17 09:44:02,713 - root - INFO - Average Epoch Train Loss: 1.26828
2024-12-17 09:44:02,713 - root - INFO - Average Epoch Train Perplexity: 3.55473
2024-12-17 09:44:02,714 - root - INFO -
2024-12-17 09:44:02,714 - root - INFO - ====================================================== Starting Valid Epoch: 2/9 ======================================================
0%| | 0/8 [00:00<?, ?it/s]
Epoch: 2, Step: 1: 0%| | 0/8 [00:00<?, ?it/s]
Epoch: 2, Step: 1: 0%| | 0/8 [00:00<?, ?it/s, total_batch_loss=272.26123, average_batch_loss=1.06352, average_batch_perplexity=2.89655]
Epoch: 2, Step: 2: 0%| | 0/8 [00:00<?, ?it/s, total_batch_loss=272.26123, average_batch_loss=1.06352, average_batch_perplexity=2.89655]
Epoch: 2, Step: 2: 0%| | 0/8 [00:00<?, ?it/s, total_batch_loss=267.66589, average_batch_loss=1.04557, average_batch_perplexity=2.84502]
Epoch: 2, Step: 3: 0%| | 0/8 [00:00<?, ?it/s, total_batch_loss=267.66589, average_batch_loss=1.04557, average_batch_perplexity=2.84502]
Epoch: 2, Step: 3: 0%| | 0/8 [00:00<?, ?it/s, total_batch_loss=267.72772, average_batch_loss=1.04581, average_batch_perplexity=2.84571]
Epoch: 2, Step: 3: 38%|███▊ | 3/8 [00:00<00:00, 23.57it/s, total_batch_loss=267.72772, average_batch_loss=1.04581, average_batch_perplexity=2.84571]
Epoch: 2, Step: 4: 38%|███▊ | 3/8 [00:00<00:00, 23.57it/s, total_batch_loss=267.72772, average_batch_loss=1.04581, average_batch_perplexity=2.84571]
Epoch: 2, Step: 4: 38%|███▊ | 3/8 [00:00<00:00, 23.57it/s, total_batch_loss=270.36462, average_batch_loss=1.05611, average_batch_perplexity=2.87517]
Epoch: 2, Step: 5: 38%|███▊ | 3/8 [00:00<00:00, 23.57it/s, total_batch_loss=270.36462, average_batch_loss=1.05611, average_batch_perplexity=2.87517]
Epoch: 2, Step: 5: 38%|███▊ | 3/8 [00:00<00:00, 23.57it/s, total_batch_loss=274.43536, average_batch_loss=1.07201, average_batch_perplexity=2.92125]
Epoch: 2, Step: 6: 38%|███▊ | 3/8 [00:00<00:00, 23.57it/s, total_batch_loss=274.43536, average_batch_loss=1.07201, average_batch_perplexity=2.92125]
Epoch: 2, Step: 6: 38%|███▊ | 3/8 [00:00<00:00, 23.57it/s, total_batch_loss=267.62402, average_batch_loss=1.04541, average_batch_perplexity=2.84455]
Epoch: 2, Step: 6: 75%|███████▌ | 6/8 [00:00<00:00, 23.80it/s, total_batch_loss=267.62402, average_batch_loss=1.04541, average_batch_perplexity=2.84455]
Epoch: 2, Step: 7: 75%|███████▌ | 6/8 [00:00<00:00, 23.80it/s, total_batch_loss=267.62402, average_batch_loss=1.04541, average_batch_perplexity=2.84455]
Epoch: 2, Step: 7: 75%|███████▌ | 6/8 [00:00<00:00, 23.80it/s, total_batch_loss=267.19635, average_batch_loss=1.04374, average_batch_perplexity=2.83981]
Epoch: 2, Step: 8: 75%|███████▌ | 6/8 [00:00<00:00, 23.80it/s, total_batch_loss=267.19635, average_batch_loss=1.04374, average_batch_perplexity=2.83981]
Epoch: 2, Step: 8: 75%|███████▌ | 6/8 [00:00<00:00, 23.80it/s, total_batch_loss=218.63084, average_batch_loss=1.05111, average_batch_perplexity=2.86082]
2024-12-17 09:44:03,048 - root - INFO - Total Samples: 2000
2024-12-17 09:44:03,049 - root - INFO - Total Batches: 8
2024-12-17 09:44:03,049 - root - INFO - Average Epoch Valid Loss: 1.05295
2024-12-17 09:44:03,050 - root - INFO - Average Epoch Valid Perplexity: 2.86610
2024-12-17 09:44:03,051 - root - INFO -
Evaluating and Generation.: 0%| | 0/4 [00:00<?, ?it/s]
Evaluating and Generation.: 0%| | 0/4 [00:00<?, ?it/s, accuracy: 0.0273]
Evaluating and Generation.: 0%| | 0/4 [00:00<?, ?it/s, accuracy: 0.0469]
Evaluating and Generation.: 50%|█████ | 2/4 [00:00<00:00, 10.59it/s, accuracy: 0.0469]
Evaluating and Generation.: 50%|█████ | 2/4 [00:00<00:00, 10.59it/s, accuracy: 0.0508]
Evaluating and Generation.: 50%|█████ | 2/4 [00:00<00:00, 10.59it/s, accuracy: 0.0431]
Evaluating and Generation.: 100%|██████████| 4/4 [00:00<00:00, 10.88it/s, accuracy: 0.0431]
2024-12-17 09:44:03,425 - root - INFO - Correct/Total Samples: 42/1000
2024-12-17 09:44:03,425 - root - INFO - Eval Accuracy: 0.042
2024-12-17 09:44:03,443 - root - INFO -
epoch batch_index sample_index equation generated correct
2 1 0 13+48=061 13+48=055 False
2 1 1 16+55=071 16+55=075 False
2 1 2 79+34=113 79+34=111 False
2 1 3 35+44=079 35+44=085 False
2 1 4 16+50=066 16+50=055 False
2 1 5 28+47=075 28+47=085 False
2 1 6 00+74=074 00+74=085 False
2 1 7 15+20=035 15+20=031 False
2 1 8 72+60=132 72+60=135 False
2 1 9 63+68=131 63+68=125 False
2 1 10 29+45=074 29+45=085 False
2 1 11 34+60=094 34+60=111 False
2 1 12 53+70=123 53+70=125 False
2 1 13 70+50=120 70+50=125 False
2 1 14 11+84=095 11+84=091 False
2 1 15 42+71=113 42+71=111 False
2 1 16 98+22=120 98+22=111 False
2 1 17 02+02=004 02+02=031 False
2 1 18 15+85=100 15+85=107 False
2 1 19 21+78=099 21+78=107 False
2 1 20 61+79=140 61+79=135 False
2 1 21 25+99=124 25+99=111 False
2 1 22 09+85=094 09+85=095 False
2 1 23 60+91=151 60+91=135 False
2 1 24 35+30=065 35+30=075 False
2 1 25 24+51=075 24+51=085 False
2 1 26 93+91=184 93+91=151 False
2 1 27 39+96=135 39+96=135 True
2 1 28 64+35=099 64+35=111 False
2 1 29 36+22=058 36+22=075 False
2 1 30 68+45=113 68+45=111 False
2 1 31 16+84=100 16+84=107 False
2 1 32 91+52=143 91+52=131 False
2 1 33 97+36=133 97+36=135 False
2 1 34 27+37=064 27+37=085 False
2 1 35 99+82=181 99+82=175 False
2 1 36 03+42=045 03+42=051 False
2 1 37 18+38=056 18+38=075 False
2 1 38 32+20=052 32+20=055 False
2 1 39 38+13=051 38+13=055 False
2 1 40 68+42=110 68+42=111 False
2 1 41 64+00=064 64+00=085 False
2 1 42 48+94=142 48+94=135 False
2 1 43 58+36=094 58+36=111 False
2 1 44 41+22=063 41+22=075 False
2 1 45 23+58=081 23+58=085 False
2 1 46 67+46=113 67+46=111 False
2 1 47 40+78=118 40+78=125 False
2 1 48 90+38=128 90+38=135 False
2 1 49 89+52=141 89+52=135 False
2 1 50 37+77=114 37+77=111 False
2 1 51 29+76=105 29+76=107 False
2 1 52 42+90=132 42+90=135 False
2 1 53 45+82=127 45+82=135 False
2 1 54 35+95=130 35+95=135 False
2 1 55 92+98=190 92+98=177 False
2 1 56 73+91=164 73+91=151 False
2 1 57 53+97=150 53+97=155 False
2 1 58 98+69=167 98+69=155 False
2 1 59 20+46=066 20+46=075 False
2 1 60 48+69=117 48+69=111 False
2 1 61 62+31=093 62+31=111 False
2 1 62 80+59=139 80+59=135 False
2 1 63 58+12=070 58+12=075 False
2 1 64 08+96=104 08+96=095 False
2 1 65 67+06=073 67+06=085 False
2 1 66 22+04=026 22+04=035 False
2 1 67 61+87=148 61+87=135 False
2 1 68 95+27=122 95+27=111 False
2 1 69 49+83=132 49+83=135 False
2 1 70 43+00=043 43+00=055 False
2 1 71 01+85=086 01+85=095 False
2 1 72 11+68=079 11+68=081 False
2 1 73 80+03=083 80+03=095 False
2 1 74 54+83=137 54+83=135 False
2 1 75 73+47=120 73+47=125 False
2 1 76 99+93=192 99+93=155 False
2 1 77 99+13=112 99+13=111 False
2 1 78 92+66=158 92+66=155 False
2 1 79 90+31=121 90+31=131 False
2 1 80 25+69=094 25+69=095 False
2 1 81 25+44=069 25+44=085 False
2 1 82 00+93=093 00+93=085 False
2 1 83 88+87=175 88+87=155 False
2 1 84 47+56=103 47+56=111 False
2 1 85 43+59=102 43+59=111 False
2 1 86 22+00=022 22+00=033 False
2 1 87 34+04=038 34+04=055 False
2 1 88 65+13=078 65+13=081 False
2 1 89 39+82=121 39+82=125 False
2 1 90 66+83=149 66+83=135 False
2 1 91 51+69=120 51+69=111 False
2 1 92 80+21=101 80+21=111 False
2 1 93 36+79=115 36+79=111 False
2 1 94 21+68=089 21+68=095 False
2 1 95 11+66=077 11+66=081 False
2 1 96 55+19=074 55+19=075 False
2 1 97 51+61=112 51+61=111 False
2 1 98 38+88=126 38+88=125 False
2 1 99 37+27=064 37+27=085 False
2 1 100 18+63=081 18+63=081 True
2 1 101 48+11=059 48+11=051 False
2 1 102 72+68=140 72+68=135 False
2 1 103 37+39=076 37+39=085 False
2 1 104 64+95=159 64+95=155 False
2 1 105 49+75=124 49+75=125 False
2 1 106 45+66=111 45+66=111 True
2 1 107 34+87=121 34+87=125 False
2 1 108 02+84=086 02+84=095 False
2 1 109 95+00=095 95+00=095 True
2 1 110 09+56=065 09+56=075 False
2 1 111 22+66=088 22+66=095 False
2 1 112 43+18=061 43+18=055 False
2 1 113 61+35=096 61+35=111 False
2 1 114 13+73=086 13+73=091 False
2 1 115 25+95=120 25+95=111 False
2 1 116 73+96=169 73+96=155 False
2 1 117 03+96=099 03+96=095 False
2 1 118 97+82=179 97+82=175 False
2 1 119 18+42=060 18+42=055 False
2 1 120 29+98=127 29+98=111 False
2 1 121 61+00=061 61+00=085 False
2 1 122 22+98=120 22+98=111 False
2 1 123 12+50=062 12+50=051 False
2 1 124 02+58=060 02+58=075 False
2 1 125 75+86=161 75+86=155 False
2 1 126 31+57=088 31+57=085 False
2 1 127 49+82=131 49+82=135 False
2 1 128 15+33=048 15+33=051 False
2 1 129 49+57=106 49+57=111 False
2 1 130 61+70=131 61+70=135 False
2 1 131 91+51=142 91+51=131 False
2 1 132 50+05=055 50+05=055 True
2 1 133 44+16=060 44+16=055 False
2 1 134 92+01=093 92+01=095 False
2 1 135 85+82=167 85+82=155 False
2 1 136 07+41=048 07+41=055 False
2 1 137 40+06=046 40+06=055 False
2 1 138 79+62=141 79+62=135 False
2 1 139 95+62=157 95+62=155 False
2 1 140 42+93=135 42+93=135 True
2 1 141 32+73=105 32+73=111 False
2 1 142 47+09=056 47+09=075 False
2 1 143 59+50=109 59+50=111 False
2 1 144 61+77=138 61+77=135 False
2 1 145 64+06=070 64+06=085 False
2 1 146 35+10=045 35+10=031 False
2 1 147 32+88=120 32+88=125 False
2 1 148 03+95=098 03+95=085 False
2 1 149 11+38=049 11+38=053 False
2 1 150 21+67=088 21+67=095 False
2 1 151 33+25=058 33+25=075 False
2 1 152 63+45=108 63+45=111 False
2 1 153 56+12=068 56+12=055 False
2 1 154 19+79=098 19+79=095 False
2 1 155 60+43=103 60+43=111 False
2 1 156 07+61=068 07+61=085 False
2 1 157 58+03=061 58+03=075 False
2 1 158 11+10=021 11+10=031 False
2 1 159 49+89=138 49+89=135 False
2 1 160 37+58=095 37+58=111 False
2 1 161 59+78=137 59+78=135 False
2 1 162 11+21=032 11+21=031 False
2 1 163 37+43=080 37+43=085 False
2 1 164 44+21=065 44+21=075 False
2 1 165 22+97=119 22+97=111 False
2 1 166 65+35=100 65+35=111 False
2 1 167 06+51=057 06+51=055 False
2 1 168 65+25=090 65+25=095 False
2 1 169 74+94=168 74+94=155 False
2 1 170 87+55=142 87+55=135 False
2 1 171 90+67=157 90+67=155 False
2 1 172 11+02=013 11+02=031 False
2 1 173 01+66=067 01+66=085 False
2 1 174 56+00=056 56+00=055 False
2 1 175 58+52=110 58+52=111 False
2 1 176 24+99=123 24+99=111 False
2 1 177 97+13=110 97+13=111 False
2 1 178 42+94=136 42+94=135 False
2 1 179 60+15=075 60+15=081 False
2 1 180 20+46=066 20+46=075 False
2 1 181 40+70=110 40+70=111 False
2 1 182 95+45=140 95+45=135 False
2 1 183 96+95=191 96+95=155 False
2 1 184 98+20=118 98+20=111 False
2 1 185 43+19=062 43+19=055 False
2 1 186 50+69=119 50+69=111 False
2 1 187 27+53=080 27+53=085 False
2 1 188 24+25=049 24+25=075 False
2 1 189 65+92=157 65+92=155 False
2 1 190 28+14=042 28+14=055 False
2 1 191 20+57=077 20+57=085 False
2 1 192 59+97=156 59+97=155 False
2 1 193 98+32=130 98+32=135 False
2 1 194 55+84=139 55+84=135 False
2 1 195 20+39=059 20+39=075 False
2 1 196 86+47=133 86+47=135 False
2 1 197 92+36=128 92+36=135 False
2 1 198 05+38=043 05+38=055 False
2 1 199 77+36=113 77+36=111 False
2 1 200 41+64=105 41+64=111 False
2 1 201 74+51=125 74+51=125 True
2 1 202 74+55=129 74+55=135 False
2 1 203 64+64=128 64+64=125 False
2 1 204 60+19=079 60+19=081 False
2 1 205 77+96=173 77+96=155 False
2 1 206 22+30=052 22+30=055 False
2 1 207 82+49=131 82+49=135 False
2 1 208 39+67=106 39+67=111 False
2 1 209 62+40=102 62+40=111 False
2 1 210 28+71=099 28+71=107 False
2 1 211 47+26=073 47+26=085 False
2 1 212 98+54=152 98+54=155 False
2 1 213 38+70=108 38+70=111 False
2 1 214 63+40=103 63+40=111 False
2 1 215 86+62=148 86+62=135 False
2 1 216 22+65=087 22+65=095 False
2 1 217 41+17=058 41+17=053 False
2 1 218 68+88=156 68+88=135 False
2 1 219 96+70=166 96+70=155 False
2 1 220 99+29=128 99+29=111 False
2 1 221 83+39=122 83+39=125 False
2 1 222 26+55=081 26+55=085 False
2 1 223 53+70=123 53+70=125 False
2 1 224 94+12=106 94+12=111 False
2 1 225 00+37=037 00+37=055 False
2 1 226 36+94=130 36+94=135 False
2 1 227 40+58=098 40+58=111 False
2 1 228 19+80=099 19+80=107 False
2 1 229 49+44=093 49+44=111 False
2 1 230 70+27=097 70+27=107 False
2 1 231 52+80=132 52+80=135 False
2 1 232 77+90=167 77+90=155 False
2 1 233 13+92=105 13+92=111 False
2 1 234 59+09=068 59+09=085 False
2 1 235 33+55=088 33+55=085 False
2 1 236 85+16=101 85+16=107 False
2 1 237 25+65=090 25+65=095 False
2 1 238 46+20=066 46+20=075 False
2 1 239 29+52=081 29+52=085 False
2 1 240 32+36=068 32+36=085 False
2 1 241 47+08=055 47+08=075 False
2 1 242 21+84=105 21+84=111 False
2 1 243 24+45=069 24+45=085 False
2 1 244 29+15=044 29+15=055 False
2 1 245 83+03=086 83+03=095 False
2 1 246 83+36=119 83+36=125 False
2 1 247 58+95=153 58+95=155 False
2 1 248 76+79=155 76+79=135 False
2 1 249 63+30=093 63+30=111 False
2 1 250 38+24=062 38+24=085 False
2 1 251 19+46=065 19+46=075 False
2 1 252 99+66=165 99+66=155 False
2 1 253 95+73=168 95+73=155 False
2 1 254 65+27=092 65+27=095 False
2 1 255 91+83=174 91+83=151 False
2 2 0 65+49=114 65+49=111 False
2 2 1 03+08=011 03+08=035 False
2 2 2 67+81=148 67+81=135 False
2 2 3 47+23=070 47+23=085 False
2 2 4 43+91=134 43+91=131 False
2 2 5 41+67=108 41+67=111 False
2 2 6 02+33=035 02+33=051 False
2 2 7 64+84=148 64+84=135 False
2 2 8 81+64=145 81+64=135 False
2 2 9 80+11=091 80+11=091 True
2 2 10 78+01=079 78+01=095 False
2 2 11 89+18=107 89+18=107 True
2 2 12 45+52=097 45+52=111 False
2 2 13 35+30=065 35+30=075 False
2 2 14 53+32=085 53+32=085 True
2 2 15 49+90=139 49+90=135 False
2 2 16 41+37=078 41+37=085 False
2 2 17 35+14=049 35+14=051 False
2 2 18 92+50=142 92+50=131 False
2 2 19 37+60=097 37+60=111 False
2 2 20 91+61=152 91+61=131 False
2 2 21 80+77=157 80+77=135 False
2 2 22 66+24=090 66+24=095 False
2 2 23 81+07=088 81+07=095 False
2 2 24 85+59=144 85+59=135 False
2 2 25 19+69=088 19+69=095 False
2 2 26 91+44=135 91+44=131 False
2 2 27 25+29=054 25+29=075 False
2 2 28 27+08=035 27+08=055 False
2 2 29 66+14=080 66+14=081 False
2 2 30 95+11=106 95+11=111 False
2 2 31 13+97=110 13+97=111 False
2 2 32 94+40=134 94+40=131 False
2 2 33 74+31=105 74+31=111 False
2 2 34 49+00=049 49+00=055 False
2 2 35 59+18=077 59+18=081 False
2 2 36 07+65=072 07+65=085 False
2 2 37 83+55=138 83+55=135 False
2 2 38 49+80=129 49+80=135 False
2 2 39 64+17=081 64+17=081 True
2 2 40 48+83=131 48+83=135 False
2 2 41 95+44=139 95+44=135 False
2 2 42 71+26=097 71+26=107 False
2 2 43 06+74=080 06+74=085 False
2 2 44 34+24=058 34+24=075 False
2 2 45 59+71=130 59+71=125 False
2 2 46 68+32=100 68+32=111 False
2 2 47 38+81=119 38+81=125 False
2 2 48 29+56=085 29+56=085 True
2 2 49 54+55=109 54+55=111 False
2 2 50 31+27=058 31+27=085 False
2 2 51 97+89=186 97+89=177 False
2 2 52 48+09=057 48+09=075 False
2 2 53 86+76=162 86+76=155 False
2 2 54 82+59=141 82+59=135 False
2 2 55 01+67=068 01+67=085 False
2 2 56 26+06=032 26+06=055 False
2 2 57 22+46=068 22+46=085 False
2 2 58 85+16=101 85+16=107 False
2 2 59 29+08=037 29+08=055 False
2 2 60 73+94=167 73+94=155 False
2 2 61 19+62=081 19+62=081 True
2 2 62 86+62=148 86+62=135 False
2 2 63 38+99=137 38+99=135 False
2 2 64 64+25=089 64+25=095 False
2 2 65 61+72=133 61+72=135 False
2 2 66 78+88=166 78+88=155 False
2 2 67 43+66=109 43+66=111 False
2 2 68 69+35=104 69+35=111 False
2 2 69 33+77=110 33+77=111 False
2 2 70 37+37=074 37+37=085 False
2 2 71 87+54=141 87+54=135 False
2 2 72 68+90=158 68+90=155 False
2 2 73 83+44=127 83+44=135 False
2 2 74 41+09=050 41+09=055 False
2 2 75 13+48=061 13+48=055 False
2 2 76 01+41=042 01+41=031 False
2 2 77 19+74=093 19+74=095 False
2 2 78 15+05=020 15+05=031 False
2 2 79 55+46=101 55+46=111 False
2 2 80 68+33=101 68+33=111 False
2 2 81 44+40=084 44+40=111 False
2 2 82 88+03=091 88+03=095 False
2 2 83 81+79=160 81+79=135 False
2 2 84 18+98=116 18+98=111 False
2 2 85 70+64=134 70+64=135 False
2 2 86 26+44=070 26+44=085 False
2 2 87 98+87=185 98+87=177 False
2 2 88 18+74=092 18+74=095 False
2 2 89 50+68=118 50+68=111 False
2 2 90 13+51=064 13+51=051 False
2 2 91 90+89=179 90+89=155 False
2 2 92 47+78=125 47+78=125 True
2 2 93 81+57=138 81+57=135 False
2 2 94 34+47=081 34+47=085 False
2 2 95 94+23=117 94+23=111 False
2 2 96 07+70=077 07+70=085 False
2 2 97 56+33=089 56+33=111 False
2 2 98 33+04=037 33+04=055 False
2 2 99 26+09=035 26+09=055 False
2 2 100 14+92=106 14+92=111 False
2 2 101 78+54=132 78+54=135 False
2 2 102 36+76=112 36+76=111 False
2 2 103 17+47=064 17+47=075 False
2 2 104 28+18=046 28+18=055 False
2 2 105 78+54=132 78+54=135 False
2 2 106 84+72=156 84+72=135 False
2 2 107 00+44=044 00+44=055 False
2 2 108 50+41=091 50+41=111 False
2 2 109 87+88=175 87+88=155 False
2 2 110 11+66=077 11+66=081 False
2 2 111 80+60=140 80+60=135 False
2 2 112 78+76=154 78+76=135 False
2 2 113 24+74=098 24+74=107 False
2 2 114 88+48=136 88+48=135 False
2 2 115 38+31=069 38+31=085 False
2 2 116 29+27=056 29+27=085 False
2 2 117 08+45=053 08+45=055 False
2 2 118 28+13=041 28+13=055 False
2 2 119 53+99=152 53+99=155 False
2 2 120 47+92=139 47+92=135 False
2 2 121 76+21=097 76+21=107 False
2 2 122 53+96=149 53+96=155 False
2 2 123 93+91=184 93+91=151 False
2 2 124 97+33=130 97+33=135 False
2 2 125 67+78=145 67+78=135 False
2 2 126 58+05=063 58+05=075 False
2 2 127 00+16=016 00+16=033 False
2 2 128 80+19=099 80+19=107 False
2 2 129 98+22=120 98+22=111 False
2 2 130 09+62=071 09+62=085 False
2 2 131 06+23=029 06+23=055 False
2 2 132 32+99=131 32+99=135 False
2 2 133 17+02=019 17+02=033 False
2 2 134 64+35=099 64+35=111 False
2 2 135 35+83=118 35+83=125 False
2 2 136 71+36=107 71+36=111 False
2 2 137 75+06=081 75+06=095 False
2 2 138 88+95=183 88+95=177 False
2 2 139 19+98=117 19+98=111 False
2 2 140 28+89=117 28+89=111 False
2 2 141 33+11=044 33+11=031 False
2 2 142 34+49=083 34+49=085 False
2 2 143 90+35=125 90+35=135 False
2 2 144 22+90=112 22+90=111 False
2 2 145 98+89=187 98+89=177 False
2 2 146 88+47=135 88+47=135 True
2 2 147 30+86=116 30+86=125 False
2 2 148 31+48=079 31+48=085 False
2 2 149 39+21=060 39+21=075 False
2 2 150 19+17=036 19+17=035 False
2 2 151 27+60=087 27+60=095 False
2 2 152 12+16=028 12+16=031 False
2 2 153 51+75=126 51+75=125 False
2 2 154 10+74=084 10+74=091 False
2 2 155 42+63=105 42+63=111 False
2 2 156 40+14=054 40+14=031 False
2 2 157 23+93=116 23+93=111 False
2 2 158 85+26=111 85+26=111 True
2 2 159 28+46=074 28+46=085 False
2 2 160 28+33=061 28+33=085 False
2 2 161 43+30=073 43+30=085 False
2 2 162 89+72=161 89+72=145 False
2 2 163 52+21=073 52+21=085 False
2 2 164 21+54=075 21+54=085 False
2 2 165 69+13=082 69+13=081 False
2 2 166 07+60=067 07+60=085 False
2 2 167 63+83=146 63+83=135 False
2 2 168 80+69=149 80+69=135 False
2 2 169 27+28=055 27+28=085 False
2 2 170 42+31=073 42+31=085 False
2 2 171 51+99=150 51+99=135 False
2 2 172 28+75=103 28+75=107 False
2 2 173 38+57=095 38+57=111 False
2 2 174 83+16=099 83+16=107 False
2 2 175 92+94=186 92+94=151 False
2 2 176 55+75=130 55+75=135 False
2 2 177 59+51=110 59+51=111 False
2 2 178 33+09=042 33+09=055 False
2 2 179 53+13=066 53+13=051 False
2 2 180 05+70=075 05+70=085 False
2 2 181 12+20=032 12+20=031 False
2 2 182 11+49=060 11+49=053 False
2 2 183 63+45=108 63+45=111 False
2 2 184 92+23=115 92+23=111 False
2 2 185 82+45=127 82+45=135 False
2 2 186 23+41=064 23+41=075 False
2 2 187 64+26=090 64+26=095 False
2 2 188 91+24=115 91+24=111 False
2 2 189 20+32=052 20+32=055 False
2 2 190 83+21=104 83+21=111 False
2 2 191 07+20=027 07+20=055 False
2 2 192 94+14=108 94+14=111 False
2 2 193 96+89=185 96+89=177 False
2 2 194 13+08=021 13+08=035 False
2 2 195 32+05=037 32+05=055 False
2 2 196 09+51=060 09+51=055 False
2 2 197 26+29=055 26+29=075 False
2 2 198 49+65=114 49+65=111 False
2 2 199 32+66=098 32+66=111 False
2 2 200 41+08=049 41+08=055 False
2 2 201 26+79=105 26+79=107 False
2 2 202 29+91=120 29+91=111 False
2 2 203 51+00=051 51+00=051 True
2 2 204 61+60=121 61+60=125 False
2 2 205 45+78=123 45+78=125 False
2 2 206 56+16=072 56+16=075 False
2 2 207 66+68=134 66+68=125 False
2 2 208 32+16=048 32+16=053 False
2 2 209 84+49=133 84+49=135 False
2 2 210 45+09=054 45+09=075 False
2 2 211 96+78=174 96+78=155 False
2 2 212 10+02=012 10+02=031 False
2 2 213 36+60=096 36+60=111 False
2 2 214 44+36=080 44+36=085 False
2 2 215 12+86=098 12+86=095 False
2 2 216 94+54=148 94+54=135 False
2 2 217 64+73=137 64+73=135 False
2 2 218 73+10=083 73+10=091 False
2 2 219 14+62=076 14+62=081 False
2 2 220 25+22=047 25+22=055 False
2 2 221 94+22=116 94+22=111 False
2 2 222 41+76=117 41+76=111 False
2 2 223 38+46=084 38+46=085 False
2 2 224 71+72=143 71+72=135 False
2 2 225 74+79=153 74+79=135 False
2 2 226 99+67=166 99+67=155 False
2 2 227 78+71=149 78+71=135 False
2 2 228 23+19=042 23+19=055 False
2 2 229 51+65=116 51+65=111 False
2 2 230 94+86=180 94+86=177 False
2 2 231 09+79=088 09+79=095 False
2 2 232 69+39=108 69+39=111 False
2 2 233 84+13=097 84+13=107 False
2 2 234 36+59=095 36+59=111 False
2 2 235 87+47=134 87+47=135 False
2 2 236 50+00=050 50+00=055 False
2 2 237 76+96=172 76+96=155 False
2 2 238 12+18=030 12+18=031 False
2 2 239 99+95=194 99+95=177 False
2 2 240 22+00=022 22+00=033 False
2 2 241 96+18=114 96+18=111 False
2 2 242 51+20=071 51+20=075 False
2 2 243 66+81=147 66+81=135 False
2 2 244 78+18=096 78+18=095 False
2 2 245 09+78=087 09+78=095 False
2 2 246 24+20=044 24+20=055 False
2 2 247 76+13=089 76+13=091 False
2 2 248 05+10=015 05+10=031 False
2 2 249 97+14=111 97+14=111 True
2 2 250 92+38=130 92+38=135 False
2 2 251 77+13=090 77+13=091 False
2 2 252 70+19=089 70+19=095 False
2 2 253 90+45=135 90+45=135 True
2 2 254 50+09=059 50+09=075 False
2 2 255 78+06=084 78+06=095 False
2 3 0 03+25=028 03+25=055 False
2 3 1 48+43=091 48+43=111 False
2 3 2 39+47=086 39+47=085 False
2 3 3 48+19=067 48+19=075 False
2 3 4 07+22=029 07+22=055 False
2 3 5 73+68=141 73+68=135 False
2 3 6 14+56=070 14+56=075 False
2 3 7 96+95=191 96+95=155 False
2 3 8 96+28=124 96+28=111 False
2 3 9 82+05=087 82+05=095 False
2 3 10 27+94=121 27+94=111 False
2 3 11 87+86=173 87+86=155 False
2 3 12 00+68=068 00+68=085 False
2 3 13 11+37=048 11+37=055 False
2 3 14 95+93=188 95+93=155 False
2 3 15 75+82=157 75+82=135 False
2 3 16 41+71=112 41+71=111 False
2 3 17 60+14=074 60+14=081 False
2 3 18 77+77=154 77+77=135 False
2 3 19 31+84=115 31+84=111 False
2 3 20 31+57=088 31+57=085 False
2 3 21 27+87=114 27+87=111 False
2 3 22 31+89=120 31+89=125 False
2 3 23 22+18=040 22+18=053 False
2 3 24 38+25=063 38+25=085 False
2 3 25 64+54=118 64+54=111 False
2 3 26 85+60=145 85+60=135 False
2 3 27 14+71=085 14+71=091 False
2 3 28 06+16=022 06+16=035 False
2 3 29 78+61=139 78+61=135 False
2 3 30 65+75=140 65+75=135 False
2 3 31 13+83=096 13+83=091 False
2 3 32 75+49=124 75+49=125 False
2 3 33 05+78=083 05+78=095 False
2 3 34 66+55=121 66+55=111 False
2 3 35 03+05=008 03+05=035 False
2 3 36 69+99=168 69+99=155 False
2 3 37 52+82=134 52+82=135 False
2 3 38 45+97=142 45+97=135 False
2 3 39 66+17=083 66+17=085 False
2 3 40 36+17=053 36+17=055 False
2 3 41 92+74=166 92+74=155 False
2 3 42 48+44=092 48+44=111 False
2 3 43 34+17=051 34+17=055 False
2 3 44 56+11=067 56+11=051 False
2 3 45 77+23=100 77+23=107 False
2 3 46 10+11=021 10+11=031 False
2 3 47 32+65=097 32+65=111 False
2 3 48 53+49=102 53+49=111 False
2 3 49 68+86=154 68+86=135 False
2 3 50 52+94=146 52+94=135 False
2 3 51 97+71=168 97+71=155 False
2 3 52 05+37=042 05+37=055 False
2 3 53 58+75=133 58+75=135 False
2 3 54 06+24=030 06+24=055 False
2 3 55 15+44=059 15+44=051 False
2 3 56 90+49=139 90+49=135 False
2 3 57 50+37=087 50+37=085 False
2 3 58 88+61=149 88+61=135 False
2 3 59 21+57=078 21+57=085 False
2 3 60 24+85=109 24+85=111 False
2 3 61 01+66=067 01+66=085 False
2 3 62 50+46=096 50+46=111 False
2 3 63 76+65=141 76+65=135 False
2 3 64 23+74=097 23+74=107 False
2 3 65 76+16=092 76+16=095 False
2 3 66 06+08=014 06+08=055 False
2 3 67 69+25=094 69+25=095 False
2 3 68 15+23=038 15+23=031 False
2 3 69 41+02=043 41+02=051 False
2 3 70 16+66=082 16+66=085 False
2 3 71 59+94=153 59+94=155 False
2 3 72 32+88=120 32+88=125 False
2 3 73 46+21=067 46+21=075 False
2 3 74 57+28=085 57+28=085 True
2 3 75 00+31=031 00+31=031 True
2 3 76 77+07=084 77+07=095 False
2 3 77 28+70=098 28+70=107 False
2 3 78 05+61=066 05+61=085 False
2 3 79 22+09=031 22+09=055 False
2 3 80 08+94=102 08+94=095 False
2 3 81 40+11=051 40+11=031 False
2 3 82 10+48=058 10+48=055 False
2 3 83 27+56=083 27+56=085 False
2 3 84 42+16=058 42+16=053 False
2 3 85 69+43=112 69+43=111 False
2 3 86 57+69=126 57+69=113 False
2 3 87 18+86=104 18+86=107 False
2 3 88 86+80=166 86+80=155 False
2 3 89 30+85=115 30+85=111 False
2 3 90 77+66=143 77+66=135 False
2 3 91 39+64=103 39+64=111 False
2 3 92 76+61=137 76+61=135 False
2 3 93 42+61=103 42+61=111 False
2 3 94 07+30=037 07+30=055 False
2 3 95 35+93=128 35+93=135 False
2 3 96 40+90=130 40+90=135 False
2 3 97 08+91=099 08+91=085 False
2 3 98 62+34=096 62+34=111 False
2 3 99 86+49=135 86+49=135 True
2 3 100 73+23=096 73+23=107 False
2 3 101 87+35=122 87+35=125 False
2 3 102 35+31=066 35+31=075 False
2 3 103 07+13=020 07+13=031 False
2 3 104 39+41=080 39+41=085 False
2 3 105 44+63=107 44+63=111 False
2 3 106 94+66=160 94+66=155 False
2 3 107 49+54=103 49+54=111 False
2 3 108 79+46=125 79+46=125 True
2 3 109 53+12=065 53+12=051 False
2 3 110 60+92=152 60+92=151 False
2 3 111 25+60=085 25+60=085 True
2 3 112 64+53=117 64+53=111 False
2 3 113 41+02=043 41+02=051 False
2 3 114 00+97=097 00+97=085 False
2 3 115 12+52=064 12+52=051 False
2 3 116 39+50=089 39+50=085 False
2 3 117 87+21=108 87+21=111 False
2 3 118 04+99=103 04+99=095 False
2 3 119 19+75=094 19+75=095 False
2 3 120 90+05=095 90+05=095 True
2 3 121 54+39=093 54+39=111 False
2 3 122 29+26=055 29+26=075 False
2 3 123 82+95=177 82+95=155 False
2 3 124 55+09=064 55+09=075 False
2 3 125 02+62=064 02+62=085 False
2 3 126 68+30=098 68+30=111 False
2 3 127 99+16=115 99+16=111 False
2 3 128 63+11=074 63+11=081 False
2 3 129 42+92=134 42+92=131 False
2 3 130 99+16=115 99+16=111 False
2 3 131 50+31=081 50+31=085 False
2 3 132 23+46=069 23+46=085 False
2 3 133 45+73=118 45+73=125 False
2 3 134 89+77=166 89+77=155 False
2 3 135 45+78=123 45+78=125 False
2 3 136 96+60=156 96+60=155 False
2 3 137 74+61=135 74+61=135 True
2 3 138 87+01=088 87+01=095 False
2 3 139 63+88=151 63+88=135 False
2 3 140 59+72=131 59+72=135 False
2 3 141 17+96=113 17+96=111 False
2 3 142 89+77=166 89+77=155 False
2 3 143 24+69=093 24+69=095 False
2 3 144 75+83=158 75+83=145 False
2 3 145 50+54=104 50+54=111 False
2 3 146 93+47=140 93+47=135 False
2 3 147 20+55=075 20+55=085 False
2 3 148 91+79=170 91+79=155 False
2 3 149 15+13=028 15+13=031 False
2 3 150 86+09=095 86+09=095 True
2 3 151 29+58=087 29+58=085 False
2 3 152 01+29=030 01+29=055 False
2 3 153 65+48=113 65+48=111 False
2 3 154 96+45=141 96+45=135 False
2 3 155 58+69=127 58+69=113 False
2 3 156 84+43=127 84+43=135 False
2 3 157 90+38=128 90+38=135 False
2 3 158 39+97=136 39+97=135 False
2 3 159 74+84=158 74+84=135 False
2 3 160 86+22=108 86+22=111 False
2 3 161 01+86=087 01+86=095 False
2 3 162 81+63=144 81+63=135 False
2 3 163 80+94=174 80+94=155 False
2 3 164 44+42=086 44+42=111 False
2 3 165 72+60=132 72+60=135 False
2 3 166 28+07=035 28+07=055 False
2 3 167 69+54=123 69+54=111 False
2 3 168 68+77=145 68+77=135 False
2 3 169 90+16=106 90+16=111 False
2 3 170 64+50=114 64+50=111 False
2 3 171 46+88=134 46+88=135 False
2 3 172 55+99=154 55+99=155 False
2 3 173 31+97=128 31+97=135 False
2 3 174 79+28=107 79+28=107 True
2 3 175 81+43=124 81+43=131 False
2 3 176 41+15=056 41+15=031 False
2 3 177 38+77=115 38+77=111 False
2 3 178 25+06=031 25+06=055 False
2 3 179 01+93=094 01+93=085 False
2 3 180 97+22=119 97+22=111 False
2 3 181 71+84=155 71+84=135 False
2 3 182 26+36=062 26+36=085 False
2 3 183 60+92=152 60+92=151 False
2 3 184 02+94=096 02+94=085 False
2 3 185 31+58=089 31+58=085 False
2 3 186 70+52=122 70+52=125 False
2 3 187 19+42=061 19+42=055 False
2 3 188 95+73=168 95+73=155 False
2 3 189 21+25=046 21+25=055 False
2 3 190 13+58=071 13+58=075 False
2 3 191 62+28=090 62+28=095 False
2 3 192 38+14=052 38+14=055 False
2 3 193 66+75=141 66+75=135 False
2 3 194 24+59=083 24+59=085 False
2 3 195 97+66=163 97+66=155 False
2 3 196 76+70=146 76+70=135 False
2 3 197 08+40=048 08+40=055 False
2 3 198 84+00=084 84+00=095 False
2 3 199 54+73=127 54+73=125 False
2 3 200 16+88=104 16+88=107 False
2 3 201 99+47=146 99+47=135 False
2 3 202 31+95=126 31+95=135 False
2 3 203 01+79=080 01+79=095 False
2 3 204 03+68=071 03+68=085 False
2 3 205 10+05=015 10+05=031 False
2 3 206 98+90=188 98+90=155 False
2 3 207 58+53=111 58+53=111 True
2 3 208 34+87=121 34+87=125 False
2 3 209 07+31=038 07+31=055 False
2 3 210 59+08=067 59+08=085 False
2 3 211 51+38=089 51+38=111 False
2 3 212 62+62=124 62+62=125 False
2 3 213 80+32=112 80+32=111 False
2 3 214 69+16=085 69+16=085 True
2 3 215 01+17=018 01+17=033 False
2 3 216 74+41=115 74+41=111 False
2 3 217 20+89=109 20+89=111 False
2 3 218 53+50=103 53+50=111 False
2 3 219 82+85=167 82+85=155 False
2 3 220 34+47=081 34+47=085 False
2 3 221 34+45=079 34+45=085 False
2 3 222 77+34=111 77+34=111 True
2 3 223 56+33=089 56+33=111 False
2 3 224 97+56=153 97+56=155 False
2 3 225 29+06=035 29+06=055 False
2 3 226 78+96=174 78+96=155 False
2 3 227 28+65=093 28+65=095 False
2 3 228 61+64=125 61+64=125 True
2 3 229 32+64=096 32+64=111 False
2 3 230 98+32=130 98+32=135 False
2 3 231 25+35=060 25+35=075 False
2 3 232 05+08=013 05+08=055 False
2 3 233 05+26=031 05+26=055 False
2 3 234 84+71=155 84+71=135 False
2 3 235 33+10=043 33+10=031 False
2 3 236 98+35=133 98+35=135 False
2 3 237 68+98=166 68+98=155 False
2 3 238 03+63=066 03+63=085 False
2 3 239 12+96=108 12+96=111 False
2 3 240 02+81=083 02+81=095 False
2 3 241 83+13=096 83+13=107 False
2 3 242 55+92=147 55+92=135 False
2 3 243 96+09=105 96+09=095 False
2 3 244 61+08=069 61+08=085 False
2 3 245 39+75=114 39+75=111 False
2 3 246 40+74=114 40+74=111 False
2 3 247 39+80=119 39+80=125 False
2 3 248 57+95=152 57+95=155 False
2 3 249 92+97=189 92+97=155 False
2 3 250 33+03=036 33+03=055 False
2 3 251 74+92=166 74+92=155 False
2 3 252 99+09=108 99+09=095 False
2 3 253 98+10=108 98+10=111 False
2 3 254 46+77=123 46+77=125 False
2 3 255 85+78=163 85+78=155 False
2 4 0 41+21=062 41+21=051 False
2 4 1 49+13=062 49+13=055 False
2 4 2 59+07=066 59+07=085 False
2 4 3 31+11=042 31+11=031 False
2 4 4 74+16=090 74+16=091 False
2 4 5 43+38=081 43+38=085 False
2 4 6 08+67=075 08+67=085 False
2 4 7 31+66=097 31+66=111 False
2 4 8 10+31=041 10+31=031 False
2 4 9 34+59=093 34+59=111 False
2 4 10 78+42=120 78+42=125 False
2 4 11 13+41=054 13+41=031 False
2 4 12 97+89=186 97+89=177 False
2 4 13 15+62=077 15+62=081 False
2 4 14 39+36=075 39+36=085 False
2 4 15 21+25=046 21+25=055 False
2 4 16 74+56=130 74+56=135 False
2 4 17 85+47=132 85+47=135 False
2 4 18 47+32=079 47+32=085 False
2 4 19 37+66=103 37+66=111 False
2 4 20 16+29=045 16+29=055 False
2 4 21 86+77=163 86+77=155 False
2 4 22 80+07=087 80+07=095 False
2 4 23 87+05=092 87+05=095 False
2 4 24 58+16=074 58+16=081 False
2 4 25 52+79=131 52+79=135 False
2 4 26 91+08=099 91+08=095 False
2 4 27 47+78=125 47+78=125 True
2 4 28 86+96=182 86+96=177 False
2 4 29 90+22=112 90+22=111 False
2 4 30 31+18=049 31+18=053 False
2 4 31 86+15=101 86+15=107 False
2 4 32 15+95=110 15+95=111 False
2 4 33 42+11=053 42+11=031 False
2 4 34 65+99=164 65+99=155 False
2 4 35 89+29=118 89+29=111 False
2 4 36 35+11=046 35+11=031 False
2 4 37 71+41=112 71+41=111 False
2 4 38 16+24=040 16+24=055 False
2 4 39 77+82=159 77+82=145 False
2 4 40 55+89=144 55+89=135 False
2 4 41 17+88=105 17+88=107 False
2 4 42 54+72=126 54+72=125 False
2 4 43 34+98=132 34+98=135 False
2 4 44 09+97=106 09+97=095 False
2 4 45 91+07=098 91+07=095 False
2 4 46 55+94=149 55+94=155 False
2 4 47 22+58=080 22+58=085 False
2 4 48 91+37=128 91+37=135 False
2 4 49 16+10=026 16+10=031 False
2 4 50 96+32=128 96+32=135 False
2 4 51 35+75=110 35+75=111 False
2 4 52 88+73=161 88+73=145 False
2 4 53 35+18=053 35+18=055 False
2 4 54 33+10=043 33+10=031 False
2 4 55 08+50=058 08+50=055 False
2 4 56 22+62=084 22+62=095 False
2 4 57 26+37=063 26+37=085 False
2 4 58 80+27=107 80+27=111 False
2 4 59 68+28=096 68+28=095 False
2 4 60 48+03=051 48+03=055 False
2 4 61 40+18=058 40+18=055 False
2 4 62 16+59=075 16+59=081 False
2 4 63 02+19=021 02+19=033 False
2 4 64 01+09=010 01+09=035 False
2 4 65 62+68=130 62+68=125 False
2 4 66 09+71=080 09+71=085 False
2 4 67 00+58=058 00+58=055 False
2 4 68 16+45=061 16+45=055 False
2 4 69 24+98=122 24+98=111 False
2 4 70 47+92=139 47+92=135 False
2 4 71 94+84=178 94+84=155 False
2 4 72 21+32=053 21+32=053 True
2 4 73 29+82=111 29+82=111 True
2 4 74 32+79=111 32+79=111 True
2 4 75 13+98=111 13+98=111 True
2 4 76 41+94=135 41+94=131 False
2 4 77 51+84=135 51+84=135 True
2 4 78 42+05=047 42+05=055 False
2 4 79 39+03=042 39+03=055 False
2 4 80 02+92=094 02+92=085 False
2 4 81 99+81=180 99+81=155 False
2 4 82 32+68=100 32+68=111 False
2 4 83 52+17=069 52+17=075 False
2 4 84 56+58=114 56+58=111 False
2 4 85 21+48=069 21+48=085 False
2 4 86 61+71=132 61+71=135 False
2 4 87 17+01=018 17+01=033 False
2 4 88 68+23=091 68+23=095 False
2 4 89 00+37=037 00+37=055 False
2 4 90 94+88=182 94+88=177 False
2 4 91 06+31=037 06+31=055 False
2 4 92 27+18=045 27+18=055 False
2 4 93 41+81=122 41+81=131 False
2 4 94 15+86=101 15+86=107 False
2 4 95 36+87=123 36+87=125 False
2 4 96 17+37=054 17+37=075 False
2 4 97 13+86=099 13+86=095 False
2 4 98 29+69=098 29+69=095 False
2 4 99 31+99=130 31+99=135 False
2 4 100 47+29=076 47+29=085 False
2 4 101 08+81=089 08+81=095 False
2 4 102 72+82=154 72+82=135 False
2 4 103 46+91=137 46+91=135 False
2 4 104 70+35=105 70+35=111 False
2 4 105 90+55=145 90+55=135 False
2 4 106 99+99=198 99+99=177 False
2 4 107 60+97=157 60+97=155 False
2 4 108 03+40=043 03+40=051 False
2 4 109 35+49=084 35+49=085 False
2 4 110 32+02=034 32+02=051 False
2 4 111 70+18=088 70+18=091 False
2 4 112 99+05=104 99+05=095 False
2 4 113 78+73=151 78+73=135 False
2 4 114 03+02=005 03+02=033 False
2 4 115 50+14=064 50+14=051 False
2 4 116 62+02=064 62+02=085 False
2 4 117 16+74=090 16+74=095 False
2 4 118 68+65=133 68+65=125 False
2 4 119 74+81=155 74+81=135 False
2 4 120 37+48=085 37+48=085 True
2 4 121 63+04=067 63+04=085 False
2 4 122 06+62=068 06+62=085 False
2 4 123 95+75=170 95+75=155 False
2 4 124 92+37=129 92+37=135 False
2 4 125 81+32=113 81+32=111 False
2 4 126 53+28=081 53+28=085 False
2 4 127 52+42=094 52+42=111 False
2 4 128 66+97=163 66+97=155 False
2 4 129 00+48=048 00+48=055 False
2 4 130 65+32=097 65+32=111 False
2 4 131 60+89=149 60+89=135 False
2 4 132 71+61=132 71+61=135 False
2 4 133 98+50=148 98+50=135 False
2 4 134 90+96=186 90+96=155 False
2 4 135 02+96=098 02+96=095 False
2 4 136 62+75=137 62+75=135 False
2 4 137 41+28=069 41+28=085 False
2 4 138 95+79=174 95+79=155 False
2 4 139 48+41=089 48+41=111 False
2 4 140 87+95=182 87+95=177 False
2 4 141 75+38=113 75+38=111 False
2 4 142 31+55=086 31+55=085 False
2 4 143 54+63=117 54+63=111 False
2 4 144 75+82=157 75+82=135 False
2 4 145 46+45=091 46+45=111 False
2 4 146 13+08=021 13+08=035 False
2 4 147 77+97=174 77+97=155 False
2 4 148 37+35=072 37+35=085 False
2 4 149 21+89=110 21+89=111 False
2 4 150 58+51=109 58+51=111 False
2 4 151 91+48=139 91+48=135 False
2 4 152 33+23=056 33+23=075 False
2 4 153 80+96=176 80+96=155 False
2 4 154 78+02=080 78+02=095 False
2 4 155 38+95=133 38+95=135 False
2 4 156 99+25=124 99+25=111 False
2 4 157 30+76=106 30+76=111 False
2 4 158 42+40=082 42+40=111 False
2 4 159 85+58=143 85+58=135 False
2 4 160 44+46=090 44+46=111 False
2 4 161 06+41=047 06+41=055 False
2 4 162 65+90=155 65+90=155 True
2 4 163 43+83=126 43+83=135 False
2 4 164 36+61=097 36+61=111 False
2 4 165 61+51=112 61+51=111 False
2 4 166 38+09=047 38+09=075 False
2 4 167 21+97=118 21+97=111 False
2 4 168 83+30=113 83+30=125 False
2 4 169 11+79=090 11+79=095 False
2 4 170 14+29=043 14+29=055 False
2 4 171 21+11=032 21+11=031 False
2 4 172 43+53=096 43+53=111 False
2 4 173 02+58=060 02+58=075 False
2 4 174 78+82=160 78+82=155 False
2 4 175 91+11=102 91+11=111 False
2 4 176 58+54=112 58+54=111 False
2 4 177 00+15=015 00+15=031 False
2 4 178 83+51=134 83+51=131 False
2 4 179 44+72=116 44+72=111 False
2 4 180 71+20=091 71+20=107 False
2 4 181 24+99=123 24+99=111 False
2 4 182 46+30=076 46+30=085 False
2 4 183 08+67=075 08+67=085 False
2 4 184 47+42=089 47+42=111 False
2 4 185 95+67=162 95+67=155 False
2 4 186 40+56=096 40+56=111 False
2 4 187 17+95=112 17+95=111 False
2 4 188 94+66=160 94+66=155 False
2 4 189 14+58=072 14+58=075 False
2 4 190 56+05=061 56+05=075 False
2 4 191 70+01=071 70+01=085 False
2 4 192 97+59=156 97+59=155 False
2 4 193 94+67=161 94+67=155 False
2 4 194 13+41=054 13+41=031 False
2 4 195 85+15=100 85+15=107 False
2 4 196 48+53=101 48+53=111 False
2 4 197 62+75=137 62+75=135 False
2 4 198 87+47=134 87+47=135 False
2 4 199 31+88=119 31+88=125 False
2 4 200 97+16=113 97+16=111 False
2 4 201 48+45=093 48+45=111 False
2 4 202 99+00=099 99+00=095 False
2 4 203 15+01=016 15+01=031 False
2 4 204 28+96=124 28+96=111 False
2 4 205 20+11=031 20+11=031 True
2 4 206 07+56=063 07+56=075 False
2 4 207 06+08=014 06+08=055 False
2 4 208 45+46=091 45+46=111 False
2 4 209 48+85=133 48+85=135 False
2 4 210 62+14=076 62+14=081 False
2 4 211 82+31=113 82+31=111 False
2 4 212 85+88=173 85+88=155 False
2 4 213 77+08=085 77+08=095 False
2 4 214 16+64=080 16+64=081 False
2 4 215 00+27=027 00+27=055 False
2 4 216 36+75=111 36+75=111 True
2 4 217 38+38=076 38+38=085 False
2 4 218 88+32=120 88+32=125 False
2 4 219 09+88=097 09+88=095 False
2 4 220 96+87=183 96+87=177 False
2 4 221 71+29=100 71+29=107 False
2 4 222 99+13=112 99+13=111 False
2 4 223 03+13=016 03+13=031 False
2 4 224 67+23=090 67+23=095 False
2 4 225 15+98=113 15+98=111 False
2 4 226 10+08=018 10+08=033 False
2 4 227 46+24=070 46+24=085 False
2 4 228 55+63=118 55+63=111 False
2 4 229 28+06=034 28+06=055 False
2 4 230 43+87=130 43+87=135 False
2 4 231 34+05=039 34+05=055 False
2024-12-17 09:44:03,445 - root - WARNING - The method `test_one_epoch` is not implemented. Please override this method in a subclass or use a custom callback.
2024-12-17 09:44:03,446 - root - INFO - ====================================================== Starting Train Epoch: 3/9 ======================================================
2024-12-17 09:44:03,447 - root - INFO - Learning rates for each parameter group: 0.00130882305771417282, 0.00130882305771417282
0%| | 0/28 [00:00<?, ?it/s]
Epoch: 3, Step: 1: 0%| | 0/28 [00:00<?, ?it/s]
Epoch: 3, Step: 1: 0%| | 0/28 [00:00<?, ?it/s, total_batch_loss=269.40225, average_batch_loss=1.05235, average_batch_perplexity=2.86438, lr=0.001308823, 0.001308823]
Epoch: 3, Step: 2: 0%| | 0/28 [00:00<?, ?it/s, total_batch_loss=269.40225, average_batch_loss=1.05235, average_batch_perplexity=2.86438, lr=0.001308823, 0.001308823]
Epoch: 3, Step: 2: 0%| | 0/28 [00:00<?, ?it/s, total_batch_loss=266.84833, average_batch_loss=1.04238, average_batch_perplexity=2.83595, lr=0.001331785, 0.001331785]
Epoch: 3, Step: 2: 7%|▋ | 2/28 [00:00<00:02, 12.33it/s, total_batch_loss=266.84833, average_batch_loss=1.04238, average_batch_perplexity=2.83595, lr=0.001331785, 0.001331785]
Epoch: 3, Step: 3: 7%|▋ | 2/28 [00:00<00:02, 12.33it/s, total_batch_loss=266.84833, average_batch_loss=1.04238, average_batch_perplexity=2.83595, lr=0.001331785, 0.001331785]
Epoch: 3, Step: 3: 7%|▋ | 2/28 [00:00<00:02, 12.33it/s, total_batch_loss=266.31369, average_batch_loss=1.04029, average_batch_perplexity=2.83003, lr=0.001354747, 0.001354747]
Epoch: 3, Step: 4: 7%|▋ | 2/28 [00:00<00:02, 12.33it/s, total_batch_loss=266.31369, average_batch_loss=1.04029, average_batch_perplexity=2.83003, lr=0.001354747, 0.001354747]
Epoch: 3, Step: 4: 7%|▋ | 2/28 [00:00<00:02, 12.33it/s, total_batch_loss=260.87216, average_batch_loss=1.01903, average_batch_perplexity=2.77051, lr=0.001377708, 0.001377708]
Epoch: 3, Step: 4: 14%|█▍ | 4/28 [00:00<00:01, 12.58it/s, total_batch_loss=260.87216, average_batch_loss=1.01903, average_batch_perplexity=2.77051, lr=0.001377708, 0.001377708]
Epoch: 3, Step: 5: 14%|█▍ | 4/28 [00:00<00:01, 12.58it/s, total_batch_loss=260.87216, average_batch_loss=1.01903, average_batch_perplexity=2.77051, lr=0.001377708, 0.001377708]
Epoch: 3, Step: 5: 14%|█▍ | 4/28 [00:00<00:01, 12.58it/s, total_batch_loss=262.74243, average_batch_loss=1.02634, average_batch_perplexity=2.79083, lr=0.001400670, 0.001400670]
Epoch: 3, Step: 6: 14%|█▍ | 4/28 [00:00<00:01, 12.58it/s, total_batch_loss=262.74243, average_batch_loss=1.02634, average_batch_perplexity=2.79083, lr=0.001400670, 0.001400670]
Epoch: 3, Step: 6: 14%|█▍ | 4/28 [00:00<00:01, 12.58it/s, total_batch_loss=262.01190, average_batch_loss=1.02348, average_batch_perplexity=2.78287, lr=0.001423632, 0.001423632]
Epoch: 3, Step: 6: 21%|██▏ | 6/28 [00:00<00:01, 12.53it/s, total_batch_loss=262.01190, average_batch_loss=1.02348, average_batch_perplexity=2.78287, lr=0.001423632, 0.001423632]
Epoch: 3, Step: 7: 21%|██▏ | 6/28 [00:00<00:01, 12.53it/s, total_batch_loss=262.01190, average_batch_loss=1.02348, average_batch_perplexity=2.78287, lr=0.001423632, 0.001423632]
Epoch: 3, Step: 7: 21%|██▏ | 6/28 [00:00<00:01, 12.53it/s, total_batch_loss=255.61806, average_batch_loss=0.99851, average_batch_perplexity=2.71423, lr=0.001446594, 0.001446594]
Epoch: 3, Step: 8: 21%|██▏ | 6/28 [00:00<00:01, 12.53it/s, total_batch_loss=255.61806, average_batch_loss=0.99851, average_batch_perplexity=2.71423, lr=0.001446594, 0.001446594]
Epoch: 3, Step: 8: 21%|██▏ | 6/28 [00:00<00:01, 12.53it/s, total_batch_loss=252.61301, average_batch_loss=0.98677, average_batch_perplexity=2.68255, lr=0.001469556, 0.001469556]
Epoch: 3, Step: 8: 29%|██▊ | 8/28 [00:00<00:01, 12.38it/s, total_batch_loss=252.61301, average_batch_loss=0.98677, average_batch_perplexity=2.68255, lr=0.001469556, 0.001469556]
Epoch: 3, Step: 9: 29%|██▊ | 8/28 [00:00<00:01, 12.38it/s, total_batch_loss=252.61301, average_batch_loss=0.98677, average_batch_perplexity=2.68255, lr=0.001469556, 0.001469556]
Epoch: 3, Step: 9: 29%|██▊ | 8/28 [00:00<00:01, 12.38it/s, total_batch_loss=254.40053, average_batch_loss=0.99375, average_batch_perplexity=2.70135, lr=0.001492518, 0.001492518]
Epoch: 3, Step: 10: 29%|██▊ | 8/28 [00:00<00:01, 12.38it/s, total_batch_loss=254.40053, average_batch_loss=0.99375, average_batch_perplexity=2.70135, lr=0.001492518, 0.001492518]
Epoch: 3, Step: 10: 29%|██▊ | 8/28 [00:00<00:01, 12.38it/s, total_batch_loss=247.41672, average_batch_loss=0.96647, average_batch_perplexity=2.62865, lr=0.001515479, 0.001515479]
Epoch: 3, Step: 10: 36%|███▌ | 10/28 [00:00<00:01, 12.34it/s, total_batch_loss=247.41672, average_batch_loss=0.96647, average_batch_perplexity=2.62865, lr=0.001515479, 0.001515479]
Epoch: 3, Step: 11: 36%|███▌ | 10/28 [00:00<00:01, 12.34it/s, total_batch_loss=247.41672, average_batch_loss=0.96647, average_batch_perplexity=2.62865, lr=0.001515479, 0.001515479]
Epoch: 3, Step: 11: 36%|███▌ | 10/28 [00:00<00:01, 12.34it/s, total_batch_loss=251.45728, average_batch_loss=0.98225, average_batch_perplexity=2.67047, lr=0.001538441, 0.001538441]
Epoch: 3, Step: 12: 36%|███▌ | 10/28 [00:00<00:01, 12.34it/s, total_batch_loss=251.45728, average_batch_loss=0.98225, average_batch_perplexity=2.67047, lr=0.001538441, 0.001538441]
Epoch: 3, Step: 12: 36%|███▌ | 10/28 [00:00<00:01, 12.34it/s, total_batch_loss=249.68826, average_batch_loss=0.97534, average_batch_perplexity=2.65208, lr=0.001561403, 0.001561403]
Epoch: 3, Step: 12: 43%|████▎ | 12/28 [00:00<00:01, 12.27it/s, total_batch_loss=249.68826, average_batch_loss=0.97534, average_batch_perplexity=2.65208, lr=0.001561403, 0.001561403]
Epoch: 3, Step: 13: 43%|████▎ | 12/28 [00:01<00:01, 12.27it/s, total_batch_loss=249.68826, average_batch_loss=0.97534, average_batch_perplexity=2.65208, lr=0.001561403, 0.001561403]
Epoch: 3, Step: 13: 43%|████▎ | 12/28 [00:01<00:01, 12.27it/s, total_batch_loss=250.21922, average_batch_loss=0.97742, average_batch_perplexity=2.65759, lr=0.001584365, 0.001584365]
Epoch: 3, Step: 14: 43%|████▎ | 12/28 [00:01<00:01, 12.27it/s, total_batch_loss=250.21922, average_batch_loss=0.97742, average_batch_perplexity=2.65759, lr=0.001584365, 0.001584365]
Epoch: 3, Step: 14: 43%|████▎ | 12/28 [00:01<00:01, 12.27it/s, total_batch_loss=248.51582, average_batch_loss=0.97076, average_batch_perplexity=2.63996, lr=0.001607327, 0.001607327]
Epoch: 3, Step: 14: 50%|█████ | 14/28 [00:01<00:01, 12.34it/s, total_batch_loss=248.51582, average_batch_loss=0.97076, average_batch_perplexity=2.63996, lr=0.001607327, 0.001607327]
Epoch: 3, Step: 15: 50%|█████ | 14/28 [00:01<00:01, 12.34it/s, total_batch_loss=248.51582, average_batch_loss=0.97076, average_batch_perplexity=2.63996, lr=0.001607327, 0.001607327]
Epoch: 3, Step: 15: 50%|█████ | 14/28 [00:01<00:01, 12.34it/s, total_batch_loss=242.27272, average_batch_loss=0.94638, average_batch_perplexity=2.57636, lr=0.001630288, 0.001630288]
Epoch: 3, Step: 16: 50%|█████ | 14/28 [00:01<00:01, 12.34it/s, total_batch_loss=242.27272, average_batch_loss=0.94638, average_batch_perplexity=2.57636, lr=0.001630288, 0.001630288]
Epoch: 3, Step: 16: 50%|█████ | 14/28 [00:01<00:01, 12.34it/s, total_batch_loss=248.85542, average_batch_loss=0.97209, average_batch_perplexity=2.64347, lr=0.001653250, 0.001653250]
Epoch: 3, Step: 16: 57%|█████▋ | 16/28 [00:01<00:00, 12.26it/s, total_batch_loss=248.85542, average_batch_loss=0.97209, average_batch_perplexity=2.64347, lr=0.001653250, 0.001653250]
Epoch: 3, Step: 17: 57%|█████▋ | 16/28 [00:01<00:00, 12.26it/s, total_batch_loss=248.85542, average_batch_loss=0.97209, average_batch_perplexity=2.64347, lr=0.001653250, 0.001653250]
Epoch: 3, Step: 17: 57%|█████▋ | 16/28 [00:01<00:00, 12.26it/s, total_batch_loss=246.14943, average_batch_loss=0.96152, average_batch_perplexity=2.61567, lr=0.001676212, 0.001676212]
Epoch: 3, Step: 18: 57%|█████▋ | 16/28 [00:01<00:00, 12.26it/s, total_batch_loss=246.14943, average_batch_loss=0.96152, average_batch_perplexity=2.61567, lr=0.001676212, 0.001676212]
Epoch: 3, Step: 18: 57%|█████▋ | 16/28 [00:01<00:00, 12.26it/s, total_batch_loss=238.63667, average_batch_loss=0.93217, average_batch_perplexity=2.54003, lr=0.001699174, 0.001699174]
Epoch: 3, Step: 18: 64%|██████▍ | 18/28 [00:01<00:00, 12.29it/s, total_batch_loss=238.63667, average_batch_loss=0.93217, average_batch_perplexity=2.54003, lr=0.001699174, 0.001699174]
Epoch: 3, Step: 19: 64%|██████▍ | 18/28 [00:01<00:00, 12.29it/s, total_batch_loss=238.63667, average_batch_loss=0.93217, average_batch_perplexity=2.54003, lr=0.001699174, 0.001699174]
Epoch: 3, Step: 19: 64%|██████▍ | 18/28 [00:01<00:00, 12.29it/s, total_batch_loss=238.24759, average_batch_loss=0.93065, average_batch_perplexity=2.53617, lr=0.001722136, 0.001722136]
Epoch: 3, Step: 20: 64%|██████▍ | 18/28 [00:01<00:00, 12.29it/s, total_batch_loss=238.24759, average_batch_loss=0.93065, average_batch_perplexity=2.53617, lr=0.001722136, 0.001722136]
Epoch: 3, Step: 20: 64%|██████▍ | 18/28 [00:01<00:00, 12.29it/s, total_batch_loss=236.54965, average_batch_loss=0.92402, average_batch_perplexity=2.51940, lr=0.001745097, 0.001745097]
Epoch: 3, Step: 20: 71%|███████▏ | 20/28 [00:01<00:00, 12.19it/s, total_batch_loss=236.54965, average_batch_loss=0.92402, average_batch_perplexity=2.51940, lr=0.001745097, 0.001745097]
Epoch: 3, Step: 21: 71%|███████▏ | 20/28 [00:01<00:00, 12.19it/s, total_batch_loss=236.54965, average_batch_loss=0.92402, average_batch_perplexity=2.51940, lr=0.001745097, 0.001745097]
Epoch: 3, Step: 21: 71%|███████▏ | 20/28 [00:01<00:00, 12.19it/s, total_batch_loss=233.92700, average_batch_loss=0.91378, average_batch_perplexity=2.49372, lr=0.001768059, 0.001768059]
Epoch: 3, Step: 22: 71%|███████▏ | 20/28 [00:01<00:00, 12.19it/s, total_batch_loss=233.92700, average_batch_loss=0.91378, average_batch_perplexity=2.49372, lr=0.001768059, 0.001768059]
Epoch: 3, Step: 22: 71%|███████▏ | 20/28 [00:01<00:00, 12.19it/s, total_batch_loss=234.13594, average_batch_loss=0.91459, average_batch_perplexity=2.49576, lr=0.001791021, 0.001791021]
Epoch: 3, Step: 22: 79%|███████▊ | 22/28 [00:01<00:00, 12.37it/s, total_batch_loss=234.13594, average_batch_loss=0.91459, average_batch_perplexity=2.49576, lr=0.001791021, 0.001791021]
Epoch: 3, Step: 23: 79%|███████▊ | 22/28 [00:01<00:00, 12.37it/s, total_batch_loss=234.13594, average_batch_loss=0.91459, average_batch_perplexity=2.49576, lr=0.001791021, 0.001791021]
Epoch: 3, Step: 23: 79%|███████▊ | 22/28 [00:01<00:00, 12.37it/s, total_batch_loss=242.58105, average_batch_loss=0.94758, average_batch_perplexity=2.57947, lr=0.001813983, 0.001813983]
Epoch: 3, Step: 24: 79%|███████▊ | 22/28 [00:01<00:00, 12.37it/s, total_batch_loss=242.58105, average_batch_loss=0.94758, average_batch_perplexity=2.57947, lr=0.001813983, 0.001813983]
Epoch: 3, Step: 24: 79%|███████▊ | 22/28 [00:01<00:00, 12.37it/s, total_batch_loss=236.37764, average_batch_loss=0.92335, average_batch_perplexity=2.51771, lr=0.001836945, 0.001836945]
Epoch: 3, Step: 24: 86%|████████▌ | 24/28 [00:01<00:00, 12.49it/s, total_batch_loss=236.37764, average_batch_loss=0.92335, average_batch_perplexity=2.51771, lr=0.001836945, 0.001836945]
Epoch: 3, Step: 25: 86%|████████▌ | 24/28 [00:02<00:00, 12.49it/s, total_batch_loss=236.37764, average_batch_loss=0.92335, average_batch_perplexity=2.51771, lr=0.001836945, 0.001836945]
Epoch: 3, Step: 25: 86%|████████▌ | 24/28 [00:02<00:00, 12.49it/s, total_batch_loss=234.06291, average_batch_loss=0.91431, average_batch_perplexity=2.49505, lr=0.001859906, 0.001859906]
Epoch: 3, Step: 26: 86%|████████▌ | 24/28 [00:02<00:00, 12.49it/s, total_batch_loss=234.06291, average_batch_loss=0.91431, average_batch_perplexity=2.49505, lr=0.001859906, 0.001859906]
Epoch: 3, Step: 26: 86%|████████▌ | 24/28 [00:02<00:00, 12.49it/s, total_batch_loss=233.00504, average_batch_loss=0.91018, average_batch_perplexity=2.48476, lr=0.001882868, 0.001882868]
Epoch: 3, Step: 26: 93%|█████████▎| 26/28 [00:02<00:00, 12.35it/s, total_batch_loss=233.00504, average_batch_loss=0.91018, average_batch_perplexity=2.48476, lr=0.001882868, 0.001882868]
Epoch: 3, Step: 27: 93%|█████████▎| 26/28 [00:02<00:00, 12.35it/s, total_batch_loss=233.00504, average_batch_loss=0.91018, average_batch_perplexity=2.48476, lr=0.001882868, 0.001882868]
Epoch: 3, Step: 27: 93%|█████████▎| 26/28 [00:02<00:00, 12.35it/s, total_batch_loss=232.18446, average_batch_loss=0.90697, average_batch_perplexity=2.47681, lr=0.001905830, 0.001905830]
Epoch: 3, Step: 28: 93%|█████████▎| 26/28 [00:02<00:00, 12.35it/s, total_batch_loss=232.18446, average_batch_loss=0.90697, average_batch_perplexity=2.47681, lr=0.001905830, 0.001905830]
Epoch: 3, Step: 28: 93%|█████████▎| 26/28 [00:02<00:00, 12.35it/s, total_batch_loss=78.54578, average_batch_loss=0.89257, average_batch_perplexity=2.44139, lr=0.001928792, 0.001928792]
Epoch: 3, Step: 28: 100%|██████████| 28/28 [00:02<00:00, 13.50it/s, total_batch_loss=78.54578, average_batch_loss=0.89257, average_batch_perplexity=2.44139, lr=0.001928792, 0.001928792]
2024-12-17 09:44:05,672 - root - INFO - Total Samples: 7000
2024-12-17 09:44:05,672 - root - INFO - Total Batches: 28
2024-12-17 09:44:05,673 - root - INFO - Average Epoch Train Loss: 0.96766
2024-12-17 09:44:05,673 - root - INFO - Average Epoch Train Perplexity: 2.63179
2024-12-17 09:44:05,674 - root - INFO -
2024-12-17 09:44:05,674 - root - INFO - ====================================================== Starting Valid Epoch: 3/9 ======================================================
0%| | 0/8 [00:00<?, ?it/s]
Epoch: 3, Step: 1: 0%| | 0/8 [00:00<?, ?it/s]
Epoch: 3, Step: 1: 0%| | 0/8 [00:00<?, ?it/s, total_batch_loss=226.52034, average_batch_loss=0.88485, average_batch_perplexity=2.42261]
Epoch: 3, Step: 2: 0%| | 0/8 [00:00<?, ?it/s, total_batch_loss=226.52034, average_batch_loss=0.88485, average_batch_perplexity=2.42261]
Epoch: 3, Step: 2: 0%| | 0/8 [00:00<?, ?it/s, total_batch_loss=220.53197, average_batch_loss=0.86145, average_batch_perplexity=2.36660]
Epoch: 3, Step: 3: 0%| | 0/8 [00:00<?, ?it/s, total_batch_loss=220.53197, average_batch_loss=0.86145, average_batch_perplexity=2.36660]
Epoch: 3, Step: 3: 0%| | 0/8 [00:00<?, ?it/s, total_batch_loss=222.36171, average_batch_loss=0.86860, average_batch_perplexity=2.38357]
Epoch: 3, Step: 3: 38%|███▊ | 3/8 [00:00<00:00, 23.14it/s, total_batch_loss=222.36171, average_batch_loss=0.86860, average_batch_perplexity=2.38357]
Epoch: 3, Step: 4: 38%|███▊ | 3/8 [00:00<00:00, 23.14it/s, total_batch_loss=222.36171, average_batch_loss=0.86860, average_batch_perplexity=2.38357]
Epoch: 3, Step: 4: 38%|███▊ | 3/8 [00:00<00:00, 23.14it/s, total_batch_loss=226.95125, average_batch_loss=0.88653, average_batch_perplexity=2.42669]
Epoch: 3, Step: 5: 38%|███▊ | 3/8 [00:00<00:00, 23.14it/s, total_batch_loss=226.95125, average_batch_loss=0.88653, average_batch_perplexity=2.42669]
Epoch: 3, Step: 5: 38%|███▊ | 3/8 [00:00<00:00, 23.14it/s, total_batch_loss=228.85178, average_batch_loss=0.89395, average_batch_perplexity=2.44477]
Epoch: 3, Step: 6: 38%|███▊ | 3/8 [00:00<00:00, 23.14it/s, total_batch_loss=228.85178, average_batch_loss=0.89395, average_batch_perplexity=2.44477]
Epoch: 3, Step: 6: 38%|███▊ | 3/8 [00:00<00:00, 23.14it/s, total_batch_loss=222.37132, average_batch_loss=0.86864, average_batch_perplexity=2.38366]
Epoch: 3, Step: 6: 75%|███████▌ | 6/8 [00:00<00:00, 23.76it/s, total_batch_loss=222.37132, average_batch_loss=0.86864, average_batch_perplexity=2.38366]
Epoch: 3, Step: 7: 75%|███████▌ | 6/8 [00:00<00:00, 23.76it/s, total_batch_loss=222.37132, average_batch_loss=0.86864, average_batch_perplexity=2.38366]
Epoch: 3, Step: 7: 75%|███████▌ | 6/8 [00:00<00:00, 23.76it/s, total_batch_loss=223.47276, average_batch_loss=0.87294, average_batch_perplexity=2.39394]
Epoch: 3, Step: 8: 75%|███████▌ | 6/8 [00:00<00:00, 23.76it/s, total_batch_loss=223.47276, average_batch_loss=0.87294, average_batch_perplexity=2.39394]
Epoch: 3, Step: 8: 75%|███████▌ | 6/8 [00:00<00:00, 23.76it/s, total_batch_loss=178.52605, average_batch_loss=0.85830, average_batch_perplexity=2.35914]
2024-12-17 09:44:06,007 - root - INFO - Total Samples: 2000
2024-12-17 09:44:06,008 - root - INFO - Total Batches: 8
2024-12-17 09:44:06,008 - root - INFO - Average Epoch Valid Loss: 0.87479
2024-12-17 09:44:06,009 - root - INFO - Average Epoch Valid Perplexity: 2.39838
2024-12-17 09:44:06,009 - root - INFO -
Evaluating and Generation.: 0%| | 0/4 [00:00<?, ?it/s]
Evaluating and Generation.: 0%| | 0/4 [00:00<?, ?it/s, accuracy: 0.0430]
Evaluating and Generation.: 0%| | 0/4 [00:00<?, ?it/s, accuracy: 0.0352]
Evaluating and Generation.: 50%|█████ | 2/4 [00:00<00:00, 10.63it/s, accuracy: 0.0352]
Evaluating and Generation.: 50%|█████ | 2/4 [00:00<00:00, 10.63it/s, accuracy: 0.0703]
Evaluating and Generation.: 50%|█████ | 2/4 [00:00<00:00, 10.63it/s, accuracy: 0.0517]
Evaluating and Generation.: 100%|██████████| 4/4 [00:00<00:00, 10.98it/s, accuracy: 0.0517]
2024-12-17 09:44:06,381 - root - INFO - Correct/Total Samples: 50/1000
2024-12-17 09:44:06,382 - root - INFO - Eval Accuracy: 0.05
2024-12-17 09:44:06,400 - root - INFO -
epoch batch_index sample_index equation generated correct
3 1 0 13+48=061 13+48=058 False
3 1 1 16+55=071 16+55=078 False
3 1 2 79+34=113 79+34=108 False
3 1 3 35+44=079 35+44=078 False
3 1 4 16+50=066 16+50=078 False
3 1 5 28+47=075 28+47=078 False
3 1 6 00+74=074 00+74=078 False
3 1 7 15+20=035 15+20=050 False
3 1 8 72+60=132 72+60=128 False
3 1 9 63+68=131 63+68=128 False
3 1 10 29+45=074 29+45=078 False
3 1 11 34+60=094 34+60=098 False
3 1 12 53+70=123 53+70=128 False
3 1 13 70+50=120 70+50=113 False
3 1 14 11+84=095 11+84=108 False
3 1 15 42+71=113 42+71=113 True
3 1 16 98+22=120 98+22=113 False
3 1 17 02+02=004 02+02=020 False
3 1 18 15+85=100 15+85=108 False
3 1 19 21+78=099 21+78=098 False
3 1 20 61+79=140 61+79=138 False
3 1 21 25+99=124 25+99=128 False
3 1 22 09+85=094 09+85=098 False
3 1 23 60+91=151 60+91=158 False
3 1 24 35+30=065 35+30=068 False
3 1 25 24+51=075 24+51=078 False
3 1 26 93+91=184 93+91=178 False
3 1 27 39+96=135 39+96=158 False
3 1 28 64+35=099 64+35=098 False
3 1 29 36+22=058 36+22=058 True
3 1 30 68+45=113 68+45=108 False
3 1 31 16+84=100 16+84=108 False
3 1 32 91+52=143 91+52=158 False
3 1 33 97+36=133 97+36=138 False
3 1 34 27+37=064 27+37=068 False
3 1 35 99+82=181 99+82=188 False
3 1 36 03+42=045 03+42=050 False
3 1 37 18+38=056 18+38=058 False
3 1 38 32+20=052 32+20=050 False
3 1 39 38+13=051 38+13=058 False
3 1 40 68+42=110 68+42=108 False
3 1 41 64+00=064 64+00=078 False
3 1 42 48+94=142 48+94=158 False
3 1 43 58+36=094 58+36=098 False
3 1 44 41+22=063 41+22=078 False
3 1 45 23+58=081 23+58=088 False
3 1 46 67+46=113 67+46=108 False
3 1 47 40+78=118 40+78=113 False
3 1 48 90+38=128 90+38=138 False
3 1 49 89+52=141 89+52=158 False
3 1 50 37+77=114 37+77=108 False
3 1 51 29+76=105 29+76=108 False
3 1 52 42+90=132 42+90=148 False
3 1 53 45+82=127 45+82=138 False
3 1 54 35+95=130 35+95=138 False
3 1 55 92+98=190 92+98=188 False
3 1 56 73+91=164 73+91=178 False
3 1 57 53+97=150 53+97=168 False
3 1 58 98+69=167 98+69=178 False
3 1 59 20+46=066 20+46=078 False
3 1 60 48+69=117 48+69=113 False
3 1 61 62+31=093 62+31=098 False
3 1 62 80+59=139 80+59=158 False
3 1 63 58+12=070 58+12=078 False
3 1 64 08+96=104 08+96=108 False
3 1 65 67+06=073 67+06=078 False
3 1 66 22+04=026 22+04=030 False
3 1 67 61+87=148 61+87=158 False
3 1 68 95+27=122 95+27=113 False
3 1 69 49+83=132 49+83=138 False
3 1 70 43+00=043 43+00=050 False
3 1 71 01+85=086 01+85=098 False
3 1 72 11+68=079 11+68=088 False
3 1 73 80+03=083 80+03=098 False
3 1 74 54+83=137 54+83=140 False
3 1 75 73+47=120 73+47=113 False
3 1 76 99+93=192 99+93=188 False
3 1 77 99+13=112 99+13=108 False
3 1 78 92+66=158 92+66=178 False
3 1 79 90+31=121 90+31=128 False
3 1 80 25+69=094 25+69=098 False
3 1 81 25+44=069 25+44=078 False
3 1 82 00+93=093 00+93=108 False
3 1 83 88+87=175 88+87=178 False
3 1 84 47+56=103 47+56=108 False
3 1 85 43+59=102 43+59=108 False
3 1 86 22+00=022 22+00=020 False
3 1 87 34+04=038 34+04=040 False
3 1 88 65+13=078 65+13=088 False
3 1 89 39+82=121 39+82=128 False
3 1 90 66+83=149 66+83=158 False
3 1 91 51+69=120 51+69=113 False
3 1 92 80+21=101 80+21=108 False
3 1 93 36+79=115 36+79=113 False
3 1 94 21+68=089 21+68=098 False
3 1 95 11+66=077 11+66=088 False
3 1 96 55+19=074 55+19=078 False
3 1 97 51+61=112 51+61=112 True
3 1 98 38+88=126 38+88=128 False
3 1 99 37+27=064 37+27=068 False
3 1 100 18+63=081 18+63=088 False
3 1 101 48+11=059 48+11=058 False
3 1 102 72+68=140 72+68=138 False
3 1 103 37+39=076 37+39=088 False
3 1 104 64+95=159 64+95=168 False
3 1 105 49+75=124 49+75=113 False
3 1 106 45+66=111 45+66=113 False
3 1 107 34+87=121 34+87=113 False
3 1 108 02+84=086 02+84=098 False
3 1 109 95+00=095 95+00=108 False
3 1 110 09+56=065 09+56=068 False
3 1 111 22+66=088 22+66=098 False
3 1 112 43+18=061 43+18=058 False
3 1 113 61+35=096 61+35=098 False
3 1 114 13+73=086 13+73=098 False
3 1 115 25+95=120 25+95=128 False
3 1 116 73+96=169 73+96=178 False
3 1 117 03+96=099 03+96=108 False
3 1 118 97+82=179 97+82=178 False
3 1 119 18+42=060 18+42=058 False
3 1 120 29+98=127 29+98=128 False
3 1 121 61+00=061 61+00=078 False
3 1 122 22+98=120 22+98=128 False
3 1 123 12+50=062 12+50=068 False
3 1 124 02+58=060 02+58=058 False
3 1 125 75+86=161 75+86=168 False
3 1 126 31+57=088 31+57=088 True
3 1 127 49+82=131 49+82=138 False
3 1 128 15+33=048 15+33=050 False
3 1 129 49+57=106 49+57=108 False
3 1 130 61+70=131 61+70=128 False
3 1 131 91+51=142 91+51=158 False
3 1 132 50+05=055 50+05=050 False
3 1 133 44+16=060 44+16=058 False
3 1 134 92+01=093 92+01=108 False
3 1 135 85+82=167 85+82=178 False
3 1 136 07+41=048 07+41=058 False
3 1 137 40+06=046 40+06=058 False
3 1 138 79+62=141 79+62=138 False
3 1 139 95+62=157 95+62=168 False
3 1 140 42+93=135 42+93=158 False
3 1 141 32+73=105 32+73=108 False
3 1 142 47+09=056 47+09=058 False
3 1 143 59+50=109 59+50=113 False
3 1 144 61+77=138 61+77=138 True
3 1 145 64+06=070 64+06=078 False
3 1 146 35+10=045 35+10=050 False
3 1 147 32+88=120 32+88=128 False
3 1 148 03+95=098 03+95=108 False
3 1 149 11+38=049 11+38=058 False
3 1 150 21+67=088 21+67=098 False
3 1 151 33+25=058 33+25=050 False
3 1 152 63+45=108 63+45=108 True
3 1 153 56+12=068 56+12=078 False
3 1 154 19+79=098 19+79=098 True
3 1 155 60+43=103 60+43=108 False
3 1 156 07+61=068 07+61=078 False
3 1 157 58+03=061 58+03=068 False
3 1 158 11+10=021 11+10=030 False
3 1 159 49+89=138 49+89=148 False
3 1 160 37+58=095 37+58=098 False
3 1 161 59+78=137 59+78=138 False
3 1 162 11+21=032 11+21=030 False
3 1 163 37+43=080 37+43=088 False
3 1 164 44+21=065 44+21=078 False
3 1 165 22+97=119 22+97=128 False
3 1 166 65+35=100 65+35=098 False
3 1 167 06+51=057 06+51=050 False
3 1 168 65+25=090 65+25=098 False
3 1 169 74+94=168 74+94=178 False
3 1 170 87+55=142 87+55=158 False
3 1 171 90+67=157 90+67=168 False
3 1 172 11+02=013 11+02=020 False
3 1 173 01+66=067 01+66=078 False
3 1 174 56+00=056 56+00=068 False
3 1 175 58+52=110 58+52=113 False
3 1 176 24+99=123 24+99=128 False
3 1 177 97+13=110 97+13=108 False
3 1 178 42+94=136 42+94=140 False
3 1 179 60+15=075 60+15=088 False
3 1 180 20+46=066 20+46=078 False
3 1 181 40+70=110 40+70=113 False
3 1 182 95+45=140 95+45=158 False
3 1 183 96+95=191 96+95=188 False
3 1 184 98+20=118 98+20=113 False
3 1 185 43+19=062 43+19=068 False
3 1 186 50+69=119 50+69=113 False
3 1 187 27+53=080 27+53=088 False
3 1 188 24+25=049 24+25=050 False
3 1 189 65+92=157 65+92=168 False
3 1 190 28+14=042 28+14=058 False
3 1 191 20+57=077 20+57=088 False
3 1 192 59+97=156 59+97=178 False
3 1 193 98+32=130 98+32=138 False
3 1 194 55+84=139 55+84=150 False
3 1 195 20+39=059 20+39=068 False
3 1 196 86+47=133 86+47=138 False
3 1 197 92+36=128 92+36=138 False
3 1 198 05+38=043 05+38=050 False
3 1 199 77+36=113 77+36=108 False
3 1 200 41+64=105 41+64=108 False
3 1 201 74+51=125 74+51=128 False
3 1 202 74+55=129 74+55=120 False
3 1 203 64+64=128 64+64=120 False
3 1 204 60+19=079 60+19=088 False
3 1 205 77+96=173 77+96=178 False
3 1 206 22+30=052 22+30=050 False
3 1 207 82+49=131 82+49=138 False
3 1 208 39+67=106 39+67=108 False
3 1 209 62+40=102 62+40=108 False
3 1 210 28+71=099 28+71=098 False
3 1 211 47+26=073 47+26=078 False
3 1 212 98+54=152 98+54=168 False
3 1 213 38+70=108 38+70=108 True
3 1 214 63+40=103 63+40=108 False
3 1 215 86+62=148 86+62=158 False
3 1 216 22+65=087 22+65=098 False
3 1 217 41+17=058 41+17=058 True
3 1 218 68+88=156 68+88=178 False
3 1 219 96+70=166 96+70=178 False
3 1 220 99+29=128 99+29=118 False
3 1 221 83+39=122 83+39=113 False
3 1 222 26+55=081 26+55=088 False
3 1 223 53+70=123 53+70=128 False
3 1 224 94+12=106 94+12=108 False
3 1 225 00+37=037 00+37=038 False
3 1 226 36+94=130 36+94=138 False
3 1 227 40+58=098 40+58=098 True
3 1 228 19+80=099 19+80=108 False
3 1 229 49+44=093 49+44=108 False
3 1 230 70+27=097 70+27=098 False
3 1 231 52+80=132 52+80=148 False
3 1 232 77+90=167 77+90=178 False
3 1 233 13+92=105 13+92=113 False
3 1 234 59+09=068 59+09=078 False
3 1 235 33+55=088 33+55=088 True
3 1 236 85+16=101 85+16=108 False
3 1 237 25+65=090 25+65=098 False
3 1 238 46+20=066 46+20=078 False
3 1 239 29+52=081 29+52=088 False
3 1 240 32+36=068 32+36=078 False
3 1 241 47+08=055 47+08=058 False
3 1 242 21+84=105 21+84=108 False
3 1 243 24+45=069 24+45=078 False
3 1 244 29+15=044 29+15=050 False
3 1 245 83+03=086 83+03=098 False
3 1 246 83+36=119 83+36=113 False
3 1 247 58+95=153 58+95=178 False
3 1 248 76+79=155 76+79=158 False
3 1 249 63+30=093 63+30=098 False
3 1 250 38+24=062 38+24=068 False
3 1 251 19+46=065 19+46=068 False
3 1 252 99+66=165 99+66=178 False
3 1 253 95+73=168 95+73=178 False
3 1 254 65+27=092 65+27=098 False
3 1 255 91+83=174 91+83=178 False
3 2 0 65+49=114 65+49=108 False
3 2 1 03+08=011 03+08=020 False
3 2 2 67+81=148 67+81=158 False
3 2 3 47+23=070 47+23=078 False
3 2 4 43+91=134 43+91=148 False
3 2 5 41+67=108 41+67=108 True
3 2 6 02+33=035 02+33=030 False
3 2 7 64+84=148 64+84=150 False
3 2 8 81+64=145 81+64=158 False
3 2 9 80+11=091 80+11=098 False
3 2 10 78+01=079 78+01=078 False
3 2 11 89+18=107 89+18=108 False
3 2 12 45+52=097 45+52=088 False
3 2 13 35+30=065 35+30=068 False
3 2 14 53+32=085 53+32=088 False
3 2 15 49+90=139 49+90=158 False
3 2 16 41+37=078 41+37=088 False
3 2 17 35+14=049 35+14=050 False
3 2 18 92+50=142 92+50=158 False
3 2 19 37+60=097 37+60=098 False
3 2 20 91+61=152 91+61=158 False
3 2 21 80+77=157 80+77=158 False
3 2 22 66+24=090 66+24=098 False
3 2 23 81+07=088 81+07=098 False
3 2 24 85+59=144 85+59=158 False
3 2 25 19+69=088 19+69=098 False
3 2 26 91+44=135 91+44=148 False
3 2 27 25+29=054 25+29=058 False
3 2 28 27+08=035 27+08=048 False
3 2 29 66+14=080 66+14=088 False
3 2 30 95+11=106 95+11=108 False
3 2 31 13+97=110 13+97=113 False
3 2 32 94+40=134 94+40=148 False
3 2 33 74+31=105 74+31=108 False
3 2 34 49+00=049 49+00=058 False
3 2 35 59+18=077 59+18=088 False
3 2 36 07+65=072 07+65=078 False
3 2 37 83+55=138 83+55=158 False
3 2 38 49+80=129 49+80=138 False
3 2 39 64+17=081 64+17=088 False
3 2 40 48+83=131 48+83=138 False
3 2 41 95+44=139 95+44=148 False
3 2 42 71+26=097 71+26=098 False
3 2 43 06+74=080 06+74=078 False
3 2 44 34+24=058 34+24=050 False
3 2 45 59+71=130 59+71=128 False
3 2 46 68+32=100 68+32=098 False
3 2 47 38+81=119 38+81=128 False
3 2 48 29+56=085 29+56=088 False
3 2 49 54+55=109 54+55=108 False
3 2 50 31+27=058 31+27=058 True
3 2 51 97+89=186 97+89=188 False
3 2 52 48+09=057 48+09=058 False
3 2 53 86+76=162 86+76=178 False
3 2 54 82+59=141 82+59=158 False
3 2 55 01+67=068 01+67=078 False
3 2 56 26+06=032 26+06=038 False
3 2 57 22+46=068 22+46=078 False
3 2 58 85+16=101 85+16=108 False
3 2 59 29+08=037 29+08=058 False
3 2 60 73+94=167 73+94=178 False
3 2 61 19+62=081 19+62=088 False
3 2 62 86+62=148 86+62=158 False
3 2 63 38+99=137 38+99=158 False
3 2 64 64+25=089 64+25=098 False
3 2 65 61+72=133 61+72=128 False
3 2 66 78+88=166 78+88=178 False
3 2 67 43+66=109 43+66=108 False
3 2 68 69+35=104 69+35=108 False
3 2 69 33+77=110 33+77=108 False
3 2 70 37+37=074 37+37=078 False
3 2 71 87+54=141 87+54=158 False
3 2 72 68+90=158 68+90=178 False
3 2 73 83+44=127 83+44=128 False
3 2 74 41+09=050 41+09=058 False
3 2 75 13+48=061 13+48=058 False
3 2 76 01+41=042 01+41=050 False
3 2 77 19+74=093 19+74=098 False
3 2 78 15+05=020 15+05=020 True
3 2 79 55+46=101 55+46=098 False
3 2 80 68+33=101 68+33=098 False
3 2 81 44+40=084 44+40=088 False
3 2 82 88+03=091 88+03=098 False
3 2 83 81+79=160 81+79=158 False
3 2 84 18+98=116 18+98=113 False
3 2 85 70+64=134 70+64=128 False
3 2 86 26+44=070 26+44=078 False
3 2 87 98+87=185 98+87=188 False
3 2 88 18+74=092 18+74=098 False
3 2 89 50+68=118 50+68=113 False
3 2 90 13+51=064 13+51=068 False
3 2 91 90+89=179 90+89=178 False
3 2 92 47+78=125 47+78=113 False
3 2 93 81+57=138 81+57=158 False
3 2 94 34+47=081 34+47=088 False
3 2 95 94+23=117 94+23=113 False
3 2 96 07+70=077 07+70=078 False
3 2 97 56+33=089 56+33=088 False
3 2 98 33+04=037 33+04=030 False
3 2 99 26+09=035 26+09=048 False
3 2 100 14+92=106 14+92=113 False
3 2 101 78+54=132 78+54=128 False
3 2 102 36+76=112 36+76=108 False
3 2 103 17+47=064 17+47=068 False
3 2 104 28+18=046 28+18=058 False
3 2 105 78+54=132 78+54=128 False
3 2 106 84+72=156 84+72=158 False
3 2 107 00+44=044 00+44=050 False
3 2 108 50+41=091 50+41=088 False
3 2 109 87+88=175 87+88=178 False
3 2 110 11+66=077 11+66=088 False
3 2 111 80+60=140 80+60=158 False
3 2 112 78+76=154 78+76=158 False
3 2 113 24+74=098 24+74=098 True
3 2 114 88+48=136 88+48=138 False
3 2 115 38+31=069 38+31=078 False
3 2 116 29+27=056 29+27=058 False
3 2 117 08+45=053 08+45=050 False
3 2 118 28+13=041 28+13=058 False
3 2 119 53+99=152 53+99=178 False
3 2 120 47+92=139 47+92=158 False
3 2 121 76+21=097 76+21=098 False
3 2 122 53+96=149 53+96=158 False
3 2 123 93+91=184 93+91=178 False
3 2 124 97+33=130 97+33=138 False
3 2 125 67+78=145 67+78=158 False
3 2 126 58+05=063 58+05=068 False
3 2 127 00+16=016 00+16=020 False
3 2 128 80+19=099 80+19=108 False
3 2 129 98+22=120 98+22=113 False
3 2 130 09+62=071 09+62=078 False
3 2 131 06+23=029 06+23=030 False
3 2 132 32+99=131 32+99=148 False
3 2 133 17+02=019 17+02=028 False
3 2 134 64+35=099 64+35=098 False
3 2 135 35+83=118 35+83=113 False
3 2 136 71+36=107 71+36=108 False
3 2 137 75+06=081 75+06=078 False
3 2 138 88+95=183 88+95=188 False
3 2 139 19+98=117 19+98=113 False
3 2 140 28+89=117 28+89=113 False
3 2 141 33+11=044 33+11=050 False
3 2 142 34+49=083 34+49=088 False
3 2 143 90+35=125 90+35=128 False
3 2 144 22+90=112 22+90=113 False
3 2 145 98+89=187 98+89=188 False
3 2 146 88+47=135 88+47=138 False
3 2 147 30+86=116 30+86=113 False
3 2 148 31+48=079 31+48=088 False
3 2 149 39+21=060 39+21=068 False
3 2 150 19+17=036 19+17=048 False
3 2 151 27+60=087 27+60=098 False
3 2 152 12+16=028 12+16=030 False
3 2 153 51+75=126 51+75=120 False
3 2 154 10+74=084 10+74=098 False
3 2 155 42+63=105 42+63=108 False
3 2 156 40+14=054 40+14=050 False
3 2 157 23+93=116 23+93=128 False
3 2 158 85+26=111 85+26=108 False
3 2 159 28+46=074 28+46=078 False
3 2 160 28+33=061 28+33=058 False
3 2 161 43+30=073 43+30=078 False
3 2 162 89+72=161 89+72=178 False
3 2 163 52+21=073 52+21=078 False
3 2 164 21+54=075 21+54=078 False
3 2 165 69+13=082 69+13=098 False
3 2 166 07+60=067 07+60=078 False
3 2 167 63+83=146 63+83=150 False
3 2 168 80+69=149 80+69=158 False
3 2 169 27+28=055 27+28=058 False
3 2 170 42+31=073 42+31=078 False
3 2 171 51+99=150 51+99=158 False
3 2 172 28+75=103 28+75=108 False
3 2 173 38+57=095 38+57=098 False
3 2 174 83+16=099 83+16=108 False
3 2 175 92+94=186 92+94=178 False
3 2 176 55+75=130 55+75=120 False
3 2 177 59+51=110 59+51=113 False
3 2 178 33+09=042 33+09=050 False
3 2 179 53+13=066 53+13=078 False
3 2 180 05+70=075 05+70=078 False
3 2 181 12+20=032 12+20=030 False
3 2 182 11+49=060 11+49=068 False
3 2 183 63+45=108 63+45=108 True
3 2 184 92+23=115 92+23=113 False
3 2 185 82+45=127 82+45=128 False
3 2 186 23+41=064 23+41=068 False
3 2 187 64+26=090 64+26=098 False
3 2 188 91+24=115 91+24=113 False
3 2 189 20+32=052 20+32=050 False
3 2 190 83+21=104 83+21=108 False
3 2 191 07+20=027 07+20=038 False
3 2 192 94+14=108 94+14=108 True
3 2 193 96+89=185 96+89=188 False
3 2 194 13+08=021 13+08=030 False
3 2 195 32+05=037 32+05=030 False
3 2 196 09+51=060 09+51=068 False
3 2 197 26+29=055 26+29=058 False
3 2 198 49+65=114 49+65=113 False
3 2 199 32+66=098 32+66=098 True
3 2 200 41+08=049 41+08=058 False
3 2 201 26+79=105 26+79=108 False
3 2 202 29+91=120 29+91=128 False
3 2 203 51+00=051 51+00=050 False
3 2 204 61+60=121 61+60=113 False
3 2 205 45+78=123 45+78=113 False
3 2 206 56+16=072 56+16=078 False
3 2 207 66+68=134 66+68=138 False
3 2 208 32+16=048 32+16=058 False
3 2 209 84+49=133 84+49=138 False
3 2 210 45+09=054 45+09=058 False
3 2 211 96+78=174 96+78=178 False
3 2 212 10+02=012 10+02=020 False
3 2 213 36+60=096 36+60=098 False
3 2 214 44+36=080 44+36=088 False
3 2 215 12+86=098 12+86=108 False
3 2 216 94+54=148 94+54=158 False
3 2 217 64+73=137 64+73=130 False
3 2 218 73+10=083 73+10=088 False
3 2 219 14+62=076 14+62=088 False
3 2 220 25+22=047 25+22=050 False
3 2 221 94+22=116 94+22=113 False
3 2 222 41+76=117 41+76=113 False
3 2 223 38+46=084 38+46=088 False
3 2 224 71+72=143 71+72=138 False
3 2 225 74+79=153 74+79=158 False
3 2 226 99+67=166 99+67=178 False
3 2 227 78+71=149 78+71=148 False
3 2 228 23+19=042 23+19=058 False
3 2 229 51+65=116 51+65=112 False
3 2 230 94+86=180 94+86=178 False
3 2 231 09+79=088 09+79=088 True
3 2 232 69+39=108 69+39=108 True
3 2 233 84+13=097 84+13=098 False
3 2 234 36+59=095 36+59=108 False
3 2 235 87+47=134 87+47=138 False
3 2 236 50+00=050 50+00=058 False
3 2 237 76+96=172 76+96=178 False
3 2 238 12+18=030 12+18=038 False
3 2 239 99+95=194 99+95=188 False
3 2 240 22+00=022 22+00=020 False
3 2 241 96+18=114 96+18=108 False
3 2 242 51+20=071 51+20=078 False
3 2 243 66+81=147 66+81=158 False
3 2 244 78+18=096 78+18=098 False
3 2 245 09+78=087 09+78=088 False
3 2 246 24+20=044 24+20=050 False
3 2 247 76+13=089 76+13=088 False
3 2 248 05+10=015 05+10=020 False
3 2 249 97+14=111 97+14=108 False
3 2 250 92+38=130 92+38=138 False
3 2 251 77+13=090 77+13=088 False
3 2 252 70+19=089 70+19=098 False
3 2 253 90+45=135 90+45=148 False
3 2 254 50+09=059 50+09=068 False
3 2 255 78+06=084 78+06=088 False
3 3 0 03+25=028 03+25=030 False
3 3 1 48+43=091 48+43=088 False
3 3 2 39+47=086 39+47=088 False
3 3 3 48+19=067 48+19=078 False
3 3 4 07+22=029 07+22=038 False
3 3 5 73+68=141 73+68=138 False
3 3 6 14+56=070 14+56=078 False
3 3 7 96+95=191 96+95=188 False
3 3 8 96+28=124 96+28=113 False
3 3 9 82+05=087 82+05=098 False
3 3 10 27+94=121 27+94=128 False
3 3 11 87+86=173 87+86=178 False
3 3 12 00+68=068 00+68=078 False
3 3 13 11+37=048 11+37=058 False
3 3 14 95+93=188 95+93=188 True
3 3 15 75+82=157 75+82=158 False
3 3 16 41+71=112 41+71=113 False
3 3 17 60+14=074 60+14=088 False
3 3 18 77+77=154 77+77=158 False
3 3 19 31+84=115 31+84=113 False
3 3 20 31+57=088 31+57=088 True
3 3 21 27+87=114 27+87=113 False
3 3 22 31+89=120 31+89=113 False
3 3 23 22+18=040 22+18=058 False
3 3 24 38+25=063 38+25=068 False
3 3 25 64+54=118 64+54=112 False
3 3 26 85+60=145 85+60=158 False
3 3 27 14+71=085 14+71=098 False
3 3 28 06+16=022 06+16=020 False
3 3 29 78+61=139 78+61=138 False
3 3 30 65+75=140 65+75=130 False
3 3 31 13+83=096 13+83=108 False
3 3 32 75+49=124 75+49=113 False
3 3 33 05+78=083 05+78=078 False
3 3 34 66+55=121 66+55=113 False
3 3 35 03+05=008 03+05=020 False
3 3 36 69+99=168 69+99=178 False
3 3 37 52+82=134 52+82=140 False
3 3 38 45+97=142 45+97=158 False
3 3 39 66+17=083 66+17=088 False
3 3 40 36+17=053 36+17=058 False
3 3 41 92+74=166 92+74=178 False
3 3 42 48+44=092 48+44=088 False
3 3 43 34+17=051 34+17=058 False
3 3 44 56+11=067 56+11=078 False
3 3 45 77+23=100 77+23=098 False
3 3 46 10+11=021 10+11=030 False
3 3 47 32+65=097 32+65=098 False
3 3 48 53+49=102 53+49=108 False
3 3 49 68+86=154 68+86=168 False
3 3 50 52+94=146 52+94=150 False
3 3 51 97+71=168 97+71=178 False
3 3 52 05+37=042 05+37=040 False
3 3 53 58+75=133 58+75=138 False
3 3 54 06+24=030 06+24=030 True
3 3 55 15+44=059 15+44=050 False
3 3 56 90+49=139 90+49=158 False
3 3 57 50+37=087 50+37=088 False
3 3 58 88+61=149 88+61=158 False
3 3 59 21+57=078 21+57=088 False
3 3 60 24+85=109 24+85=113 False
3 3 61 01+66=067 01+66=078 False
3 3 62 50+46=096 50+46=088 False
3 3 63 76+65=141 76+65=138 False
3 3 64 23+74=097 23+74=098 False
3 3 65 76+16=092 76+16=098 False
3 3 66 06+08=014 06+08=028 False
3 3 67 69+25=094 69+25=098 False
3 3 68 15+23=038 15+23=050 False
3 3 69 41+02=043 41+02=050 False
3 3 70 16+66=082 16+66=088 False
3 3 71 59+94=153 59+94=168 False
3 3 72 32+88=120 32+88=128 False
3 3 73 46+21=067 46+21=078 False
3 3 74 57+28=085 57+28=088 False
3 3 75 00+31=031 00+31=030 False
3 3 76 77+07=084 77+07=078 False
3 3 77 28+70=098 28+70=098 True
3 3 78 05+61=066 05+61=078 False
3 3 79 22+09=031 22+09=038 False
3 3 80 08+94=102 08+94=108 False
3 3 81 40+11=051 40+11=050 False
3 3 82 10+48=058 10+48=058 True
3 3 83 27+56=083 27+56=088 False
3 3 84 42+16=058 42+16=058 True
3 3 85 69+43=112 69+43=108 False
3 3 86 57+69=126 57+69=128 False
3 3 87 18+86=104 18+86=108 False
3 3 88 86+80=166 86+80=178 False
3 3 89 30+85=115 30+85=113 False
3 3 90 77+66=143 77+66=138 False
3 3 91 39+64=103 39+64=108 False
3 3 92 76+61=137 76+61=138 False
3 3 93 42+61=103 42+61=108 False
3 3 94 07+30=037 07+30=038 False
3 3 95 35+93=128 35+93=138 False
3 3 96 40+90=130 40+90=148 False
3 3 97 08+91=099 08+91=108 False
3 3 98 62+34=096 62+34=098 False
3 3 99 86+49=135 86+49=138 False
3 3 100 73+23=096 73+23=098 False
3 3 101 87+35=122 87+35=113 False
3 3 102 35+31=066 35+31=068 False
3 3 103 07+13=020 07+13=020 True
3 3 104 39+41=080 39+41=088 False
3 3 105 44+63=107 44+63=108 False
3 3 106 94+66=160 94+66=178 False
3 3 107 49+54=103 49+54=108 False
3 3 108 79+46=125 79+46=113 False
3 3 109 53+12=065 53+12=068 False
3 3 110 60+92=152 60+92=158 False
3 3 111 25+60=085 25+60=098 False
3 3 112 64+53=117 64+53=113 False
3 3 113 41+02=043 41+02=050 False
3 3 114 00+97=097 00+97=108 False
3 3 115 12+52=064 12+52=068 False
3 3 116 39+50=089 39+50=088 False
3 3 117 87+21=108 87+21=108 True
3 3 118 04+99=103 04+99=108 False
3 3 119 19+75=094 19+75=098 False
3 3 120 90+05=095 90+05=108 False
3 3 121 54+39=093 54+39=098 False
3 3 122 29+26=055 29+26=058 False
3 3 123 82+95=177 82+95=178 False
3 3 124 55+09=064 55+09=078 False
3 3 125 02+62=064 02+62=078 False
3 3 126 68+30=098 68+30=098 True
3 3 127 99+16=115 99+16=108 False
3 3 128 63+11=074 63+11=088 False
3 3 129 42+92=134 42+92=148 False
3 3 130 99+16=115 99+16=108 False
3 3 131 50+31=081 50+31=088 False
3 3 132 23+46=069 23+46=078 False
3 3 133 45+73=118 45+73=112 False
3 3 134 89+77=166 89+77=178 False
3 3 135 45+78=123 45+78=113 False
3 3 136 96+60=156 96+60=168 False
3 3 137 74+61=135 74+61=128 False
3 3 138 87+01=088 87+01=098 False
3 3 139 63+88=151 63+88=158 False
3 3 140 59+72=131 59+72=128 False
3 3 141 17+96=113 17+96=113 True
3 3 142 89+77=166 89+77=178 False
3 3 143 24+69=093 24+69=098 False
3 3 144 75+83=158 75+83=158 True
3 3 145 50+54=104 50+54=108 False
3 3 146 93+47=140 93+47=158 False
3 3 147 20+55=075 20+55=078 False
3 3 148 91+79=170 91+79=178 False
3 3 149 15+13=028 15+13=030 False
3 3 150 86+09=095 86+09=098 False
3 3 151 29+58=087 29+58=088 False
3 3 152 01+29=030 01+29=030 True
3 3 153 65+48=113 65+48=108 False
3 3 154 96+45=141 96+45=158 False
3 3 155 58+69=127 58+69=128 False
3 3 156 84+43=127 84+43=128 False
3 3 157 90+38=128 90+38=138 False
3 3 158 39+97=136 39+97=158 False
3 3 159 74+84=158 74+84=158 True
3 3 160 86+22=108 86+22=108 True
3 3 161 01+86=087 01+86=098 False
3 3 162 81+63=144 81+63=158 False
3 3 163 80+94=174 80+94=178 False
3 3 164 44+42=086 44+42=088 False
3 3 165 72+60=132 72+60=128 False
3 3 166 28+07=035 28+07=048 False
3 3 167 69+54=123 69+54=113 False
3 3 168 68+77=145 68+77=158 False
3 3 169 90+16=106 90+16=108 False
3 3 170 64+50=114 64+50=113 False
3 3 171 46+88=134 46+88=138 False
3 3 172 55+99=154 55+99=178 False
3 3 173 31+97=128 31+97=138 False
3 3 174 79+28=107 79+28=108 False
3 3 175 81+43=124 81+43=128 False
3 3 176 41+15=056 41+15=050 False
3 3 177 38+77=115 38+77=113 False
3 3 178 25+06=031 25+06=030 False
3 3 179 01+93=094 01+93=108 False
3 3 180 97+22=119 97+22=113 False
3 3 181 71+84=155 71+84=150 False
3 3 182 26+36=062 26+36=058 False
3 3 183 60+92=152 60+92=158 False
3 3 184 02+94=096 02+94=108 False
3 3 185 31+58=089 31+58=088 False
3 3 186 70+52=122 70+52=113 False
3 3 187 19+42=061 19+42=068 False
3 3 188 95+73=168 95+73=178 False
3 3 189 21+25=046 21+25=050 False
3 3 190 13+58=071 13+58=078 False
3 3 191 62+28=090 62+28=098 False
3 3 192 38+14=052 38+14=058 False
3 3 193 66+75=141 66+75=138 False
3 3 194 24+59=083 24+59=088 False
3 3 195 97+66=163 97+66=178 False
3 3 196 76+70=146 76+70=148 False
3 3 197 08+40=048 08+40=058 False
3 3 198 84+00=084 84+00=098 False
3 3 199 54+73=127 54+73=120 False
3 3 200 16+88=104 16+88=108 False
3 3 201 99+47=146 99+47=158 False
3 3 202 31+95=126 31+95=138 False
3 3 203 01+79=080 01+79=078 False
3 3 204 03+68=071 03+68=078 False
3 3 205 10+05=015 10+05=020 False
3 3 206 98+90=188 98+90=188 True
3 3 207 58+53=111 58+53=113 False
3 3 208 34+87=121 34+87=113 False
3 3 209 07+31=038 07+31=038 True
3 3 210 59+08=067 59+08=078 False
3 3 211 51+38=089 51+38=098 False
3 3 212 62+62=124 62+62=113 False
3 3 213 80+32=112 80+32=113 False
3 3 214 69+16=085 69+16=098 False
3 3 215 01+17=018 01+17=020 False
3 3 216 74+41=115 74+41=113 False
3 3 217 20+89=109 20+89=113 False
3 3 218 53+50=103 53+50=108 False
3 3 219 82+85=167 82+85=178 False
3 3 220 34+47=081 34+47=088 False
3 3 221 34+45=079 34+45=078 False
3 3 222 77+34=111 77+34=108 False
3 3 223 56+33=089 56+33=088 False
3 3 224 97+56=153 97+56=178 False
3 3 225 29+06=035 29+06=058 False
3 3 226 78+96=174 78+96=178 False
3 3 227 28+65=093 28+65=098 False
3 3 228 61+64=125 61+64=113 False
3 3 229 32+64=096 32+64=098 False
3 3 230 98+32=130 98+32=138 False
3 3 231 25+35=060 25+35=050 False
3 3 232 05+08=013 05+08=020 False
3 3 233 05+26=031 05+26=030 False
3 3 234 84+71=155 84+71=158 False
3 3 235 33+10=043 33+10=050 False
3 3 236 98+35=133 98+35=138 False
3 3 237 68+98=166 68+98=178 False
3 3 238 03+63=066 03+63=078 False
3 3 239 12+96=108 12+96=113 False
3 3 240 02+81=083 02+81=098 False
3 3 241 83+13=096 83+13=098 False
3 3 242 55+92=147 55+92=158 False
3 3 243 96+09=105 96+09=108 False
3 3 244 61+08=069 61+08=078 False
3 3 245 39+75=114 39+75=108 False
3 3 246 40+74=114 40+74=113 False
3 3 247 39+80=119 39+80=128 False
3 3 248 57+95=152 57+95=168 False
3 3 249 92+97=189 92+97=188 False
3 3 250 33+03=036 33+03=030 False
3 3 251 74+92=166 74+92=178 False
3 3 252 99+09=108 99+09=108 True
3 3 253 98+10=108 98+10=108 True
3 3 254 46+77=123 46+77=113 False
3 3 255 85+78=163 85+78=178 False
3 4 0 41+21=062 41+21=068 False
3 4 1 49+13=062 49+13=068 False
3 4 2 59+07=066 59+07=078 False
3 4 3 31+11=042 31+11=050 False
3 4 4 74+16=090 74+16=098 False
3 4 5 43+38=081 43+38=088 False
3 4 6 08+67=075 08+67=078 False
3 4 7 31+66=097 31+66=098 False
3 4 8 10+31=041 10+31=050 False
3 4 9 34+59=093 34+59=098 False
3 4 10 78+42=120 78+42=113 False
3 4 11 13+41=054 13+41=050 False
3 4 12 97+89=186 97+89=188 False
3 4 13 15+62=077 15+62=088 False
3 4 14 39+36=075 39+36=078 False
3 4 15 21+25=046 21+25=050 False
3 4 16 74+56=130 74+56=128 False
3 4 17 85+47=132 85+47=138 False
3 4 18 47+32=079 47+32=088 False
3 4 19 37+66=103 37+66=098 False
3 4 20 16+29=045 16+29=058 False
3 4 21 86+77=163 86+77=178 False
3 4 22 80+07=087 80+07=098 False
3 4 23 87+05=092 87+05=098 False
3 4 24 58+16=074 58+16=078 False
3 4 25 52+79=131 52+79=128 False
3 4 26 91+08=099 91+08=108 False
3 4 27 47+78=125 47+78=113 False
3 4 28 86+96=182 86+96=188 False
3 4 29 90+22=112 90+22=113 False
3 4 30 31+18=049 31+18=058 False
3 4 31 86+15=101 86+15=108 False
3 4 32 15+95=110 15+95=113 False
3 4 33 42+11=053 42+11=050 False
3 4 34 65+99=164 65+99=178 False
3 4 35 89+29=118 89+29=118 True
3 4 36 35+11=046 35+11=050 False
3 4 37 71+41=112 71+41=108 False
3 4 38 16+24=040 16+24=050 False
3 4 39 77+82=159 77+82=158 False
3 4 40 55+89=144 55+89=158 False
3 4 41 17+88=105 17+88=108 False
3 4 42 54+72=126 54+72=120 False
3 4 43 34+98=132 34+98=148 False
3 4 44 09+97=106 09+97=108 False
3 4 45 91+07=098 91+07=108 False
3 4 46 55+94=149 55+94=150 False
3 4 47 22+58=080 22+58=088 False
3 4 48 91+37=128 91+37=128 True
3 4 49 16+10=026 16+10=038 False
3 4 50 96+32=128 96+32=138 False
3 4 51 35+75=110 35+75=108 False
3 4 52 88+73=161 88+73=178 False
3 4 53 35+18=053 35+18=058 False
3 4 54 33+10=043 33+10=050 False
3 4 55 08+50=058 08+50=058 True
3 4 56 22+62=084 22+62=098 False
3 4 57 26+37=063 26+37=058 False
3 4 58 80+27=107 80+27=108 False
3 4 59 68+28=096 68+28=098 False
3 4 60 48+03=051 48+03=058 False
3 4 61 40+18=058 40+18=058 True
3 4 62 16+59=075 16+59=078 False
3 4 63 02+19=021 02+19=020 False
3 4 64 01+09=010 01+09=020 False
3 4 65 62+68=130 62+68=128 False
3 4 66 09+71=080 09+71=078 False
3 4 67 00+58=058 00+58=058 True
3 4 68 16+45=061 16+45=050 False
3 4 69 24+98=122 24+98=128 False
3 4 70 47+92=139 47+92=158 False
3 4 71 94+84=178 94+84=178 True
3 4 72 21+32=053 21+32=050 False
3 4 73 29+82=111 29+82=113 False
3 4 74 32+79=111 32+79=108 False
3 4 75 13+98=111 13+98=113 False
3 4 76 41+94=135 41+94=140 False
3 4 77 51+84=135 51+84=130 False
3 4 78 42+05=047 42+05=050 False
3 4 79 39+03=042 39+03=058 False
3 4 80 02+92=094 02+92=108 False
3 4 81 99+81=180 99+81=178 False
3 4 82 32+68=100 32+68=098 False
3 4 83 52+17=069 52+17=078 False
3 4 84 56+58=114 56+58=113 False
3 4 85 21+48=069 21+48=078 False
3 4 86 61+71=132 61+71=128 False
3 4 87 17+01=018 17+01=028 False
3 4 88 68+23=091 68+23=098 False
3 4 89 00+37=037 00+37=038 False
3 4 90 94+88=182 94+88=178 False
3 4 91 06+31=037 06+31=030 False
3 4 92 27+18=045 27+18=058 False
3 4 93 41+81=122 41+81=128 False
3 4 94 15+86=101 15+86=108 False
3 4 95 36+87=123 36+87=128 False
3 4 96 17+37=054 17+37=058 False
3 4 97 13+86=099 13+86=108 False
3 4 98 29+69=098 29+69=098 True
3 4 99 31+99=130 31+99=138 False
3 4 100 47+29=076 47+29=088 False
3 4 101 08+81=089 08+81=098 False
3 4 102 72+82=154 72+82=158 False
3 4 103 46+91=137 46+91=158 False
3 4 104 70+35=105 70+35=108 False
3 4 105 90+55=145 90+55=158 False
3 4 106 99+99=198 99+99=188 False
3 4 107 60+97=157 60+97=168 False
3 4 108 03+40=043 03+40=050 False
3 4 109 35+49=084 35+49=088 False
3 4 110 32+02=034 32+02=030 False
3 4 111 70+18=088 70+18=088 True
3 4 112 99+05=104 99+05=108 False
3 4 113 78+73=151 78+73=158 False
3 4 114 03+02=005 03+02=020 False
3 4 115 50+14=064 50+14=068 False
3 4 116 62+02=064 62+02=078 False
3 4 117 16+74=090 16+74=098 False
3 4 118 68+65=133 68+65=128 False
3 4 119 74+81=155 74+81=158 False
3 4 120 37+48=085 37+48=088 False
3 4 121 63+04=067 63+04=078 False
3 4 122 06+62=068 06+62=078 False
3 4 123 95+75=170 95+75=178 False
3 4 124 92+37=129 92+37=138 False
3 4 125 81+32=113 81+32=113 True
3 4 126 53+28=081 53+28=088 False
3 4 127 52+42=094 52+42=088 False
3 4 128 66+97=163 66+97=178 False
3 4 129 00+48=048 00+48=058 False
3 4 130 65+32=097 65+32=098 False
3 4 131 60+89=149 60+89=158 False
3 4 132 71+61=132 71+61=128 False
3 4 133 98+50=148 98+50=158 False
3 4 134 90+96=186 90+96=178 False
3 4 135 02+96=098 02+96=108 False
3 4 136 62+75=137 62+75=130 False
3 4 137 41+28=069 41+28=078 False
3 4 138 95+79=174 95+79=178 False
3 4 139 48+41=089 48+41=088 False
3 4 140 87+95=182 87+95=188 False
3 4 141 75+38=113 75+38=108 False
3 4 142 31+55=086 31+55=088 False
3 4 143 54+63=117 54+63=112 False
3 4 144 75+82=157 75+82=158 False
3 4 145 46+45=091 46+45=088 False
3 4 146 13+08=021 13+08=030 False
3 4 147 77+97=174 77+97=178 False
3 4 148 37+35=072 37+35=078 False
3 4 149 21+89=110 21+89=113 False
3 4 150 58+51=109 58+51=113 False
3 4 151 91+48=139 91+48=158 False
3 4 152 33+23=056 33+23=050 False
3 4 153 80+96=176 80+96=178 False
3 4 154 78+02=080 78+02=078 False
3 4 155 38+95=133 38+95=148 False
3 4 156 99+25=124 99+25=113 False
3 4 157 30+76=106 30+76=108 False
3 4 158 42+40=082 42+40=088 False
3 4 159 85+58=143 85+58=158 False
3 4 160 44+46=090 44+46=088 False
3 4 161 06+41=047 06+41=050 False
3 4 162 65+90=155 65+90=168 False
3 4 163 43+83=126 43+83=138 False
3 4 164 36+61=097 36+61=098 False
3 4 165 61+51=112 61+51=113 False
3 4 166 38+09=047 38+09=058 False
3 4 167 21+97=118 21+97=113 False
3 4 168 83+30=113 83+30=113 True
3 4 169 11+79=090 11+79=098 False
3 4 170 14+29=043 14+29=050 False
3 4 171 21+11=032 21+11=030 False
3 4 172 43+53=096 43+53=088 False
3 4 173 02+58=060 02+58=058 False
3 4 174 78+82=160 78+82=168 False
3 4 175 91+11=102 91+11=108 False
3 4 176 58+54=112 58+54=113 False
3 4 177 00+15=015 00+15=020 False
3 4 178 83+51=134 83+51=148 False
3 4 179 44+72=116 44+72=113 False
3 4 180 71+20=091 71+20=098 False
3 4 181 24+99=123 24+99=128 False
3 4 182 46+30=076 46+30=078 False
3 4 183 08+67=075 08+67=078 False
3 4 184 47+42=089 47+42=088 False
3 4 185 95+67=162 95+67=178 False
3 4 186 40+56=096 40+56=088 False
3 4 187 17+95=112 17+95=113 False
3 4 188 94+66=160 94+66=178 False
3 4 189 14+58=072 14+58=078 False
3 4 190 56+05=061 56+05=068 False
3 4 191 70+01=071 70+01=078 False
3 4 192 97+59=156 97+59=178 False
3 4 193 94+67=161 94+67=178 False
3 4 194 13+41=054 13+41=050 False
3 4 195 85+15=100 85+15=108 False
3 4 196 48+53=101 48+53=108 False
3 4 197 62+75=137 62+75=130 False
3 4 198 87+47=134 87+47=138 False
3 4 199 31+88=119 31+88=113 False
3 4 200 97+16=113 97+16=108 False
3 4 201 48+45=093 48+45=088 False
3 4 202 99+00=099 99+00=108 False
3 4 203 15+01=016 15+01=020 False
3 4 204 28+96=124 28+96=128 False
3 4 205 20+11=031 20+11=030 False
3 4 206 07+56=063 07+56=058 False
3 4 207 06+08=014 06+08=028 False
3 4 208 45+46=091 45+46=088 False
3 4 209 48+85=133 48+85=138 False
3 4 210 62+14=076 62+14=088 False
3 4 211 82+31=113 82+31=113 True
3 4 212 85+88=173 85+88=178 False
3 4 213 77+08=085 77+08=088 False
3 4 214 16+64=080 16+64=088 False
3 4 215 00+27=027 00+27=030 False
3 4 216 36+75=111 36+75=108 False
3 4 217 38+38=076 38+38=078 False
3 4 218 88+32=120 88+32=113 False
3 4 219 09+88=097 09+88=098 False
3 4 220 96+87=183 96+87=188 False
3 4 221 71+29=100 71+29=098 False
3 4 222 99+13=112 99+13=108 False
3 4 223 03+13=016 03+13=020 False
3 4 224 67+23=090 67+23=098 False
3 4 225 15+98=113 15+98=113 True
3 4 226 10+08=018 10+08=038 False
3 4 227 46+24=070 46+24=078 False
3 4 228 55+63=118 55+63=112 False
3 4 229 28+06=034 28+06=048 False
3 4 230 43+87=130 43+87=138 False
3 4 231 34+05=039 34+05=050 False
2024-12-17 09:44:06,401 - root - WARNING - The method `test_one_epoch` is not implemented. Please override this method in a subclass or use a custom callback.
2024-12-17 09:44:06,403 - root - INFO - ====================================================== Starting Train Epoch: 4/9 ======================================================
2024-12-17 09:44:06,403 - root - INFO - Learning rates for each parameter group: 0.00191741247211842623, 0.00191741247211842623
0%| | 0/28 [00:00<?, ?it/s]
Epoch: 4, Step: 1: 0%| | 0/28 [00:00<?, ?it/s]
Epoch: 4, Step: 1: 0%| | 0/28 [00:00<?, ?it/s, total_batch_loss=230.64146, average_batch_loss=0.90094, average_batch_perplexity=2.46192, lr=0.001917412, 0.001917412]
Epoch: 4, Step: 2: 0%| | 0/28 [00:00<?, ?it/s, total_batch_loss=230.64146, average_batch_loss=0.90094, average_batch_perplexity=2.46192, lr=0.001917412, 0.001917412]
Epoch: 4, Step: 2: 0%| | 0/28 [00:00<?, ?it/s, total_batch_loss=234.89429, average_batch_loss=0.91756, average_batch_perplexity=2.50316, lr=0.001906232, 0.001906232]
Epoch: 4, Step: 2: 7%|▋ | 2/28 [00:00<00:02, 12.52it/s, total_batch_loss=234.89429, average_batch_loss=0.91756, average_batch_perplexity=2.50316, lr=0.001906232, 0.001906232]
Epoch: 4, Step: 3: 7%|▋ | 2/28 [00:00<00:02, 12.52it/s, total_batch_loss=234.89429, average_batch_loss=0.91756, average_batch_perplexity=2.50316, lr=0.001906232, 0.001906232]
Epoch: 4, Step: 3: 7%|▋ | 2/28 [00:00<00:02, 12.52it/s, total_batch_loss=225.58919, average_batch_loss=0.88121, average_batch_perplexity=2.41381, lr=0.001895245, 0.001895245]
Epoch: 4, Step: 4: 7%|▋ | 2/28 [00:00<00:02, 12.52it/s, total_batch_loss=225.58919, average_batch_loss=0.88121, average_batch_perplexity=2.41381, lr=0.001895245, 0.001895245]
Epoch: 4, Step: 4: 7%|▋ | 2/28 [00:00<00:02, 12.52it/s, total_batch_loss=237.27705, average_batch_loss=0.92686, average_batch_perplexity=2.52657, lr=0.001884446, 0.001884446]
Epoch: 4, Step: 4: 14%|█▍ | 4/28 [00:00<00:01, 12.31it/s, total_batch_loss=237.27705, average_batch_loss=0.92686, average_batch_perplexity=2.52657, lr=0.001884446, 0.001884446]
Epoch: 4, Step: 5: 14%|█▍ | 4/28 [00:00<00:01, 12.31it/s, total_batch_loss=237.27705, average_batch_loss=0.92686, average_batch_perplexity=2.52657, lr=0.001884446, 0.001884446]
Epoch: 4, Step: 5: 14%|█▍ | 4/28 [00:00<00:01, 12.31it/s, total_batch_loss=234.00360, average_batch_loss=0.91408, average_batch_perplexity=2.49447, lr=0.001873829, 0.001873829]
Epoch: 4, Step: 6: 14%|█▍ | 4/28 [00:00<00:01, 12.31it/s, total_batch_loss=234.00360, average_batch_loss=0.91408, average_batch_perplexity=2.49447, lr=0.001873829, 0.001873829]
Epoch: 4, Step: 6: 14%|█▍ | 4/28 [00:00<00:01, 12.31it/s, total_batch_loss=227.43008, average_batch_loss=0.88840, average_batch_perplexity=2.43123, lr=0.001863390, 0.001863390]
Epoch: 4, Step: 6: 21%|██▏ | 6/28 [00:00<00:01, 12.30it/s, total_batch_loss=227.43008, average_batch_loss=0.88840, average_batch_perplexity=2.43123, lr=0.001863390, 0.001863390]
Epoch: 4, Step: 7: 21%|██▏ | 6/28 [00:00<00:01, 12.30it/s, total_batch_loss=227.43008, average_batch_loss=0.88840, average_batch_perplexity=2.43123, lr=0.001863390, 0.001863390]
Epoch: 4, Step: 7: 21%|██▏ | 6/28 [00:00<00:01, 12.30it/s, total_batch_loss=228.49860, average_batch_loss=0.89257, average_batch_perplexity=2.44140, lr=0.001853123, 0.001853123]
Epoch: 4, Step: 8: 21%|██▏ | 6/28 [00:00<00:01, 12.30it/s, total_batch_loss=228.49860, average_batch_loss=0.89257, average_batch_perplexity=2.44140, lr=0.001853123, 0.001853123]
Epoch: 4, Step: 8: 21%|██▏ | 6/28 [00:00<00:01, 12.30it/s, total_batch_loss=229.30400, average_batch_loss=0.89572, average_batch_perplexity=2.44910, lr=0.001843024, 0.001843024]
Epoch: 4, Step: 8: 29%|██▊ | 8/28 [00:00<00:01, 12.45it/s, total_batch_loss=229.30400, average_batch_loss=0.89572, average_batch_perplexity=2.44910, lr=0.001843024, 0.001843024]
Epoch: 4, Step: 9: 29%|██▊ | 8/28 [00:00<00:01, 12.45it/s, total_batch_loss=229.30400, average_batch_loss=0.89572, average_batch_perplexity=2.44910, lr=0.001843024, 0.001843024]
Epoch: 4, Step: 9: 29%|██▊ | 8/28 [00:00<00:01, 12.45it/s, total_batch_loss=218.08556, average_batch_loss=0.85190, average_batch_perplexity=2.34409, lr=0.001833089, 0.001833089]
Epoch: 4, Step: 10: 29%|██▊ | 8/28 [00:00<00:01, 12.45it/s, total_batch_loss=218.08556, average_batch_loss=0.85190, average_batch_perplexity=2.34409, lr=0.001833089, 0.001833089]
Epoch: 4, Step: 10: 29%|██▊ | 8/28 [00:00<00:01, 12.45it/s, total_batch_loss=221.96524, average_batch_loss=0.86705, average_batch_perplexity=2.37988, lr=0.001823312, 0.001823312]
Epoch: 4, Step: 10: 36%|███▌ | 10/28 [00:00<00:01, 12.53it/s, total_batch_loss=221.96524, average_batch_loss=0.86705, average_batch_perplexity=2.37988, lr=0.001823312, 0.001823312]
Epoch: 4, Step: 11: 36%|███▌ | 10/28 [00:00<00:01, 12.53it/s, total_batch_loss=221.96524, average_batch_loss=0.86705, average_batch_perplexity=2.37988, lr=0.001823312, 0.001823312]
Epoch: 4, Step: 11: 36%|███▌ | 10/28 [00:00<00:01, 12.53it/s, total_batch_loss=219.22734, average_batch_loss=0.85636, average_batch_perplexity=2.35457, lr=0.001813691, 0.001813691]
Epoch: 4, Step: 12: 36%|███▌ | 10/28 [00:00<00:01, 12.53it/s, total_batch_loss=219.22734, average_batch_loss=0.85636, average_batch_perplexity=2.35457, lr=0.001813691, 0.001813691]
Epoch: 4, Step: 12: 36%|███▌ | 10/28 [00:00<00:01, 12.53it/s, total_batch_loss=220.11047, average_batch_loss=0.85981, average_batch_perplexity=2.36270, lr=0.001804220, 0.001804220]
Epoch: 4, Step: 12: 43%|████▎ | 12/28 [00:00<00:01, 12.65it/s, total_batch_loss=220.11047, average_batch_loss=0.85981, average_batch_perplexity=2.36270, lr=0.001804220, 0.001804220]
Epoch: 4, Step: 13: 43%|████▎ | 12/28 [00:01<00:01, 12.65it/s, total_batch_loss=220.11047, average_batch_loss=0.85981, average_batch_perplexity=2.36270, lr=0.001804220, 0.001804220]
Epoch: 4, Step: 13: 43%|████▎ | 12/28 [00:01<00:01, 12.65it/s, total_batch_loss=214.46967, average_batch_loss=0.83777, average_batch_perplexity=2.31121, lr=0.001794895, 0.001794895]
Epoch: 4, Step: 14: 43%|████▎ | 12/28 [00:01<00:01, 12.65it/s, total_batch_loss=214.46967, average_batch_loss=0.83777, average_batch_perplexity=2.31121, lr=0.001794895, 0.001794895]
Epoch: 4, Step: 14: 43%|████▎ | 12/28 [00:01<00:01, 12.65it/s, total_batch_loss=219.96057, average_batch_loss=0.85922, average_batch_perplexity=2.36132, lr=0.001785714, 0.001785714]
Epoch: 4, Step: 14: 50%|█████ | 14/28 [00:01<00:01, 12.52it/s, total_batch_loss=219.96057, average_batch_loss=0.85922, average_batch_perplexity=2.36132, lr=0.001785714, 0.001785714]
Epoch: 4, Step: 15: 50%|█████ | 14/28 [00:01<00:01, 12.52it/s, total_batch_loss=219.96057, average_batch_loss=0.85922, average_batch_perplexity=2.36132, lr=0.001785714, 0.001785714]
Epoch: 4, Step: 15: 50%|█████ | 14/28 [00:01<00:01, 12.52it/s, total_batch_loss=218.02727, average_batch_loss=0.85167, average_batch_perplexity=2.34355, lr=0.001776673, 0.001776673]
2024-12-17 09:44:07,683 - root - INFO - Epoch: 4, Step: 100, Avg Batch Loss: 0.84106, Avg Batch Perplexity: 2.31884, LR: 0.001767767
Epoch: 4, Step: 16: 50%|█████ | 14/28 [00:01<00:01, 12.52it/s, total_batch_loss=218.02727, average_batch_loss=0.85167, average_batch_perplexity=2.34355, lr=0.001776673, 0.001776673]
Epoch: 4, Step: 16: 50%|█████ | 14/28 [00:01<00:01, 12.52it/s, total_batch_loss=215.31261, average_batch_loss=0.84106, average_batch_perplexity=2.31884, lr=0.001767767, 0.001767767]
Epoch: 4, Step: 16: 57%|█████▋ | 16/28 [00:01<00:00, 12.54it/s, total_batch_loss=215.31261, average_batch_loss=0.84106, average_batch_perplexity=2.31884, lr=0.001767767, 0.001767767]
Epoch: 4, Step: 17: 57%|█████▋ | 16/28 [00:01<00:00, 12.54it/s, total_batch_loss=215.31261, average_batch_loss=0.84106, average_batch_perplexity=2.31884, lr=0.001767767, 0.001767767]
Epoch: 4, Step: 17: 57%|█████▋ | 16/28 [00:01<00:00, 12.54it/s, total_batch_loss=226.95306, average_batch_loss=0.88654, average_batch_perplexity=2.42671, lr=0.001758994, 0.001758994]
Epoch: 4, Step: 18: 57%|█████▋ | 16/28 [00:01<00:00, 12.54it/s, total_batch_loss=226.95306, average_batch_loss=0.88654, average_batch_perplexity=2.42671, lr=0.001758994, 0.001758994]
Epoch: 4, Step: 18: 57%|█████▋ | 16/28 [00:01<00:00, 12.54it/s, total_batch_loss=223.14464, average_batch_loss=0.87166, average_batch_perplexity=2.39087, lr=0.001750350, 0.001750350]
Epoch: 4, Step: 18: 64%|██████▍ | 18/28 [00:01<00:00, 12.45it/s, total_batch_loss=223.14464, average_batch_loss=0.87166, average_batch_perplexity=2.39087, lr=0.001750350, 0.001750350]
Epoch: 4, Step: 19: 64%|██████▍ | 18/28 [00:01<00:00, 12.45it/s, total_batch_loss=223.14464, average_batch_loss=0.87166, average_batch_perplexity=2.39087, lr=0.001750350, 0.001750350]
Epoch: 4, Step: 19: 64%|██████▍ | 18/28 [00:01<00:00, 12.45it/s, total_batch_loss=223.86075, average_batch_loss=0.87446, average_batch_perplexity=2.39757, lr=0.001741833, 0.001741833]
Epoch: 4, Step: 20: 64%|██████▍ | 18/28 [00:01<00:00, 12.45it/s, total_batch_loss=223.86075, average_batch_loss=0.87446, average_batch_perplexity=2.39757, lr=0.001741833, 0.001741833]
Epoch: 4, Step: 20: 64%|██████▍ | 18/28 [00:01<00:00, 12.45it/s, total_batch_loss=220.87898, average_batch_loss=0.86281, average_batch_perplexity=2.36981, lr=0.001733438, 0.001733438]
Epoch: 4, Step: 20: 71%|███████▏ | 20/28 [00:01<00:00, 12.50it/s, total_batch_loss=220.87898, average_batch_loss=0.86281, average_batch_perplexity=2.36981, lr=0.001733438, 0.001733438]
Epoch: 4, Step: 21: 71%|███████▏ | 20/28 [00:01<00:00, 12.50it/s, total_batch_loss=220.87898, average_batch_loss=0.86281, average_batch_perplexity=2.36981, lr=0.001733438, 0.001733438]
Epoch: 4, Step: 21: 71%|███████▏ | 20/28 [00:01<00:00, 12.50it/s, total_batch_loss=212.23076, average_batch_loss=0.82903, average_batch_perplexity=2.29109, lr=0.001725164, 0.001725164]
Epoch: 4, Step: 22: 71%|███████▏ | 20/28 [00:01<00:00, 12.50it/s, total_batch_loss=212.23076, average_batch_loss=0.82903, average_batch_perplexity=2.29109, lr=0.001725164, 0.001725164]
Epoch: 4, Step: 22: 71%|███████▏ | 20/28 [00:01<00:00, 12.50it/s, total_batch_loss=208.13551, average_batch_loss=0.81303, average_batch_perplexity=2.25473, lr=0.001717007, 0.001717007]
Epoch: 4, Step: 22: 79%|███████▊ | 22/28 [00:01<00:00, 12.52it/s, total_batch_loss=208.13551, average_batch_loss=0.81303, average_batch_perplexity=2.25473, lr=0.001717007, 0.001717007]
Epoch: 4, Step: 23: 79%|███████▊ | 22/28 [00:01<00:00, 12.52it/s, total_batch_loss=208.13551, average_batch_loss=0.81303, average_batch_perplexity=2.25473, lr=0.001717007, 0.001717007]
Epoch: 4, Step: 23: 79%|███████▊ | 22/28 [00:01<00:00, 12.52it/s, total_batch_loss=213.27568, average_batch_loss=0.83311, average_batch_perplexity=2.30046, lr=0.001708965, 0.001708965]
Epoch: 4, Step: 24: 79%|███████▊ | 22/28 [00:01<00:00, 12.52it/s, total_batch_loss=213.27568, average_batch_loss=0.83311, average_batch_perplexity=2.30046, lr=0.001708965, 0.001708965]
Epoch: 4, Step: 24: 79%|███████▊ | 22/28 [00:01<00:00, 12.52it/s, total_batch_loss=213.01154, average_batch_loss=0.83208, average_batch_perplexity=2.29809, lr=0.001701035, 0.001701035]
Epoch: 4, Step: 24: 86%|████████▌ | 24/28 [00:01<00:00, 12.65it/s, total_batch_loss=213.01154, average_batch_loss=0.83208, average_batch_perplexity=2.29809, lr=0.001701035, 0.001701035]
Epoch: 4, Step: 25: 86%|████████▌ | 24/28 [00:01<00:00, 12.65it/s, total_batch_loss=213.01154, average_batch_loss=0.83208, average_batch_perplexity=2.29809, lr=0.001701035, 0.001701035]
Epoch: 4, Step: 25: 86%|████████▌ | 24/28 [00:01<00:00, 12.65it/s, total_batch_loss=207.95612, average_batch_loss=0.81233, average_batch_perplexity=2.25315, lr=0.001693214, 0.001693214]
Epoch: 4, Step: 26: 86%|████████▌ | 24/28 [00:02<00:00, 12.65it/s, total_batch_loss=207.95612, average_batch_loss=0.81233, average_batch_perplexity=2.25315, lr=0.001693214, 0.001693214]
Epoch: 4, Step: 26: 86%|████████▌ | 24/28 [00:02<00:00, 12.65it/s, total_batch_loss=206.17104, average_batch_loss=0.80536, average_batch_perplexity=2.23749, lr=0.001685500, 0.001685500]
Epoch: 4, Step: 26: 93%|█████████▎| 26/28 [00:02<00:00, 12.63it/s, total_batch_loss=206.17104, average_batch_loss=0.80536, average_batch_perplexity=2.23749, lr=0.001685500, 0.001685500]
Epoch: 4, Step: 27: 93%|█████████▎| 26/28 [00:02<00:00, 12.63it/s, total_batch_loss=206.17104, average_batch_loss=0.80536, average_batch_perplexity=2.23749, lr=0.001685500, 0.001685500]
Epoch: 4, Step: 27: 93%|█████████▎| 26/28 [00:02<00:00, 12.63it/s, total_batch_loss=206.14790, average_batch_loss=0.80527, average_batch_perplexity=2.23729, lr=0.001677890, 0.001677890]
Epoch: 4, Step: 28: 93%|█████████▎| 26/28 [00:02<00:00, 12.63it/s, total_batch_loss=206.14790, average_batch_loss=0.80527, average_batch_perplexity=2.23729, lr=0.001677890, 0.001677890]
Epoch: 4, Step: 28: 93%|█████████▎| 26/28 [00:02<00:00, 12.63it/s, total_batch_loss=70.41332, average_batch_loss=0.80015, average_batch_perplexity=2.22588, lr=0.001670383, 0.001670383]
Epoch: 4, Step: 28: 100%|██████████| 28/28 [00:02<00:00, 13.90it/s, total_batch_loss=70.41332, average_batch_loss=0.80015, average_batch_perplexity=2.22588, lr=0.001670383, 0.001670383]
2024-12-17 09:44:08,591 - root - INFO - Total Samples: 7000
2024-12-17 09:44:08,592 - root - INFO - Total Batches: 28
2024-12-17 09:44:08,593 - root - INFO - Average Epoch Train Loss: 0.86100
2024-12-17 09:44:08,594 - root - INFO - Average Epoch Train Perplexity: 2.36552
2024-12-17 09:44:08,594 - root - INFO -
2024-12-17 09:44:08,595 - root - INFO - ====================================================== Starting Valid Epoch: 4/9 ======================================================
0%| | 0/8 [00:00<?, ?it/s]
Epoch: 4, Step: 1: 0%| | 0/8 [00:00<?, ?it/s]
Epoch: 4, Step: 1: 0%| | 0/8 [00:00<?, ?it/s, total_batch_loss=199.28583, average_batch_loss=0.77846, average_batch_perplexity=2.17812]
Epoch: 4, Step: 2: 0%| | 0/8 [00:00<?, ?it/s, total_batch_loss=199.28583, average_batch_loss=0.77846, average_batch_perplexity=2.17812]
Epoch: 4, Step: 2: 0%| | 0/8 [00:00<?, ?it/s, total_batch_loss=200.44511, average_batch_loss=0.78299, average_batch_perplexity=2.18800]
Epoch: 4, Step: 3: 0%| | 0/8 [00:00<?, ?it/s, total_batch_loss=200.44511, average_batch_loss=0.78299, average_batch_perplexity=2.18800]
Epoch: 4, Step: 3: 0%| | 0/8 [00:00<?, ?it/s, total_batch_loss=200.06065, average_batch_loss=0.78149, average_batch_perplexity=2.18472]
Epoch: 4, Step: 3: 38%|███▊ | 3/8 [00:00<00:00, 22.52it/s, total_batch_loss=200.06065, average_batch_loss=0.78149, average_batch_perplexity=2.18472]
Epoch: 4, Step: 4: 38%|███▊ | 3/8 [00:00<00:00, 22.52it/s, total_batch_loss=200.06065, average_batch_loss=0.78149, average_batch_perplexity=2.18472]
Epoch: 4, Step: 4: 38%|███▊ | 3/8 [00:00<00:00, 22.52it/s, total_batch_loss=198.88388, average_batch_loss=0.77689, average_batch_perplexity=2.17470]
Epoch: 4, Step: 5: 38%|███▊ | 3/8 [00:00<00:00, 22.52it/s, total_batch_loss=198.88388, average_batch_loss=0.77689, average_batch_perplexity=2.17470]
Epoch: 4, Step: 5: 38%|███▊ | 3/8 [00:00<00:00, 22.52it/s, total_batch_loss=200.88089, average_batch_loss=0.78469, average_batch_perplexity=2.19173]
Epoch: 4, Step: 6: 38%|███▊ | 3/8 [00:00<00:00, 22.52it/s, total_batch_loss=200.88089, average_batch_loss=0.78469, average_batch_perplexity=2.19173]
Epoch: 4, Step: 6: 38%|███▊ | 3/8 [00:00<00:00, 22.52it/s, total_batch_loss=199.88879, average_batch_loss=0.78082, average_batch_perplexity=2.18325]
Epoch: 4, Step: 6: 75%|███████▌ | 6/8 [00:00<00:00, 21.19it/s, total_batch_loss=199.88879, average_batch_loss=0.78082, average_batch_perplexity=2.18325]
Epoch: 4, Step: 7: 75%|███████▌ | 6/8 [00:00<00:00, 21.19it/s, total_batch_loss=199.88879, average_batch_loss=0.78082, average_batch_perplexity=2.18325]
Epoch: 4, Step: 7: 75%|███████▌ | 6/8 [00:00<00:00, 21.19it/s, total_batch_loss=197.75778, average_batch_loss=0.77249, average_batch_perplexity=2.16515]
Epoch: 4, Step: 8: 75%|███████▌ | 6/8 [00:00<00:00, 21.19it/s, total_batch_loss=197.75778, average_batch_loss=0.77249, average_batch_perplexity=2.16515]
Epoch: 4, Step: 8: 75%|███████▌ | 6/8 [00:00<00:00, 21.19it/s, total_batch_loss=162.22050, average_batch_loss=0.77991, average_batch_perplexity=2.18127]
2024-12-17 09:44:08,955 - root - INFO - Total Samples: 2000
2024-12-17 09:44:08,956 - root - INFO - Total Batches: 8
2024-12-17 09:44:08,956 - root - INFO - Average Epoch Valid Loss: 0.77971
2024-12-17 09:44:08,957 - root - INFO - Average Epoch Valid Perplexity: 2.18084
2024-12-17 09:44:08,957 - root - INFO -
Evaluating and Generation.: 0%| | 0/4 [00:00<?, ?it/s]
Evaluating and Generation.: 0%| | 0/4 [00:00<?, ?it/s, accuracy: 0.0703]
Evaluating and Generation.: 0%| | 0/4 [00:00<?, ?it/s, accuracy: 0.0898]
Evaluating and Generation.: 50%|█████ | 2/4 [00:00<00:00, 10.63it/s, accuracy: 0.0898]
Evaluating and Generation.: 50%|█████ | 2/4 [00:00<00:00, 10.63it/s, accuracy: 0.0938]
Evaluating and Generation.: 50%|█████ | 2/4 [00:00<00:00, 10.63it/s, accuracy: 0.0690]
Evaluating and Generation.: 100%|██████████| 4/4 [00:00<00:00, 10.95it/s, accuracy: 0.0690]
2024-12-17 09:44:09,331 - root - INFO - Correct/Total Samples: 81/1000
2024-12-17 09:44:09,331 - root - INFO - Eval Accuracy: 0.081
2024-12-17 09:44:09,349 - root - INFO -
epoch batch_index sample_index equation generated correct
4 1 0 13+48=061 13+48=068 False
4 1 1 16+55=071 16+55=064 False
4 1 2 79+34=113 79+34=110 False
4 1 3 35+44=079 35+44=078 False
4 1 4 16+50=066 16+50=064 False
4 1 5 28+47=075 28+47=078 False
4 1 6 00+74=074 00+74=070 False
4 1 7 15+20=035 15+20=030 False
4 1 8 72+60=132 72+60=130 False
4 1 9 63+68=131 63+68=120 False
4 1 10 29+45=074 29+45=078 False
4 1 11 34+60=094 34+60=098 False
4 1 12 53+70=123 53+70=120 False
4 1 13 70+50=120 70+50=110 False
4 1 14 11+84=095 11+84=098 False
4 1 15 42+71=113 42+71=110 False
4 1 16 98+22=120 98+22=128 False
4 1 17 02+02=004 02+02=010 False
4 1 18 15+85=100 15+85=108 False
4 1 19 21+78=099 21+78=108 False
4 1 20 61+79=140 61+79=130 False
4 1 21 25+99=124 25+99=118 False
4 1 22 09+85=094 09+85=098 False
4 1 23 60+91=151 60+91=140 False
4 1 24 35+30=065 35+30=060 False
4 1 25 24+51=075 24+51=070 False
4 1 26 93+91=184 93+91=170 False
4 1 27 39+96=135 39+96=128 False
4 1 28 64+35=099 64+35=108 False
4 1 29 36+22=058 36+22=054 False
4 1 30 68+45=113 68+45=110 False
4 1 31 16+84=100 16+84=108 False
4 1 32 91+52=143 91+52=130 False
4 1 33 97+36=133 97+36=138 False
4 1 34 27+37=064 27+37=068 False
4 1 35 99+82=181 99+82=188 False
4 1 36 03+42=045 03+42=050 False
4 1 37 18+38=056 18+38=058 False
4 1 38 32+20=052 32+20=050 False
4 1 39 38+13=051 38+13=050 False
4 1 40 68+42=110 68+42=110 True
4 1 41 64+00=064 64+00=060 False
4 1 42 48+94=142 48+94=148 False
4 1 43 58+36=094 58+36=088 False
4 1 44 41+22=063 41+22=064 False
4 1 45 23+58=081 23+58=078 False
4 1 46 67+46=113 67+46=113 True
4 1 47 40+78=118 40+78=110 False
4 1 48 90+38=128 90+38=128 True
4 1 49 89+52=141 89+52=138 False
4 1 50 37+77=114 37+77=114 True
4 1 51 29+76=105 29+76=108 False
4 1 52 42+90=132 42+90=130 False
4 1 53 45+82=127 45+82=120 False
4 1 54 35+95=130 35+95=128 False
4 1 55 92+98=190 92+98=188 False
4 1 56 73+91=164 73+91=160 False
4 1 57 53+97=150 53+97=148 False
4 1 58 98+69=167 98+69=178 False
4 1 59 20+46=066 20+46=064 False
4 1 60 48+69=117 48+69=114 False
4 1 61 62+31=093 62+31=090 False
4 1 62 80+59=139 80+59=138 False
4 1 63 58+12=070 58+12=078 False
4 1 64 08+96=104 08+96=108 False
4 1 65 67+06=073 67+06=075 False
4 1 66 22+04=026 22+04=030 False
4 1 67 61+87=148 61+87=140 False
4 1 68 95+27=122 95+27=113 False
4 1 69 49+83=132 49+83=128 False
4 1 70 43+00=043 43+00=040 False
4 1 71 01+85=086 01+85=088 False
4 1 72 11+68=079 11+68=088 False
4 1 73 80+03=083 80+03=088 False
4 1 74 54+83=137 54+83=130 False
4 1 75 73+47=120 73+47=110 False
4 1 76 99+93=192 99+93=188 False
4 1 77 99+13=112 99+13=113 False
4 1 78 92+66=158 92+66=164 False
4 1 79 90+31=121 90+31=128 False
4 1 80 25+69=094 25+69=098 False
4 1 81 25+44=069 25+44=060 False
4 1 82 00+93=093 00+93=098 False
4 1 83 88+87=175 88+87=175 True
4 1 84 47+56=103 47+56=108 False
4 1 85 43+59=102 43+59=108 False
4 1 86 22+00=022 22+00=020 False
4 1 87 34+04=038 34+04=040 False
4 1 88 65+13=078 65+13=070 False
4 1 89 39+82=121 39+82=113 False
4 1 90 66+83=149 66+83=144 False
4 1 91 51+69=120 51+69=110 False
4 1 92 80+21=101 80+21=108 False
4 1 93 36+79=115 36+79=113 False
4 1 94 21+68=089 21+68=088 False
4 1 95 11+66=077 11+66=074 False
4 1 96 55+19=074 55+19=078 False
4 1 97 51+61=112 51+61=110 False
4 1 98 38+88=126 38+88=114 False
4 1 99 37+27=064 37+27=068 False
4 1 100 18+63=081 18+63=088 False
4 1 101 48+11=059 48+11=050 False
4 1 102 72+68=140 72+68=138 False
4 1 103 37+39=076 37+39=078 False
4 1 104 64+95=159 64+95=150 False
4 1 105 49+75=124 49+75=128 False
4 1 106 45+66=111 45+66=110 False
4 1 107 34+87=121 34+87=110 False
4 1 108 02+84=086 02+84=088 False
4 1 109 95+00=095 95+00=098 False
4 1 110 09+56=065 09+56=068 False
4 1 111 22+66=088 22+66=088 True
4 1 112 43+18=061 43+18=068 False
4 1 113 61+35=096 61+35=098 False
4 1 114 13+73=086 13+73=080 False
4 1 115 25+95=120 25+95=110 False
4 1 116 73+96=169 73+96=164 False
4 1 117 03+96=099 03+96=098 False
4 1 118 97+82=179 97+82=178 False
4 1 119 18+42=060 18+42=058 False
4 1 120 29+98=127 29+98=128 False
4 1 121 61+00=061 61+00=060 False
4 1 122 22+98=120 22+98=113 False
4 1 123 12+50=062 12+50=064 False
4 1 124 02+58=060 02+58=068 False
4 1 125 75+86=161 75+86=154 False
4 1 126 31+57=088 31+57=088 True
4 1 127 49+82=131 49+82=128 False
4 1 128 15+33=048 15+33=040 False
4 1 129 49+57=106 49+57=108 False
4 1 130 61+70=131 61+70=120 False
4 1 131 91+51=142 91+51=130 False
4 1 132 50+05=055 50+05=060 False
4 1 133 44+16=060 44+16=050 False
4 1 134 92+01=093 92+01=098 False
4 1 135 85+82=167 85+82=164 False
4 1 136 07+41=048 07+41=050 False
4 1 137 40+06=046 40+06=054 False
4 1 138 79+62=141 79+62=138 False
4 1 139 95+62=157 95+62=150 False
4 1 140 42+93=135 42+93=130 False
4 1 141 32+73=105 32+73=108 False
4 1 142 47+09=056 47+09=058 False
4 1 143 59+50=109 59+50=108 False
4 1 144 61+77=138 61+77=130 False
4 1 145 64+06=070 64+06=078 False
4 1 146 35+10=045 35+10=040 False
4 1 147 32+88=120 32+88=113 False
4 1 148 03+95=098 03+95=098 True
4 1 149 11+38=049 11+38=040 False
4 1 150 21+67=088 21+67=088 True
4 1 151 33+25=058 33+25=050 False
4 1 152 63+45=108 63+45=110 False
4 1 153 56+12=068 56+12=064 False
4 1 154 19+79=098 19+79=098 True
4 1 155 60+43=103 60+43=108 False
4 1 156 07+61=068 07+61=078 False
4 1 157 58+03=061 58+03=068 False
4 1 158 11+10=021 11+10=020 False
4 1 159 49+89=138 49+89=138 True
4 1 160 37+58=095 37+58=088 False
4 1 161 59+78=137 59+78=138 False
4 1 162 11+21=032 11+21=030 False
4 1 163 37+43=080 37+43=078 False
4 1 164 44+21=065 44+21=060 False
4 1 165 22+97=119 22+97=113 False
4 1 166 65+35=100 65+35=108 False
4 1 167 06+51=057 06+51=064 False
4 1 168 65+25=090 65+25=088 False
4 1 169 74+94=168 74+94=160 False
4 1 170 87+55=142 87+55=148 False
4 1 171 90+67=157 90+67=164 False
4 1 172 11+02=013 11+02=020 False
4 1 173 01+66=067 01+66=074 False
4 1 174 56+00=056 56+00=064 False
4 1 175 58+52=110 58+52=110 True
4 1 176 24+99=123 24+99=118 False
4 1 177 97+13=110 97+13=113 False
4 1 178 42+94=136 42+94=130 False
4 1 179 60+15=075 60+15=070 False
4 1 180 20+46=066 20+46=064 False
4 1 181 40+70=110 40+70=110 True
4 1 182 95+45=140 95+45=140 True
4 1 183 96+95=191 96+95=184 False
4 1 184 98+20=118 98+20=114 False
4 1 185 43+19=062 43+19=060 False
4 1 186 50+69=119 50+69=110 False
4 1 187 27+53=080 27+53=078 False
4 1 188 24+25=049 24+25=040 False
4 1 189 65+92=157 65+92=150 False
4 1 190 28+14=042 28+14=040 False
4 1 191 20+57=077 20+57=078 False
4 1 192 59+97=156 59+97=158 False
4 1 193 98+32=130 98+32=128 False
4 1 194 55+84=139 55+84=130 False
4 1 195 20+39=059 20+39=068 False
4 1 196 86+47=133 86+47=138 False
4 1 197 92+36=128 92+36=128 True
4 1 198 05+38=043 05+38=040 False
4 1 199 77+36=113 77+36=114 False
4 1 200 41+64=105 41+64=108 False
4 1 201 74+51=125 74+51=120 False
4 1 202 74+55=129 74+55=120 False
4 1 203 64+64=128 64+64=120 False
4 1 204 60+19=079 60+19=088 False
4 1 205 77+96=173 77+96=174 False
4 1 206 22+30=052 22+30=050 False
4 1 207 82+49=131 82+49=128 False
4 1 208 39+67=106 39+67=108 False
4 1 209 62+40=102 62+40=108 False
4 1 210 28+71=099 28+71=098 False
4 1 211 47+26=073 47+26=065 False
4 1 212 98+54=152 98+54=148 False
4 1 213 38+70=108 38+70=108 True
4 1 214 63+40=103 63+40=108 False
4 1 215 86+62=148 86+62=144 False
4 1 216 22+65=087 22+65=088 False
4 1 217 41+17=058 41+17=050 False
4 1 218 68+88=156 68+88=155 False
4 1 219 96+70=166 96+70=164 False
4 1 220 99+29=128 99+29=128 True
4 1 221 83+39=122 83+39=110 False
4 1 222 26+55=081 26+55=078 False
4 1 223 53+70=123 53+70=120 False
4 1 224 94+12=106 94+12=108 False
4 1 225 00+37=037 00+37=040 False
4 1 226 36+94=130 36+94=128 False
4 1 227 40+58=098 40+58=108 False
4 1 228 19+80=099 19+80=098 False
4 1 229 49+44=093 49+44=108 False
4 1 230 70+27=097 70+27=098 False
4 1 231 52+80=132 52+80=130 False
4 1 232 77+90=167 77+90=164 False
4 1 233 13+92=105 13+92=108 False
4 1 234 59+09=068 59+09=078 False
4 1 235 33+55=088 33+55=088 True
4 1 236 85+16=101 85+16=108 False
4 1 237 25+65=090 25+65=088 False
4 1 238 46+20=066 46+20=064 False
4 1 239 29+52=081 29+52=078 False
4 1 240 32+36=068 32+36=064 False
4 1 241 47+08=055 47+08=058 False
4 1 242 21+84=105 21+84=108 False
4 1 243 24+45=069 24+45=060 False
4 1 244 29+15=044 29+15=040 False
4 1 245 83+03=086 83+03=088 False
4 1 246 83+36=119 83+36=113 False
4 1 247 58+95=153 58+95=148 False
4 1 248 76+79=155 76+79=144 False
4 1 249 63+30=093 63+30=090 False
4 1 250 38+24=062 38+24=068 False
4 1 251 19+46=065 19+46=068 False
4 1 252 99+66=165 99+66=164 False
4 1 253 95+73=168 95+73=164 False
4 1 254 65+27=092 65+27=088 False
4 1 255 91+83=174 91+83=170 False
4 2 0 65+49=114 65+49=110 False
4 2 1 03+08=011 03+08=010 False
4 2 2 67+81=148 67+81=140 False
4 2 3 47+23=070 47+23=068 False
4 2 4 43+91=134 43+91=130 False
4 2 5 41+67=108 41+67=110 False
4 2 6 02+33=035 02+33=040 False
4 2 7 64+84=148 64+84=140 False
4 2 8 81+64=145 81+64=140 False
4 2 9 80+11=091 80+11=098 False
4 2 10 78+01=079 78+01=078 False
4 2 11 89+18=107 89+18=108 False
4 2 12 45+52=097 45+52=088 False
4 2 13 35+30=065 35+30=060 False
4 2 14 53+32=085 53+32=088 False
4 2 15 49+90=139 49+90=148 False
4 2 16 41+37=078 41+37=078 True
4 2 17 35+14=049 35+14=040 False
4 2 18 92+50=142 92+50=140 False
4 2 19 37+60=097 37+60=098 False
4 2 20 91+61=152 91+61=140 False
4 2 21 80+77=157 80+77=154 False
4 2 22 66+24=090 66+24=088 False
4 2 23 81+07=088 81+07=088 True
4 2 24 85+59=144 85+59=148 False
4 2 25 19+69=088 19+69=088 True
4 2 26 91+44=135 91+44=130 False
4 2 27 25+29=054 25+29=058 False
4 2 28 27+08=035 27+08=038 False
4 2 29 66+14=080 66+14=088 False
4 2 30 95+11=106 95+11=108 False
4 2 31 13+97=110 13+97=108 False
4 2 32 94+40=134 94+40=130 False
4 2 33 74+31=105 74+31=108 False
4 2 34 49+00=049 49+00=058 False
4 2 35 59+18=077 59+18=078 False
4 2 36 07+65=072 07+65=078 False
4 2 37 83+55=138 83+55=130 False
4 2 38 49+80=129 49+80=128 False
4 2 39 64+17=081 64+17=088 False
4 2 40 48+83=131 48+83=128 False
4 2 41 95+44=139 95+44=140 False
4 2 42 71+26=097 71+26=098 False
4 2 43 06+74=080 06+74=078 False
4 2 44 34+24=058 34+24=050 False
4 2 45 59+71=130 59+71=128 False
4 2 46 68+32=100 68+32=108 False
4 2 47 38+81=119 38+81=113 False
4 2 48 29+56=085 29+56=088 False
4 2 49 54+55=109 54+55=110 False
4 2 50 31+27=058 31+27=050 False
4 2 51 97+89=186 97+89=188 False
4 2 52 48+09=057 48+09=068 False
4 2 53 86+76=162 86+76=154 False
4 2 54 82+59=141 82+59=138 False
4 2 55 01+67=068 01+67=078 False
4 2 56 26+06=032 26+06=034 False
4 2 57 22+46=068 22+46=064 False
4 2 58 85+16=101 85+16=108 False
4 2 59 29+08=037 29+08=038 False
4 2 60 73+94=167 73+94=160 False
4 2 61 19+62=081 19+62=088 False
4 2 62 86+62=148 86+62=144 False
4 2 63 38+99=137 38+99=128 False
4 2 64 64+25=089 64+25=088 False
4 2 65 61+72=133 61+72=120 False
4 2 66 78+88=166 78+88=165 False
4 2 67 43+66=109 43+66=110 False
4 2 68 69+35=104 69+35=108 False
4 2 69 33+77=110 33+77=110 True
4 2 70 37+37=074 37+37=078 False
4 2 71 87+54=141 87+54=138 False
4 2 72 68+90=158 68+90=164 False
4 2 73 83+44=127 83+44=120 False
4 2 74 41+09=050 41+09=050 True
4 2 75 13+48=061 13+48=068 False
4 2 76 01+41=042 01+41=040 False
4 2 77 19+74=093 19+74=098 False
4 2 78 15+05=020 15+05=020 True
4 2 79 55+46=101 55+46=109 False
4 2 80 68+33=101 68+33=108 False
4 2 81 44+40=084 44+40=088 False
4 2 82 88+03=091 88+03=088 False
4 2 83 81+79=160 81+79=158 False
4 2 84 18+98=116 18+98=108 False
4 2 85 70+64=134 70+64=130 False
4 2 86 26+44=070 26+44=068 False
4 2 87 98+87=185 98+87=185 True
4 2 88 18+74=092 18+74=098 False
4 2 89 50+68=118 50+68=110 False
4 2 90 13+51=064 13+51=060 False
4 2 91 90+89=179 90+89=178 False
4 2 92 47+78=125 47+78=128 False
4 2 93 81+57=138 81+57=138 True
4 2 94 34+47=081 34+47=078 False
4 2 95 94+23=117 94+23=110 False
4 2 96 07+70=077 07+70=078 False
4 2 97 56+33=089 56+33=088 False
4 2 98 33+04=037 33+04=040 False
4 2 99 26+09=035 26+09=038 False
4 2 100 14+92=106 14+92=108 False
4 2 101 78+54=132 78+54=138 False
4 2 102 36+76=112 36+76=113 False
4 2 103 17+47=064 17+47=064 True
4 2 104 28+18=046 28+18=048 False
4 2 105 78+54=132 78+54=138 False
4 2 106 84+72=156 84+72=140 False
4 2 107 00+44=044 00+44=050 False
4 2 108 50+41=091 50+41=080 False
4 2 109 87+88=175 87+88=175 True
4 2 110 11+66=077 11+66=074 False
4 2 111 80+60=140 80+60=140 True
4 2 112 78+76=154 78+76=144 False
4 2 113 24+74=098 24+74=098 True
4 2 114 88+48=136 88+48=138 False
4 2 115 38+31=069 38+31=064 False
4 2 116 29+27=056 29+27=058 False
4 2 117 08+45=053 08+45=050 False
4 2 118 28+13=041 28+13=040 False
4 2 119 53+99=152 53+99=148 False
4 2 120 47+92=139 47+92=138 False
4 2 121 76+21=097 76+21=098 False
4 2 122 53+96=149 53+96=140 False
4 2 123 93+91=184 93+91=170 False
4 2 124 97+33=130 97+33=128 False
4 2 125 67+78=145 67+78=144 False
4 2 126 58+05=063 58+05=068 False
4 2 127 00+16=016 00+16=020 False
4 2 128 80+19=099 80+19=108 False
4 2 129 98+22=120 98+22=128 False
4 2 130 09+62=071 09+62=078 False
4 2 131 06+23=029 06+23=030 False
4 2 132 32+99=131 32+99=128 False
4 2 133 17+02=019 17+02=020 False
4 2 134 64+35=099 64+35=108 False
4 2 135 35+83=118 35+83=110 False
4 2 136 71+36=107 71+36=108 False
4 2 137 75+06=081 75+06=078 False
4 2 138 88+95=183 88+95=178 False
4 2 139 19+98=117 19+98=108 False
4 2 140 28+89=117 28+89=108 False
4 2 141 33+11=044 33+11=040 False
4 2 142 34+49=083 34+49=088 False
4 2 143 90+35=125 90+35=128 False
4 2 144 22+90=112 22+90=110 False
4 2 145 98+89=187 98+89=186 False
4 2 146 88+47=135 88+47=138 False
4 2 147 30+86=116 30+86=113 False
4 2 148 31+48=079 31+48=078 False
4 2 149 39+21=060 39+21=068 False
4 2 150 19+17=036 19+17=038 False
4 2 151 27+60=087 27+60=088 False
4 2 152 12+16=028 12+16=020 False
4 2 153 51+75=126 51+75=120 False
4 2 154 10+74=084 10+74=088 False
4 2 155 42+63=105 42+63=108 False
4 2 156 40+14=054 40+14=050 False
4 2 157 23+93=116 23+93=110 False
4 2 158 85+26=111 85+26=113 False
4 2 159 28+46=074 28+46=078 False
4 2 160 28+33=061 28+33=068 False
4 2 161 43+30=073 43+30=070 False
4 2 162 89+72=161 89+72=168 False
4 2 163 52+21=073 52+21=075 False
4 2 164 21+54=075 21+54=070 False
4 2 165 69+13=082 69+13=088 False
4 2 166 07+60=067 07+60=078 False
4 2 167 63+83=146 63+83=140 False
4 2 168 80+69=149 80+69=148 False
4 2 169 27+28=055 27+28=058 False
4 2 170 42+31=073 42+31=075 False
4 2 171 51+99=150 51+99=148 False
4 2 172 28+75=103 28+75=108 False
4 2 173 38+57=095 38+57=088 False
4 2 174 83+16=099 83+16=108 False
4 2 175 92+94=186 92+94=188 False
4 2 176 55+75=130 55+75=120 False
4 2 177 59+51=110 59+51=110 True
4 2 178 33+09=042 33+09=040 False
4 2 179 53+13=066 53+13=064 False
4 2 180 05+70=075 05+70=070 False
4 2 181 12+20=032 12+20=030 False
4 2 182 11+49=060 11+49=060 True
4 2 183 63+45=108 63+45=110 False
4 2 184 92+23=115 92+23=110 False
4 2 185 82+45=127 82+45=120 False
4 2 186 23+41=064 23+41=064 True
4 2 187 64+26=090 64+26=088 False
4 2 188 91+24=115 91+24=110 False
4 2 189 20+32=052 20+32=050 False
4 2 190 83+21=104 83+21=108 False
4 2 191 07+20=027 07+20=030 False
4 2 192 94+14=108 94+14=110 False
4 2 193 96+89=185 96+89=185 True
4 2 194 13+08=021 13+08=020 False
4 2 195 32+05=037 32+05=040 False
4 2 196 09+51=060 09+51=060 True
4 2 197 26+29=055 26+29=058 False
4 2 198 49+65=114 49+65=110 False
4 2 199 32+66=098 32+66=108 False
4 2 200 41+08=049 41+08=050 False
4 2 201 26+79=105 26+79=108 False
4 2 202 29+91=120 29+91=110 False
4 2 203 51+00=051 51+00=060 False
4 2 204 61+60=121 61+60=110 False
4 2 205 45+78=123 45+78=110 False
4 2 206 56+16=072 56+16=074 False
4 2 207 66+68=134 66+68=134 True
4 2 208 32+16=048 32+16=040 False
4 2 209 84+49=133 84+49=138 False
4 2 210 45+09=054 45+09=050 False
4 2 211 96+78=174 96+78=175 False
4 2 212 10+02=012 10+02=020 False
4 2 213 36+60=096 36+60=098 False
4 2 214 44+36=080 44+36=078 False
4 2 215 12+86=098 12+86=098 True
4 2 216 94+54=148 94+54=140 False
4 2 217 64+73=137 64+73=130 False
4 2 218 73+10=083 73+10=080 False
4 2 219 14+62=076 14+62=078 False
4 2 220 25+22=047 25+22=040 False
4 2 221 94+22=116 94+22=110 False
4 2 222 41+76=117 41+76=110 False
4 2 223 38+46=084 38+46=088 False
4 2 224 71+72=143 71+72=130 False
4 2 225 74+79=153 74+79=140 False
4 2 226 99+67=166 99+67=165 False
4 2 227 78+71=149 78+71=148 False
4 2 228 23+19=042 23+19=040 False
4 2 229 51+65=116 51+65=110 False
4 2 230 94+86=180 94+86=178 False
4 2 231 09+79=088 09+79=088 True
4 2 232 69+39=108 69+39=108 True
4 2 233 84+13=097 84+13=098 False
4 2 234 36+59=095 36+59=098 False
4 2 235 87+47=134 87+47=138 False
4 2 236 50+00=050 50+00=060 False
4 2 237 76+96=172 76+96=174 False
4 2 238 12+18=030 12+18=030 True
4 2 239 99+95=194 99+95=188 False
4 2 240 22+00=022 22+00=020 False
4 2 241 96+18=114 96+18=114 True
4 2 242 51+20=071 51+20=070 False
4 2 243 66+81=147 66+81=144 False
4 2 244 78+18=096 78+18=098 False
4 2 245 09+78=087 09+78=088 False
4 2 246 24+20=044 24+20=040 False
4 2 247 76+13=089 76+13=088 False
4 2 248 05+10=015 05+10=020 False
4 2 249 97+14=111 97+14=113 False
4 2 250 92+38=130 92+38=128 False
4 2 251 77+13=090 77+13=098 False
4 2 252 70+19=089 70+19=088 False
4 2 253 90+45=135 90+45=130 False
4 2 254 50+09=059 50+09=068 False
4 2 255 78+06=084 78+06=076 False
4 3 0 03+25=028 03+25=030 False
4 3 1 48+43=091 48+43=088 False
4 3 2 39+47=086 39+47=088 False
4 3 3 48+19=067 48+19=068 False
4 3 4 07+22=029 07+22=030 False
4 3 5 73+68=141 73+68=130 False
4 3 6 14+56=070 14+56=064 False
4 3 7 96+95=191 96+95=184 False
4 3 8 96+28=124 96+28=128 False
4 3 9 82+05=087 82+05=088 False
4 3 10 27+94=121 27+94=113 False
4 3 11 87+86=173 87+86=164 False
4 3 12 00+68=068 00+68=078 False
4 3 13 11+37=048 11+37=040 False
4 3 14 95+93=188 95+93=188 True
4 3 15 75+82=157 75+82=140 False
4 3 16 41+71=112 41+71=110 False
4 3 17 60+14=074 60+14=070 False
4 3 18 77+77=154 77+77=144 False
4 3 19 31+84=115 31+84=110 False
4 3 20 31+57=088 31+57=088 True
4 3 21 27+87=114 27+87=108 False
4 3 22 31+89=120 31+89=110 False
4 3 23 22+18=040 22+18=040 True
4 3 24 38+25=063 38+25=068 False
4 3 25 64+54=118 64+54=110 False
4 3 26 85+60=145 85+60=140 False
4 3 27 14+71=085 14+71=080 False
4 3 28 06+16=022 06+16=024 False
4 3 29 78+61=139 78+61=130 False
4 3 30 65+75=140 65+75=130 False
4 3 31 13+83=096 13+83=098 False
4 3 32 75+49=124 75+49=128 False
4 3 33 05+78=083 05+78=088 False
4 3 34 66+55=121 66+55=110 False
4 3 35 03+05=008 03+05=010 False
4 3 36 69+99=168 69+99=178 False
4 3 37 52+82=134 52+82=130 False
4 3 38 45+97=142 45+97=148 False
4 3 39 66+17=083 66+17=085 False
4 3 40 36+17=053 36+17=054 False
4 3 41 92+74=166 92+74=164 False
4 3 42 48+44=092 48+44=088 False
4 3 43 34+17=051 34+17=040 False
4 3 44 56+11=067 56+11=064 False
4 3 45 77+23=100 77+23=108 False
4 3 46 10+11=021 10+11=020 False
4 3 47 32+65=097 32+65=098 False
4 3 48 53+49=102 53+49=108 False
4 3 49 68+86=154 68+86=154 True
4 3 50 52+94=146 52+94=140 False
4 3 51 97+71=168 97+71=164 False
4 3 52 05+37=042 05+37=040 False
4 3 53 58+75=133 58+75=138 False
4 3 54 06+24=030 06+24=030 True
4 3 55 15+44=059 15+44=050 False
4 3 56 90+49=139 90+49=148 False
4 3 57 50+37=087 50+37=088 False
4 3 58 88+61=149 88+61=148 False
4 3 59 21+57=078 21+57=078 True
4 3 60 24+85=109 24+85=108 False
4 3 61 01+66=067 01+66=074 False
4 3 62 50+46=096 50+46=088 False
4 3 63 76+65=141 76+65=130 False
4 3 64 23+74=097 23+74=098 False
4 3 65 76+16=092 76+16=085 False
4 3 66 06+08=014 06+08=014 True
4 3 67 69+25=094 69+25=088 False
4 3 68 15+23=038 15+23=040 False
4 3 69 41+02=043 41+02=040 False
4 3 70 16+66=082 16+66=084 False
4 3 71 59+94=153 59+94=148 False
4 3 72 32+88=120 32+88=113 False
4 3 73 46+21=067 46+21=064 False
4 3 74 57+28=085 57+28=088 False
4 3 75 00+31=031 00+31=040 False
4 3 76 77+07=084 77+07=078 False
4 3 77 28+70=098 28+70=098 True
4 3 78 05+61=066 05+61=070 False
4 3 79 22+09=031 22+09=030 False
4 3 80 08+94=102 08+94=108 False
4 3 81 40+11=051 40+11=050 False
4 3 82 10+48=058 10+48=058 True
4 3 83 27+56=083 27+56=078 False
4 3 84 42+16=058 42+16=054 False
4 3 85 69+43=112 69+43=110 False
4 3 86 57+69=126 57+69=128 False
4 3 87 18+86=104 18+86=108 False
4 3 88 86+80=166 86+80=164 False
4 3 89 30+85=115 30+85=110 False
4 3 90 77+66=143 77+66=144 False
4 3 91 39+64=103 39+64=108 False
4 3 92 76+61=137 76+61=130 False
4 3 93 42+61=103 42+61=108 False
4 3 94 07+30=037 07+30=040 False
4 3 95 35+93=128 35+93=128 True
4 3 96 40+90=130 40+90=130 True
4 3 97 08+91=099 08+91=098 False
4 3 98 62+34=096 62+34=098 False
4 3 99 86+49=135 86+49=138 False
4 3 100 73+23=096 73+23=098 False
4 3 101 87+35=122 87+35=113 False
4 3 102 35+31=066 35+31=060 False
4 3 103 07+13=020 07+13=020 True
4 3 104 39+41=080 39+41=078 False
4 3 105 44+63=107 44+63=110 False
4 3 106 94+66=160 94+66=164 False
4 3 107 49+54=103 49+54=108 False
4 3 108 79+46=125 79+46=128 False
4 3 109 53+12=065 53+12=064 False
4 3 110 60+92=152 60+92=150 False
4 3 111 25+60=085 25+60=088 False
4 3 112 64+53=117 64+53=110 False
4 3 113 41+02=043 41+02=040 False
4 3 114 00+97=097 00+97=098 False
4 3 115 12+52=064 12+52=064 True
4 3 116 39+50=089 39+50=088 False
4 3 117 87+21=108 87+21=108 True
4 3 118 04+99=103 04+99=108 False
4 3 119 19+75=094 19+75=098 False
4 3 120 90+05=095 90+05=098 False
4 3 121 54+39=093 54+39=088 False
4 3 122 29+26=055 29+26=058 False
4 3 123 82+95=177 82+95=178 False
4 3 124 55+09=064 55+09=068 False
4 3 125 02+62=064 02+62=064 True
4 3 126 68+30=098 68+30=098 True
4 3 127 99+16=115 99+16=114 False
4 3 128 63+11=074 63+11=070 False
4 3 129 42+92=134 42+92=130 False
4 3 130 99+16=115 99+16=114 False
4 3 131 50+31=081 50+31=070 False
4 3 132 23+46=069 23+46=064 False
4 3 133 45+73=118 45+73=110 False
4 3 134 89+77=166 89+77=165 False
4 3 135 45+78=123 45+78=110 False
4 3 136 96+60=156 96+60=164 False
4 3 137 74+61=135 74+61=130 False
4 3 138 87+01=088 87+01=088 True
4 3 139 63+88=151 63+88=148 False
4 3 140 59+72=131 59+72=128 False
4 3 141 17+96=113 17+96=108 False
4 3 142 89+77=166 89+77=165 False
4 3 143 24+69=093 24+69=098 False
4 3 144 75+83=158 75+83=150 False
4 3 145 50+54=104 50+54=108 False
4 3 146 93+47=140 93+47=148 False
4 3 147 20+55=075 20+55=078 False
4 3 148 91+79=170 91+79=178 False
4 3 149 15+13=028 15+13=020 False
4 3 150 86+09=095 86+09=088 False
4 3 151 29+58=087 29+58=088 False
4 3 152 01+29=030 01+29=030 True
4 3 153 65+48=113 65+48=110 False
4 3 154 96+45=141 96+45=148 False
4 3 155 58+69=127 58+69=128 False
4 3 156 84+43=127 84+43=120 False
4 3 157 90+38=128 90+38=128 True
4 3 158 39+97=136 39+97=128 False
4 3 159 74+84=158 74+84=140 False
4 3 160 86+22=108 86+22=108 True
4 3 161 01+86=087 01+86=088 False
4 3 162 81+63=144 81+63=140 False
4 3 163 80+94=174 80+94=178 False
4 3 164 44+42=086 44+42=088 False
4 3 165 72+60=132 72+60=130 False
4 3 166 28+07=035 28+07=038 False
4 3 167 69+54=123 69+54=120 False
4 3 168 68+77=145 68+77=144 False
4 3 169 90+16=106 90+16=113 False
4 3 170 64+50=114 64+50=110 False
4 3 171 46+88=134 46+88=138 False
4 3 172 55+99=154 55+99=148 False
4 3 173 31+97=128 31+97=128 True
4 3 174 79+28=107 79+28=108 False
4 3 175 81+43=124 81+43=120 False
4 3 176 41+15=056 41+15=050 False
4 3 177 38+77=115 38+77=114 False
4 3 178 25+06=031 25+06=030 False
4 3 179 01+93=094 01+93=090 False
4 3 180 97+22=119 97+22=113 False
4 3 181 71+84=155 71+84=140 False
4 3 182 26+36=062 26+36=064 False
4 3 183 60+92=152 60+92=150 False
4 3 184 02+94=096 02+94=098 False
4 3 185 31+58=089 31+58=088 False
4 3 186 70+52=122 70+52=120 False
4 3 187 19+42=061 19+42=068 False
4 3 188 95+73=168 95+73=164 False
4 3 189 21+25=046 21+25=040 False
4 3 190 13+58=071 13+58=078 False
4 3 191 62+28=090 62+28=088 False
4 3 192 38+14=052 38+14=058 False
4 3 193 66+75=141 66+75=130 False
4 3 194 24+59=083 24+59=088 False
4 3 195 97+66=163 97+66=164 False
4 3 196 76+70=146 76+70=144 False
4 3 197 08+40=048 08+40=058 False
4 3 198 84+00=084 84+00=088 False
4 3 199 54+73=127 54+73=120 False
4 3 200 16+88=104 16+88=108 False
4 3 201 99+47=146 99+47=148 False
4 3 202 31+95=126 31+95=128 False
4 3 203 01+79=080 01+79=088 False
4 3 204 03+68=071 03+68=078 False
4 3 205 10+05=015 10+05=020 False
4 3 206 98+90=188 98+90=188 True
4 3 207 58+53=111 58+53=110 False
4 3 208 34+87=121 34+87=110 False
4 3 209 07+31=038 07+31=040 False
4 3 210 59+08=067 59+08=068 False
4 3 211 51+38=089 51+38=088 False
4 3 212 62+62=124 62+62=120 False
4 3 213 80+32=112 80+32=110 False
4 3 214 69+16=085 69+16=088 False
4 3 215 01+17=018 01+17=020 False
4 3 216 74+41=115 74+41=110 False
4 3 217 20+89=109 20+89=108 False
4 3 218 53+50=103 53+50=108 False
4 3 219 82+85=167 82+85=164 False
4 3 220 34+47=081 34+47=078 False
4 3 221 34+45=079 34+45=078 False
4 3 222 77+34=111 77+34=110 False
4 3 223 56+33=089 56+33=088 False
4 3 224 97+56=153 97+56=144 False
4 3 225 29+06=035 29+06=038 False
4 3 226 78+96=174 78+96=175 False
4 3 227 28+65=093 28+65=088 False
4 3 228 61+64=125 61+64=120 False
4 3 229 32+64=096 32+64=098 False
4 3 230 98+32=130 98+32=128 False
4 3 231 25+35=060 25+35=050 False
4 3 232 05+08=013 05+08=010 False
4 3 233 05+26=031 05+26=030 False
4 3 234 84+71=155 84+71=140 False
4 3 235 33+10=043 33+10=040 False
4 3 236 98+35=133 98+35=138 False
4 3 237 68+98=166 68+98=165 False
4 3 238 03+63=066 03+63=070 False
4 3 239 12+96=108 12+96=108 True
4 3 240 02+81=083 02+81=080 False
4 3 241 83+13=096 83+13=098 False
4 3 242 55+92=147 55+92=140 False
4 3 243 96+09=105 96+09=108 False
4 3 244 61+08=069 61+08=078 False
4 3 245 39+75=114 39+75=110 False
4 3 246 40+74=114 40+74=110 False
4 3 247 39+80=119 39+80=113 False
4 3 248 57+95=152 57+95=148 False
4 3 249 92+97=189 92+97=188 False
4 3 250 33+03=036 33+03=040 False
4 3 251 74+92=166 74+92=164 False
4 3 252 99+09=108 99+09=108 True
4 3 253 98+10=108 98+10=113 False
4 3 254 46+77=123 46+77=113 False
4 3 255 85+78=163 85+78=158 False
4 4 0 41+21=062 41+21=060 False
4 4 1 49+13=062 49+13=068 False
4 4 2 59+07=066 59+07=068 False
4 4 3 31+11=042 31+11=040 False
4 4 4 74+16=090 74+16=088 False
4 4 5 43+38=081 43+38=078 False
4 4 6 08+67=075 08+67=078 False
4 4 7 31+66=097 31+66=098 False
4 4 8 10+31=041 10+31=040 False
4 4 9 34+59=093 34+59=088 False
4 4 10 78+42=120 78+42=113 False
4 4 11 13+41=054 13+41=050 False
4 4 12 97+89=186 97+89=188 False
4 4 13 15+62=077 15+62=078 False
4 4 14 39+36=075 39+36=078 False
4 4 15 21+25=046 21+25=040 False
4 4 16 74+56=130 74+56=120 False
4 4 17 85+47=132 85+47=138 False
4 4 18 47+32=079 47+32=078 False
4 4 19 37+66=103 37+66=108 False
4 4 20 16+29=045 16+29=048 False
4 4 21 86+77=163 86+77=164 False
4 4 22 80+07=087 80+07=088 False
4 4 23 87+05=092 87+05=088 False
4 4 24 58+16=074 58+16=078 False
4 4 25 52+79=131 52+79=128 False
4 4 26 91+08=099 91+08=108 False
4 4 27 47+78=125 47+78=128 False
4 4 28 86+96=182 86+96=174 False
4 4 29 90+22=112 90+22=110 False
4 4 30 31+18=049 31+18=040 False
4 4 31 86+15=101 86+15=108 False
4 4 32 15+95=110 15+95=108 False
4 4 33 42+11=053 42+11=050 False
4 4 34 65+99=164 65+99=168 False
4 4 35 89+29=118 89+29=118 True
4 4 36 35+11=046 35+11=040 False
4 4 37 71+41=112 71+41=110 False
4 4 38 16+24=040 16+24=040 True
4 4 39 77+82=159 77+82=154 False
4 4 40 55+89=144 55+89=138 False
4 4 41 17+88=105 17+88=108 False
4 4 42 54+72=126 54+72=120 False
4 4 43 34+98=132 34+98=128 False
4 4 44 09+97=106 09+97=108 False
4 4 45 91+07=098 91+07=098 True
4 4 46 55+94=149 55+94=140 False
4 4 47 22+58=080 22+58=078 False
4 4 48 91+37=128 91+37=128 True
4 4 49 16+10=026 16+10=020 False
4 4 50 96+32=128 96+32=128 True
4 4 51 35+75=110 35+75=110 True
4 4 52 88+73=161 88+73=164 False
4 4 53 35+18=053 35+18=058 False
4 4 54 33+10=043 33+10=040 False
4 4 55 08+50=058 08+50=064 False
4 4 56 22+62=084 22+62=088 False
4 4 57 26+37=063 26+37=064 False
4 4 58 80+27=107 80+27=108 False
4 4 59 68+28=096 68+28=088 False
4 4 60 48+03=051 48+03=058 False
4 4 61 40+18=058 40+18=058 True
4 4 62 16+59=075 16+59=078 False
4 4 63 02+19=021 02+19=020 False
4 4 64 01+09=010 01+09=010 True
4 4 65 62+68=130 62+68=120 False
4 4 66 09+71=080 09+71=088 False
4 4 67 00+58=058 00+58=068 False
4 4 68 16+45=061 16+45=050 False
4 4 69 24+98=122 24+98=113 False
4 4 70 47+92=139 47+92=138 False
4 4 71 94+84=178 94+84=178 True
4 4 72 21+32=053 21+32=050 False
4 4 73 29+82=111 29+82=108 False
4 4 74 32+79=111 32+79=110 False
4 4 75 13+98=111 13+98=108 False
4 4 76 41+94=135 41+94=130 False
4 4 77 51+84=135 51+84=130 False
4 4 78 42+05=047 42+05=050 False
4 4 79 39+03=042 39+03=040 False
4 4 80 02+92=094 02+92=098 False
4 4 81 99+81=180 99+81=178 False
4 4 82 32+68=100 32+68=108 False
4 4 83 52+17=069 52+17=064 False
4 4 84 56+58=114 56+58=113 False
4 4 85 21+48=069 21+48=068 False
4 4 86 61+71=132 61+71=120 False
4 4 87 17+01=018 17+01=020 False
4 4 88 68+23=091 68+23=088 False
4 4 89 00+37=037 00+37=040 False
4 4 90 94+88=182 94+88=178 False
4 4 91 06+31=037 06+31=040 False
4 4 92 27+18=045 27+18=048 False
4 4 93 41+81=122 41+81=110 False
4 4 94 15+86=101 15+86=108 False
4 4 95 36+87=123 36+87=114 False
4 4 96 17+37=054 17+37=058 False
4 4 97 13+86=099 13+86=108 False
4 4 98 29+69=098 29+69=098 True
4 4 99 31+99=130 31+99=128 False
4 4 100 47+29=076 47+29=078 False
4 4 101 08+81=089 08+81=088 False
4 4 102 72+82=154 72+82=140 False
4 4 103 46+91=137 46+91=130 False
4 4 104 70+35=105 70+35=108 False
4 4 105 90+55=145 90+55=140 False
4 4 106 99+99=198 99+99=188 False
4 4 107 60+97=157 60+97=154 False
4 4 108 03+40=043 03+40=050 False
4 4 109 35+49=084 35+49=088 False
4 4 110 32+02=034 32+02=040 False
4 4 111 70+18=088 70+18=088 True
4 4 112 99+05=104 99+05=108 False
4 4 113 78+73=151 78+73=148 False
4 4 114 03+02=005 03+02=010 False
4 4 115 50+14=064 50+14=060 False
4 4 116 62+02=064 62+02=064 True
4 4 117 16+74=090 16+74=098 False
4 4 118 68+65=133 68+65=130 False
4 4 119 74+81=155 74+81=140 False
4 4 120 37+48=085 37+48=088 False
4 4 121 63+04=067 63+04=060 False
4 4 122 06+62=068 06+62=078 False
4 4 123 95+75=170 95+75=164 False
4 4 124 92+37=129 92+37=128 False
4 4 125 81+32=113 81+32=110 False
4 4 126 53+28=081 53+28=078 False
4 4 127 52+42=094 52+42=088 False
4 4 128 66+97=163 66+97=164 False
4 4 129 00+48=048 00+48=058 False
4 4 130 65+32=097 65+32=098 False
4 4 131 60+89=149 60+89=148 False
4 4 132 71+61=132 71+61=120 False
4 4 133 98+50=148 98+50=148 True
4 4 134 90+96=186 90+96=174 False
4 4 135 02+96=098 02+96=098 True
4 4 136 62+75=137 62+75=130 False
4 4 137 41+28=069 41+28=068 False
4 4 138 95+79=174 95+79=178 False
4 4 139 48+41=089 48+41=088 False
4 4 140 87+95=182 87+95=178 False
4 4 141 75+38=113 75+38=113 True
4 4 142 31+55=086 31+55=088 False
4 4 143 54+63=117 54+63=110 False
4 4 144 75+82=157 75+82=140 False
4 4 145 46+45=091 46+45=088 False
4 4 146 13+08=021 13+08=020 False
4 4 147 77+97=174 77+97=175 False
4 4 148 37+35=072 37+35=068 False
4 4 149 21+89=110 21+89=108 False
4 4 150 58+51=109 58+51=108 False
4 4 151 91+48=139 91+48=148 False
4 4 152 33+23=056 33+23=050 False
4 4 153 80+96=176 80+96=174 False
4 4 154 78+02=080 78+02=078 False
4 4 155 38+95=133 38+95=128 False
4 4 156 99+25=124 99+25=128 False
4 4 157 30+76=106 30+76=108 False
4 4 158 42+40=082 42+40=078 False
4 4 159 85+58=143 85+58=148 False
4 4 160 44+46=090 44+46=088 False
4 4 161 06+41=047 06+41=050 False
4 4 162 65+90=155 65+90=150 False
4 4 163 43+83=126 43+83=120 False
4 4 164 36+61=097 36+61=098 False
4 4 165 61+51=112 61+51=110 False
4 4 166 38+09=047 38+09=048 False
4 4 167 21+97=118 21+97=110 False
4 4 168 83+30=113 83+30=110 False
4 4 169 11+79=090 11+79=098 False
4 4 170 14+29=043 14+29=040 False
4 4 171 21+11=032 21+11=030 False
4 4 172 43+53=096 43+53=080 False
4 4 173 02+58=060 02+58=068 False
4 4 174 78+82=160 78+82=154 False
4 4 175 91+11=102 91+11=108 False
4 4 176 58+54=112 58+54=110 False
4 4 177 00+15=015 00+15=020 False
4 4 178 83+51=134 83+51=130 False
4 4 179 44+72=116 44+72=110 False
4 4 180 71+20=091 71+20=098 False
4 4 181 24+99=123 24+99=118 False
4 4 182 46+30=076 46+30=078 False
4 4 183 08+67=075 08+67=078 False
4 4 184 47+42=089 47+42=088 False
4 4 185 95+67=162 95+67=164 False
4 4 186 40+56=096 40+56=088 False
4 4 187 17+95=112 17+95=110 False
4 4 188 94+66=160 94+66=164 False
4 4 189 14+58=072 14+58=078 False
4 4 190 56+05=061 56+05=068 False
4 4 191 70+01=071 70+01=070 False
4 4 192 97+59=156 97+59=158 False
4 4 193 94+67=161 94+67=164 False
4 4 194 13+41=054 13+41=050 False
4 4 195 85+15=100 85+15=108 False
4 4 196 48+53=101 48+53=108 False
4 4 197 62+75=137 62+75=130 False
4 4 198 87+47=134 87+47=138 False
4 4 199 31+88=119 31+88=110 False
4 4 200 97+16=113 97+16=114 False
4 4 201 48+45=093 48+45=088 False
4 4 202 99+00=099 99+00=098 False
4 4 203 15+01=016 15+01=020 False
4 4 204 28+96=124 28+96=114 False
4 4 205 20+11=031 20+11=030 False
4 4 206 07+56=063 07+56=064 False
4 4 207 06+08=014 06+08=014 True
4 4 208 45+46=091 45+46=088 False
4 4 209 48+85=133 48+85=138 False
4 4 210 62+14=076 62+14=070 False
4 4 211 82+31=113 82+31=110 False
4 4 212 85+88=173 85+88=168 False
4 4 213 77+08=085 77+08=088 False
4 4 214 16+64=080 16+64=088 False
4 4 215 00+27=027 00+27=030 False
4 4 216 36+75=111 36+75=110 False
4 4 217 38+38=076 38+38=078 False
4 4 218 88+32=120 88+32=113 False
4 4 219 09+88=097 09+88=098 False
4 4 220 96+87=183 96+87=184 False
4 4 221 71+29=100 71+29=108 False
4 4 222 99+13=112 99+13=113 False
4 4 223 03+13=016 03+13=020 False
4 4 224 67+23=090 67+23=088 False
4 4 225 15+98=113 15+98=108 False
4 4 226 10+08=018 10+08=028 False
4 4 227 46+24=070 46+24=068 False
4 4 228 55+63=118 55+63=110 False
4 4 229 28+06=034 28+06=038 False
4 4 230 43+87=130 43+87=128 False
4 4 231 34+05=039 34+05=040 False
2024-12-17 09:44:09,350 - root - WARNING - The method `test_one_epoch` is not implemented. Please override this method in a subclass or use a custom callback.
2024-12-17 09:44:09,352 - root - INFO - ====================================================== Starting Train Epoch: 5/9 ======================================================
2024-12-17 09:44:09,352 - root - INFO - Learning rates for each parameter group: 0.00166297526309434830, 0.00166297526309434830
0%| | 0/28 [00:00<?, ?it/s]
Epoch: 5, Step: 1: 0%| | 0/28 [00:00<?, ?it/s]
Epoch: 5, Step: 1: 0%| | 0/28 [00:00<?, ?it/s, total_batch_loss=204.42258, average_batch_loss=0.79853, average_batch_perplexity=2.22226, lr=0.001662975, 0.001662975]
Epoch: 5, Step: 2: 0%| | 0/28 [00:00<?, ?it/s, total_batch_loss=204.42258, average_batch_loss=0.79853, average_batch_perplexity=2.22226, lr=0.001662975, 0.001662975]
Epoch: 5, Step: 2: 0%| | 0/28 [00:00<?, ?it/s, total_batch_loss=204.94623, average_batch_loss=0.80057, average_batch_perplexity=2.22681, lr=0.001655665, 0.001655665]
Epoch: 5, Step: 2: 7%|▋ | 2/28 [00:00<00:02, 11.54it/s, total_batch_loss=204.94623, average_batch_loss=0.80057, average_batch_perplexity=2.22681, lr=0.001655665, 0.001655665]
Epoch: 5, Step: 3: 7%|▋ | 2/28 [00:00<00:02, 11.54it/s, total_batch_loss=204.94623, average_batch_loss=0.80057, average_batch_perplexity=2.22681, lr=0.001655665, 0.001655665]
Epoch: 5, Step: 3: 7%|▋ | 2/28 [00:00<00:02, 11.54it/s, total_batch_loss=207.90443, average_batch_loss=0.81213, average_batch_perplexity=2.25269, lr=0.001648451, 0.001648451]
Epoch: 5, Step: 4: 7%|▋ | 2/28 [00:00<00:02, 11.54it/s, total_batch_loss=207.90443, average_batch_loss=0.81213, average_batch_perplexity=2.25269, lr=0.001648451, 0.001648451]
Epoch: 5, Step: 4: 7%|▋ | 2/28 [00:00<00:02, 11.54it/s, total_batch_loss=203.25394, average_batch_loss=0.79396, average_batch_perplexity=2.21214, lr=0.001641330, 0.001641330]
Epoch: 5, Step: 4: 14%|█▍ | 4/28 [00:00<00:01, 12.11it/s, total_batch_loss=203.25394, average_batch_loss=0.79396, average_batch_perplexity=2.21214, lr=0.001641330, 0.001641330]
Epoch: 5, Step: 5: 14%|█▍ | 4/28 [00:00<00:01, 12.11it/s, total_batch_loss=203.25394, average_batch_loss=0.79396, average_batch_perplexity=2.21214, lr=0.001641330, 0.001641330]
Epoch: 5, Step: 5: 14%|█▍ | 4/28 [00:00<00:01, 12.11it/s, total_batch_loss=202.27339, average_batch_loss=0.79013, average_batch_perplexity=2.20368, lr=0.001634301, 0.001634301]
Epoch: 5, Step: 6: 14%|█▍ | 4/28 [00:00<00:01, 12.11it/s, total_batch_loss=202.27339, average_batch_loss=0.79013, average_batch_perplexity=2.20368, lr=0.001634301, 0.001634301]
Epoch: 5, Step: 6: 14%|█▍ | 4/28 [00:00<00:01, 12.11it/s, total_batch_loss=212.64465, average_batch_loss=0.83064, average_batch_perplexity=2.29479, lr=0.001627361, 0.001627361]
Epoch: 5, Step: 6: 21%|██▏ | 6/28 [00:00<00:01, 12.15it/s, total_batch_loss=212.64465, average_batch_loss=0.83064, average_batch_perplexity=2.29479, lr=0.001627361, 0.001627361]
Epoch: 5, Step: 7: 21%|██▏ | 6/28 [00:00<00:01, 12.15it/s, total_batch_loss=212.64465, average_batch_loss=0.83064, average_batch_perplexity=2.29479, lr=0.001627361, 0.001627361]
Epoch: 5, Step: 7: 21%|██▏ | 6/28 [00:00<00:01, 12.15it/s, total_batch_loss=201.84828, average_batch_loss=0.78847, average_batch_perplexity=2.20003, lr=0.001620509, 0.001620509]
Epoch: 5, Step: 8: 21%|██▏ | 6/28 [00:00<00:01, 12.15it/s, total_batch_loss=201.84828, average_batch_loss=0.78847, average_batch_perplexity=2.20003, lr=0.001620509, 0.001620509]
Epoch: 5, Step: 8: 21%|██▏ | 6/28 [00:00<00:01, 12.15it/s, total_batch_loss=198.03368, average_batch_loss=0.77357, average_batch_perplexity=2.16749, lr=0.001613743, 0.001613743]
Epoch: 5, Step: 8: 29%|██▊ | 8/28 [00:00<00:01, 12.20it/s, total_batch_loss=198.03368, average_batch_loss=0.77357, average_batch_perplexity=2.16749, lr=0.001613743, 0.001613743]
Epoch: 5, Step: 9: 29%|██▊ | 8/28 [00:00<00:01, 12.20it/s, total_batch_loss=198.03368, average_batch_loss=0.77357, average_batch_perplexity=2.16749, lr=0.001613743, 0.001613743]
Epoch: 5, Step: 9: 29%|██▊ | 8/28 [00:00<00:01, 12.20it/s, total_batch_loss=200.52016, average_batch_loss=0.78328, average_batch_perplexity=2.18864, lr=0.001607061, 0.001607061]
Epoch: 5, Step: 10: 29%|██▊ | 8/28 [00:00<00:01, 12.20it/s, total_batch_loss=200.52016, average_batch_loss=0.78328, average_batch_perplexity=2.18864, lr=0.001607061, 0.001607061]
Epoch: 5, Step: 10: 29%|██▊ | 8/28 [00:00<00:01, 12.20it/s, total_batch_loss=201.06064, average_batch_loss=0.78539, average_batch_perplexity=2.19327, lr=0.001600461, 0.001600461]
Epoch: 5, Step: 10: 36%|███▌ | 10/28 [00:00<00:01, 12.36it/s, total_batch_loss=201.06064, average_batch_loss=0.78539, average_batch_perplexity=2.19327, lr=0.001600461, 0.001600461]
Epoch: 5, Step: 11: 36%|███▌ | 10/28 [00:00<00:01, 12.36it/s, total_batch_loss=201.06064, average_batch_loss=0.78539, average_batch_perplexity=2.19327, lr=0.001600461, 0.001600461]
Epoch: 5, Step: 11: 36%|███▌ | 10/28 [00:00<00:01, 12.36it/s, total_batch_loss=191.97321, average_batch_loss=0.74990, average_batch_perplexity=2.11678, lr=0.001593942, 0.001593942]
Epoch: 5, Step: 12: 36%|███▌ | 10/28 [00:00<00:01, 12.36it/s, total_batch_loss=191.97321, average_batch_loss=0.74990, average_batch_perplexity=2.11678, lr=0.001593942, 0.001593942]
Epoch: 5, Step: 12: 36%|███▌ | 10/28 [00:00<00:01, 12.36it/s, total_batch_loss=197.50186, average_batch_loss=0.77149, average_batch_perplexity=2.16299, lr=0.001587502, 0.001587502]
Epoch: 5, Step: 12: 43%|████▎ | 12/28 [00:00<00:01, 12.33it/s, total_batch_loss=197.50186, average_batch_loss=0.77149, average_batch_perplexity=2.16299, lr=0.001587502, 0.001587502]
Epoch: 5, Step: 13: 43%|████▎ | 12/28 [00:01<00:01, 12.33it/s, total_batch_loss=197.50186, average_batch_loss=0.77149, average_batch_perplexity=2.16299, lr=0.001587502, 0.001587502]
Epoch: 5, Step: 13: 43%|████▎ | 12/28 [00:01<00:01, 12.33it/s, total_batch_loss=199.21339, average_batch_loss=0.77818, average_batch_perplexity=2.17750, lr=0.001581139, 0.001581139]
Epoch: 5, Step: 14: 43%|████▎ | 12/28 [00:01<00:01, 12.33it/s, total_batch_loss=199.21339, average_batch_loss=0.77818, average_batch_perplexity=2.17750, lr=0.001581139, 0.001581139]
Epoch: 5, Step: 14: 43%|████▎ | 12/28 [00:01<00:01, 12.33it/s, total_batch_loss=195.82437, average_batch_loss=0.76494, average_batch_perplexity=2.14886, lr=0.001574852, 0.001574852]
Epoch: 5, Step: 14: 50%|█████ | 14/28 [00:01<00:01, 12.44it/s, total_batch_loss=195.82437, average_batch_loss=0.76494, average_batch_perplexity=2.14886, lr=0.001574852, 0.001574852]
Epoch: 5, Step: 15: 50%|█████ | 14/28 [00:01<00:01, 12.44it/s, total_batch_loss=195.82437, average_batch_loss=0.76494, average_batch_perplexity=2.14886, lr=0.001574852, 0.001574852]
Epoch: 5, Step: 15: 50%|█████ | 14/28 [00:01<00:01, 12.44it/s, total_batch_loss=184.58960, average_batch_loss=0.72105, average_batch_perplexity=2.05660, lr=0.001568640, 0.001568640]
Epoch: 5, Step: 16: 50%|█████ | 14/28 [00:01<00:01, 12.44it/s, total_batch_loss=184.58960, average_batch_loss=0.72105, average_batch_perplexity=2.05660, lr=0.001568640, 0.001568640]
Epoch: 5, Step: 16: 50%|█████ | 14/28 [00:01<00:01, 12.44it/s, total_batch_loss=192.18750, average_batch_loss=0.75073, average_batch_perplexity=2.11855, lr=0.001562500, 0.001562500]
Epoch: 5, Step: 16: 57%|█████▋ | 16/28 [00:01<00:00, 12.44it/s, total_batch_loss=192.18750, average_batch_loss=0.75073, average_batch_perplexity=2.11855, lr=0.001562500, 0.001562500]
Epoch: 5, Step: 17: 57%|█████▋ | 16/28 [00:01<00:00, 12.44it/s, total_batch_loss=192.18750, average_batch_loss=0.75073, average_batch_perplexity=2.11855, lr=0.001562500, 0.001562500]
Epoch: 5, Step: 17: 57%|█████▋ | 16/28 [00:01<00:00, 12.44it/s, total_batch_loss=184.87943, average_batch_loss=0.72219, average_batch_perplexity=2.05893, lr=0.001556432, 0.001556432]
Epoch: 5, Step: 18: 57%|█████▋ | 16/28 [00:01<00:00, 12.44it/s, total_batch_loss=184.87943, average_batch_loss=0.72219, average_batch_perplexity=2.05893, lr=0.001556432, 0.001556432]
Epoch: 5, Step: 18: 57%|█████▋ | 16/28 [00:01<00:00, 12.44it/s, total_batch_loss=181.38622, average_batch_loss=0.70854, average_batch_perplexity=2.03102, lr=0.001550434, 0.001550434]
Epoch: 5, Step: 18: 64%|██████▍ | 18/28 [00:01<00:00, 12.44it/s, total_batch_loss=181.38622, average_batch_loss=0.70854, average_batch_perplexity=2.03102, lr=0.001550434, 0.001550434]
Epoch: 5, Step: 19: 64%|██████▍ | 18/28 [00:01<00:00, 12.44it/s, total_batch_loss=181.38622, average_batch_loss=0.70854, average_batch_perplexity=2.03102, lr=0.001550434, 0.001550434]
Epoch: 5, Step: 19: 64%|██████▍ | 18/28 [00:01<00:00, 12.44it/s, total_batch_loss=178.76575, average_batch_loss=0.69830, average_batch_perplexity=2.01034, lr=0.001544505, 0.001544505]
Epoch: 5, Step: 20: 64%|██████▍ | 18/28 [00:01<00:00, 12.44it/s, total_batch_loss=178.76575, average_batch_loss=0.69830, average_batch_perplexity=2.01034, lr=0.001544505, 0.001544505]
Epoch: 5, Step: 20: 64%|██████▍ | 18/28 [00:01<00:00, 12.44it/s, total_batch_loss=175.23106, average_batch_loss=0.68450, average_batch_perplexity=1.98277, lr=0.001538644, 0.001538644]
Epoch: 5, Step: 20: 71%|███████▏ | 20/28 [00:01<00:00, 12.59it/s, total_batch_loss=175.23106, average_batch_loss=0.68450, average_batch_perplexity=1.98277, lr=0.001538644, 0.001538644]
Epoch: 5, Step: 21: 71%|███████▏ | 20/28 [00:01<00:00, 12.59it/s, total_batch_loss=175.23106, average_batch_loss=0.68450, average_batch_perplexity=1.98277, lr=0.001538644, 0.001538644]
Epoch: 5, Step: 21: 71%|███████▏ | 20/28 [00:01<00:00, 12.59it/s, total_batch_loss=175.56744, average_batch_loss=0.68581, average_batch_perplexity=1.98538, lr=0.001532848, 0.001532848]
Epoch: 5, Step: 22: 71%|███████▏ | 20/28 [00:01<00:00, 12.59it/s, total_batch_loss=175.56744, average_batch_loss=0.68581, average_batch_perplexity=1.98538, lr=0.001532848, 0.001532848]
Epoch: 5, Step: 22: 71%|███████▏ | 20/28 [00:01<00:00, 12.59it/s, total_batch_loss=172.26785, average_batch_loss=0.67292, average_batch_perplexity=1.95995, lr=0.001527118, 0.001527118]
Epoch: 5, Step: 22: 79%|███████▊ | 22/28 [00:01<00:00, 12.70it/s, total_batch_loss=172.26785, average_batch_loss=0.67292, average_batch_perplexity=1.95995, lr=0.001527118, 0.001527118]
Epoch: 5, Step: 23: 79%|███████▊ | 22/28 [00:01<00:00, 12.70it/s, total_batch_loss=172.26785, average_batch_loss=0.67292, average_batch_perplexity=1.95995, lr=0.001527118, 0.001527118]
Epoch: 5, Step: 23: 79%|███████▊ | 22/28 [00:01<00:00, 12.70it/s, total_batch_loss=172.82648, average_batch_loss=0.67510, average_batch_perplexity=1.96424, lr=0.001521452, 0.001521452]
Epoch: 5, Step: 24: 79%|███████▊ | 22/28 [00:01<00:00, 12.70it/s, total_batch_loss=172.82648, average_batch_loss=0.67510, average_batch_perplexity=1.96424, lr=0.001521452, 0.001521452]
Epoch: 5, Step: 24: 79%|███████▊ | 22/28 [00:01<00:00, 12.70it/s, total_batch_loss=185.04572, average_batch_loss=0.72283, average_batch_perplexity=2.06027, lr=0.001515848, 0.001515848]
Epoch: 5, Step: 24: 86%|████████▌ | 24/28 [00:01<00:00, 12.60it/s, total_batch_loss=185.04572, average_batch_loss=0.72283, average_batch_perplexity=2.06027, lr=0.001515848, 0.001515848]
Epoch: 5, Step: 25: 86%|████████▌ | 24/28 [00:02<00:00, 12.60it/s, total_batch_loss=185.04572, average_batch_loss=0.72283, average_batch_perplexity=2.06027, lr=0.001515848, 0.001515848]
Epoch: 5, Step: 25: 86%|████████▌ | 24/28 [00:02<00:00, 12.60it/s, total_batch_loss=165.06934, average_batch_loss=0.64480, average_batch_perplexity=1.90561, lr=0.001510305, 0.001510305]
Epoch: 5, Step: 26: 86%|████████▌ | 24/28 [00:02<00:00, 12.60it/s, total_batch_loss=165.06934, average_batch_loss=0.64480, average_batch_perplexity=1.90561, lr=0.001510305, 0.001510305]
Epoch: 5, Step: 26: 86%|████████▌ | 24/28 [00:02<00:00, 12.60it/s, total_batch_loss=161.05930, average_batch_loss=0.62914, average_batch_perplexity=1.87599, lr=0.001504823, 0.001504823]
Epoch: 5, Step: 26: 93%|█████████▎| 26/28 [00:02<00:00, 12.61it/s, total_batch_loss=161.05930, average_batch_loss=0.62914, average_batch_perplexity=1.87599, lr=0.001504823, 0.001504823]
Epoch: 5, Step: 27: 93%|█████████▎| 26/28 [00:02<00:00, 12.61it/s, total_batch_loss=161.05930, average_batch_loss=0.62914, average_batch_perplexity=1.87599, lr=0.001504823, 0.001504823]
Epoch: 5, Step: 27: 93%|█████████▎| 26/28 [00:02<00:00, 12.61it/s, total_batch_loss=158.47107, average_batch_loss=0.61903, average_batch_perplexity=1.85712, lr=0.001499400, 0.001499400]
Epoch: 5, Step: 28: 93%|█████████▎| 26/28 [00:02<00:00, 12.61it/s, total_batch_loss=158.47107, average_batch_loss=0.61903, average_batch_perplexity=1.85712, lr=0.001499400, 0.001499400]
Epoch: 5, Step: 28: 93%|█████████▎| 26/28 [00:02<00:00, 12.61it/s, total_batch_loss=58.26158, average_batch_loss=0.66206, average_batch_perplexity=1.93879, lr=0.001494036, 0.001494036]
Epoch: 5, Step: 28: 100%|██████████| 28/28 [00:02<00:00, 13.84it/s, total_batch_loss=58.26158, average_batch_loss=0.66206, average_batch_perplexity=1.93879, lr=0.001494036, 0.001494036]
2024-12-17 09:44:11,557 - root - INFO - Total Samples: 7000
2024-12-17 09:44:11,557 - root - INFO - Total Batches: 28
2024-12-17 09:44:11,558 - root - INFO - Average Epoch Train Loss: 0.73815
2024-12-17 09:44:11,559 - root - INFO - Average Epoch Train Perplexity: 2.09206
2024-12-17 09:44:11,559 - root - INFO -
2024-12-17 09:44:11,560 - root - INFO - ====================================================== Starting Valid Epoch: 5/9 ======================================================
0%| | 0/8 [00:00<?, ?it/s]
Epoch: 5, Step: 1: 0%| | 0/8 [00:00<?, ?it/s]
Epoch: 5, Step: 1: 0%| | 0/8 [00:00<?, ?it/s, total_batch_loss=143.81348, average_batch_loss=0.56177, average_batch_perplexity=1.75378]
Epoch: 5, Step: 2: 0%| | 0/8 [00:00<?, ?it/s, total_batch_loss=143.81348, average_batch_loss=0.56177, average_batch_perplexity=1.75378]
Epoch: 5, Step: 2: 0%| | 0/8 [00:00<?, ?it/s, total_batch_loss=143.66689, average_batch_loss=0.56120, average_batch_perplexity=1.75277]
Epoch: 5, Step: 3: 0%| | 0/8 [00:00<?, ?it/s, total_batch_loss=143.66689, average_batch_loss=0.56120, average_batch_perplexity=1.75277]
Epoch: 5, Step: 3: 0%| | 0/8 [00:00<?, ?it/s, total_batch_loss=142.74083, average_batch_loss=0.55758, average_batch_perplexity=1.74644]
Epoch: 5, Step: 3: 38%|███▊ | 3/8 [00:00<00:00, 23.01it/s, total_batch_loss=142.74083, average_batch_loss=0.55758, average_batch_perplexity=1.74644]
Epoch: 5, Step: 4: 38%|███▊ | 3/8 [00:00<00:00, 23.01it/s, total_batch_loss=142.74083, average_batch_loss=0.55758, average_batch_perplexity=1.74644]
Epoch: 5, Step: 4: 38%|███▊ | 3/8 [00:00<00:00, 23.01it/s, total_batch_loss=138.69589, average_batch_loss=0.54178, average_batch_perplexity=1.71907]
Epoch: 5, Step: 5: 38%|███▊ | 3/8 [00:00<00:00, 23.01it/s, total_batch_loss=138.69589, average_batch_loss=0.54178, average_batch_perplexity=1.71907]
Epoch: 5, Step: 5: 38%|███▊ | 3/8 [00:00<00:00, 23.01it/s, total_batch_loss=145.46661, average_batch_loss=0.56823, average_batch_perplexity=1.76514]
Epoch: 5, Step: 6: 38%|███▊ | 3/8 [00:00<00:00, 23.01it/s, total_batch_loss=145.46661, average_batch_loss=0.56823, average_batch_perplexity=1.76514]
Epoch: 5, Step: 6: 38%|███▊ | 3/8 [00:00<00:00, 23.01it/s, total_batch_loss=143.80544, average_batch_loss=0.56174, average_batch_perplexity=1.75372]
Epoch: 5, Step: 6: 75%|███████▌ | 6/8 [00:00<00:00, 22.74it/s, total_batch_loss=143.80544, average_batch_loss=0.56174, average_batch_perplexity=1.75372]
Epoch: 5, Step: 7: 75%|███████▌ | 6/8 [00:00<00:00, 22.74it/s, total_batch_loss=143.80544, average_batch_loss=0.56174, average_batch_perplexity=1.75372]
Epoch: 5, Step: 7: 75%|███████▌ | 6/8 [00:00<00:00, 22.74it/s, total_batch_loss=141.84766, average_batch_loss=0.55409, average_batch_perplexity=1.74036]
Epoch: 5, Step: 8: 75%|███████▌ | 6/8 [00:00<00:00, 22.74it/s, total_batch_loss=141.84766, average_batch_loss=0.55409, average_batch_perplexity=1.74036]
Epoch: 5, Step: 8: 75%|███████▌ | 6/8 [00:00<00:00, 22.74it/s, total_batch_loss=112.36506, average_batch_loss=0.54022, average_batch_perplexity=1.71638]
2024-12-17 09:44:11,903 - root - INFO - Total Samples: 2000
2024-12-17 09:44:11,903 - root - INFO - Total Batches: 8
2024-12-17 09:44:11,904 - root - INFO - Average Epoch Valid Loss: 0.55620
2024-12-17 09:44:11,904 - root - INFO - Average Epoch Valid Perplexity: 1.74403
2024-12-17 09:44:11,905 - root - INFO -
Evaluating and Generation.: 0%| | 0/4 [00:00<?, ?it/s]
Evaluating and Generation.: 0%| | 0/4 [00:00<?, ?it/s, accuracy: 0.2852]
Evaluating and Generation.: 0%| | 0/4 [00:00<?, ?it/s, accuracy: 0.2969]
Evaluating and Generation.: 50%|█████ | 2/4 [00:00<00:00, 10.57it/s, accuracy: 0.2969]
Evaluating and Generation.: 50%|█████ | 2/4 [00:00<00:00, 10.57it/s, accuracy: 0.2305]
Evaluating and Generation.: 50%|█████ | 2/4 [00:00<00:00, 10.57it/s, accuracy: 0.2672]
Evaluating and Generation.: 100%|██████████| 4/4 [00:00<00:00, 10.86it/s, accuracy: 0.2672]
2024-12-17 09:44:12,281 - root - INFO - Correct/Total Samples: 270/1000
2024-12-17 09:44:12,281 - root - INFO - Eval Accuracy: 0.27
2024-12-17 09:44:12,300 - root - INFO -
epoch batch_index sample_index equation generated correct
5 1 0 13+48=061 13+48=061 True
5 1 1 16+55=071 16+55=071 True
5 1 2 79+34=113 79+34=112 False
5 1 3 35+44=079 35+44=088 False
5 1 4 16+50=066 16+50=064 False
5 1 5 28+47=075 28+47=075 True
5 1 6 00+74=074 00+74=077 False
5 1 7 15+20=035 15+20=037 False
5 1 8 72+60=132 72+60=132 True
5 1 9 63+68=131 63+68=131 True
5 1 10 29+45=074 29+45=072 False
5 1 11 34+60=094 34+60=093 False
5 1 12 53+70=123 53+70=123 True
5 1 13 70+50=120 70+50=120 True
5 1 14 11+84=095 11+84=097 False
5 1 15 42+71=113 42+71=117 False
5 1 16 98+22=120 98+22=111 False
5 1 17 02+02=004 02+02=007 False
5 1 18 15+85=100 15+85=102 False
5 1 19 21+78=099 21+78=109 False
5 1 20 61+79=140 61+79=140 True
5 1 21 25+99=124 25+99=123 False
5 1 22 09+85=094 09+85=092 False
5 1 23 60+91=151 60+91=151 True
5 1 24 35+30=065 35+30=063 False
5 1 25 24+51=075 24+51=077 False
5 1 26 93+91=184 93+91=183 False
5 1 27 39+96=135 39+96=134 False
5 1 28 64+35=099 64+35=098 False
5 1 29 36+22=058 36+22=059 False
5 1 30 68+45=113 68+45=112 False
5 1 31 16+84=100 16+84=100 True
5 1 32 91+52=143 91+52=147 False
5 1 33 97+36=133 97+36=134 False
5 1 34 27+37=064 27+37=065 False
5 1 35 99+82=181 99+82=181 True
5 1 36 03+42=045 03+42=057 False
5 1 37 18+38=056 18+38=057 False
5 1 38 32+20=052 32+20=057 False
5 1 39 38+13=051 38+13=051 True
5 1 40 68+42=110 68+42=111 False
5 1 41 64+00=064 64+00=077 False
5 1 42 48+94=142 48+94=142 True
5 1 43 58+36=094 58+36=094 True
5 1 44 41+22=063 41+22=067 False
5 1 45 23+58=081 23+58=081 True
5 1 46 67+46=113 67+46=114 False
5 1 47 40+78=118 40+78=117 False
5 1 48 90+38=128 90+38=127 False
5 1 49 89+52=141 89+52=141 True
5 1 50 37+77=114 37+77=115 False
5 1 51 29+76=105 29+76=105 True
5 1 52 42+90=132 42+90=132 True
5 1 53 45+82=127 45+82=137 False
5 1 54 35+95=130 35+95=132 False
5 1 55 92+98=190 92+98=191 False
5 1 56 73+91=164 73+91=167 False
5 1 57 53+97=150 53+97=159 False
5 1 58 98+69=167 98+69=165 False
5 1 59 20+46=066 20+46=065 False
5 1 60 48+69=117 48+69=115 False
5 1 61 62+31=093 62+31=097 False
5 1 62 80+59=139 80+59=149 False
5 1 63 58+12=070 58+12=071 False
5 1 64 08+96=104 08+96=105 False
5 1 65 67+06=073 67+06=074 False
5 1 66 22+04=026 22+04=027 False
5 1 67 61+87=148 61+87=157 False
5 1 68 95+27=122 95+27=122 True
5 1 69 49+83=132 49+83=132 True
5 1 70 43+00=043 43+00=053 False
5 1 71 01+85=086 01+85=097 False
5 1 72 11+68=079 11+68=078 False
5 1 73 80+03=083 80+03=087 False
5 1 74 54+83=137 54+83=137 True
5 1 75 73+47=120 73+47=129 False
5 1 76 99+93=192 99+93=192 True
5 1 77 99+13=112 99+13=112 True
5 1 78 92+66=158 92+66=158 True
5 1 79 90+31=121 90+31=120 False
5 1 80 25+69=094 25+69=092 False
5 1 81 25+44=069 25+44=078 False
5 1 82 00+93=093 00+93=097 False
5 1 83 88+87=175 88+87=175 True
5 1 84 47+56=103 47+56=105 False
5 1 85 43+59=102 43+59=102 True
5 1 86 22+00=022 22+00=017 False
5 1 87 34+04=038 34+04=037 False
5 1 88 65+13=078 65+13=077 False
5 1 89 39+82=121 39+82=121 True
5 1 90 66+83=149 66+83=159 False
5 1 91 51+69=120 51+69=120 True
5 1 92 80+21=101 80+21=109 False
5 1 93 36+79=115 36+79=114 False
5 1 94 21+68=089 21+68=098 False
5 1 95 11+66=077 11+66=076 False
5 1 96 55+19=074 55+19=072 False
5 1 97 51+61=112 51+61=110 False
5 1 98 38+88=126 38+88=126 True
5 1 99 37+27=064 37+27=065 False
5 1 100 18+63=081 18+63=081 True
5 1 101 48+11=059 48+11=069 False
5 1 102 72+68=140 72+68=131 False
5 1 103 37+39=076 37+39=076 True
5 1 104 64+95=159 64+95=152 False
5 1 105 49+75=124 49+75=122 False
5 1 106 45+66=111 45+66=110 False
5 1 107 34+87=121 34+87=121 True
5 1 108 02+84=086 02+84=087 False
5 1 109 95+00=095 95+00=093 False
5 1 110 09+56=065 09+56=064 False
5 1 111 22+66=088 22+66=098 False
5 1 112 43+18=061 43+18=061 True
5 1 113 61+35=096 61+35=097 False
5 1 114 13+73=086 13+73=087 False
5 1 115 25+95=120 25+95=122 False
5 1 116 73+96=169 73+96=169 True
5 1 117 03+96=099 03+96=108 False
5 1 118 97+82=179 97+82=189 False
5 1 119 18+42=060 18+42=061 False
5 1 120 29+98=127 29+98=127 True
5 1 121 61+00=061 61+00=060 False
5 1 122 22+98=120 22+98=110 False
5 1 123 12+50=062 12+50=063 False
5 1 124 02+58=060 02+58=060 True
5 1 125 75+86=161 75+86=150 False
5 1 126 31+57=088 31+57=097 False
5 1 127 49+82=131 49+82=131 True
5 1 128 15+33=048 15+33=058 False
5 1 129 49+57=106 49+57=115 False
5 1 130 61+70=131 61+70=130 False
5 1 131 91+51=142 91+51=141 False
5 1 132 50+05=055 50+05=063 False
5 1 133 44+16=060 44+16=060 True
5 1 134 92+01=093 92+01=097 False
5 1 135 85+82=167 85+82=167 True
5 1 136 07+41=048 07+41=058 False
5 1 137 40+06=046 40+06=055 False
5 1 138 79+62=141 79+62=141 True
5 1 139 95+62=157 95+62=157 True
5 1 140 42+93=135 42+93=137 False
5 1 141 32+73=105 32+73=107 False
5 1 142 47+09=056 47+09=055 False
5 1 143 59+50=109 59+50=110 False
5 1 144 61+77=138 61+77=137 False
5 1 145 64+06=070 64+06=070 True
5 1 146 35+10=045 35+10=047 False
5 1 147 32+88=120 32+88=120 True
5 1 148 03+95=098 03+95=097 False
5 1 149 11+38=049 11+38=059 False
5 1 150 21+67=088 21+67=097 False
5 1 151 33+25=058 33+25=058 True
5 1 152 63+45=108 63+45=107 False
5 1 153 56+12=068 56+12=079 False
5 1 154 19+79=098 19+79=097 False
5 1 155 60+43=103 60+43=107 False
5 1 156 07+61=068 07+61=078 False
5 1 157 58+03=061 58+03=061 True
5 1 158 11+10=021 11+10=010 False
5 1 159 49+89=138 49+89=138 True
5 1 160 37+58=095 37+58=095 True
5 1 161 59+78=137 59+78=137 True
5 1 162 11+21=032 11+21=030 False
5 1 163 37+43=080 37+43=081 False
5 1 164 44+21=065 44+21=067 False
5 1 165 22+97=119 22+97=118 False
5 1 166 65+35=100 65+35=102 False
5 1 167 06+51=057 06+51=065 False
5 1 168 65+25=090 65+25=092 False
5 1 169 74+94=168 74+94=167 False
5 1 170 87+55=142 87+55=142 True
5 1 171 90+67=157 90+67=155 False
5 1 172 11+02=013 11+02=007 False
5 1 173 01+66=067 01+66=075 False
5 1 174 56+00=056 56+00=065 False
5 1 175 58+52=110 58+52=111 False
5 1 176 24+99=123 24+99=123 True
5 1 177 97+13=110 97+13=110 True
5 1 178 42+94=136 42+94=137 False
5 1 179 60+15=075 60+15=077 False
5 1 180 20+46=066 20+46=065 False
5 1 181 40+70=110 40+70=110 True
5 1 182 95+45=140 95+45=132 False
5 1 183 96+95=191 96+95=191 True
5 1 184 98+20=118 98+20=115 False
5 1 185 43+19=062 43+19=061 False
5 1 186 50+69=119 50+69=129 False
5 1 187 27+53=080 27+53=081 False
5 1 188 24+25=049 24+25=058 False
5 1 189 65+92=157 65+92=157 True
5 1 190 28+14=042 28+14=032 False
5 1 191 20+57=077 20+57=086 False
5 1 192 59+97=156 59+97=155 False
5 1 193 98+32=130 98+32=121 False
5 1 194 55+84=139 55+84=147 False
5 1 195 20+39=059 20+39=050 False
5 1 196 86+47=133 86+47=135 False
5 1 197 92+36=128 92+36=128 True
5 1 198 05+38=043 05+38=042 False
5 1 199 77+36=113 77+36=114 False
5 1 200 41+64=105 41+64=107 False
5 1 201 74+51=125 74+51=127 False
5 1 202 74+55=129 74+55=127 False
5 1 203 64+64=128 64+64=127 False
5 1 204 60+19=079 60+19=078 False
5 1 205 77+96=173 77+96=164 False
5 1 206 22+30=052 22+30=053 False
5 1 207 82+49=131 82+49=131 True
5 1 208 39+67=106 39+67=106 True
5 1 209 62+40=102 62+40=102 True
5 1 210 28+71=099 28+71=099 True
5 1 211 47+26=073 47+26=075 False
5 1 212 98+54=152 98+54=152 True
5 1 213 38+70=108 38+70=108 True
5 1 214 63+40=103 63+40=103 True
5 1 215 86+62=148 86+62=159 False
5 1 216 22+65=087 22+65=087 True
5 1 217 41+17=058 41+17=067 False
5 1 218 68+88=156 68+88=155 False
5 1 219 96+70=166 96+70=164 False
5 1 220 99+29=128 99+29=127 False
5 1 221 83+39=122 83+39=122 True
5 1 222 26+55=081 26+55=081 True
5 1 223 53+70=123 53+70=123 True
5 1 224 94+12=106 94+12=107 False
5 1 225 00+37=037 00+37=036 False
5 1 226 36+94=130 36+94=120 False
5 1 227 40+58=098 40+58=108 False
5 1 228 19+80=099 19+80=098 False
5 1 229 49+44=093 49+44=102 False
5 1 230 70+27=097 70+27=095 False
5 1 231 52+80=132 52+80=132 True
5 1 232 77+90=167 77+90=165 False
5 1 233 13+92=105 13+92=107 False
5 1 234 59+09=068 59+09=077 False
5 1 235 33+55=088 33+55=097 False
5 1 236 85+16=101 85+16=101 True
5 1 237 25+65=090 25+65=092 False
5 1 238 46+20=066 46+20=065 False
5 1 239 29+52=081 29+52=081 True
5 1 240 32+36=068 32+36=068 True
5 1 241 47+08=055 47+08=055 True
5 1 242 21+84=105 21+84=107 False
5 1 243 24+45=069 24+45=068 False
5 1 244 29+15=044 29+15=042 False
5 1 245 83+03=086 83+03=097 False
5 1 246 83+36=119 83+36=115 False
5 1 247 58+95=153 58+95=152 False
5 1 248 76+79=155 76+79=155 True
5 1 249 63+30=093 63+30=093 True
5 1 250 38+24=062 38+24=061 False
5 1 251 19+46=065 19+46=064 False
5 1 252 99+66=165 99+66=164 False
5 1 253 95+73=168 95+73=167 False
5 1 254 65+27=092 65+27=091 False
5 1 255 91+83=174 91+83=177 False
5 2 0 65+49=114 65+49=112 False
5 2 1 03+08=011 03+08=000 False
5 2 2 67+81=148 67+81=158 False
5 2 3 47+23=070 47+23=071 False
5 2 4 43+91=134 43+91=137 False
5 2 5 41+67=108 41+67=107 False
5 2 6 02+33=035 02+33=037 False
5 2 7 64+84=148 64+84=158 False
5 2 8 81+64=145 81+64=147 False
5 2 9 80+11=091 80+11=091 True
5 2 10 78+01=079 78+01=089 False
5 2 11 89+18=107 89+18=107 True
5 2 12 45+52=097 45+52=107 False
5 2 13 35+30=065 35+30=063 False
5 2 14 53+32=085 53+32=097 False
5 2 15 49+90=139 49+90=149 False
5 2 16 41+37=078 41+37=087 False
5 2 17 35+14=049 35+14=058 False
5 2 18 92+50=142 92+50=143 False
5 2 19 37+60=097 37+60=095 False
5 2 20 91+61=152 91+61=151 False
5 2 21 80+77=157 80+77=155 False
5 2 22 66+24=090 66+24=090 True
5 2 23 81+07=088 81+07=097 False
5 2 24 85+59=144 85+59=142 False
5 2 25 19+69=088 19+69=087 False
5 2 26 91+44=135 91+44=137 False
5 2 27 25+29=054 25+29=052 False
5 2 28 27+08=035 27+08=035 True
5 2 29 66+14=080 66+14=071 False
5 2 30 95+11=106 95+11=107 False
5 2 31 13+97=110 13+97=110 True
5 2 32 94+40=134 94+40=133 False
5 2 33 74+31=105 74+31=107 False
5 2 34 49+00=049 49+00=050 False
5 2 35 59+18=077 59+18=076 False
5 2 36 07+65=072 07+65=071 False
5 2 37 83+55=138 83+55=137 False
5 2 38 49+80=129 49+80=139 False
5 2 39 64+17=081 64+17=081 True
5 2 40 48+83=131 48+83=131 True
5 2 41 95+44=139 95+44=137 False
5 2 42 71+26=097 71+26=096 False
5 2 43 06+74=080 06+74=071 False
5 2 44 34+24=058 34+24=058 True
5 2 45 59+71=130 59+71=130 True
5 2 46 68+32=100 68+32=101 False
5 2 47 38+81=119 38+81=119 True
5 2 48 29+56=085 29+56=084 False
5 2 49 54+55=109 54+55=117 False
5 2 50 31+27=058 31+27=057 False
5 2 51 97+89=186 97+89=195 False
5 2 52 48+09=057 48+09=057 True
5 2 53 86+76=162 86+76=154 False
5 2 54 82+59=141 82+59=141 True
5 2 55 01+67=068 01+67=077 False
5 2 56 26+06=032 26+06=035 False
5 2 57 22+46=068 22+46=068 True
5 2 58 85+16=101 85+16=101 True
5 2 59 29+08=037 29+08=037 True
5 2 60 73+94=167 73+94=167 True
5 2 61 19+62=081 19+62=081 True
5 2 62 86+62=148 86+62=159 False
5 2 63 38+99=137 38+99=137 True
5 2 64 64+25=089 64+25=097 False
5 2 65 61+72=133 61+72=137 False
5 2 66 78+88=166 78+88=165 False
5 2 67 43+66=109 43+66=108 False
5 2 68 69+35=104 69+35=103 False
5 2 69 33+77=110 33+77=110 True
5 2 70 37+37=074 37+37=075 False
5 2 71 87+54=141 87+54=141 True
5 2 72 68+90=158 68+90=157 False
5 2 73 83+44=127 83+44=127 True
5 2 74 41+09=050 41+09=050 True
5 2 75 13+48=061 13+48=061 True
5 2 76 01+41=042 01+41=050 False
5 2 77 19+74=093 19+74=092 False
5 2 78 15+05=020 15+05=012 False
5 2 79 55+46=101 55+46=100 False
5 2 80 68+33=101 68+33=101 True
5 2 81 44+40=084 44+40=083 False
5 2 82 88+03=091 88+03=091 True
5 2 83 81+79=160 81+79=150 False
5 2 84 18+98=116 18+98=107 False
5 2 85 70+64=134 70+64=137 False
5 2 86 26+44=070 26+44=071 False
5 2 87 98+87=185 98+87=185 True
5 2 88 18+74=092 18+74=092 True
5 2 89 50+68=118 50+68=117 False
5 2 90 13+51=064 13+51=067 False
5 2 91 90+89=179 90+89=179 True
5 2 92 47+78=125 47+78=125 True
5 2 93 81+57=138 81+57=147 False
5 2 94 34+47=081 34+47=081 True
5 2 95 94+23=117 94+23=117 True
5 2 96 07+70=077 07+70=075 False
5 2 97 56+33=089 56+33=099 False
5 2 98 33+04=037 33+04=037 True
5 2 99 26+09=035 26+09=035 True
5 2 100 14+92=106 14+92=107 False
5 2 101 78+54=132 78+54=132 True
5 2 102 36+76=112 36+76=114 False
5 2 103 17+47=064 17+47=065 False
5 2 104 28+18=046 28+18=047 False
5 2 105 78+54=132 78+54=132 True
5 2 106 84+72=156 84+72=157 False
5 2 107 00+44=044 00+44=057 False
5 2 108 50+41=091 50+41=092 False
5 2 109 87+88=175 87+88=175 True
5 2 110 11+66=077 11+66=076 False
5 2 111 80+60=140 80+60=140 True
5 2 112 78+76=154 78+76=154 True
5 2 113 24+74=098 24+74=098 True
5 2 114 88+48=136 88+48=135 False
5 2 115 38+31=069 38+31=079 False
5 2 116 29+27=056 29+27=055 False
5 2 117 08+45=053 08+45=052 False
5 2 118 28+13=041 28+13=031 False
5 2 119 53+99=152 53+99=152 True
5 2 120 47+92=139 47+92=139 True
5 2 121 76+21=097 76+21=095 False
5 2 122 53+96=149 53+96=159 False
5 2 123 93+91=184 93+91=183 False
5 2 124 97+33=130 97+33=121 False
5 2 125 67+78=145 67+78=145 True
5 2 126 58+05=063 58+05=072 False
5 2 127 00+16=016 00+16=015 False
5 2 128 80+19=099 80+19=098 False
5 2 129 98+22=120 98+22=111 False
5 2 130 09+62=071 09+62=071 True
5 2 131 06+23=029 06+23=029 True
5 2 132 32+99=131 32+99=121 False
5 2 133 17+02=019 17+02=010 False
5 2 134 64+35=099 64+35=098 False
5 2 135 35+83=118 35+83=117 False
5 2 136 71+36=107 71+36=107 True
5 2 137 75+06=081 75+06=081 True
5 2 138 88+95=183 88+95=182 False
5 2 139 19+98=117 19+98=115 False
5 2 140 28+89=117 28+89=115 False
5 2 141 33+11=044 33+11=047 False
5 2 142 34+49=083 34+49=082 False
5 2 143 90+35=125 90+35=127 False
5 2 144 22+90=112 22+90=112 True
5 2 145 98+89=187 98+89=195 False
5 2 146 88+47=135 88+47=135 True
5 2 147 30+86=116 30+86=115 False
5 2 148 31+48=079 31+48=088 False
5 2 149 39+21=060 39+21=050 False
5 2 150 19+17=036 19+17=035 False
5 2 151 27+60=087 27+60=085 False
5 2 152 12+16=028 12+16=028 True
5 2 153 51+75=126 51+75=127 False
5 2 154 10+74=084 10+74=087 False
5 2 155 42+63=105 42+63=107 False
5 2 156 40+14=054 40+14=053 False
5 2 157 23+93=116 23+93=117 False
5 2 158 85+26=111 85+26=111 True
5 2 159 28+46=074 28+46=074 True
5 2 160 28+33=061 28+33=051 False
5 2 161 43+30=073 43+30=075 False
5 2 162 89+72=161 89+72=151 False
5 2 163 52+21=073 52+21=077 False
5 2 164 21+54=075 21+54=077 False
5 2 165 69+13=082 69+13=081 False
5 2 166 07+60=067 07+60=075 False
5 2 167 63+83=146 63+83=157 False
5 2 168 80+69=149 80+69=150 False
5 2 169 27+28=055 27+28=056 False
5 2 170 42+31=073 42+31=077 False
5 2 171 51+99=150 51+99=150 True
5 2 172 28+75=103 28+75=102 False
5 2 173 38+57=095 38+57=095 True
5 2 174 83+16=099 83+16=108 False
5 2 175 92+94=186 92+94=187 False
5 2 176 55+75=130 55+75=132 False
5 2 177 59+51=110 59+51=110 True
5 2 178 33+09=042 33+09=031 False
5 2 179 53+13=066 53+13=077 False
5 2 180 05+70=075 05+70=077 False
5 2 181 12+20=032 12+20=037 False
5 2 182 11+49=060 11+49=060 True
5 2 183 63+45=108 63+45=107 False
5 2 184 92+23=115 92+23=117 False
5 2 185 82+45=127 82+45=127 True
5 2 186 23+41=064 23+41=067 False
5 2 187 64+26=090 64+26=099 False
5 2 188 91+24=115 91+24=117 False
5 2 189 20+32=052 20+32=052 True
5 2 190 83+21=104 83+21=107 False
5 2 191 07+20=027 07+20=025 False
5 2 192 94+14=108 94+14=107 False
5 2 193 96+89=185 96+89=194 False
5 2 194 13+08=021 13+08=010 False
5 2 195 32+05=037 32+05=037 True
5 2 196 09+51=060 09+51=060 True
5 2 197 26+29=055 26+29=055 True
5 2 198 49+65=114 49+65=112 False
5 2 199 32+66=098 32+66=098 True
5 2 200 41+08=049 41+08=059 False
5 2 201 26+79=105 26+79=105 True
5 2 202 29+91=120 29+91=110 False
5 2 203 51+00=051 51+00=050 False
5 2 204 61+60=121 61+60=120 False
5 2 205 45+78=123 45+78=122 False
5 2 206 56+16=072 56+16=075 False
5 2 207 66+68=134 66+68=134 True
5 2 208 32+16=048 32+16=058 False
5 2 209 84+49=133 84+49=133 True
5 2 210 45+09=054 45+09=052 False
5 2 211 96+78=174 96+78=174 True
5 2 212 10+02=012 10+02=007 False
5 2 213 36+60=096 36+60=094 False
5 2 214 44+36=080 44+36=089 False
5 2 215 12+86=098 12+86=098 True
5 2 216 94+54=148 94+54=147 False
5 2 217 64+73=137 64+73=137 True
5 2 218 73+10=083 73+10=083 True
5 2 219 14+62=076 14+62=077 False
5 2 220 25+22=047 25+22=057 False
5 2 221 94+22=116 94+22=117 False
5 2 222 41+76=117 41+76=117 True
5 2 223 38+46=084 38+46=084 True
5 2 224 71+72=143 71+72=137 False
5 2 225 74+79=153 74+79=143 False
5 2 226 99+67=166 99+67=165 False
5 2 227 78+71=149 78+71=149 True
5 2 228 23+19=042 23+19=031 False
5 2 229 51+65=116 51+65=117 False
5 2 230 94+86=180 94+86=180 True
5 2 231 09+79=088 09+79=087 False
5 2 232 69+39=108 69+39=108 True
5 2 233 84+13=097 84+13=097 True
5 2 234 36+59=095 36+59=094 False
5 2 235 87+47=134 87+47=135 False
5 2 236 50+00=050 50+00=050 True
5 2 237 76+96=172 76+96=164 False
5 2 238 12+18=030 12+18=020 False
5 2 239 99+95=194 99+95=193 False
5 2 240 22+00=022 22+00=017 False
5 2 241 96+18=114 96+18=105 False
5 2 242 51+20=071 51+20=070 False
5 2 243 66+81=147 66+81=155 False
5 2 244 78+18=096 78+18=095 False
5 2 245 09+78=087 09+78=086 False
5 2 246 24+20=044 24+20=047 False
5 2 247 76+13=089 76+13=099 False
5 2 248 05+10=015 05+10=007 False
5 2 249 97+14=111 97+14=112 False
5 2 250 92+38=130 92+38=121 False
5 2 251 77+13=090 77+13=091 False
5 2 252 70+19=089 70+19=098 False
5 2 253 90+45=135 90+45=137 False
5 2 254 50+09=059 50+09=068 False
5 2 255 78+06=084 78+06=084 True
5 3 0 03+25=028 03+25=027 False
5 3 1 48+43=091 48+43=091 True
5 3 2 39+47=086 39+47=095 False
5 3 3 48+19=067 48+19=067 True
5 3 4 07+22=029 07+22=029 True
5 3 5 73+68=141 73+68=131 False
5 3 6 14+56=070 14+56=070 True
5 3 7 96+95=191 96+95=191 True
5 3 8 96+28=124 96+28=125 False
5 3 9 82+05=087 82+05=097 False
5 3 10 27+94=121 27+94=112 False
5 3 11 87+86=173 87+86=164 False
5 3 12 00+68=068 00+68=077 False
5 3 13 11+37=048 11+37=058 False
5 3 14 95+93=188 95+93=192 False
5 3 15 75+82=157 75+82=157 True
5 3 16 41+71=112 41+71=110 False
5 3 17 60+14=074 60+14=077 False
5 3 18 77+77=154 77+77=155 False
5 3 19 31+84=115 31+84=117 False
5 3 20 31+57=088 31+57=097 False
5 3 21 27+87=114 27+87=115 False
5 3 22 31+89=120 31+89=129 False
5 3 23 22+18=040 22+18=030 False
5 3 24 38+25=063 38+25=062 False
5 3 25 64+54=118 64+54=127 False
5 3 26 85+60=145 85+60=142 False
5 3 27 14+71=085 14+71=087 False
5 3 28 06+16=022 06+16=014 False
5 3 29 78+61=139 78+61=139 True
5 3 30 65+75=140 65+75=132 False
5 3 31 13+83=096 13+83=097 False
5 3 32 75+49=124 75+49=122 False
5 3 33 05+78=083 05+78=082 False
5 3 34 66+55=121 66+55=121 True
5 3 35 03+05=008 03+05=007 False
5 3 36 69+99=168 69+99=165 False
5 3 37 52+82=134 52+82=137 False
5 3 38 45+97=142 45+97=142 True
5 3 39 66+17=083 66+17=085 False
5 3 40 36+17=053 36+17=055 False
5 3 41 92+74=166 92+74=167 False
5 3 42 48+44=092 48+44=091 False
5 3 43 34+17=051 34+17=051 True
5 3 44 56+11=067 56+11=075 False
5 3 45 77+23=100 77+23=100 True
5 3 46 10+11=021 10+11=010 False
5 3 47 32+65=097 32+65=097 True
5 3 48 53+49=102 53+49=102 True
5 3 49 68+86=154 68+86=154 True
5 3 50 52+94=146 52+94=147 False
5 3 51 97+71=168 97+71=167 False
5 3 52 05+37=042 05+37=041 False
5 3 53 58+75=133 58+75=132 False
5 3 54 06+24=030 06+24=020 False
5 3 55 15+44=059 15+44=068 False
5 3 56 90+49=139 90+49=139 True
5 3 57 50+37=087 50+37=095 False
5 3 58 88+61=149 88+61=159 False
5 3 59 21+57=078 21+57=087 False
5 3 60 24+85=109 24+85=112 False
5 3 61 01+66=067 01+66=075 False
5 3 62 50+46=096 50+46=095 False
5 3 63 76+65=141 76+65=131 False
5 3 64 23+74=097 23+74=097 True
5 3 65 76+16=092 76+16=094 False
5 3 66 06+08=014 06+08=015 False
5 3 67 69+25=094 69+25=092 False
5 3 68 15+23=038 15+23=037 False
5 3 69 41+02=043 41+02=053 False
5 3 70 16+66=082 16+66=075 False
5 3 71 59+94=153 59+94=152 False
5 3 72 32+88=120 32+88=120 True
5 3 73 46+21=067 46+21=075 False
5 3 74 57+28=085 57+28=085 True
5 3 75 00+31=031 00+31=030 False
5 3 76 77+07=084 77+07=085 False
5 3 77 28+70=098 28+70=095 False
5 3 78 05+61=066 05+61=077 False
5 3 79 22+09=031 22+09=021 False
5 3 80 08+94=102 08+94=102 True
5 3 81 40+11=051 40+11=050 False
5 3 82 10+48=058 10+48=067 False
5 3 83 27+56=083 27+56=084 False
5 3 84 42+16=058 42+16=068 False
5 3 85 69+43=112 69+43=112 True
5 3 86 57+69=126 57+69=125 False
5 3 87 18+86=104 18+86=105 False
5 3 88 86+80=166 86+80=164 False
5 3 89 30+85=115 30+85=117 False
5 3 90 77+66=143 77+66=144 False
5 3 91 39+64=103 39+64=102 False
5 3 92 76+61=137 76+61=135 False
5 3 93 42+61=103 42+61=108 False
5 3 94 07+30=037 07+30=035 False
5 3 95 35+93=128 35+93=127 False
5 3 96 40+90=130 40+90=130 True
5 3 97 08+91=099 08+91=099 True
5 3 98 62+34=096 62+34=097 False
5 3 99 86+49=135 86+49=135 True
5 3 100 73+23=096 73+23=097 False
5 3 101 87+35=122 87+35=122 True
5 3 102 35+31=066 35+31=067 False
5 3 103 07+13=020 07+13=010 False
5 3 104 39+41=080 39+41=080 True
5 3 105 44+63=107 44+63=107 True
5 3 106 94+66=160 94+66=159 False
5 3 107 49+54=103 49+54=102 False
5 3 108 79+46=125 79+46=124 False
5 3 109 53+12=065 53+12=067 False
5 3 110 60+92=152 60+92=157 False
5 3 111 25+60=085 25+60=083 False
5 3 112 64+53=117 64+53=117 True
5 3 113 41+02=043 41+02=053 False
5 3 114 00+97=097 00+97=095 False
5 3 115 12+52=064 12+52=067 False
5 3 116 39+50=089 39+50=090 False
5 3 117 87+21=108 87+21=107 False
5 3 118 04+99=103 04+99=102 False
5 3 119 19+75=094 19+75=092 False
5 3 120 90+05=095 90+05=097 False
5 3 121 54+39=093 54+39=092 False
5 3 122 29+26=055 29+26=054 False
5 3 123 82+95=177 82+95=177 True
5 3 124 55+09=064 55+09=072 False
5 3 125 02+62=064 02+62=077 False
5 3 126 68+30=098 68+30=095 False
5 3 127 99+16=115 99+16=114 False
5 3 128 63+11=074 63+11=077 False
5 3 129 42+92=134 42+92=137 False
5 3 130 99+16=115 99+16=114 False
5 3 131 50+31=081 50+31=081 True
5 3 132 23+46=069 23+46=079 False
5 3 133 45+73=118 45+73=127 False
5 3 134 89+77=166 89+77=165 False
5 3 135 45+78=123 45+78=122 False
5 3 136 96+60=156 96+60=155 False
5 3 137 74+61=135 74+61=137 False
5 3 138 87+01=088 87+01=097 False
5 3 139 63+88=151 63+88=151 True
5 3 140 59+72=131 59+72=131 True
5 3 141 17+96=113 17+96=114 False
5 3 142 89+77=166 89+77=165 False
5 3 143 24+69=093 24+69=092 False
5 3 144 75+83=158 75+83=151 False
5 3 145 50+54=104 50+54=107 False
5 3 146 93+47=140 93+47=130 False
5 3 147 20+55=075 20+55=075 True
5 3 148 91+79=170 91+79=169 False
5 3 149 15+13=028 15+13=027 False
5 3 150 86+09=095 86+09=094 False
5 3 151 29+58=087 29+58=086 False
5 3 152 01+29=030 01+29=020 False
5 3 153 65+48=113 65+48=112 False
5 3 154 96+45=141 96+45=131 False
5 3 155 58+69=127 58+69=127 True
5 3 156 84+43=127 84+43=127 True
5 3 157 90+38=128 90+38=127 False
5 3 158 39+97=136 39+97=135 False
5 3 159 74+84=158 74+84=158 True
5 3 160 86+22=108 86+22=108 True
5 3 161 01+86=087 01+86=096 False
5 3 162 81+63=144 81+63=147 False
5 3 163 80+94=174 80+94=167 False
5 3 164 44+42=086 44+42=087 False
5 3 165 72+60=132 72+60=132 True
5 3 166 28+07=035 28+07=035 True
5 3 167 69+54=123 69+54=122 False
5 3 168 68+77=145 68+77=145 True
5 3 169 90+16=106 90+16=105 False
5 3 170 64+50=114 64+50=113 False
5 3 171 46+88=134 46+88=135 False
5 3 172 55+99=154 55+99=153 False
5 3 173 31+97=128 31+97=127 False
5 3 174 79+28=107 79+28=107 True
5 3 175 81+43=124 81+43=127 False
5 3 176 41+15=056 41+15=057 False
5 3 177 38+77=115 38+77=115 True
5 3 178 25+06=031 25+06=030 False
5 3 179 01+93=094 01+93=097 False
5 3 180 97+22=119 97+22=119 True
5 3 181 71+84=155 71+84=157 False
5 3 182 26+36=062 26+36=065 False
5 3 183 60+92=152 60+92=157 False
5 3 184 02+94=096 02+94=097 False
5 3 185 31+58=089 31+58=099 False
5 3 186 70+52=122 70+52=123 False
5 3 187 19+42=061 19+42=061 True
5 3 188 95+73=168 95+73=167 False
5 3 189 21+25=046 21+25=057 False
5 3 190 13+58=071 13+58=071 True
5 3 191 62+28=090 62+28=090 True
5 3 192 38+14=052 38+14=051 False
5 3 193 66+75=141 66+75=131 False
5 3 194 24+59=083 24+59=082 False
5 3 195 97+66=163 97+66=154 False
5 3 196 76+70=146 76+70=144 False
5 3 197 08+40=048 08+40=058 False
5 3 198 84+00=084 84+00=097 False
5 3 199 54+73=127 54+73=127 True
5 3 200 16+88=104 16+88=105 False
5 3 201 99+47=146 99+47=145 False
5 3 202 31+95=126 31+95=127 False
5 3 203 01+79=080 01+79=070 False
5 3 204 03+68=071 03+68=071 True
5 3 205 10+05=015 10+05=007 False
5 3 206 98+90=188 98+90=185 False
5 3 207 58+53=111 58+53=112 False
5 3 208 34+87=121 34+87=121 True
5 3 209 07+31=038 07+31=037 False
5 3 210 59+08=067 59+08=076 False
5 3 211 51+38=089 51+38=099 False
5 3 212 62+62=124 62+62=127 False
5 3 213 80+32=112 80+32=112 True
5 3 214 69+16=085 69+16=084 False
5 3 215 01+17=018 01+17=017 False
5 3 216 74+41=115 74+41=117 False
5 3 217 20+89=109 20+89=118 False
5 3 218 53+50=103 53+50=103 True
5 3 219 82+85=167 82+85=167 True
5 3 220 34+47=081 34+47=081 True
5 3 221 34+45=079 34+45=088 False
5 3 222 77+34=111 77+34=111 True
5 3 223 56+33=089 56+33=099 False
5 3 224 97+56=153 97+56=144 False
5 3 225 29+06=035 29+06=034 False
5 3 226 78+96=174 78+96=174 True
5 3 227 28+65=093 28+65=092 False
5 3 228 61+64=125 61+64=127 False
5 3 229 32+64=096 32+64=097 False
5 3 230 98+32=130 98+32=121 False
5 3 231 25+35=060 25+35=062 False
5 3 232 05+08=013 05+08=003 False
5 3 233 05+26=031 05+26=030 False
5 3 234 84+71=155 84+71=157 False
5 3 235 33+10=043 33+10=047 False
5 3 236 98+35=133 98+35=132 False
5 3 237 68+98=166 68+98=165 False
5 3 238 03+63=066 03+63=077 False
5 3 239 12+96=108 12+96=108 True
5 3 240 02+81=083 02+81=087 False
5 3 241 83+13=096 83+13=097 False
5 3 242 55+92=147 55+92=147 True
5 3 243 96+09=105 96+09=105 True
5 3 244 61+08=069 61+08=079 False
5 3 245 39+75=114 39+75=112 False
5 3 246 40+74=114 40+74=113 False
5 3 247 39+80=119 39+80=118 False
5 3 248 57+95=152 57+95=152 True
5 3 249 92+97=189 92+97=199 False
5 3 250 33+03=036 33+03=037 False
5 3 251 74+92=166 74+92=167 False
5 3 252 99+09=108 99+09=108 True
5 3 253 98+10=108 98+10=107 False
5 3 254 46+77=123 46+77=125 False
5 3 255 85+78=163 85+78=152 False
5 4 0 41+21=062 41+21=061 False
5 4 1 49+13=062 49+13=061 False
5 4 2 59+07=066 59+07=075 False
5 4 3 31+11=042 31+11=040 False
5 4 4 74+16=090 74+16=090 True
5 4 5 43+38=081 43+38=081 True
5 4 6 08+67=075 08+67=075 True
5 4 7 31+66=097 31+66=097 True
5 4 8 10+31=041 10+31=040 False
5 4 9 34+59=093 34+59=092 False
5 4 10 78+42=120 78+42=121 False
5 4 11 13+41=054 13+41=057 False
5 4 12 97+89=186 97+89=195 False
5 4 13 15+62=077 15+62=077 True
5 4 14 39+36=075 39+36=075 True
5 4 15 21+25=046 21+25=057 False
5 4 16 74+56=130 74+56=129 False
5 4 17 85+47=132 85+47=132 True
5 4 18 47+32=079 47+32=089 False
5 4 19 37+66=103 37+66=105 False
5 4 20 16+29=045 16+29=045 True
5 4 21 86+77=163 86+77=155 False
5 4 22 80+07=087 80+07=095 False
5 4 23 87+05=092 87+05=092 True
5 4 24 58+16=074 58+16=074 True
5 4 25 52+79=131 52+79=131 True
5 4 26 91+08=099 91+08=098 False
5 4 27 47+78=125 47+78=125 True
5 4 28 86+96=182 86+96=184 False
5 4 29 90+22=112 90+22=113 False
5 4 30 31+18=049 31+18=059 False
5 4 31 86+15=101 86+15=101 True
5 4 32 15+95=110 15+95=102 False
5 4 33 42+11=053 42+11=057 False
5 4 34 65+99=164 65+99=163 False
5 4 35 89+29=118 89+29=117 False
5 4 36 35+11=046 35+11=057 False
5 4 37 71+41=112 71+41=110 False
5 4 38 16+24=040 16+24=040 True
5 4 39 77+82=159 77+82=159 True
5 4 40 55+89=144 55+89=143 False
5 4 41 17+88=105 17+88=106 False
5 4 42 54+72=126 54+72=127 False
5 4 43 34+98=132 34+98=132 True
5 4 44 09+97=106 09+97=105 False
5 4 45 91+07=098 91+07=097 False
5 4 46 55+94=149 55+94=157 False
5 4 47 22+58=080 22+58=080 True
5 4 48 91+37=128 91+37=127 False
5 4 49 16+10=026 16+10=025 False
5 4 50 96+32=128 96+32=128 True
5 4 51 35+75=110 35+75=112 False
5 4 52 88+73=161 88+73=151 False
5 4 53 35+18=053 35+18=052 False
5 4 54 33+10=043 33+10=047 False
5 4 55 08+50=058 08+50=065 False
5 4 56 22+62=084 22+62=087 False
5 4 57 26+37=063 26+37=065 False
5 4 58 80+27=107 80+27=107 True
5 4 59 68+28=096 68+28=095 False
5 4 60 48+03=051 48+03=051 True
5 4 61 40+18=058 40+18=057 False
5 4 62 16+59=075 16+59=075 True
5 4 63 02+19=021 02+19=010 False
5 4 64 01+09=010 01+09=009 False
5 4 65 62+68=130 62+68=130 True
5 4 66 09+71=080 09+71=070 False
5 4 67 00+58=058 00+58=067 False
5 4 68 16+45=061 16+45=061 True
5 4 69 24+98=122 24+98=122 True
5 4 70 47+92=139 47+92=139 True
5 4 71 94+84=178 94+84=177 False
5 4 72 21+32=053 21+32=057 False
5 4 73 29+82=111 29+82=112 False
5 4 74 32+79=111 32+79=111 True
5 4 75 13+98=111 13+98=112 False
5 4 76 41+94=135 41+94=137 False
5 4 77 51+84=135 51+84=137 False
5 4 78 42+05=047 42+05=057 False
5 4 79 39+03=042 39+03=032 False
5 4 80 02+92=094 02+92=097 False
5 4 81 99+81=180 99+81=170 False
5 4 82 32+68=100 32+68=100 True
5 4 83 52+17=069 52+17=079 False
5 4 84 56+58=114 56+58=114 True
5 4 85 21+48=069 21+48=079 False
5 4 86 61+71=132 61+71=131 False
5 4 87 17+01=018 17+01=017 False
5 4 88 68+23=091 68+23=091 True
5 4 89 00+37=037 00+37=036 False
5 4 90 94+88=182 94+88=182 True
5 4 91 06+31=037 06+31=035 False
5 4 92 27+18=045 27+18=045 True
5 4 93 41+81=122 41+81=121 False
5 4 94 15+86=101 15+86=101 True
5 4 95 36+87=123 36+87=125 False
5 4 96 17+37=054 17+37=055 False
5 4 97 13+86=099 13+86=108 False
5 4 98 29+69=098 29+69=097 False
5 4 99 31+99=130 31+99=129 False
5 4 100 47+29=076 47+29=075 False
5 4 101 08+81=089 08+81=099 False
5 4 102 72+82=154 72+82=157 False
5 4 103 46+91=137 46+91=137 True
5 4 104 70+35=105 70+35=107 False
5 4 105 90+55=145 90+55=147 False
5 4 106 99+99=198 99+99=197 False
5 4 107 60+97=157 60+97=155 False
5 4 108 03+40=043 03+40=057 False
5 4 109 35+49=084 35+49=092 False
5 4 110 32+02=034 32+02=037 False
5 4 111 70+18=088 70+18=095 False
5 4 112 99+05=104 99+05=103 False
5 4 113 78+73=151 78+73=141 False
5 4 114 03+02=005 03+02=007 False
5 4 115 50+14=064 50+14=063 False
5 4 116 62+02=064 62+02=077 False
5 4 117 16+74=090 16+74=090 True
5 4 118 68+65=133 68+65=132 False
5 4 119 74+81=155 74+81=157 False
5 4 120 37+48=085 37+48=085 True
5 4 121 63+04=067 63+04=077 False
5 4 122 06+62=068 06+62=079 False
5 4 123 95+75=170 95+75=162 False
5 4 124 92+37=129 92+37=129 True
5 4 125 81+32=113 81+32=117 False
5 4 126 53+28=081 53+28=081 True
5 4 127 52+42=094 52+42=097 False
5 4 128 66+97=163 66+97=164 False
5 4 129 00+48=048 00+48=057 False
5 4 130 65+32=097 65+32=098 False
5 4 131 60+89=149 60+89=150 False
5 4 132 71+61=132 71+61=131 False
5 4 133 98+50=148 98+50=147 False
5 4 134 90+96=186 90+96=185 False
5 4 135 02+96=098 02+96=098 True
5 4 136 62+75=137 62+75=137 True
5 4 137 41+28=069 41+28=079 False
5 4 138 95+79=174 95+79=173 False
5 4 139 48+41=089 48+41=099 False
5 4 140 87+95=182 87+95=172 False
5 4 141 75+38=113 75+38=112 False
5 4 142 31+55=086 31+55=097 False
5 4 143 54+63=117 54+63=117 True
5 4 144 75+82=157 75+82=157 True
5 4 145 46+45=091 46+45=091 True
5 4 146 13+08=021 13+08=010 False
5 4 147 77+97=174 77+97=175 False
5 4 148 37+35=072 37+35=071 False
5 4 149 21+89=110 21+89=110 True
5 4 150 58+51=109 58+51=119 False
5 4 151 91+48=139 91+48=149 False
5 4 152 33+23=056 33+23=057 False
5 4 153 80+96=176 80+96=165 False
5 4 154 78+02=080 78+02=081 False
5 4 155 38+95=133 38+95=132 False
5 4 156 99+25=124 99+25=123 False
5 4 157 30+76=106 30+76=105 False
5 4 158 42+40=082 42+40=083 False
5 4 159 85+58=143 85+58=142 False
5 4 160 44+46=090 44+46=099 False
5 4 161 06+41=047 06+41=057 False
5 4 162 65+90=155 65+90=157 False
5 4 163 43+83=126 43+83=137 False
5 4 164 36+61=097 36+61=095 False
5 4 165 61+51=112 61+51=110 False
5 4 166 38+09=047 38+09=047 True
5 4 167 21+97=118 21+97=117 False
5 4 168 83+30=113 83+30=113 True
5 4 169 11+79=090 11+79=090 True
5 4 170 14+29=043 14+29=042 False
5 4 171 21+11=032 21+11=030 False
5 4 172 43+53=096 43+53=107 False
5 4 173 02+58=060 02+58=060 True
5 4 174 78+82=160 78+82=151 False
5 4 175 91+11=102 91+11=100 False
5 4 176 58+54=112 58+54=112 True
5 4 177 00+15=015 00+15=007 False
5 4 178 83+51=134 83+51=137 False
5 4 179 44+72=116 44+72=117 False
5 4 180 71+20=091 71+20=090 False
5 4 181 24+99=123 24+99=123 True
5 4 182 46+30=076 46+30=075 False
5 4 183 08+67=075 08+67=075 True
5 4 184 47+42=089 47+42=099 False
5 4 185 95+67=162 95+67=152 False
5 4 186 40+56=096 40+56=095 False
5 4 187 17+95=112 17+95=112 True
5 4 188 94+66=160 94+66=159 False
5 4 189 14+58=072 14+58=071 False
5 4 190 56+05=061 56+05=061 True
5 4 191 70+01=071 70+01=071 True
5 4 192 97+59=156 97+59=155 False
5 4 193 94+67=161 94+67=151 False
5 4 194 13+41=054 13+41=057 False
5 4 195 85+15=100 85+15=102 False
5 4 196 48+53=101 48+53=101 True
5 4 197 62+75=137 62+75=137 True
5 4 198 87+47=134 87+47=135 False
5 4 199 31+88=119 31+88=118 False
5 4 200 97+16=113 97+16=105 False
5 4 201 48+45=093 48+45=092 False
5 4 202 99+00=099 99+00=098 False
5 4 203 15+01=016 15+01=017 False
5 4 204 28+96=124 28+96=124 True
5 4 205 20+11=031 20+11=030 False
5 4 206 07+56=063 07+56=064 False
5 4 207 06+08=014 06+08=015 False
5 4 208 45+46=091 45+46=090 False
5 4 209 48+85=133 48+85=132 False
5 4 210 62+14=076 62+14=077 False
5 4 211 82+31=113 82+31=117 False
5 4 212 85+88=173 85+88=173 True
5 4 213 77+08=085 77+08=085 True
5 4 214 16+64=080 16+64=071 False
5 4 215 00+27=027 00+27=026 False
5 4 216 36+75=111 36+75=111 True
5 4 217 38+38=076 38+38=077 False
5 4 218 88+32=120 88+32=111 False
5 4 219 09+88=097 09+88=095 False
5 4 220 96+87=183 96+87=184 False
5 4 221 71+29=100 71+29=100 True
5 4 222 99+13=112 99+13=112 True
5 4 223 03+13=016 03+13=017 False
5 4 224 67+23=090 67+23=091 False
5 4 225 15+98=113 15+98=112 False
5 4 226 10+08=018 10+08=017 False
5 4 227 46+24=070 46+24=071 False
5 4 228 55+63=118 55+63=127 False
5 4 229 28+06=034 28+06=034 True
5 4 230 43+87=130 43+87=139 False
5 4 231 34+05=039 34+05=038 False
2024-12-17 09:44:12,301 - root - WARNING - The method `test_one_epoch` is not implemented. Please override this method in a subclass or use a custom callback.
2024-12-17 09:44:12,302 - root - INFO - ====================================================== Starting Train Epoch: 6/9 ======================================================
2024-12-17 09:44:12,303 - root - INFO - Learning rates for each parameter group: 0.00148872833543853430, 0.00148872833543853430
0%| | 0/28 [00:00<?, ?it/s]
Epoch: 6, Step: 1: 0%| | 0/28 [00:00<?, ?it/s]
Epoch: 6, Step: 1: 0%| | 0/28 [00:00<?, ?it/s, total_batch_loss=155.79370, average_batch_loss=0.60857, average_batch_perplexity=1.83780, lr=0.001488728, 0.001488728]
Epoch: 6, Step: 2: 0%| | 0/28 [00:00<?, ?it/s, total_batch_loss=155.79370, average_batch_loss=0.60857, average_batch_perplexity=1.83780, lr=0.001488728, 0.001488728]
Epoch: 6, Step: 2: 0%| | 0/28 [00:00<?, ?it/s, total_batch_loss=147.72687, average_batch_loss=0.57706, average_batch_perplexity=1.78079, lr=0.001483477, 0.001483477]
Epoch: 6, Step: 2: 7%|▋ | 2/28 [00:00<00:02, 12.46it/s, total_batch_loss=147.72687, average_batch_loss=0.57706, average_batch_perplexity=1.78079, lr=0.001483477, 0.001483477]
Epoch: 6, Step: 3: 7%|▋ | 2/28 [00:00<00:02, 12.46it/s, total_batch_loss=147.72687, average_batch_loss=0.57706, average_batch_perplexity=1.78079, lr=0.001483477, 0.001483477]
Epoch: 6, Step: 3: 7%|▋ | 2/28 [00:00<00:02, 12.46it/s, total_batch_loss=148.80716, average_batch_loss=0.58128, average_batch_perplexity=1.78832, lr=0.001478281, 0.001478281]
Epoch: 6, Step: 4: 7%|▋ | 2/28 [00:00<00:02, 12.46it/s, total_batch_loss=148.80716, average_batch_loss=0.58128, average_batch_perplexity=1.78832, lr=0.001478281, 0.001478281]
Epoch: 6, Step: 4: 7%|▋ | 2/28 [00:00<00:02, 12.46it/s, total_batch_loss=153.21822, average_batch_loss=0.59851, average_batch_perplexity=1.81940, lr=0.001473139, 0.001473139]
Epoch: 6, Step: 4: 14%|█▍ | 4/28 [00:00<00:01, 12.63it/s, total_batch_loss=153.21822, average_batch_loss=0.59851, average_batch_perplexity=1.81940, lr=0.001473139, 0.001473139]
Epoch: 6, Step: 5: 14%|█▍ | 4/28 [00:00<00:01, 12.63it/s, total_batch_loss=153.21822, average_batch_loss=0.59851, average_batch_perplexity=1.81940, lr=0.001473139, 0.001473139]
Epoch: 6, Step: 5: 14%|█▍ | 4/28 [00:00<00:01, 12.63it/s, total_batch_loss=143.07899, average_batch_loss=0.55890, average_batch_perplexity=1.74875, lr=0.001468051, 0.001468051]
Epoch: 6, Step: 6: 14%|█▍ | 4/28 [00:00<00:01, 12.63it/s, total_batch_loss=143.07899, average_batch_loss=0.55890, average_batch_perplexity=1.74875, lr=0.001468051, 0.001468051]
Epoch: 6, Step: 6: 14%|█▍ | 4/28 [00:00<00:01, 12.63it/s, total_batch_loss=146.54678, average_batch_loss=0.57245, average_batch_perplexity=1.77260, lr=0.001463014, 0.001463014]
Epoch: 6, Step: 6: 21%|██▏ | 6/28 [00:00<00:01, 12.60it/s, total_batch_loss=146.54678, average_batch_loss=0.57245, average_batch_perplexity=1.77260, lr=0.001463014, 0.001463014]
Epoch: 6, Step: 7: 21%|██▏ | 6/28 [00:00<00:01, 12.60it/s, total_batch_loss=146.54678, average_batch_loss=0.57245, average_batch_perplexity=1.77260, lr=0.001463014, 0.001463014]
Epoch: 6, Step: 7: 21%|██▏ | 6/28 [00:00<00:01, 12.60it/s, total_batch_loss=133.86508, average_batch_loss=0.52291, average_batch_perplexity=1.68693, lr=0.001458030, 0.001458030]
Epoch: 6, Step: 8: 21%|██▏ | 6/28 [00:00<00:01, 12.60it/s, total_batch_loss=133.86508, average_batch_loss=0.52291, average_batch_perplexity=1.68693, lr=0.001458030, 0.001458030]
Epoch: 6, Step: 8: 21%|██▏ | 6/28 [00:00<00:01, 12.60it/s, total_batch_loss=134.05029, average_batch_loss=0.52363, average_batch_perplexity=1.68815, lr=0.001453095, 0.001453095]
Epoch: 6, Step: 8: 29%|██▊ | 8/28 [00:00<00:01, 12.69it/s, total_batch_loss=134.05029, average_batch_loss=0.52363, average_batch_perplexity=1.68815, lr=0.001453095, 0.001453095]
Epoch: 6, Step: 9: 29%|██▊ | 8/28 [00:00<00:01, 12.69it/s, total_batch_loss=134.05029, average_batch_loss=0.52363, average_batch_perplexity=1.68815, lr=0.001453095, 0.001453095]
Epoch: 6, Step: 9: 29%|██▊ | 8/28 [00:00<00:01, 12.69it/s, total_batch_loss=124.76402, average_batch_loss=0.48736, average_batch_perplexity=1.62801, lr=0.001448211, 0.001448211]
Epoch: 6, Step: 10: 29%|██▊ | 8/28 [00:00<00:01, 12.69it/s, total_batch_loss=124.76402, average_batch_loss=0.48736, average_batch_perplexity=1.62801, lr=0.001448211, 0.001448211]
Epoch: 6, Step: 10: 29%|██▊ | 8/28 [00:00<00:01, 12.69it/s, total_batch_loss=134.03320, average_batch_loss=0.52357, average_batch_perplexity=1.68804, lr=0.001443376, 0.001443376]
Epoch: 6, Step: 10: 36%|███▌ | 10/28 [00:00<00:01, 12.57it/s, total_batch_loss=134.03320, average_batch_loss=0.52357, average_batch_perplexity=1.68804, lr=0.001443376, 0.001443376]
Epoch: 6, Step: 11: 36%|███▌ | 10/28 [00:00<00:01, 12.57it/s, total_batch_loss=134.03320, average_batch_loss=0.52357, average_batch_perplexity=1.68804, lr=0.001443376, 0.001443376]
Epoch: 6, Step: 11: 36%|███▌ | 10/28 [00:00<00:01, 12.57it/s, total_batch_loss=119.91843, average_batch_loss=0.46843, average_batch_perplexity=1.59749, lr=0.001438588, 0.001438588]
Epoch: 6, Step: 12: 36%|███▌ | 10/28 [00:00<00:01, 12.57it/s, total_batch_loss=119.91843, average_batch_loss=0.46843, average_batch_perplexity=1.59749, lr=0.001438588, 0.001438588]
Epoch: 6, Step: 12: 36%|███▌ | 10/28 [00:00<00:01, 12.57it/s, total_batch_loss=114.41341, average_batch_loss=0.44693, average_batch_perplexity=1.56350, lr=0.001433848, 0.001433848]
Epoch: 6, Step: 12: 43%|████▎ | 12/28 [00:00<00:01, 12.56it/s, total_batch_loss=114.41341, average_batch_loss=0.44693, average_batch_perplexity=1.56350, lr=0.001433848, 0.001433848]
Epoch: 6, Step: 13: 43%|████▎ | 12/28 [00:01<00:01, 12.56it/s, total_batch_loss=114.41341, average_batch_loss=0.44693, average_batch_perplexity=1.56350, lr=0.001433848, 0.001433848]
Epoch: 6, Step: 13: 43%|████▎ | 12/28 [00:01<00:01, 12.56it/s, total_batch_loss=121.07416, average_batch_loss=0.47295, average_batch_perplexity=1.60471, lr=0.001429155, 0.001429155]
Epoch: 6, Step: 14: 43%|████▎ | 12/28 [00:01<00:01, 12.56it/s, total_batch_loss=121.07416, average_batch_loss=0.47295, average_batch_perplexity=1.60471, lr=0.001429155, 0.001429155]
Epoch: 6, Step: 14: 43%|████▎ | 12/28 [00:01<00:01, 12.56it/s, total_batch_loss=121.88602, average_batch_loss=0.47612, average_batch_perplexity=1.60981, lr=0.001424507, 0.001424507]
Epoch: 6, Step: 14: 50%|█████ | 14/28 [00:01<00:01, 12.50it/s, total_batch_loss=121.88602, average_batch_loss=0.47612, average_batch_perplexity=1.60981, lr=0.001424507, 0.001424507]
Epoch: 6, Step: 15: 50%|█████ | 14/28 [00:01<00:01, 12.50it/s, total_batch_loss=121.88602, average_batch_loss=0.47612, average_batch_perplexity=1.60981, lr=0.001424507, 0.001424507]
Epoch: 6, Step: 15: 50%|█████ | 14/28 [00:01<00:01, 12.50it/s, total_batch_loss=109.31966, average_batch_loss=0.42703, average_batch_perplexity=1.53270, lr=0.001419905, 0.001419905]
Epoch: 6, Step: 16: 50%|█████ | 14/28 [00:01<00:01, 12.50it/s, total_batch_loss=109.31966, average_batch_loss=0.42703, average_batch_perplexity=1.53270, lr=0.001419905, 0.001419905]
Epoch: 6, Step: 16: 50%|█████ | 14/28 [00:01<00:01, 12.50it/s, total_batch_loss=112.27247, average_batch_loss=0.43856, average_batch_perplexity=1.55048, lr=0.001415346, 0.001415346]
Epoch: 6, Step: 16: 57%|█████▋ | 16/28 [00:01<00:00, 12.38it/s, total_batch_loss=112.27247, average_batch_loss=0.43856, average_batch_perplexity=1.55048, lr=0.001415346, 0.001415346]
Epoch: 6, Step: 17: 57%|█████▋ | 16/28 [00:01<00:00, 12.38it/s, total_batch_loss=112.27247, average_batch_loss=0.43856, average_batch_perplexity=1.55048, lr=0.001415346, 0.001415346]
Epoch: 6, Step: 17: 57%|█████▋ | 16/28 [00:01<00:00, 12.38it/s, total_batch_loss=102.06506, average_batch_loss=0.39869, average_batch_perplexity=1.48987, lr=0.001410832, 0.001410832]
Epoch: 6, Step: 18: 57%|█████▋ | 16/28 [00:01<00:00, 12.38it/s, total_batch_loss=102.06506, average_batch_loss=0.39869, average_batch_perplexity=1.48987, lr=0.001410832, 0.001410832]
Epoch: 6, Step: 18: 57%|█████▋ | 16/28 [00:01<00:00, 12.38it/s, total_batch_loss=110.41208, average_batch_loss=0.43130, average_batch_perplexity=1.53925, lr=0.001406360, 0.001406360]
Epoch: 6, Step: 18: 64%|██████▍ | 18/28 [00:01<00:00, 12.50it/s, total_batch_loss=110.41208, average_batch_loss=0.43130, average_batch_perplexity=1.53925, lr=0.001406360, 0.001406360]
Epoch: 6, Step: 19: 64%|██████▍ | 18/28 [00:01<00:00, 12.50it/s, total_batch_loss=110.41208, average_batch_loss=0.43130, average_batch_perplexity=1.53925, lr=0.001406360, 0.001406360]
Epoch: 6, Step: 19: 64%|██████▍ | 18/28 [00:01<00:00, 12.50it/s, total_batch_loss=100.90804, average_batch_loss=0.39417, average_batch_perplexity=1.48316, lr=0.001401930, 0.001401930]
Epoch: 6, Step: 20: 64%|██████▍ | 18/28 [00:01<00:00, 12.50it/s, total_batch_loss=100.90804, average_batch_loss=0.39417, average_batch_perplexity=1.48316, lr=0.001401930, 0.001401930]
Epoch: 6, Step: 20: 64%|██████▍ | 18/28 [00:01<00:00, 12.50it/s, total_batch_loss=99.20811, average_batch_loss=0.38753, average_batch_perplexity=1.47334, lr=0.001397542, 0.001397542]
Epoch: 6, Step: 20: 71%|███████▏ | 20/28 [00:01<00:00, 12.42it/s, total_batch_loss=99.20811, average_batch_loss=0.38753, average_batch_perplexity=1.47334, lr=0.001397542, 0.001397542]
Epoch: 6, Step: 21: 71%|███████▏ | 20/28 [00:01<00:00, 12.42it/s, total_batch_loss=99.20811, average_batch_loss=0.38753, average_batch_perplexity=1.47334, lr=0.001397542, 0.001397542]
Epoch: 6, Step: 21: 71%|███████▏ | 20/28 [00:01<00:00, 12.42it/s, total_batch_loss=98.92679, average_batch_loss=0.38643, average_batch_perplexity=1.47172, lr=0.001393196, 0.001393196]
Epoch: 6, Step: 22: 71%|███████▏ | 20/28 [00:01<00:00, 12.42it/s, total_batch_loss=98.92679, average_batch_loss=0.38643, average_batch_perplexity=1.47172, lr=0.001393196, 0.001393196]
Epoch: 6, Step: 22: 71%|███████▏ | 20/28 [00:01<00:00, 12.42it/s, total_batch_loss=103.29178, average_batch_loss=0.40348, average_batch_perplexity=1.49703, lr=0.001388889, 0.001388889]
Epoch: 6, Step: 22: 79%|███████▊ | 22/28 [00:01<00:00, 12.46it/s, total_batch_loss=103.29178, average_batch_loss=0.40348, average_batch_perplexity=1.49703, lr=0.001388889, 0.001388889]
Epoch: 6, Step: 23: 79%|███████▊ | 22/28 [00:01<00:00, 12.46it/s, total_batch_loss=103.29178, average_batch_loss=0.40348, average_batch_perplexity=1.49703, lr=0.001388889, 0.001388889]
Epoch: 6, Step: 23: 79%|███████▊ | 22/28 [00:01<00:00, 12.46it/s, total_batch_loss=92.24723, average_batch_loss=0.36034, average_batch_perplexity=1.43382, lr=0.001384622, 0.001384622]
Epoch: 6, Step: 24: 79%|███████▊ | 22/28 [00:01<00:00, 12.46it/s, total_batch_loss=92.24723, average_batch_loss=0.36034, average_batch_perplexity=1.43382, lr=0.001384622, 0.001384622]
Epoch: 6, Step: 24: 79%|███████▊ | 22/28 [00:01<00:00, 12.46it/s, total_batch_loss=108.63124, average_batch_loss=0.42434, average_batch_perplexity=1.52858, lr=0.001380394, 0.001380394]
Epoch: 6, Step: 24: 86%|████████▌ | 24/28 [00:01<00:00, 12.54it/s, total_batch_loss=108.63124, average_batch_loss=0.42434, average_batch_perplexity=1.52858, lr=0.001380394, 0.001380394]
Epoch: 6, Step: 25: 86%|████████▌ | 24/28 [00:01<00:00, 12.54it/s, total_batch_loss=108.63124, average_batch_loss=0.42434, average_batch_perplexity=1.52858, lr=0.001380394, 0.001380394]
Epoch: 6, Step: 25: 86%|████████▌ | 24/28 [00:01<00:00, 12.54it/s, total_batch_loss=102.35754, average_batch_loss=0.39983, average_batch_perplexity=1.49158, lr=0.001376205, 0.001376205]
Epoch: 6, Step: 26: 86%|████████▌ | 24/28 [00:02<00:00, 12.54it/s, total_batch_loss=102.35754, average_batch_loss=0.39983, average_batch_perplexity=1.49158, lr=0.001376205, 0.001376205]
Epoch: 6, Step: 26: 86%|████████▌ | 24/28 [00:02<00:00, 12.54it/s, total_batch_loss=97.85474, average_batch_loss=0.38225, average_batch_perplexity=1.46557, lr=0.001372053, 0.001372053]
Epoch: 6, Step: 26: 93%|█████████▎| 26/28 [00:02<00:00, 12.51it/s, total_batch_loss=97.85474, average_batch_loss=0.38225, average_batch_perplexity=1.46557, lr=0.001372053, 0.001372053]
Epoch: 6, Step: 27: 93%|█████████▎| 26/28 [00:02<00:00, 12.51it/s, total_batch_loss=97.85474, average_batch_loss=0.38225, average_batch_perplexity=1.46557, lr=0.001372053, 0.001372053]
Epoch: 6, Step: 27: 93%|█████████▎| 26/28 [00:02<00:00, 12.51it/s, total_batch_loss=99.34847, average_batch_loss=0.38808, average_batch_perplexity=1.47415, lr=0.001367939, 0.001367939]
Epoch: 6, Step: 28: 93%|█████████▎| 26/28 [00:02<00:00, 12.51it/s, total_batch_loss=99.34847, average_batch_loss=0.38808, average_batch_perplexity=1.47415, lr=0.001367939, 0.001367939]
Epoch: 6, Step: 28: 93%|█████████▎| 26/28 [00:02<00:00, 12.51it/s, total_batch_loss=30.92808, average_batch_loss=0.35146, average_batch_perplexity=1.42113, lr=0.001363862, 0.001363862]
Epoch: 6, Step: 28: 100%|██████████| 28/28 [00:02<00:00, 13.60it/s, total_batch_loss=30.92808, average_batch_loss=0.35146, average_batch_perplexity=1.42113, lr=0.001363862, 0.001363862]
2024-12-17 09:44:14,502 - root - INFO - Total Samples: 7000
2024-12-17 09:44:14,503 - root - INFO - Total Batches: 28
2024-12-17 09:44:14,503 - root - INFO - Average Epoch Train Loss: 0.46671
2024-12-17 09:44:14,504 - root - INFO - Average Epoch Train Perplexity: 1.59473
2024-12-17 09:44:14,504 - root - INFO -
2024-12-17 09:44:14,505 - root - INFO - ====================================================== Starting Valid Epoch: 6/9 ======================================================
0%| | 0/8 [00:00<?, ?it/s]
Epoch: 6, Step: 1: 0%| | 0/8 [00:00<?, ?it/s]
Epoch: 6, Step: 1: 0%| | 0/8 [00:00<?, ?it/s, total_batch_loss=90.70788, average_batch_loss=0.35433, average_batch_perplexity=1.42522]
Epoch: 6, Step: 2: 0%| | 0/8 [00:00<?, ?it/s, total_batch_loss=90.70788, average_batch_loss=0.35433, average_batch_perplexity=1.42522]
Epoch: 6, Step: 2: 0%| | 0/8 [00:00<?, ?it/s, total_batch_loss=93.68127, average_batch_loss=0.36594, average_batch_perplexity=1.44187]
Epoch: 6, Step: 3: 0%| | 0/8 [00:00<?, ?it/s, total_batch_loss=93.68127, average_batch_loss=0.36594, average_batch_perplexity=1.44187]
Epoch: 6, Step: 3: 0%| | 0/8 [00:00<?, ?it/s, total_batch_loss=89.34997, average_batch_loss=0.34902, average_batch_perplexity=1.41768]
Epoch: 6, Step: 3: 38%|███▊ | 3/8 [00:00<00:00, 22.47it/s, total_batch_loss=89.34997, average_batch_loss=0.34902, average_batch_perplexity=1.41768]
Epoch: 6, Step: 4: 38%|███▊ | 3/8 [00:00<00:00, 22.47it/s, total_batch_loss=89.34997, average_batch_loss=0.34902, average_batch_perplexity=1.41768]
Epoch: 6, Step: 4: 38%|███▊ | 3/8 [00:00<00:00, 22.47it/s, total_batch_loss=89.19657, average_batch_loss=0.34842, average_batch_perplexity=1.41683]
Epoch: 6, Step: 5: 38%|███▊ | 3/8 [00:00<00:00, 22.47it/s, total_batch_loss=89.19657, average_batch_loss=0.34842, average_batch_perplexity=1.41683]
Epoch: 6, Step: 5: 38%|███▊ | 3/8 [00:00<00:00, 22.47it/s, total_batch_loss=97.31064, average_batch_loss=0.38012, average_batch_perplexity=1.46246]
Epoch: 6, Step: 6: 38%|███▊ | 3/8 [00:00<00:00, 22.47it/s, total_batch_loss=97.31064, average_batch_loss=0.38012, average_batch_perplexity=1.46246]
Epoch: 6, Step: 6: 38%|███▊ | 3/8 [00:00<00:00, 22.47it/s, total_batch_loss=90.34011, average_batch_loss=0.35289, average_batch_perplexity=1.42318]
Epoch: 6, Step: 6: 75%|███████▌ | 6/8 [00:00<00:00, 23.36it/s, total_batch_loss=90.34011, average_batch_loss=0.35289, average_batch_perplexity=1.42318]
Epoch: 6, Step: 7: 75%|███████▌ | 6/8 [00:00<00:00, 23.36it/s, total_batch_loss=90.34011, average_batch_loss=0.35289, average_batch_perplexity=1.42318]
Epoch: 6, Step: 7: 75%|███████▌ | 6/8 [00:00<00:00, 23.36it/s, total_batch_loss=90.64169, average_batch_loss=0.35407, average_batch_perplexity=1.42485]
Epoch: 6, Step: 8: 75%|███████▌ | 6/8 [00:00<00:00, 23.36it/s, total_batch_loss=90.64169, average_batch_loss=0.35407, average_batch_perplexity=1.42485]
Epoch: 6, Step: 8: 75%|███████▌ | 6/8 [00:00<00:00, 23.36it/s, total_batch_loss=69.68761, average_batch_loss=0.33504, average_batch_perplexity=1.39799]
2024-12-17 09:44:14,846 - root - INFO - Total Samples: 2000
2024-12-17 09:44:14,846 - root - INFO - Total Batches: 8
2024-12-17 09:44:14,847 - root - INFO - Average Epoch Valid Loss: 0.35546
2024-12-17 09:44:14,847 - root - INFO - Average Epoch Valid Perplexity: 1.42683
2024-12-17 09:44:14,848 - root - INFO -
Evaluating and Generation.: 0%| | 0/4 [00:00<?, ?it/s]
Evaluating and Generation.: 0%| | 0/4 [00:00<?, ?it/s, accuracy: 0.4414]
Evaluating and Generation.: 0%| | 0/4 [00:00<?, ?it/s, accuracy: 0.4414]
Evaluating and Generation.: 50%|█████ | 2/4 [00:00<00:00, 10.58it/s, accuracy: 0.4414]
Evaluating and Generation.: 50%|█████ | 2/4 [00:00<00:00, 10.58it/s, accuracy: 0.4922]
Evaluating and Generation.: 50%|█████ | 2/4 [00:00<00:00, 10.58it/s, accuracy: 0.4224]
Evaluating and Generation.: 100%|██████████| 4/4 [00:00<00:00, 10.87it/s, accuracy: 0.4224]
2024-12-17 09:44:15,223 - root - INFO - Correct/Total Samples: 450/1000
2024-12-17 09:44:15,224 - root - INFO - Eval Accuracy: 0.45
2024-12-17 09:44:15,242 - root - INFO -
epoch batch_index sample_index equation generated correct
6 1 0 13+48=061 13+48=071 False
6 1 1 16+55=071 16+55=071 True
6 1 2 79+34=113 79+34=113 True
6 1 3 35+44=079 35+44=089 False
6 1 4 16+50=066 16+50=076 False
6 1 5 28+47=075 28+47=075 True
6 1 6 00+74=074 00+74=073 False
6 1 7 15+20=035 15+20=035 True
6 1 8 72+60=132 72+60=132 True
6 1 9 63+68=131 63+68=121 False
6 1 10 29+45=074 29+45=074 True
6 1 11 34+60=094 34+60=094 True
6 1 12 53+70=123 53+70=123 True
6 1 13 70+50=120 70+50=110 False
6 1 14 11+84=095 11+84=095 True
6 1 15 42+71=113 42+71=113 True
6 1 16 98+22=120 98+22=110 False
6 1 17 02+02=004 02+02=013 False
6 1 18 15+85=100 15+85=100 True
6 1 19 21+78=099 21+78=099 True
6 1 20 61+79=140 61+79=130 False
6 1 21 25+99=124 25+99=114 False
6 1 22 09+85=094 09+85=094 True
6 1 23 60+91=151 60+91=141 False
6 1 24 35+30=065 35+30=075 False
6 1 25 24+51=075 24+51=075 True
6 1 26 93+91=184 93+91=174 False
6 1 27 39+96=135 39+96=135 True
6 1 28 64+35=099 64+35=100 False
6 1 29 36+22=058 36+22=068 False
6 1 30 68+45=113 68+45=113 True
6 1 31 16+84=100 16+84=100 True
6 1 32 91+52=143 91+52=132 False
6 1 33 97+36=133 97+36=133 True
6 1 34 27+37=064 27+37=075 False
6 1 35 99+82=181 99+82=181 True
6 1 36 03+42=045 03+42=055 False
6 1 37 18+38=056 18+38=066 False
6 1 38 32+20=052 32+20=052 True
6 1 39 38+13=051 38+13=051 True
6 1 40 68+42=110 68+42=110 True
6 1 41 64+00=064 64+00=074 False
6 1 42 48+94=142 48+94=132 False
6 1 43 58+36=094 58+36=095 False
6 1 44 41+22=063 41+22=063 True
6 1 45 23+58=081 23+58=081 True
6 1 46 67+46=113 67+46=113 True
6 1 47 40+78=118 40+78=118 True
6 1 48 90+38=128 90+38=128 True
6 1 49 89+52=141 89+52=141 True
6 1 50 37+77=114 37+77=115 False
6 1 51 29+76=105 29+76=105 True
6 1 52 42+90=132 42+90=131 False
6 1 53 45+82=127 45+82=128 False
6 1 54 35+95=130 35+95=120 False
6 1 55 92+98=190 92+98=180 False
6 1 56 73+91=164 73+91=164 True
6 1 57 53+97=150 53+97=140 False
6 1 58 98+69=167 98+69=167 True
6 1 59 20+46=066 20+46=076 False
6 1 60 48+69=117 48+69=116 False
6 1 61 62+31=093 62+31=092 False
6 1 62 80+59=139 80+59=139 True
6 1 63 58+12=070 58+12=070 True
6 1 64 08+96=104 08+96=105 False
6 1 65 67+06=073 67+06=073 True
6 1 66 22+04=026 22+04=036 False
6 1 67 61+87=148 61+87=148 True
6 1 68 95+27=122 95+27=112 False
6 1 69 49+83=132 49+83=131 False
6 1 70 43+00=043 43+00=053 False
6 1 71 01+85=086 01+85=096 False
6 1 72 11+68=079 11+68=089 False
6 1 73 80+03=083 80+03=082 False
6 1 74 54+83=137 54+83=138 False
6 1 75 73+47=120 73+47=110 False
6 1 76 99+93=192 99+93=181 False
6 1 77 99+13=112 99+13=102 False
6 1 78 92+66=158 92+66=158 True
6 1 79 90+31=121 90+31=111 False
6 1 80 25+69=094 25+69=094 True
6 1 81 25+44=069 25+44=079 False
6 1 82 00+93=093 00+93=092 False
6 1 83 88+87=175 88+87=165 False
6 1 84 47+56=103 47+56=103 True
6 1 85 43+59=102 43+59=102 True
6 1 86 22+00=022 22+00=022 True
6 1 87 34+04=038 34+04=039 False
6 1 88 65+13=078 65+13=088 False
6 1 89 39+82=121 39+82=111 False
6 1 90 66+83=149 66+83=149 True
6 1 91 51+69=120 51+69=110 False
6 1 92 80+21=101 80+21=101 True
6 1 93 36+79=115 36+79=115 True
6 1 94 21+68=089 21+68=098 False
6 1 95 11+66=077 11+66=077 True
6 1 96 55+19=074 55+19=074 True
6 1 97 51+61=112 51+61=112 True
6 1 98 38+88=126 38+88=126 True
6 1 99 37+27=064 37+27=075 False
6 1 100 18+63=081 18+63=081 True
6 1 101 48+11=059 48+11=068 False
6 1 102 72+68=140 72+68=130 False
6 1 103 37+39=076 37+39=086 False
6 1 104 64+95=159 64+95=150 False
6 1 105 49+75=124 49+75=124 True
6 1 106 45+66=111 45+66=111 True
6 1 107 34+87=121 34+87=111 False
6 1 108 02+84=086 02+84=096 False
6 1 109 95+00=095 95+00=096 False
6 1 110 09+56=065 09+56=075 False
6 1 111 22+66=088 22+66=088 True
6 1 112 43+18=061 43+18=071 False
6 1 113 61+35=096 61+35=096 True
6 1 114 13+73=086 13+73=087 False
6 1 115 25+95=120 25+95=111 False
6 1 116 73+96=169 73+96=169 True
6 1 117 03+96=099 03+96=099 True
6 1 118 97+82=179 97+82=179 True
6 1 119 18+42=060 18+42=060 True
6 1 120 29+98=127 29+98=127 True
6 1 121 61+00=061 61+00=061 True
6 1 122 22+98=120 22+98=110 False
6 1 123 12+50=062 12+50=071 False
6 1 124 02+58=060 02+58=070 False
6 1 125 75+86=161 75+86=151 False
6 1 126 31+57=088 31+57=098 False
6 1 127 49+82=131 49+82=131 True
6 1 128 15+33=048 15+33=058 False
6 1 129 49+57=106 49+57=106 True
6 1 130 61+70=131 61+70=121 False
6 1 131 91+51=142 91+51=131 False
6 1 132 50+05=055 50+05=065 False
6 1 133 44+16=060 44+16=060 True
6 1 134 92+01=093 92+01=092 False
6 1 135 85+82=167 85+82=168 False
6 1 136 07+41=048 07+41=058 False
6 1 137 40+06=046 40+06=056 False
6 1 138 79+62=141 79+62=131 False
6 1 139 95+62=157 95+62=158 False
6 1 140 42+93=135 42+93=136 False
6 1 141 32+73=105 32+73=106 False
6 1 142 47+09=056 47+09=066 False
6 1 143 59+50=109 59+50=108 False
6 1 144 61+77=138 61+77=138 True
6 1 145 64+06=070 64+06=070 True
6 1 146 35+10=045 35+10=055 False
6 1 147 32+88=120 32+88=110 False
6 1 148 03+95=098 03+95=099 False
6 1 149 11+38=049 11+38=059 False
6 1 150 21+67=088 21+67=088 True
6 1 151 33+25=058 33+25=068 False
6 1 152 63+45=108 63+45=109 False
6 1 153 56+12=068 56+12=078 False
6 1 154 19+79=098 19+79=098 True
6 1 155 60+43=103 60+43=103 True
6 1 156 07+61=068 07+61=078 False
6 1 157 58+03=061 58+03=071 False
6 1 158 11+10=021 11+10=021 True
6 1 159 49+89=138 49+89=138 True
6 1 160 37+58=095 37+58=095 True
6 1 161 59+78=137 59+78=137 True
6 1 162 11+21=032 11+21=031 False
6 1 163 37+43=080 37+43=080 True
6 1 164 44+21=065 44+21=075 False
6 1 165 22+97=119 22+97=119 True
6 1 166 65+35=100 65+35=100 True
6 1 167 06+51=057 06+51=067 False
6 1 168 65+25=090 65+25=091 False
6 1 169 74+94=168 74+94=169 False
6 1 170 87+55=142 87+55=142 True
6 1 171 90+67=157 90+67=157 True
6 1 172 11+02=013 11+02=013 True
6 1 173 01+66=067 01+66=077 False
6 1 174 56+00=056 56+00=066 False
6 1 175 58+52=110 58+52=110 True
6 1 176 24+99=123 24+99=113 False
6 1 177 97+13=110 97+13=100 False
6 1 178 42+94=136 42+94=137 False
6 1 179 60+15=075 60+15=075 True
6 1 180 20+46=066 20+46=076 False
6 1 181 40+70=110 40+70=110 True
6 1 182 95+45=140 95+45=131 False
6 1 183 96+95=191 96+95=181 False
6 1 184 98+20=118 98+20=117 False
6 1 185 43+19=062 43+19=072 False
6 1 186 50+69=119 50+69=119 True
6 1 187 27+53=080 27+53=080 True
6 1 188 24+25=049 24+25=050 False
6 1 189 65+92=157 65+92=158 False
6 1 190 28+14=042 28+14=042 True
6 1 191 20+57=077 20+57=087 False
6 1 192 59+97=156 59+97=156 True
6 1 193 98+32=130 98+32=120 False
6 1 194 55+84=139 55+84=130 False
6 1 195 20+39=059 20+39=069 False
6 1 196 86+47=133 86+47=133 True
6 1 197 92+36=128 92+36=128 True
6 1 198 05+38=043 05+38=043 True
6 1 199 77+36=113 77+36=113 True
6 1 200 41+64=105 41+64=106 False
6 1 201 74+51=125 74+51=126 False
6 1 202 74+55=129 74+55=120 False
6 1 203 64+64=128 64+64=129 False
6 1 204 60+19=079 60+19=089 False
6 1 205 77+96=173 77+96=163 False
6 1 206 22+30=052 22+30=052 True
6 1 207 82+49=131 82+49=131 True
6 1 208 39+67=106 39+67=106 True
6 1 209 62+40=102 62+40=101 False
6 1 210 28+71=099 28+71=098 False
6 1 211 47+26=073 47+26=073 True
6 1 212 98+54=152 98+54=142 False
6 1 213 38+70=108 38+70=107 False
6 1 214 63+40=103 63+40=103 True
6 1 215 86+62=148 86+62=148 True
6 1 216 22+65=087 22+65=088 False
6 1 217 41+17=058 41+17=068 False
6 1 218 68+88=156 68+88=156 True
6 1 219 96+70=166 96+70=166 True
6 1 220 99+29=128 99+29=128 True
6 1 221 83+39=122 83+39=122 True
6 1 222 26+55=081 26+55=081 True
6 1 223 53+70=123 53+70=123 True
6 1 224 94+12=106 94+12=107 False
6 1 225 00+37=037 00+37=047 False
6 1 226 36+94=130 36+94=120 False
6 1 227 40+58=098 40+58=098 True
6 1 228 19+80=099 19+80=098 False
6 1 229 49+44=093 49+44=093 True
6 1 230 70+27=097 70+27=097 True
6 1 231 52+80=132 52+80=132 True
6 1 232 77+90=167 77+90=167 True
6 1 233 13+92=105 13+92=106 False
6 1 234 59+09=068 59+09=078 False
6 1 235 33+55=088 33+55=089 False
6 1 236 85+16=101 85+16=101 True
6 1 237 25+65=090 25+65=091 False
6 1 238 46+20=066 46+20=076 False
6 1 239 29+52=081 29+52=081 True
6 1 240 32+36=068 32+36=078 False
6 1 241 47+08=055 47+08=065 False
6 1 242 21+84=105 21+84=106 False
6 1 243 24+45=069 24+45=070 False
6 1 244 29+15=044 29+15=044 True
6 1 245 83+03=086 83+03=087 False
6 1 246 83+36=119 83+36=119 True
6 1 247 58+95=153 58+95=143 False
6 1 248 76+79=155 76+79=145 False
6 1 249 63+30=093 63+30=092 False
6 1 250 38+24=062 38+24=062 True
6 1 251 19+46=065 19+46=075 False
6 1 252 99+66=165 99+66=165 True
6 1 253 95+73=168 95+73=168 True
6 1 254 65+27=092 65+27=092 True
6 1 255 91+83=174 91+83=174 True
6 2 0 65+49=114 65+49=114 True
6 2 1 03+08=011 03+08=011 True
6 2 2 67+81=148 67+81=148 True
6 2 3 47+23=070 47+23=070 True
6 2 4 43+91=134 43+91=134 True
6 2 5 41+67=108 41+67=108 True
6 2 6 02+33=035 02+33=045 False
6 2 7 64+84=148 64+84=149 False
6 2 8 81+64=145 81+64=146 False
6 2 9 80+11=091 80+11=091 True
6 2 10 78+01=079 78+01=088 False
6 2 11 89+18=107 89+18=107 True
6 2 12 45+52=097 45+52=098 False
6 2 13 35+30=065 35+30=075 False
6 2 14 53+32=085 53+32=086 False
6 2 15 49+90=139 49+90=138 False
6 2 16 41+37=078 41+37=088 False
6 2 17 35+14=049 35+14=059 False
6 2 18 92+50=142 92+50=131 False
6 2 19 37+60=097 37+60=097 True
6 2 20 91+61=152 91+61=141 False
6 2 21 80+77=157 80+77=157 True
6 2 22 66+24=090 66+24=090 True
6 2 23 81+07=088 81+07=098 False
6 2 24 85+59=144 85+59=144 True
6 2 25 19+69=088 19+69=088 True
6 2 26 91+44=135 91+44=136 False
6 2 27 25+29=054 25+29=064 False
6 2 28 27+08=035 27+08=045 False
6 2 29 66+14=080 66+14=080 True
6 2 30 95+11=106 95+11=107 False
6 2 31 13+97=110 13+97=100 False
6 2 32 94+40=134 94+40=133 False
6 2 33 74+31=105 74+31=106 False
6 2 34 49+00=049 49+00=058 False
6 2 35 59+18=077 59+18=087 False
6 2 36 07+65=072 07+65=072 True
6 2 37 83+55=138 83+55=139 False
6 2 38 49+80=129 49+80=138 False
6 2 39 64+17=081 64+17=081 True
6 2 40 48+83=131 48+83=131 True
6 2 41 95+44=139 95+44=139 True
6 2 42 71+26=097 71+26=097 True
6 2 43 06+74=080 06+74=080 True
6 2 44 34+24=058 34+24=068 False
6 2 45 59+71=130 59+71=120 False
6 2 46 68+32=100 68+32=100 True
6 2 47 38+81=119 38+81=118 False
6 2 48 29+56=085 29+56=085 True
6 2 49 54+55=109 54+55=100 False
6 2 50 31+27=058 31+27=068 False
6 2 51 97+89=186 97+89=186 True
6 2 52 48+09=057 48+09=067 False
6 2 53 86+76=162 86+76=153 False
6 2 54 82+59=141 82+59=131 False
6 2 55 01+67=068 01+67=078 False
6 2 56 26+06=032 26+06=033 False
6 2 57 22+46=068 22+46=078 False
6 2 58 85+16=101 85+16=101 True
6 2 59 29+08=037 29+08=047 False
6 2 60 73+94=167 73+94=168 False
6 2 61 19+62=081 19+62=081 True
6 2 62 86+62=148 86+62=148 True
6 2 63 38+99=137 38+99=137 True
6 2 64 64+25=089 64+25=090 False
6 2 65 61+72=133 61+72=122 False
6 2 66 78+88=166 78+88=166 True
6 2 67 43+66=109 43+66=109 True
6 2 68 69+35=104 69+35=103 False
6 2 69 33+77=110 33+77=100 False
6 2 70 37+37=074 37+37=075 False
6 2 71 87+54=141 87+54=141 True
6 2 72 68+90=158 68+90=157 False
6 2 73 83+44=127 83+44=128 False
6 2 74 41+09=050 41+09=050 True
6 2 75 13+48=061 13+48=071 False
6 2 76 01+41=042 01+41=051 False
6 2 77 19+74=093 19+74=093 True
6 2 78 15+05=020 15+05=020 True
6 2 79 55+46=101 55+46=101 True
6 2 80 68+33=101 68+33=101 True
6 2 81 44+40=084 44+40=084 True
6 2 82 88+03=091 88+03=091 True
6 2 83 81+79=160 81+79=150 False
6 2 84 18+98=116 18+98=106 False
6 2 85 70+64=134 70+64=134 True
6 2 86 26+44=070 26+44=070 True
6 2 87 98+87=185 98+87=186 False
6 2 88 18+74=092 18+74=092 True
6 2 89 50+68=118 50+68=118 True
6 2 90 13+51=064 13+51=073 False
6 2 91 90+89=179 90+89=179 True
6 2 92 47+78=125 47+78=125 True
6 2 93 81+57=138 81+57=138 True
6 2 94 34+47=081 34+47=081 True
6 2 95 94+23=117 94+23=118 False
6 2 96 07+70=077 07+70=087 False
6 2 97 56+33=089 56+33=099 False
6 2 98 33+04=037 33+04=038 False
6 2 99 26+09=035 26+09=035 True
6 2 100 14+92=106 14+92=107 False
6 2 101 78+54=132 78+54=132 True
6 2 102 36+76=112 36+76=113 False
6 2 103 17+47=064 17+47=075 False
6 2 104 28+18=046 28+18=056 False
6 2 105 78+54=132 78+54=132 True
6 2 106 84+72=156 84+72=157 False
6 2 107 00+44=044 00+44=053 False
6 2 108 50+41=091 50+41=091 True
6 2 109 87+88=175 87+88=165 False
6 2 110 11+66=077 11+66=077 True
6 2 111 80+60=140 80+60=140 True
6 2 112 78+76=154 78+76=145 False
6 2 113 24+74=098 24+74=099 False
6 2 114 88+48=136 88+48=136 True
6 2 115 38+31=069 38+31=078 False
6 2 116 29+27=056 29+27=066 False
6 2 117 08+45=053 08+45=063 False
6 2 118 28+13=041 28+13=041 True
6 2 119 53+99=152 53+99=142 False
6 2 120 47+92=139 47+92=139 True
6 2 121 76+21=097 76+21=097 True
6 2 122 53+96=149 53+96=149 True
6 2 123 93+91=184 93+91=174 False
6 2 124 97+33=130 97+33=120 False
6 2 125 67+78=145 67+78=145 True
6 2 126 58+05=063 58+05=073 False
6 2 127 00+16=016 00+16=026 False
6 2 128 80+19=099 80+19=099 True
6 2 129 98+22=120 98+22=110 False
6 2 130 09+62=071 09+62=071 True
6 2 131 06+23=029 06+23=039 False
6 2 132 32+99=131 32+99=121 False
6 2 133 17+02=019 17+02=029 False
6 2 134 64+35=099 64+35=100 False
6 2 135 35+83=118 35+83=118 True
6 2 136 71+36=107 71+36=107 True
6 2 137 75+06=081 75+06=081 True
6 2 138 88+95=183 88+95=173 False
6 2 139 19+98=117 19+98=107 False
6 2 140 28+89=117 28+89=116 False
6 2 141 33+11=044 33+11=053 False
6 2 142 34+49=083 34+49=083 True
6 2 143 90+35=125 90+35=125 True
6 2 144 22+90=112 22+90=111 False
6 2 145 98+89=187 98+89=187 True
6 2 146 88+47=135 88+47=135 True
6 2 147 30+86=116 30+86=116 True
6 2 148 31+48=079 31+48=089 False
6 2 149 39+21=060 39+21=060 True
6 2 150 19+17=036 19+17=046 False
6 2 151 27+60=087 27+60=087 True
6 2 152 12+16=028 12+16=038 False
6 2 153 51+75=126 51+75=127 False
6 2 154 10+74=084 10+74=083 False
6 2 155 42+63=105 42+63=106 False
6 2 156 40+14=054 40+14=064 False
6 2 157 23+93=116 23+93=117 False
6 2 158 85+26=111 85+26=111 True
6 2 159 28+46=074 28+46=075 False
6 2 160 28+33=061 28+33=061 True
6 2 161 43+30=073 43+30=073 True
6 2 162 89+72=161 89+72=161 True
6 2 163 52+21=073 52+21=072 False
6 2 164 21+54=075 21+54=075 True
6 2 165 69+13=082 69+13=081 False
6 2 166 07+60=067 07+60=077 False
6 2 167 63+83=146 63+83=147 False
6 2 168 80+69=149 80+69=149 True
6 2 169 27+28=055 27+28=065 False
6 2 170 42+31=073 42+31=072 False
6 2 171 51+99=150 51+99=140 False
6 2 172 28+75=103 28+75=103 True
6 2 173 38+57=095 38+57=095 True
6 2 174 83+16=099 83+16=099 True
6 2 175 92+94=186 92+94=187 False
6 2 176 55+75=130 55+75=121 False
6 2 177 59+51=110 59+51=100 False
6 2 178 33+09=042 33+09=042 True
6 2 179 53+13=066 53+13=077 False
6 2 180 05+70=075 05+70=085 False
6 2 181 12+20=032 12+20=032 True
6 2 182 11+49=060 11+49=060 True
6 2 183 63+45=108 63+45=109 False
6 2 184 92+23=115 92+23=115 True
6 2 185 82+45=127 82+45=128 False
6 2 186 23+41=064 23+41=073 False
6 2 187 64+26=090 64+26=090 True
6 2 188 91+24=115 91+24=116 False
6 2 189 20+32=052 20+32=052 True
6 2 190 83+21=104 83+21=104 True
6 2 191 07+20=027 07+20=037 False
6 2 192 94+14=108 94+14=109 False
6 2 193 96+89=185 96+89=185 True
6 2 194 13+08=021 13+08=021 True
6 2 195 32+05=037 32+05=038 False
6 2 196 09+51=060 09+51=060 True
6 2 197 26+29=055 26+29=065 False
6 2 198 49+65=114 49+65=114 True
6 2 199 32+66=098 32+66=098 True
6 2 200 41+08=049 41+08=059 False
6 2 201 26+79=105 26+79=105 True
6 2 202 29+91=120 29+91=110 False
6 2 203 51+00=051 51+00=061 False
6 2 204 61+60=121 61+60=111 False
6 2 205 45+78=123 45+78=113 False
6 2 206 56+16=072 56+16=073 False
6 2 207 66+68=134 66+68=134 True
6 2 208 32+16=048 32+16=058 False
6 2 209 84+49=133 84+49=133 True
6 2 210 45+09=054 45+09=064 False
6 2 211 96+78=174 96+78=175 False
6 2 212 10+02=012 10+02=012 True
6 2 213 36+60=096 36+60=096 True
6 2 214 44+36=080 44+36=080 True
6 2 215 12+86=098 12+86=098 True
6 2 216 94+54=148 94+54=149 False
6 2 217 64+73=137 64+73=138 False
6 2 218 73+10=083 73+10=092 False
6 2 219 14+62=076 14+62=077 False
6 2 220 25+22=047 25+22=058 False
6 2 221 94+22=116 94+22=117 False
6 2 222 41+76=117 41+76=117 True
6 2 223 38+46=084 38+46=085 False
6 2 224 71+72=143 71+72=132 False
6 2 225 74+79=153 74+79=143 False
6 2 226 99+67=166 99+67=166 True
6 2 227 78+71=149 78+71=149 True
6 2 228 23+19=042 23+19=042 True
6 2 229 51+65=116 51+65=117 False
6 2 230 94+86=180 94+86=170 False
6 2 231 09+79=088 09+79=088 True
6 2 232 69+39=108 69+39=108 True
6 2 233 84+13=097 84+13=098 False
6 2 234 36+59=095 36+59=095 True
6 2 235 87+47=134 87+47=135 False
6 2 236 50+00=050 50+00=060 False
6 2 237 76+96=172 76+96=163 False
6 2 238 12+18=030 12+18=030 True
6 2 239 99+95=194 99+95=184 False
6 2 240 22+00=022 22+00=022 True
6 2 241 96+18=114 96+18=105 False
6 2 242 51+20=071 51+20=071 True
6 2 243 66+81=147 66+81=147 True
6 2 244 78+18=096 78+18=096 True
6 2 245 09+78=087 09+78=087 True
6 2 246 24+20=044 24+20=044 True
6 2 247 76+13=089 76+13=099 False
6 2 248 05+10=015 05+10=026 False
6 2 249 97+14=111 97+14=101 False
6 2 250 92+38=130 92+38=120 False
6 2 251 77+13=090 77+13=090 True
6 2 252 70+19=089 70+19=099 False
6 2 253 90+45=135 90+45=135 True
6 2 254 50+09=059 50+09=069 False
6 2 255 78+06=084 78+06=085 False
6 3 0 03+25=028 03+25=038 False
6 3 1 48+43=091 48+43=091 True
6 3 2 39+47=086 39+47=086 True
6 3 3 48+19=067 48+19=077 False
6 3 4 07+22=029 07+22=039 False
6 3 5 73+68=141 73+68=131 False
6 3 6 14+56=070 14+56=070 True
6 3 7 96+95=191 96+95=181 False
6 3 8 96+28=124 96+28=114 False
6 3 9 82+05=087 82+05=088 False
6 3 10 27+94=121 27+94=111 False
6 3 11 87+86=173 87+86=163 False
6 3 12 00+68=068 00+68=078 False
6 3 13 11+37=048 11+37=058 False
6 3 14 95+93=188 95+93=188 True
6 3 15 75+82=157 75+82=158 False
6 3 16 41+71=112 41+71=112 True
6 3 17 60+14=074 60+14=073 False
6 3 18 77+77=154 77+77=145 False
6 3 19 31+84=115 31+84=116 False
6 3 20 31+57=088 31+57=098 False
6 3 21 27+87=114 27+87=115 False
6 3 22 31+89=120 31+89=110 False
6 3 23 22+18=040 22+18=040 True
6 3 24 38+25=063 38+25=063 True
6 3 25 64+54=118 64+54=119 False
6 3 26 85+60=145 85+60=145 True
6 3 27 14+71=085 14+71=085 True
6 3 28 06+16=022 06+16=023 False
6 3 29 78+61=139 78+61=138 False
6 3 30 65+75=140 65+75=131 False
6 3 31 13+83=096 13+83=097 False
6 3 32 75+49=124 75+49=124 True
6 3 33 05+78=083 05+78=083 True
6 3 34 66+55=121 66+55=111 False
6 3 35 03+05=008 03+05=018 False
6 3 36 69+99=168 69+99=168 True
6 3 37 52+82=134 52+82=134 True
6 3 38 45+97=142 45+97=132 False
6 3 39 66+17=083 66+17=083 True
6 3 40 36+17=053 36+17=063 False
6 3 41 92+74=166 92+74=166 True
6 3 42 48+44=092 48+44=092 True
6 3 43 34+17=051 34+17=051 True
6 3 44 56+11=067 56+11=077 False
6 3 45 77+23=100 77+23=090 False
6 3 46 10+11=021 10+11=021 True
6 3 47 32+65=097 32+65=098 False
6 3 48 53+49=102 53+49=102 True
6 3 49 68+86=154 68+86=155 False
6 3 50 52+94=146 52+94=147 False
6 3 51 97+71=168 97+71=168 True
6 3 52 05+37=042 05+37=042 True
6 3 53 58+75=133 58+75=133 True
6 3 54 06+24=030 06+24=030 True
6 3 55 15+44=059 15+44=069 False
6 3 56 90+49=139 90+49=139 True
6 3 57 50+37=087 50+37=097 False
6 3 58 88+61=149 88+61=149 True
6 3 59 21+57=078 21+57=088 False
6 3 60 24+85=109 24+85=100 False
6 3 61 01+66=067 01+66=077 False
6 3 62 50+46=096 50+46=096 True
6 3 63 76+65=141 76+65=131 False
6 3 64 23+74=097 23+74=098 False
6 3 65 76+16=092 76+16=093 False
6 3 66 06+08=014 06+08=024 False
6 3 67 69+25=094 69+25=094 True
6 3 68 15+23=038 15+23=048 False
6 3 69 41+02=043 41+02=052 False
6 3 70 16+66=082 16+66=083 False
6 3 71 59+94=153 59+94=143 False
6 3 72 32+88=120 32+88=110 False
6 3 73 46+21=067 46+21=077 False
6 3 74 57+28=085 57+28=085 True
6 3 75 00+31=031 00+31=031 True
6 3 76 77+07=084 77+07=085 False
6 3 77 28+70=098 28+70=097 False
6 3 78 05+61=066 05+61=076 False
6 3 79 22+09=031 22+09=031 True
6 3 80 08+94=102 08+94=102 True
6 3 81 40+11=051 40+11=061 False
6 3 82 10+48=058 10+48=068 False
6 3 83 27+56=083 27+56=083 True
6 3 84 42+16=058 42+16=068 False
6 3 85 69+43=112 69+43=112 True
6 3 86 57+69=126 57+69=126 True
6 3 87 18+86=104 18+86=105 False
6 3 88 86+80=166 86+80=166 True
6 3 89 30+85=115 30+85=116 False
6 3 90 77+66=143 77+66=133 False
6 3 91 39+64=103 39+64=103 True
6 3 92 76+61=137 76+61=137 True
6 3 93 42+61=103 42+61=103 True
6 3 94 07+30=037 07+30=047 False
6 3 95 35+93=128 35+93=128 True
6 3 96 40+90=130 40+90=130 True
6 3 97 08+91=099 08+91=098 False
6 3 98 62+34=096 62+34=096 True
6 3 99 86+49=135 86+49=135 True
6 3 100 73+23=096 73+23=097 False
6 3 101 87+35=122 87+35=122 True
6 3 102 35+31=066 35+31=077 False
6 3 103 07+13=020 07+13=020 True
6 3 104 39+41=080 39+41=080 True
6 3 105 44+63=107 44+63=108 False
6 3 106 94+66=160 94+66=150 False
6 3 107 49+54=103 49+54=103 True
6 3 108 79+46=125 79+46=125 True
6 3 109 53+12=065 53+12=075 False
6 3 110 60+92=152 60+92=152 True
6 3 111 25+60=085 25+60=084 False
6 3 112 64+53=117 64+53=118 False
6 3 113 41+02=043 41+02=052 False
6 3 114 00+97=097 00+97=097 True
6 3 115 12+52=064 12+52=073 False
6 3 116 39+50=089 39+50=088 False
6 3 117 87+21=108 87+21=108 True
6 3 118 04+99=103 04+99=103 True
6 3 119 19+75=094 19+75=094 True
6 3 120 90+05=095 90+05=094 False
6 3 121 54+39=093 54+39=093 True
6 3 122 29+26=055 29+26=065 False
6 3 123 82+95=177 82+95=178 False
6 3 124 55+09=064 55+09=074 False
6 3 125 02+62=064 02+62=073 False
6 3 126 68+30=098 68+30=097 False
6 3 127 99+16=115 99+16=105 False
6 3 128 63+11=074 63+11=073 False
6 3 129 42+92=134 42+92=134 True
6 3 130 99+16=115 99+16=105 False
6 3 131 50+31=081 50+31=081 True
6 3 132 23+46=069 23+46=079 False
6 3 133 45+73=118 45+73=118 True
6 3 134 89+77=166 89+77=166 True
6 3 135 45+78=123 45+78=113 False
6 3 136 96+60=156 96+60=156 True
6 3 137 74+61=135 74+61=135 True
6 3 138 87+01=088 87+01=097 False
6 3 139 63+88=151 63+88=151 True
6 3 140 59+72=131 59+72=131 True
6 3 141 17+96=113 17+96=103 False
6 3 142 89+77=166 89+77=166 True
6 3 143 24+69=093 24+69=093 True
6 3 144 75+83=158 75+83=158 True
6 3 145 50+54=104 50+54=104 True
6 3 146 93+47=140 93+47=130 False
6 3 147 20+55=075 20+55=075 True
6 3 148 91+79=170 91+79=170 True
6 3 149 15+13=028 15+13=038 False
6 3 150 86+09=095 86+09=095 True
6 3 151 29+58=087 29+58=087 True
6 3 152 01+29=030 01+29=030 True
6 3 153 65+48=113 65+48=113 True
6 3 154 96+45=141 96+45=131 False
6 3 155 58+69=127 58+69=127 True
6 3 156 84+43=127 84+43=128 False
6 3 157 90+38=128 90+38=128 True
6 3 158 39+97=136 39+97=136 True
6 3 159 74+84=158 74+84=159 False
6 3 160 86+22=108 86+22=108 True
6 3 161 01+86=087 01+86=097 False
6 3 162 81+63=144 81+63=144 True
6 3 163 80+94=174 80+94=174 True
6 3 164 44+42=086 44+42=087 False
6 3 165 72+60=132 72+60=132 True
6 3 166 28+07=035 28+07=045 False
6 3 167 69+54=123 69+54=123 True
6 3 168 68+77=145 68+77=145 True
6 3 169 90+16=106 90+16=106 True
6 3 170 64+50=114 64+50=114 True
6 3 171 46+88=134 46+88=134 True
6 3 172 55+99=154 55+99=144 False
6 3 173 31+97=128 31+97=128 True
6 3 174 79+28=107 79+28=107 True
6 3 175 81+43=124 81+43=124 True
6 3 176 41+15=056 41+15=066 False
6 3 177 38+77=115 38+77=115 True
6 3 178 25+06=031 25+06=031 True
6 3 179 01+93=094 01+93=094 True
6 3 180 97+22=119 97+22=119 True
6 3 181 71+84=155 71+84=155 True
6 3 182 26+36=062 26+36=062 True
6 3 183 60+92=152 60+92=152 True
6 3 184 02+94=096 02+94=096 True
6 3 185 31+58=089 31+58=099 False
6 3 186 70+52=122 70+52=112 False
6 3 187 19+42=061 19+42=061 True
6 3 188 95+73=168 95+73=168 True
6 3 189 21+25=046 21+25=057 False
6 3 190 13+58=071 13+58=071 True
6 3 191 62+28=090 62+28=090 True
6 3 192 38+14=052 38+14=052 True
6 3 193 66+75=141 66+75=131 False
6 3 194 24+59=083 24+59=083 True
6 3 195 97+66=163 97+66=153 False
6 3 196 76+70=146 76+70=146 True
6 3 197 08+40=048 08+40=057 False
6 3 198 84+00=084 84+00=094 False
6 3 199 54+73=127 54+73=128 False
6 3 200 16+88=104 16+88=105 False
6 3 201 99+47=146 99+47=146 True
6 3 202 31+95=126 31+95=127 False
6 3 203 01+79=080 01+79=080 True
6 3 204 03+68=071 03+68=071 True
6 3 205 10+05=015 10+05=015 True
6 3 206 98+90=188 98+90=187 False
6 3 207 58+53=111 58+53=111 True
6 3 208 34+87=121 34+87=111 False
6 3 209 07+31=038 07+31=047 False
6 3 210 59+08=067 59+08=077 False
6 3 211 51+38=089 51+38=099 False
6 3 212 62+62=124 62+62=114 False
6 3 213 80+32=112 80+32=112 True
6 3 214 69+16=085 69+16=085 True
6 3 215 01+17=018 01+17=028 False
6 3 216 74+41=115 74+41=116 False
6 3 217 20+89=109 20+89=109 True
6 3 218 53+50=103 53+50=103 True
6 3 219 82+85=167 82+85=168 False
6 3 220 34+47=081 34+47=081 True
6 3 221 34+45=079 34+45=080 False
6 3 222 77+34=111 77+34=111 True
6 3 223 56+33=089 56+33=099 False
6 3 224 97+56=153 97+56=143 False
6 3 225 29+06=035 29+06=035 True
6 3 226 78+96=174 78+96=165 False
6 3 227 28+65=093 28+65=093 True
6 3 228 61+64=125 61+64=116 False
6 3 229 32+64=096 32+64=097 False
6 3 230 98+32=130 98+32=120 False
6 3 231 25+35=060 25+35=060 True
6 3 232 05+08=013 05+08=013 True
6 3 233 05+26=031 05+26=031 True
6 3 234 84+71=155 84+71=155 True
6 3 235 33+10=043 33+10=053 False
6 3 236 98+35=133 98+35=133 True
6 3 237 68+98=166 68+98=166 True
6 3 238 03+63=066 03+63=077 False
6 3 239 12+96=108 12+96=108 True
6 3 240 02+81=083 02+81=082 False
6 3 241 83+13=096 83+13=097 False
6 3 242 55+92=147 55+92=148 False
6 3 243 96+09=105 96+09=105 True
6 3 244 61+08=069 61+08=079 False
6 3 245 39+75=114 39+75=114 True
6 3 246 40+74=114 40+74=114 True
6 3 247 39+80=119 39+80=118 False
6 3 248 57+95=152 57+95=142 False
6 3 249 92+97=189 92+97=189 True
6 3 250 33+03=036 33+03=037 False
6 3 251 74+92=166 74+92=167 False
6 3 252 99+09=108 99+09=108 True
6 3 253 98+10=108 98+10=107 False
6 3 254 46+77=123 46+77=113 False
6 3 255 85+78=163 85+78=153 False
6 4 0 41+21=062 41+21=062 True
6 4 1 49+13=062 49+13=071 False
6 4 2 59+07=066 59+07=076 False
6 4 3 31+11=042 31+11=051 False
6 4 4 74+16=090 74+16=090 True
6 4 5 43+38=081 43+38=081 True
6 4 6 08+67=075 08+67=075 True
6 4 7 31+66=097 31+66=097 True
6 4 8 10+31=041 10+31=051 False
6 4 9 34+59=093 34+59=093 True
6 4 10 78+42=120 78+42=110 False
6 4 11 13+41=054 13+41=063 False
6 4 12 97+89=186 97+89=186 True
6 4 13 15+62=077 15+62=088 False
6 4 14 39+36=075 39+36=075 True
6 4 15 21+25=046 21+25=057 False
6 4 16 74+56=130 74+56=120 False
6 4 17 85+47=132 85+47=132 True
6 4 18 47+32=079 47+32=089 False
6 4 19 37+66=103 37+66=103 True
6 4 20 16+29=045 16+29=055 False
6 4 21 86+77=163 86+77=153 False
6 4 22 80+07=087 80+07=097 False
6 4 23 87+05=092 87+05=092 True
6 4 24 58+16=074 58+16=075 False
6 4 25 52+79=131 52+79=121 False
6 4 26 91+08=099 91+08=099 True
6 4 27 47+78=125 47+78=125 True
6 4 28 86+96=182 86+96=173 False
6 4 29 90+22=112 90+22=111 False
6 4 30 31+18=049 31+18=059 False
6 4 31 86+15=101 86+15=101 True
6 4 32 15+95=110 15+95=100 False
6 4 33 42+11=053 42+11=062 False
6 4 34 65+99=164 65+99=154 False
6 4 35 89+29=118 89+29=117 False
6 4 36 35+11=046 35+11=057 False
6 4 37 71+41=112 71+41=111 False
6 4 38 16+24=040 16+24=040 True
6 4 39 77+82=159 77+82=159 True
6 4 40 55+89=144 55+89=144 True
6 4 41 17+88=105 17+88=106 False
6 4 42 54+72=126 54+72=127 False
6 4 43 34+98=132 34+98=132 True
6 4 44 09+97=106 09+97=106 True
6 4 45 91+07=098 91+07=098 True
6 4 46 55+94=149 55+94=140 False
6 4 47 22+58=080 22+58=080 True
6 4 48 91+37=128 91+37=128 True
6 4 49 16+10=026 16+10=036 False
6 4 50 96+32=128 96+32=128 True
6 4 51 35+75=110 35+75=111 False
6 4 52 88+73=161 88+73=151 False
6 4 53 35+18=053 35+18=063 False
6 4 54 33+10=043 33+10=053 False
6 4 55 08+50=058 08+50=067 False
6 4 56 22+62=084 22+62=083 False
6 4 57 26+37=063 26+37=063 True
6 4 58 80+27=107 80+27=107 True
6 4 59 68+28=096 68+28=096 True
6 4 60 48+03=051 48+03=061 False
6 4 61 40+18=058 40+18=068 False
6 4 62 16+59=075 16+59=075 True
6 4 63 02+19=021 02+19=021 True
6 4 64 01+09=010 01+09=010 True
6 4 65 62+68=130 62+68=120 False
6 4 66 09+71=080 09+71=080 True
6 4 67 00+58=058 00+58=068 False
6 4 68 16+45=061 16+45=061 True
6 4 69 24+98=122 24+98=112 False
6 4 70 47+92=139 47+92=139 True
6 4 71 94+84=178 94+84=178 True
6 4 72 21+32=053 21+32=062 False
6 4 73 29+82=111 29+82=100 False
6 4 74 32+79=111 32+79=101 False
6 4 75 13+98=111 13+98=101 False
6 4 76 41+94=135 41+94=136 False
6 4 77 51+84=135 51+84=136 False
6 4 78 42+05=047 42+05=058 False
6 4 79 39+03=042 39+03=041 False
6 4 80 02+92=094 02+92=093 False
6 4 81 99+81=180 99+81=170 False
6 4 82 32+68=100 32+68=100 True
6 4 83 52+17=069 52+17=079 False
6 4 84 56+58=114 56+58=114 True
6 4 85 21+48=069 21+48=079 False
6 4 86 61+71=132 61+71=121 False
6 4 87 17+01=018 17+01=027 False
6 4 88 68+23=091 68+23=091 True
6 4 89 00+37=037 00+37=047 False
6 4 90 94+88=182 94+88=172 False
6 4 91 06+31=037 06+31=047 False
6 4 92 27+18=045 27+18=055 False
6 4 93 41+81=122 41+81=121 False
6 4 94 15+86=101 15+86=101 True
6 4 95 36+87=123 36+87=113 False
6 4 96 17+37=054 17+37=065 False
6 4 97 13+86=099 13+86=099 True
6 4 98 29+69=098 29+69=098 True
6 4 99 31+99=130 31+99=120 False
6 4 100 47+29=076 47+29=086 False
6 4 101 08+81=089 08+81=098 False
6 4 102 72+82=154 72+82=154 True
6 4 103 46+91=137 46+91=137 True
6 4 104 70+35=105 70+35=106 False
6 4 105 90+55=145 90+55=134 False
6 4 106 99+99=198 99+99=188 False
6 4 107 60+97=157 60+97=157 True
6 4 108 03+40=043 03+40=053 False
6 4 109 35+49=084 35+49=084 True
6 4 110 32+02=034 32+02=033 False
6 4 111 70+18=088 70+18=098 False
6 4 112 99+05=104 99+05=104 True
6 4 113 78+73=151 78+73=141 False
6 4 114 03+02=005 03+02=015 False
6 4 115 50+14=064 50+14=074 False
6 4 116 62+02=064 62+02=073 False
6 4 117 16+74=090 16+74=090 True
6 4 118 68+65=133 68+65=133 True
6 4 119 74+81=155 74+81=155 True
6 4 120 37+48=085 37+48=085 True
6 4 121 63+04=067 63+04=078 False
6 4 122 06+62=068 06+62=078 False
6 4 123 95+75=170 95+75=161 False
6 4 124 92+37=129 92+37=129 True
6 4 125 81+32=113 81+32=113 True
6 4 126 53+28=081 53+28=081 True
6 4 127 52+42=094 52+42=094 True
6 4 128 66+97=163 66+97=153 False
6 4 129 00+48=048 00+48=058 False
6 4 130 65+32=097 65+32=098 False
6 4 131 60+89=149 60+89=149 True
6 4 132 71+61=132 71+61=121 False
6 4 133 98+50=148 98+50=147 False
6 4 134 90+96=186 90+96=176 False
6 4 135 02+96=098 02+96=098 True
6 4 136 62+75=137 62+75=138 False
6 4 137 41+28=069 41+28=079 False
6 4 138 95+79=174 95+79=174 True
6 4 139 48+41=089 48+41=098 False
6 4 140 87+95=182 87+95=172 False
6 4 141 75+38=113 75+38=113 True
6 4 142 31+55=086 31+55=086 True
6 4 143 54+63=117 54+63=118 False
6 4 144 75+82=157 75+82=158 False
6 4 145 46+45=091 46+45=091 True
6 4 146 13+08=021 13+08=021 True
6 4 147 77+97=174 77+97=165 False
6 4 148 37+35=072 37+35=072 True
6 4 149 21+89=110 21+89=100 False
6 4 150 58+51=109 58+51=108 False
6 4 151 91+48=139 91+48=139 True
6 4 152 33+23=056 33+23=067 False
6 4 153 80+96=176 80+96=176 True
6 4 154 78+02=080 78+02=080 True
6 4 155 38+95=133 38+95=133 True
6 4 156 99+25=124 99+25=114 False
6 4 157 30+76=106 30+76=106 True
6 4 158 42+40=082 42+40=081 False
6 4 159 85+58=143 85+58=143 True
6 4 160 44+46=090 44+46=090 True
6 4 161 06+41=047 06+41=057 False
6 4 162 65+90=155 65+90=155 True
6 4 163 43+83=126 43+83=128 False
6 4 164 36+61=097 36+61=097 True
6 4 165 61+51=112 61+51=112 True
6 4 166 38+09=047 38+09=057 False
6 4 167 21+97=118 21+97=118 True
6 4 168 83+30=113 83+30=111 False
6 4 169 11+79=090 11+79=090 True
6 4 170 14+29=043 14+29=043 True
6 4 171 21+11=032 21+11=031 False
6 4 172 43+53=096 43+53=107 False
6 4 173 02+58=060 02+58=070 False
6 4 174 78+82=160 78+82=150 False
6 4 175 91+11=102 91+11=101 False
6 4 176 58+54=112 58+54=112 True
6 4 177 00+15=015 00+15=015 True
6 4 178 83+51=134 83+51=134 True
6 4 179 44+72=116 44+72=117 False
6 4 180 71+20=091 71+20=091 True
6 4 181 24+99=123 24+99=113 False
6 4 182 46+30=076 46+30=086 False
6 4 183 08+67=075 08+67=075 True
6 4 184 47+42=089 47+42=099 False
6 4 185 95+67=162 95+67=152 False
6 4 186 40+56=096 40+56=096 True
6 4 187 17+95=112 17+95=112 True
6 4 188 94+66=160 94+66=150 False
6 4 189 14+58=072 14+58=072 True
6 4 190 56+05=061 56+05=061 True
6 4 191 70+01=071 70+01=071 True
6 4 192 97+59=156 97+59=146 False
6 4 193 94+67=161 94+67=151 False
6 4 194 13+41=054 13+41=063 False
6 4 195 85+15=100 85+15=100 True
6 4 196 48+53=101 48+53=101 True
6 4 197 62+75=137 62+75=138 False
6 4 198 87+47=134 87+47=135 False
6 4 199 31+88=119 31+88=118 False
6 4 200 97+16=113 97+16=103 False
6 4 201 48+45=093 48+45=093 True
6 4 202 99+00=099 99+00=108 False
6 4 203 15+01=016 15+01=026 False
6 4 204 28+96=124 28+96=125 False
6 4 205 20+11=031 20+11=031 True
6 4 206 07+56=063 07+56=073 False
6 4 207 06+08=014 06+08=024 False
6 4 208 45+46=091 45+46=091 True
6 4 209 48+85=133 48+85=133 True
6 4 210 62+14=076 62+14=076 True
6 4 211 82+31=113 82+31=113 True
6 4 212 85+88=173 85+88=163 False
6 4 213 77+08=085 77+08=085 True
6 4 214 16+64=080 16+64=080 True
6 4 215 00+27=027 00+27=037 False
6 4 216 36+75=111 36+75=111 True
6 4 217 38+38=076 38+38=086 False
6 4 218 88+32=120 88+32=110 False
6 4 219 09+88=097 09+88=097 True
6 4 220 96+87=183 96+87=173 False
6 4 221 71+29=100 71+29=090 False
6 4 222 99+13=112 99+13=102 False
6 4 223 03+13=016 03+13=027 False
6 4 224 67+23=090 67+23=090 True
6 4 225 15+98=113 15+98=103 False
6 4 226 10+08=018 10+08=028 False
6 4 227 46+24=070 46+24=070 True
6 4 228 55+63=118 55+63=118 True
6 4 229 28+06=034 28+06=035 False
6 4 230 43+87=130 43+87=120 False
6 4 231 34+05=039 34+05=040 False
2024-12-17 09:44:15,244 - root - WARNING - The method `test_one_epoch` is not implemented. Please override this method in a subclass or use a custom callback.
2024-12-17 09:44:15,245 - root - INFO - ====================================================== Starting Train Epoch: 7/9 ======================================================
2024-12-17 09:44:15,245 - root - INFO - Learning rates for each parameter group: 0.00135982073305105332, 0.00135982073305105332
0%| | 0/28 [00:00<?, ?it/s]
Epoch: 7, Step: 1: 0%| | 0/28 [00:00<?, ?it/s]
Epoch: 7, Step: 1: 0%| | 0/28 [00:00<?, ?it/s, total_batch_loss=106.61858, average_batch_loss=0.41648, average_batch_perplexity=1.51661, lr=0.001359821, 0.001359821]
Epoch: 7, Step: 2: 0%| | 0/28 [00:00<?, ?it/s, total_batch_loss=106.61858, average_batch_loss=0.41648, average_batch_perplexity=1.51661, lr=0.001359821, 0.001359821]
Epoch: 7, Step: 2: 0%| | 0/28 [00:00<?, ?it/s, total_batch_loss=105.15369, average_batch_loss=0.41076, average_batch_perplexity=1.50796, lr=0.001355815, 0.001355815]
Epoch: 7, Step: 2: 7%|▋ | 2/28 [00:00<00:02, 11.55it/s, total_batch_loss=105.15369, average_batch_loss=0.41076, average_batch_perplexity=1.50796, lr=0.001355815, 0.001355815]
Epoch: 7, Step: 3: 7%|▋ | 2/28 [00:00<00:02, 11.55it/s, total_batch_loss=105.15369, average_batch_loss=0.41076, average_batch_perplexity=1.50796, lr=0.001355815, 0.001355815]
Epoch: 7, Step: 3: 7%|▋ | 2/28 [00:00<00:02, 11.55it/s, total_batch_loss=98.28551, average_batch_loss=0.38393, average_batch_perplexity=1.46804, lr=0.001351845, 0.001351845]
Epoch: 7, Step: 4: 7%|▋ | 2/28 [00:00<00:02, 11.55it/s, total_batch_loss=98.28551, average_batch_loss=0.38393, average_batch_perplexity=1.46804, lr=0.001351845, 0.001351845]
Epoch: 7, Step: 4: 7%|▋ | 2/28 [00:00<00:02, 11.55it/s, total_batch_loss=97.85777, average_batch_loss=0.38226, average_batch_perplexity=1.46559, lr=0.001347910, 0.001347910]
Epoch: 7, Step: 4: 14%|█▍ | 4/28 [00:00<00:02, 11.98it/s, total_batch_loss=97.85777, average_batch_loss=0.38226, average_batch_perplexity=1.46559, lr=0.001347910, 0.001347910]
Epoch: 7, Step: 5: 14%|█▍ | 4/28 [00:00<00:02, 11.98it/s, total_batch_loss=97.85777, average_batch_loss=0.38226, average_batch_perplexity=1.46559, lr=0.001347910, 0.001347910]
Epoch: 7, Step: 5: 14%|█▍ | 4/28 [00:00<00:02, 11.98it/s, total_batch_loss=91.14902, average_batch_loss=0.35605, average_batch_perplexity=1.42768, lr=0.001344008, 0.001344008]
Epoch: 7, Step: 6: 14%|█▍ | 4/28 [00:00<00:02, 11.98it/s, total_batch_loss=91.14902, average_batch_loss=0.35605, average_batch_perplexity=1.42768, lr=0.001344008, 0.001344008]
Epoch: 7, Step: 6: 14%|█▍ | 4/28 [00:00<00:02, 11.98it/s, total_batch_loss=95.17233, average_batch_loss=0.37177, average_batch_perplexity=1.45029, lr=0.001340141, 0.001340141]
Epoch: 7, Step: 6: 21%|██▏ | 6/28 [00:00<00:01, 11.84it/s, total_batch_loss=95.17233, average_batch_loss=0.37177, average_batch_perplexity=1.45029, lr=0.001340141, 0.001340141]
Epoch: 7, Step: 7: 21%|██▏ | 6/28 [00:00<00:01, 11.84it/s, total_batch_loss=95.17233, average_batch_loss=0.37177, average_batch_perplexity=1.45029, lr=0.001340141, 0.001340141]
Epoch: 7, Step: 7: 21%|██▏ | 6/28 [00:00<00:01, 11.84it/s, total_batch_loss=84.90469, average_batch_loss=0.33166, average_batch_perplexity=1.39328, lr=0.001336306, 0.001336306]
Epoch: 7, Step: 8: 21%|██▏ | 6/28 [00:00<00:01, 11.84it/s, total_batch_loss=84.90469, average_batch_loss=0.33166, average_batch_perplexity=1.39328, lr=0.001336306, 0.001336306]
Epoch: 7, Step: 8: 21%|██▏ | 6/28 [00:00<00:01, 11.84it/s, total_batch_loss=79.87619, average_batch_loss=0.31202, average_batch_perplexity=1.36618, lr=0.001332504, 0.001332504]
Epoch: 7, Step: 8: 29%|██▊ | 8/28 [00:00<00:01, 11.78it/s, total_batch_loss=79.87619, average_batch_loss=0.31202, average_batch_perplexity=1.36618, lr=0.001332504, 0.001332504]
Epoch: 7, Step: 9: 29%|██▊ | 8/28 [00:00<00:01, 11.78it/s, total_batch_loss=79.87619, average_batch_loss=0.31202, average_batch_perplexity=1.36618, lr=0.001332504, 0.001332504]
Epoch: 7, Step: 9: 29%|██▊ | 8/28 [00:00<00:01, 11.78it/s, total_batch_loss=80.34425, average_batch_loss=0.31384, average_batch_perplexity=1.36868, lr=0.001328735, 0.001328735]
Epoch: 7, Step: 10: 29%|██▊ | 8/28 [00:00<00:01, 11.78it/s, total_batch_loss=80.34425, average_batch_loss=0.31384, average_batch_perplexity=1.36868, lr=0.001328735, 0.001328735]
Epoch: 7, Step: 10: 29%|██▊ | 8/28 [00:00<00:01, 11.78it/s, total_batch_loss=86.42328, average_batch_loss=0.33759, average_batch_perplexity=1.40157, lr=0.001324997, 0.001324997]
Epoch: 7, Step: 10: 36%|███▌ | 10/28 [00:00<00:01, 12.06it/s, total_batch_loss=86.42328, average_batch_loss=0.33759, average_batch_perplexity=1.40157, lr=0.001324997, 0.001324997]
Epoch: 7, Step: 11: 36%|███▌ | 10/28 [00:00<00:01, 12.06it/s, total_batch_loss=86.42328, average_batch_loss=0.33759, average_batch_perplexity=1.40157, lr=0.001324997, 0.001324997]
Epoch: 7, Step: 11: 36%|███▌ | 10/28 [00:00<00:01, 12.06it/s, total_batch_loss=78.26029, average_batch_loss=0.30570, average_batch_perplexity=1.35758, lr=0.001321291, 0.001321291]
Epoch: 7, Step: 12: 36%|███▌ | 10/28 [00:00<00:01, 12.06it/s, total_batch_loss=78.26029, average_batch_loss=0.30570, average_batch_perplexity=1.35758, lr=0.001321291, 0.001321291]
Epoch: 7, Step: 12: 36%|███▌ | 10/28 [00:00<00:01, 12.06it/s, total_batch_loss=86.62181, average_batch_loss=0.33837, average_batch_perplexity=1.40265, lr=0.001317616, 0.001317616]
Epoch: 7, Step: 12: 43%|████▎ | 12/28 [00:00<00:01, 12.27it/s, total_batch_loss=86.62181, average_batch_loss=0.33837, average_batch_perplexity=1.40265, lr=0.001317616, 0.001317616]
Epoch: 7, Step: 13: 43%|████▎ | 12/28 [00:01<00:01, 12.27it/s, total_batch_loss=86.62181, average_batch_loss=0.33837, average_batch_perplexity=1.40265, lr=0.001317616, 0.001317616]
Epoch: 7, Step: 13: 43%|████▎ | 12/28 [00:01<00:01, 12.27it/s, total_batch_loss=82.72236, average_batch_loss=0.32313, average_batch_perplexity=1.38145, lr=0.001313971, 0.001313971]
Epoch: 7, Step: 14: 43%|████▎ | 12/28 [00:01<00:01, 12.27it/s, total_batch_loss=82.72236, average_batch_loss=0.32313, average_batch_perplexity=1.38145, lr=0.001313971, 0.001313971]
Epoch: 7, Step: 14: 43%|████▎ | 12/28 [00:01<00:01, 12.27it/s, total_batch_loss=73.91183, average_batch_loss=0.28872, average_batch_perplexity=1.33472, lr=0.001310356, 0.001310356]
Epoch: 7, Step: 14: 50%|█████ | 14/28 [00:01<00:01, 12.01it/s, total_batch_loss=73.91183, average_batch_loss=0.28872, average_batch_perplexity=1.33472, lr=0.001310356, 0.001310356]
Epoch: 7, Step: 15: 50%|█████ | 14/28 [00:01<00:01, 12.01it/s, total_batch_loss=73.91183, average_batch_loss=0.28872, average_batch_perplexity=1.33472, lr=0.001310356, 0.001310356]
Epoch: 7, Step: 15: 50%|█████ | 14/28 [00:01<00:01, 12.01it/s, total_batch_loss=74.20255, average_batch_loss=0.28985, average_batch_perplexity=1.33623, lr=0.001306771, 0.001306771]
Epoch: 7, Step: 16: 50%|█████ | 14/28 [00:01<00:01, 12.01it/s, total_batch_loss=74.20255, average_batch_loss=0.28985, average_batch_perplexity=1.33623, lr=0.001306771, 0.001306771]
Epoch: 7, Step: 16: 50%|█████ | 14/28 [00:01<00:01, 12.01it/s, total_batch_loss=86.90319, average_batch_loss=0.33947, average_batch_perplexity=1.40420, lr=0.001303215, 0.001303215]
Epoch: 7, Step: 16: 57%|█████▋ | 16/28 [00:01<00:01, 11.69it/s, total_batch_loss=86.90319, average_batch_loss=0.33947, average_batch_perplexity=1.40420, lr=0.001303215, 0.001303215]
Epoch: 7, Step: 17: 57%|█████▋ | 16/28 [00:01<00:01, 11.69it/s, total_batch_loss=86.90319, average_batch_loss=0.33947, average_batch_perplexity=1.40420, lr=0.001303215, 0.001303215]
Epoch: 7, Step: 17: 57%|█████▋ | 16/28 [00:01<00:01, 11.69it/s, total_batch_loss=76.07186, average_batch_loss=0.29716, average_batch_perplexity=1.34602, lr=0.001299688, 0.001299688]
Epoch: 7, Step: 18: 57%|█████▋ | 16/28 [00:01<00:01, 11.69it/s, total_batch_loss=76.07186, average_batch_loss=0.29716, average_batch_perplexity=1.34602, lr=0.001299688, 0.001299688]
Epoch: 7, Step: 18: 57%|█████▋ | 16/28 [00:01<00:01, 11.69it/s, total_batch_loss=71.24876, average_batch_loss=0.27832, average_batch_perplexity=1.32090, lr=0.001296190, 0.001296190]
Epoch: 7, Step: 18: 64%|██████▍ | 18/28 [00:01<00:00, 11.81it/s, total_batch_loss=71.24876, average_batch_loss=0.27832, average_batch_perplexity=1.32090, lr=0.001296190, 0.001296190]
Epoch: 7, Step: 19: 64%|██████▍ | 18/28 [00:01<00:00, 11.81it/s, total_batch_loss=71.24876, average_batch_loss=0.27832, average_batch_perplexity=1.32090, lr=0.001296190, 0.001296190]
Epoch: 7, Step: 19: 64%|██████▍ | 18/28 [00:01<00:00, 11.81it/s, total_batch_loss=81.55933, average_batch_loss=0.31859, average_batch_perplexity=1.37519, lr=0.001292719, 0.001292719]
Epoch: 7, Step: 20: 64%|██████▍ | 18/28 [00:01<00:00, 11.81it/s, total_batch_loss=81.55933, average_batch_loss=0.31859, average_batch_perplexity=1.37519, lr=0.001292719, 0.001292719]
Epoch: 7, Step: 20: 64%|██████▍ | 18/28 [00:01<00:00, 11.81it/s, total_batch_loss=72.77303, average_batch_loss=0.28427, average_batch_perplexity=1.32879, lr=0.001289277, 0.001289277]
Epoch: 7, Step: 20: 71%|███████▏ | 20/28 [00:01<00:00, 12.08it/s, total_batch_loss=72.77303, average_batch_loss=0.28427, average_batch_perplexity=1.32879, lr=0.001289277, 0.001289277]
Epoch: 7, Step: 21: 71%|███████▏ | 20/28 [00:01<00:00, 12.08it/s, total_batch_loss=72.77303, average_batch_loss=0.28427, average_batch_perplexity=1.32879, lr=0.001289277, 0.001289277]
Epoch: 7, Step: 21: 71%|███████▏ | 20/28 [00:01<00:00, 12.08it/s, total_batch_loss=76.14906, average_batch_loss=0.29746, average_batch_perplexity=1.34643, lr=0.001285861, 0.001285861]
Epoch: 7, Step: 22: 71%|███████▏ | 20/28 [00:01<00:00, 12.08it/s, total_batch_loss=76.14906, average_batch_loss=0.29746, average_batch_perplexity=1.34643, lr=0.001285861, 0.001285861]
Epoch: 7, Step: 22: 71%|███████▏ | 20/28 [00:01<00:00, 12.08it/s, total_batch_loss=75.58844, average_batch_loss=0.29527, average_batch_perplexity=1.34349, lr=0.001282473, 0.001282473]
Epoch: 7, Step: 22: 79%|███████▊ | 22/28 [00:01<00:00, 11.89it/s, total_batch_loss=75.58844, average_batch_loss=0.29527, average_batch_perplexity=1.34349, lr=0.001282473, 0.001282473]
Epoch: 7, Step: 23: 79%|███████▊ | 22/28 [00:01<00:00, 11.89it/s, total_batch_loss=75.58844, average_batch_loss=0.29527, average_batch_perplexity=1.34349, lr=0.001282473, 0.001282473]
Epoch: 7, Step: 23: 79%|███████▊ | 22/28 [00:01<00:00, 11.89it/s, total_batch_loss=67.71696, average_batch_loss=0.26452, average_batch_perplexity=1.30280, lr=0.001279111, 0.001279111]
Epoch: 7, Step: 24: 79%|███████▊ | 22/28 [00:02<00:00, 11.89it/s, total_batch_loss=67.71696, average_batch_loss=0.26452, average_batch_perplexity=1.30280, lr=0.001279111, 0.001279111]
Epoch: 7, Step: 24: 79%|███████▊ | 22/28 [00:02<00:00, 11.89it/s, total_batch_loss=62.94439, average_batch_loss=0.24588, average_batch_perplexity=1.27874, lr=0.001275776, 0.001275776]
Epoch: 7, Step: 24: 86%|████████▌ | 24/28 [00:02<00:00, 11.96it/s, total_batch_loss=62.94439, average_batch_loss=0.24588, average_batch_perplexity=1.27874, lr=0.001275776, 0.001275776]
Epoch: 7, Step: 25: 86%|████████▌ | 24/28 [00:02<00:00, 11.96it/s, total_batch_loss=62.94439, average_batch_loss=0.24588, average_batch_perplexity=1.27874, lr=0.001275776, 0.001275776]
Epoch: 7, Step: 25: 86%|████████▌ | 24/28 [00:02<00:00, 11.96it/s, total_batch_loss=70.13992, average_batch_loss=0.27398, average_batch_perplexity=1.31519, lr=0.001272466, 0.001272466]
Epoch: 7, Step: 26: 86%|████████▌ | 24/28 [00:02<00:00, 11.96it/s, total_batch_loss=70.13992, average_batch_loss=0.27398, average_batch_perplexity=1.31519, lr=0.001272466, 0.001272466]
Epoch: 7, Step: 26: 86%|████████▌ | 24/28 [00:02<00:00, 11.96it/s, total_batch_loss=72.43277, average_batch_loss=0.28294, average_batch_perplexity=1.32703, lr=0.001269183, 0.001269183]
Epoch: 7, Step: 26: 93%|█████████▎| 26/28 [00:02<00:00, 11.98it/s, total_batch_loss=72.43277, average_batch_loss=0.28294, average_batch_perplexity=1.32703, lr=0.001269183, 0.001269183]
Epoch: 7, Step: 27: 93%|█████████▎| 26/28 [00:02<00:00, 11.98it/s, total_batch_loss=72.43277, average_batch_loss=0.28294, average_batch_perplexity=1.32703, lr=0.001269183, 0.001269183]
Epoch: 7, Step: 27: 93%|█████████▎| 26/28 [00:02<00:00, 11.98it/s, total_batch_loss=65.42526, average_batch_loss=0.25557, average_batch_perplexity=1.29119, lr=0.001265924, 0.001265924]
Epoch: 7, Step: 28: 93%|█████████▎| 26/28 [00:02<00:00, 11.98it/s, total_batch_loss=65.42526, average_batch_loss=0.25557, average_batch_perplexity=1.29119, lr=0.001265924, 0.001265924]
Epoch: 7, Step: 28: 93%|█████████▎| 26/28 [00:02<00:00, 11.98it/s, total_batch_loss=24.33375, average_batch_loss=0.27652, average_batch_perplexity=1.31853, lr=0.001262691, 0.001262691]
Epoch: 7, Step: 28: 100%|██████████| 28/28 [00:02<00:00, 13.18it/s, total_batch_loss=24.33375, average_batch_loss=0.27652, average_batch_perplexity=1.31853, lr=0.001262691, 0.001262691]
2024-12-17 09:44:17,543 - root - INFO - Total Samples: 7000
2024-12-17 09:44:17,544 - root - INFO - Total Batches: 28
2024-12-17 09:44:17,544 - root - INFO - Average Epoch Train Loss: 0.31783
2024-12-17 09:44:17,545 - root - INFO - Average Epoch Train Perplexity: 1.37414
2024-12-17 09:44:17,545 - root - INFO -
2024-12-17 09:44:17,546 - root - INFO - ====================================================== Starting Valid Epoch: 7/9 ======================================================
0%| | 0/8 [00:00<?, ?it/s]
Epoch: 7, Step: 1: 0%| | 0/8 [00:00<?, ?it/s]
Epoch: 7, Step: 1: 0%| | 0/8 [00:00<?, ?it/s, total_batch_loss=51.61576, average_batch_loss=0.20162, average_batch_perplexity=1.22339]
Epoch: 7, Step: 2: 0%| | 0/8 [00:00<?, ?it/s, total_batch_loss=51.61576, average_batch_loss=0.20162, average_batch_perplexity=1.22339]
Epoch: 7, Step: 2: 0%| | 0/8 [00:00<?, ?it/s, total_batch_loss=47.79982, average_batch_loss=0.18672, average_batch_perplexity=1.20529]
Epoch: 7, Step: 2: 25%|██▌ | 2/8 [00:00<00:00, 19.22it/s, total_batch_loss=47.79982, average_batch_loss=0.18672, average_batch_perplexity=1.20529]
Epoch: 7, Step: 3: 25%|██▌ | 2/8 [00:00<00:00, 19.22it/s, total_batch_loss=47.79982, average_batch_loss=0.18672, average_batch_perplexity=1.20529]
Epoch: 7, Step: 3: 25%|██▌ | 2/8 [00:00<00:00, 19.22it/s, total_batch_loss=49.58239, average_batch_loss=0.19368, average_batch_perplexity=1.21371]
Epoch: 7, Step: 4: 25%|██▌ | 2/8 [00:00<00:00, 19.22it/s, total_batch_loss=49.58239, average_batch_loss=0.19368, average_batch_perplexity=1.21371]
Epoch: 7, Step: 4: 25%|██▌ | 2/8 [00:00<00:00, 19.22it/s, total_batch_loss=49.55921, average_batch_loss=0.19359, average_batch_perplexity=1.21360]
Epoch: 7, Step: 5: 25%|██▌ | 2/8 [00:00<00:00, 19.22it/s, total_batch_loss=49.55921, average_batch_loss=0.19359, average_batch_perplexity=1.21360]
Epoch: 7, Step: 5: 25%|██▌ | 2/8 [00:00<00:00, 19.22it/s, total_batch_loss=50.46335, average_batch_loss=0.19712, average_batch_perplexity=1.21789]
Epoch: 7, Step: 5: 62%|██████▎ | 5/8 [00:00<00:00, 21.52it/s, total_batch_loss=50.46335, average_batch_loss=0.19712, average_batch_perplexity=1.21789]
Epoch: 7, Step: 6: 62%|██████▎ | 5/8 [00:00<00:00, 21.52it/s, total_batch_loss=50.46335, average_batch_loss=0.19712, average_batch_perplexity=1.21789]
Epoch: 7, Step: 6: 62%|██████▎ | 5/8 [00:00<00:00, 21.52it/s, total_batch_loss=50.37434, average_batch_loss=0.19677, average_batch_perplexity=1.21747]
Epoch: 7, Step: 7: 62%|██████▎ | 5/8 [00:00<00:00, 21.52it/s, total_batch_loss=50.37434, average_batch_loss=0.19677, average_batch_perplexity=1.21747]
Epoch: 7, Step: 7: 62%|██████▎ | 5/8 [00:00<00:00, 21.52it/s, total_batch_loss=49.94740, average_batch_loss=0.19511, average_batch_perplexity=1.21544]
Epoch: 7, Step: 8: 62%|██████▎ | 5/8 [00:00<00:00, 21.52it/s, total_batch_loss=49.94740, average_batch_loss=0.19511, average_batch_perplexity=1.21544]
Epoch: 7, Step: 8: 62%|██████▎ | 5/8 [00:00<00:00, 21.52it/s, total_batch_loss=38.95474, average_batch_loss=0.18728, average_batch_perplexity=1.20597]
Epoch: 7, Step: 8: 100%|██████████| 8/8 [00:00<00:00, 23.09it/s, total_batch_loss=38.95474, average_batch_loss=0.18728, average_batch_perplexity=1.20597]
2024-12-17 09:44:17,906 - root - INFO - Total Samples: 2000
2024-12-17 09:44:17,906 - root - INFO - Total Batches: 8
2024-12-17 09:44:17,908 - root - INFO - Average Epoch Valid Loss: 0.19415
2024-12-17 09:44:17,908 - root - INFO - Average Epoch Valid Perplexity: 1.21428
2024-12-17 09:44:17,909 - root - INFO -
Evaluating and Generation.: 0%| | 0/4 [00:00<?, ?it/s]
Evaluating and Generation.: 0%| | 0/4 [00:00<?, ?it/s, accuracy: 0.7773]
Evaluating and Generation.: 0%| | 0/4 [00:00<?, ?it/s, accuracy: 0.8008]
Evaluating and Generation.: 50%|█████ | 2/4 [00:00<00:00, 10.50it/s, accuracy: 0.8008]
Evaluating and Generation.: 50%|█████ | 2/4 [00:00<00:00, 10.50it/s, accuracy: 0.8008]
Evaluating and Generation.: 50%|█████ | 2/4 [00:00<00:00, 10.50it/s, accuracy: 0.7845]
Evaluating and Generation.: 100%|██████████| 4/4 [00:00<00:00, 10.83it/s, accuracy: 0.7845]
2024-12-17 09:44:18,285 - root - INFO - Correct/Total Samples: 791/1000
2024-12-17 09:44:18,286 - root - INFO - Eval Accuracy: 0.791
2024-12-17 09:44:18,304 - root - INFO -
epoch batch_index sample_index equation generated correct
7 1 0 13+48=061 13+48=061 True
7 1 1 16+55=071 16+55=071 True
7 1 2 79+34=113 79+34=103 False
7 1 3 35+44=079 35+44=089 False
7 1 4 16+50=066 16+50=065 False
7 1 5 28+47=075 28+47=075 True
7 1 6 00+74=074 00+74=074 True
7 1 7 15+20=035 15+20=035 True
7 1 8 72+60=132 72+60=132 True
7 1 9 63+68=131 63+68=131 True
7 1 10 29+45=074 29+45=074 True
7 1 11 34+60=094 34+60=094 True
7 1 12 53+70=123 53+70=123 True
7 1 13 70+50=120 70+50=120 True
7 1 14 11+84=095 11+84=095 True
7 1 15 42+71=113 42+71=113 True
7 1 16 98+22=120 98+22=120 True
7 1 17 02+02=004 02+02=014 False
7 1 18 15+85=100 15+85=100 True
7 1 19 21+78=099 21+78=099 True
7 1 20 61+79=140 61+79=140 True
7 1 21 25+99=124 25+99=124 True
7 1 22 09+85=094 09+85=094 True
7 1 23 60+91=151 60+91=151 True
7 1 24 35+30=065 35+30=065 True
7 1 25 24+51=075 24+51=075 True
7 1 26 93+91=184 93+91=184 True
7 1 27 39+96=135 39+96=135 True
7 1 28 64+35=099 64+35=099 True
7 1 29 36+22=058 36+22=058 True
7 1 30 68+45=113 68+45=113 True
7 1 31 16+84=100 16+84=100 True
7 1 32 91+52=143 91+52=143 True
7 1 33 97+36=133 97+36=133 True
7 1 34 27+37=064 27+37=064 True
7 1 35 99+82=181 99+82=181 True
7 1 36 03+42=045 03+42=055 False
7 1 37 18+38=056 18+38=056 True
7 1 38 32+20=052 32+20=052 True
7 1 39 38+13=051 38+13=051 True
7 1 40 68+42=110 68+42=110 True
7 1 41 64+00=064 64+00=074 False
7 1 42 48+94=142 48+94=142 True
7 1 43 58+36=094 58+36=094 True
7 1 44 41+22=063 41+22=063 True
7 1 45 23+58=081 23+58=081 True
7 1 46 67+46=113 67+46=113 True
7 1 47 40+78=118 40+78=117 False
7 1 48 90+38=128 90+38=128 True
7 1 49 89+52=141 89+52=141 True
7 1 50 37+77=114 37+77=114 True
7 1 51 29+76=105 29+76=105 True
7 1 52 42+90=132 42+90=132 True
7 1 53 45+82=127 45+82=137 False
7 1 54 35+95=130 35+95=130 True
7 1 55 92+98=190 92+98=180 False
7 1 56 73+91=164 73+91=174 False
7 1 57 53+97=150 53+97=150 True
7 1 58 98+69=167 98+69=167 True
7 1 59 20+46=066 20+46=065 False
7 1 60 48+69=117 48+69=117 True
7 1 61 62+31=093 62+31=092 False
7 1 62 80+59=139 80+59=149 False
7 1 63 58+12=070 58+12=070 True
7 1 64 08+96=104 08+96=104 True
7 1 65 67+06=073 67+06=073 True
7 1 66 22+04=026 22+04=026 True
7 1 67 61+87=148 61+87=158 False
7 1 68 95+27=122 95+27=122 True
7 1 69 49+83=132 49+83=132 True
7 1 70 43+00=043 43+00=053 False
7 1 71 01+85=086 01+85=086 True
7 1 72 11+68=079 11+68=089 False
7 1 73 80+03=083 80+03=083 True
7 1 74 54+83=137 54+83=147 False
7 1 75 73+47=120 73+47=120 True
7 1 76 99+93=192 99+93=192 True
7 1 77 99+13=112 99+13=112 True
7 1 78 92+66=158 92+66=158 True
7 1 79 90+31=121 90+31=121 True
7 1 80 25+69=094 25+69=094 True
7 1 81 25+44=069 25+44=079 False
7 1 82 00+93=093 00+93=093 True
7 1 83 88+87=175 88+87=175 True
7 1 84 47+56=103 47+56=103 True
7 1 85 43+59=102 43+59=102 True
7 1 86 22+00=022 22+00=022 True
7 1 87 34+04=038 34+04=048 False
7 1 88 65+13=078 65+13=078 True
7 1 89 39+82=121 39+82=122 False
7 1 90 66+83=149 66+83=159 False
7 1 91 51+69=120 51+69=120 True
7 1 92 80+21=101 80+21=101 True
7 1 93 36+79=115 36+79=115 True
7 1 94 21+68=089 21+68=089 True
7 1 95 11+66=077 11+66=077 True
7 1 96 55+19=074 55+19=074 True
7 1 97 51+61=112 51+61=112 True
7 1 98 38+88=126 38+88=126 True
7 1 99 37+27=064 37+27=064 True
7 1 100 18+63=081 18+63=081 True
7 1 101 48+11=059 48+11=069 False
7 1 102 72+68=140 72+68=140 True
7 1 103 37+39=076 37+39=076 True
7 1 104 64+95=159 64+95=169 False
7 1 105 49+75=124 49+75=124 True
7 1 106 45+66=111 45+66=111 True
7 1 107 34+87=121 34+87=121 True
7 1 108 02+84=086 02+84=086 True
7 1 109 95+00=095 95+00=095 True
7 1 110 09+56=065 09+56=065 True
7 1 111 22+66=088 22+66=088 True
7 1 112 43+18=061 43+18=071 False
7 1 113 61+35=096 61+35=096 True
7 1 114 13+73=086 13+73=086 True
7 1 115 25+95=120 25+95=120 True
7 1 116 73+96=169 73+96=179 False
7 1 117 03+96=099 03+96=099 True
7 1 118 97+82=179 97+82=189 False
7 1 119 18+42=060 18+42=060 True
7 1 120 29+98=127 29+98=127 True
7 1 121 61+00=061 61+00=061 True
7 1 122 22+98=120 22+98=120 True
7 1 123 12+50=062 12+50=062 True
7 1 124 02+58=060 02+58=060 True
7 1 125 75+86=161 75+86=161 True
7 1 126 31+57=088 31+57=088 True
7 1 127 49+82=131 49+82=131 True
7 1 128 15+33=048 15+33=058 False
7 1 129 49+57=106 49+57=106 True
7 1 130 61+70=131 61+70=131 True
7 1 131 91+51=142 91+51=142 True
7 1 132 50+05=055 50+05=055 True
7 1 133 44+16=060 44+16=060 True
7 1 134 92+01=093 92+01=093 True
7 1 135 85+82=167 85+82=167 True
7 1 136 07+41=048 07+41=058 False
7 1 137 40+06=046 40+06=055 False
7 1 138 79+62=141 79+62=141 True
7 1 139 95+62=157 95+62=157 True
7 1 140 42+93=135 42+93=135 True
7 1 141 32+73=105 32+73=105 True
7 1 142 47+09=056 47+09=056 True
7 1 143 59+50=109 59+50=109 True
7 1 144 61+77=138 61+77=138 True
7 1 145 64+06=070 64+06=070 True
7 1 146 35+10=045 35+10=045 True
7 1 147 32+88=120 32+88=120 True
7 1 148 03+95=098 03+95=098 True
7 1 149 11+38=049 11+38=059 False
7 1 150 21+67=088 21+67=088 True
7 1 151 33+25=058 33+25=058 True
7 1 152 63+45=108 63+45=108 True
7 1 153 56+12=068 56+12=078 False
7 1 154 19+79=098 19+79=098 True
7 1 155 60+43=103 60+43=103 True
7 1 156 07+61=068 07+61=078 False
7 1 157 58+03=061 58+03=061 True
7 1 158 11+10=021 11+10=021 True
7 1 159 49+89=138 49+89=138 True
7 1 160 37+58=095 37+58=095 True
7 1 161 59+78=137 59+78=137 True
7 1 162 11+21=032 11+21=032 True
7 1 163 37+43=080 37+43=080 True
7 1 164 44+21=065 44+21=075 False
7 1 165 22+97=119 22+97=119 True
7 1 166 65+35=100 65+35=090 False
7 1 167 06+51=057 06+51=067 False
7 1 168 65+25=090 65+25=090 True
7 1 169 74+94=168 74+94=178 False
7 1 170 87+55=142 87+55=142 True
7 1 171 90+67=157 90+67=157 True
7 1 172 11+02=013 11+02=023 False
7 1 173 01+66=067 01+66=077 False
7 1 174 56+00=056 56+00=056 True
7 1 175 58+52=110 58+52=110 True
7 1 176 24+99=123 24+99=123 True
7 1 177 97+13=110 97+13=110 True
7 1 178 42+94=136 42+94=136 True
7 1 179 60+15=075 60+15=075 True
7 1 180 20+46=066 20+46=065 False
7 1 181 40+70=110 40+70=110 True
7 1 182 95+45=140 95+45=140 True
7 1 183 96+95=191 96+95=181 False
7 1 184 98+20=118 98+20=118 True
7 1 185 43+19=062 43+19=072 False
7 1 186 50+69=119 50+69=119 True
7 1 187 27+53=080 27+53=080 True
7 1 188 24+25=049 24+25=059 False
7 1 189 65+92=157 65+92=157 True
7 1 190 28+14=042 28+14=042 True
7 1 191 20+57=077 20+57=077 True
7 1 192 59+97=156 59+97=156 True
7 1 193 98+32=130 98+32=130 True
7 1 194 55+84=139 55+84=149 False
7 1 195 20+39=059 20+39=069 False
7 1 196 86+47=133 86+47=133 True
7 1 197 92+36=128 92+36=138 False
7 1 198 05+38=043 05+38=043 True
7 1 199 77+36=113 77+36=113 True
7 1 200 41+64=105 41+64=105 True
7 1 201 74+51=125 74+51=125 True
7 1 202 74+55=129 74+55=129 True
7 1 203 64+64=128 64+64=128 True
7 1 204 60+19=079 60+19=089 False
7 1 205 77+96=173 77+96=173 True
7 1 206 22+30=052 22+30=052 True
7 1 207 82+49=131 82+49=131 True
7 1 208 39+67=106 39+67=106 True
7 1 209 62+40=102 62+40=102 True
7 1 210 28+71=099 28+71=099 True
7 1 211 47+26=073 47+26=073 True
7 1 212 98+54=152 98+54=152 True
7 1 213 38+70=108 38+70=108 True
7 1 214 63+40=103 63+40=103 True
7 1 215 86+62=148 86+62=148 True
7 1 216 22+65=087 22+65=087 True
7 1 217 41+17=058 41+17=068 False
7 1 218 68+88=156 68+88=156 True
7 1 219 96+70=166 96+70=175 False
7 1 220 99+29=128 99+29=128 True
7 1 221 83+39=122 83+39=122 True
7 1 222 26+55=081 26+55=071 False
7 1 223 53+70=123 53+70=123 True
7 1 224 94+12=106 94+12=106 True
7 1 225 00+37=037 00+37=047 False
7 1 226 36+94=130 36+94=130 True
7 1 227 40+58=098 40+58=098 True
7 1 228 19+80=099 19+80=099 True
7 1 229 49+44=093 49+44=093 True
7 1 230 70+27=097 70+27=096 False
7 1 231 52+80=132 52+80=132 True
7 1 232 77+90=167 77+90=177 False
7 1 233 13+92=105 13+92=105 True
7 1 234 59+09=068 59+09=078 False
7 1 235 33+55=088 33+55=088 True
7 1 236 85+16=101 85+16=101 True
7 1 237 25+65=090 25+65=090 True
7 1 238 46+20=066 46+20=075 False
7 1 239 29+52=081 29+52=081 True
7 1 240 32+36=068 32+36=078 False
7 1 241 47+08=055 47+08=055 True
7 1 242 21+84=105 21+84=105 True
7 1 243 24+45=069 24+45=079 False
7 1 244 29+15=044 29+15=044 True
7 1 245 83+03=086 83+03=086 True
7 1 246 83+36=119 83+36=119 True
7 1 247 58+95=153 58+95=153 True
7 1 248 76+79=155 76+79=155 True
7 1 249 63+30=093 63+30=093 True
7 1 250 38+24=062 38+24=062 True
7 1 251 19+46=065 19+46=065 True
7 1 252 99+66=165 99+66=165 True
7 1 253 95+73=168 95+73=178 False
7 1 254 65+27=092 65+27=092 True
7 1 255 91+83=174 91+83=174 True
7 2 0 65+49=114 65+49=114 True
7 2 1 03+08=011 03+08=011 True
7 2 2 67+81=148 67+81=148 True
7 2 3 47+23=070 47+23=070 True
7 2 4 43+91=134 43+91=134 True
7 2 5 41+67=108 41+67=108 True
7 2 6 02+33=035 02+33=045 False
7 2 7 64+84=148 64+84=158 False
7 2 8 81+64=145 81+64=145 True
7 2 9 80+11=091 80+11=091 True
7 2 10 78+01=079 78+01=079 True
7 2 11 89+18=107 89+18=107 True
7 2 12 45+52=097 45+52=097 True
7 2 13 35+30=065 35+30=065 True
7 2 14 53+32=085 53+32=085 True
7 2 15 49+90=139 49+90=149 False
7 2 16 41+37=078 41+37=088 False
7 2 17 35+14=049 35+14=059 False
7 2 18 92+50=142 92+50=142 True
7 2 19 37+60=097 37+60=097 True
7 2 20 91+61=152 91+61=152 True
7 2 21 80+77=157 80+77=157 True
7 2 22 66+24=090 66+24=090 True
7 2 23 81+07=088 81+07=098 False
7 2 24 85+59=144 85+59=144 True
7 2 25 19+69=088 19+69=088 True
7 2 26 91+44=135 91+44=135 True
7 2 27 25+29=054 25+29=054 True
7 2 28 27+08=035 27+08=035 True
7 2 29 66+14=080 66+14=080 True
7 2 30 95+11=106 95+11=106 True
7 2 31 13+97=110 13+97=100 False
7 2 32 94+40=134 94+40=134 True
7 2 33 74+31=105 74+31=105 True
7 2 34 49+00=049 49+00=059 False
7 2 35 59+18=077 59+18=077 True
7 2 36 07+65=072 07+65=072 True
7 2 37 83+55=138 83+55=138 True
7 2 38 49+80=129 49+80=139 False
7 2 39 64+17=081 64+17=081 True
7 2 40 48+83=131 48+83=131 True
7 2 41 95+44=139 95+44=149 False
7 2 42 71+26=097 71+26=097 True
7 2 43 06+74=080 06+74=080 True
7 2 44 34+24=058 34+24=058 True
7 2 45 59+71=130 59+71=130 True
7 2 46 68+32=100 68+32=100 True
7 2 47 38+81=119 38+81=129 False
7 2 48 29+56=085 29+56=085 True
7 2 49 54+55=109 54+55=109 True
7 2 50 31+27=058 31+27=058 True
7 2 51 97+89=186 97+89=186 True
7 2 52 48+09=057 48+09=057 True
7 2 53 86+76=162 86+76=163 False
7 2 54 82+59=141 82+59=141 True
7 2 55 01+67=068 01+67=078 False
7 2 56 26+06=032 26+06=033 False
7 2 57 22+46=068 22+46=078 False
7 2 58 85+16=101 85+16=101 True
7 2 59 29+08=037 29+08=037 True
7 2 60 73+94=167 73+94=177 False
7 2 61 19+62=081 19+62=081 True
7 2 62 86+62=148 86+62=148 True
7 2 63 38+99=137 38+99=137 True
7 2 64 64+25=089 64+25=089 True
7 2 65 61+72=133 61+72=133 True
7 2 66 78+88=166 78+88=166 True
7 2 67 43+66=109 43+66=109 True
7 2 68 69+35=104 69+35=104 True
7 2 69 33+77=110 33+77=100 False
7 2 70 37+37=074 37+37=074 True
7 2 71 87+54=141 87+54=141 True
7 2 72 68+90=158 68+90=158 True
7 2 73 83+44=127 83+44=137 False
7 2 74 41+09=050 41+09=050 True
7 2 75 13+48=061 13+48=061 True
7 2 76 01+41=042 01+41=052 False
7 2 77 19+74=093 19+74=093 True
7 2 78 15+05=020 15+05=020 True
7 2 79 55+46=101 55+46=101 True
7 2 80 68+33=101 68+33=101 True
7 2 81 44+40=084 44+40=084 True
7 2 82 88+03=091 88+03=091 True
7 2 83 81+79=160 81+79=160 True
7 2 84 18+98=116 18+98=116 True
7 2 85 70+64=134 70+64=134 True
7 2 86 26+44=070 26+44=070 True
7 2 87 98+87=185 98+87=185 True
7 2 88 18+74=092 18+74=092 True
7 2 89 50+68=118 50+68=117 False
7 2 90 13+51=064 13+51=064 True
7 2 91 90+89=179 90+89=189 False
7 2 92 47+78=125 47+78=125 True
7 2 93 81+57=138 81+57=138 True
7 2 94 34+47=081 34+47=081 True
7 2 95 94+23=117 94+23=117 True
7 2 96 07+70=077 07+70=077 True
7 2 97 56+33=089 56+33=089 True
7 2 98 33+04=037 33+04=047 False
7 2 99 26+09=035 26+09=035 True
7 2 100 14+92=106 14+92=106 True
7 2 101 78+54=132 78+54=132 True
7 2 102 36+76=112 36+76=103 False
7 2 103 17+47=064 17+47=064 True
7 2 104 28+18=046 28+18=046 True
7 2 105 78+54=132 78+54=132 True
7 2 106 84+72=156 84+72=156 True
7 2 107 00+44=044 00+44=054 False
7 2 108 50+41=091 50+41=091 True
7 2 109 87+88=175 87+88=175 True
7 2 110 11+66=077 11+66=077 True
7 2 111 80+60=140 80+60=140 True
7 2 112 78+76=154 78+76=154 True
7 2 113 24+74=098 24+74=098 True
7 2 114 88+48=136 88+48=136 True
7 2 115 38+31=069 38+31=079 False
7 2 116 29+27=056 29+27=056 True
7 2 117 08+45=053 08+45=053 True
7 2 118 28+13=041 28+13=051 False
7 2 119 53+99=152 53+99=152 True
7 2 120 47+92=139 47+92=149 False
7 2 121 76+21=097 76+21=097 True
7 2 122 53+96=149 53+96=149 True
7 2 123 93+91=184 93+91=184 True
7 2 124 97+33=130 97+33=130 True
7 2 125 67+78=145 67+78=145 True
7 2 126 58+05=063 58+05=063 True
7 2 127 00+16=016 00+16=025 False
7 2 128 80+19=099 80+19=099 True
7 2 129 98+22=120 98+22=120 True
7 2 130 09+62=071 09+62=071 True
7 2 131 06+23=029 06+23=039 False
7 2 132 32+99=131 32+99=131 True
7 2 133 17+02=019 17+02=029 False
7 2 134 64+35=099 64+35=099 True
7 2 135 35+83=118 35+83=118 True
7 2 136 71+36=107 71+36=107 True
7 2 137 75+06=081 75+06=081 True
7 2 138 88+95=183 88+95=183 True
7 2 139 19+98=117 19+98=117 True
7 2 140 28+89=117 28+89=117 True
7 2 141 33+11=044 33+11=044 True
7 2 142 34+49=083 34+49=083 True
7 2 143 90+35=125 90+35=125 True
7 2 144 22+90=112 22+90=112 True
7 2 145 98+89=187 98+89=187 True
7 2 146 88+47=135 88+47=135 True
7 2 147 30+86=116 30+86=115 False
7 2 148 31+48=079 31+48=089 False
7 2 149 39+21=060 39+21=060 True
7 2 150 19+17=036 19+17=036 True
7 2 151 27+60=087 27+60=087 True
7 2 152 12+16=028 12+16=038 False
7 2 153 51+75=126 51+75=126 True
7 2 154 10+74=084 10+74=084 True
7 2 155 42+63=105 42+63=105 True
7 2 156 40+14=054 40+14=054 True
7 2 157 23+93=116 23+93=116 True
7 2 158 85+26=111 85+26=111 True
7 2 159 28+46=074 28+46=074 True
7 2 160 28+33=061 28+33=061 True
7 2 161 43+30=073 43+30=073 True
7 2 162 89+72=161 89+72=161 True
7 2 163 52+21=073 52+21=073 True
7 2 164 21+54=075 21+54=075 True
7 2 165 69+13=082 69+13=082 True
7 2 166 07+60=067 07+60=077 False
7 2 167 63+83=146 63+83=146 True
7 2 168 80+69=149 80+69=159 False
7 2 169 27+28=055 27+28=055 True
7 2 170 42+31=073 42+31=073 True
7 2 171 51+99=150 51+99=150 True
7 2 172 28+75=103 28+75=104 False
7 2 173 38+57=095 38+57=095 True
7 2 174 83+16=099 83+16=099 True
7 2 175 92+94=186 92+94=186 True
7 2 176 55+75=130 55+75=130 True
7 2 177 59+51=110 59+51=100 False
7 2 178 33+09=042 33+09=052 False
7 2 179 53+13=066 53+13=066 True
7 2 180 05+70=075 05+70=075 True
7 2 181 12+20=032 12+20=032 True
7 2 182 11+49=060 11+49=060 True
7 2 183 63+45=108 63+45=108 True
7 2 184 92+23=115 92+23=115 True
7 2 185 82+45=127 82+45=137 False
7 2 186 23+41=064 23+41=064 True
7 2 187 64+26=090 64+26=090 True
7 2 188 91+24=115 91+24=115 True
7 2 189 20+32=052 20+32=052 True
7 2 190 83+21=104 83+21=104 True
7 2 191 07+20=027 07+20=037 False
7 2 192 94+14=108 94+14=108 True
7 2 193 96+89=185 96+89=185 True
7 2 194 13+08=021 13+08=021 True
7 2 195 32+05=037 32+05=047 False
7 2 196 09+51=060 09+51=060 True
7 2 197 26+29=055 26+29=055 True
7 2 198 49+65=114 49+65=114 True
7 2 199 32+66=098 32+66=098 True
7 2 200 41+08=049 41+08=059 False
7 2 201 26+79=105 26+79=105 True
7 2 202 29+91=120 29+91=120 True
7 2 203 51+00=051 51+00=051 True
7 2 204 61+60=121 61+60=121 True
7 2 205 45+78=123 45+78=123 True
7 2 206 56+16=072 56+16=073 False
7 2 207 66+68=134 66+68=134 True
7 2 208 32+16=048 32+16=058 False
7 2 209 84+49=133 84+49=133 True
7 2 210 45+09=054 45+09=054 True
7 2 211 96+78=174 96+78=174 True
7 2 212 10+02=012 10+02=022 False
7 2 213 36+60=096 36+60=096 True
7 2 214 44+36=080 44+36=080 True
7 2 215 12+86=098 12+86=098 True
7 2 216 94+54=148 94+54=148 True
7 2 217 64+73=137 64+73=137 True
7 2 218 73+10=083 73+10=083 True
7 2 219 14+62=076 14+62=076 True
7 2 220 25+22=047 25+22=057 False
7 2 221 94+22=116 94+22=116 True
7 2 222 41+76=117 41+76=117 True
7 2 223 38+46=084 38+46=084 True
7 2 224 71+72=143 71+72=143 True
7 2 225 74+79=153 74+79=153 True
7 2 226 99+67=166 99+67=166 True
7 2 227 78+71=149 78+71=159 False
7 2 228 23+19=042 23+19=042 True
7 2 229 51+65=116 51+65=116 True
7 2 230 94+86=180 94+86=180 True
7 2 231 09+79=088 09+79=088 True
7 2 232 69+39=108 69+39=108 True
7 2 233 84+13=097 84+13=097 True
7 2 234 36+59=095 36+59=095 True
7 2 235 87+47=134 87+47=134 True
7 2 236 50+00=050 50+00=050 True
7 2 237 76+96=172 76+96=173 False
7 2 238 12+18=030 12+18=030 True
7 2 239 99+95=194 99+95=184 False
7 2 240 22+00=022 22+00=022 True
7 2 241 96+18=114 96+18=114 True
7 2 242 51+20=071 51+20=071 True
7 2 243 66+81=147 66+81=147 True
7 2 244 78+18=096 78+18=096 True
7 2 245 09+78=087 09+78=087 True
7 2 246 24+20=044 24+20=044 True
7 2 247 76+13=089 76+13=089 True
7 2 248 05+10=015 05+10=015 True
7 2 249 97+14=111 97+14=111 True
7 2 250 92+38=130 92+38=130 True
7 2 251 77+13=090 77+13=090 True
7 2 252 70+19=089 70+19=099 False
7 2 253 90+45=135 90+45=135 True
7 2 254 50+09=059 50+09=069 False
7 2 255 78+06=084 78+06=084 True
7 3 0 03+25=028 03+25=028 True
7 3 1 48+43=091 48+43=091 True
7 3 2 39+47=086 39+47=086 True
7 3 3 48+19=067 48+19=067 True
7 3 4 07+22=029 07+22=039 False
7 3 5 73+68=141 73+68=141 True
7 3 6 14+56=070 14+56=070 True
7 3 7 96+95=191 96+95=181 False
7 3 8 96+28=124 96+28=124 True
7 3 9 82+05=087 82+05=097 False
7 3 10 27+94=121 27+94=121 True
7 3 11 87+86=173 87+86=173 True
7 3 12 00+68=068 00+68=078 False
7 3 13 11+37=048 11+37=058 False
7 3 14 95+93=188 95+93=188 True
7 3 15 75+82=157 75+82=157 True
7 3 16 41+71=112 41+71=112 True
7 3 17 60+14=074 60+14=074 True
7 3 18 77+77=154 77+77=154 True
7 3 19 31+84=115 31+84=115 True
7 3 20 31+57=088 31+57=088 True
7 3 21 27+87=114 27+87=114 True
7 3 22 31+89=120 31+89=120 True
7 3 23 22+18=040 22+18=040 True
7 3 24 38+25=063 38+25=063 True
7 3 25 64+54=118 64+54=118 True
7 3 26 85+60=145 85+60=145 True
7 3 27 14+71=085 14+71=085 True
7 3 28 06+16=022 06+16=023 False
7 3 29 78+61=139 78+61=149 False
7 3 30 65+75=140 65+75=140 True
7 3 31 13+83=096 13+83=096 True
7 3 32 75+49=124 75+49=124 True
7 3 33 05+78=083 05+78=083 True
7 3 34 66+55=121 66+55=121 True
7 3 35 03+05=008 03+05=007 False
7 3 36 69+99=168 69+99=179 False
7 3 37 52+82=134 52+82=134 True
7 3 38 45+97=142 45+97=142 True
7 3 39 66+17=083 66+17=083 True
7 3 40 36+17=053 36+17=053 True
7 3 41 92+74=166 92+74=176 False
7 3 42 48+44=092 48+44=092 True
7 3 43 34+17=051 34+17=051 True
7 3 44 56+11=067 56+11=077 False
7 3 45 77+23=100 77+23=090 False
7 3 46 10+11=021 10+11=021 True
7 3 47 32+65=097 32+65=097 True
7 3 48 53+49=102 53+49=102 True
7 3 49 68+86=154 68+86=154 True
7 3 50 52+94=146 52+94=146 True
7 3 51 97+71=168 97+71=178 False
7 3 52 05+37=042 05+37=052 False
7 3 53 58+75=133 58+75=133 True
7 3 54 06+24=030 06+24=030 True
7 3 55 15+44=059 15+44=069 False
7 3 56 90+49=139 90+49=149 False
7 3 57 50+37=087 50+37=087 True
7 3 58 88+61=149 88+61=159 False
7 3 59 21+57=078 21+57=078 True
7 3 60 24+85=109 24+85=109 True
7 3 61 01+66=067 01+66=077 False
7 3 62 50+46=096 50+46=095 False
7 3 63 76+65=141 76+65=141 True
7 3 64 23+74=097 23+74=097 True
7 3 65 76+16=092 76+16=093 False
7 3 66 06+08=014 06+08=014 True
7 3 67 69+25=094 69+25=094 True
7 3 68 15+23=038 15+23=048 False
7 3 69 41+02=043 41+02=053 False
7 3 70 16+66=082 16+66=082 True
7 3 71 59+94=153 59+94=153 True
7 3 72 32+88=120 32+88=120 True
7 3 73 46+21=067 46+21=077 False
7 3 74 57+28=085 57+28=085 True
7 3 75 00+31=031 00+31=031 True
7 3 76 77+07=084 77+07=084 True
7 3 77 28+70=098 28+70=098 True
7 3 78 05+61=066 05+61=076 False
7 3 79 22+09=031 22+09=031 True
7 3 80 08+94=102 08+94=102 True
7 3 81 40+11=051 40+11=051 True
7 3 82 10+48=058 10+48=058 True
7 3 83 27+56=083 27+56=083 True
7 3 84 42+16=058 42+16=068 False
7 3 85 69+43=112 69+43=112 True
7 3 86 57+69=126 57+69=126 True
7 3 87 18+86=104 18+86=104 True
7 3 88 86+80=166 86+80=165 False
7 3 89 30+85=115 30+85=115 True
7 3 90 77+66=143 77+66=143 True
7 3 91 39+64=103 39+64=103 True
7 3 92 76+61=137 76+61=137 True
7 3 93 42+61=103 42+61=103 True
7 3 94 07+30=037 07+30=047 False
7 3 95 35+93=128 35+93=138 False
7 3 96 40+90=130 40+90=130 True
7 3 97 08+91=099 08+91=099 True
7 3 98 62+34=096 62+34=096 True
7 3 99 86+49=135 86+49=135 True
7 3 100 73+23=096 73+23=096 True
7 3 101 87+35=122 87+35=122 True
7 3 102 35+31=066 35+31=076 False
7 3 103 07+13=020 07+13=020 True
7 3 104 39+41=080 39+41=080 True
7 3 105 44+63=107 44+63=107 True
7 3 106 94+66=160 94+66=160 True
7 3 107 49+54=103 49+54=103 True
7 3 108 79+46=125 79+46=125 True
7 3 109 53+12=065 53+12=065 True
7 3 110 60+92=152 60+92=152 True
7 3 111 25+60=085 25+60=085 True
7 3 112 64+53=117 64+53=117 True
7 3 113 41+02=043 41+02=053 False
7 3 114 00+97=097 00+97=096 False
7 3 115 12+52=064 12+52=064 True
7 3 116 39+50=089 39+50=089 True
7 3 117 87+21=108 87+21=108 True
7 3 118 04+99=103 04+99=103 True
7 3 119 19+75=094 19+75=094 True
7 3 120 90+05=095 90+05=095 True
7 3 121 54+39=093 54+39=093 True
7 3 122 29+26=055 29+26=055 True
7 3 123 82+95=177 82+95=177 True
7 3 124 55+09=064 55+09=064 True
7 3 125 02+62=064 02+62=064 True
7 3 126 68+30=098 68+30=098 True
7 3 127 99+16=115 99+16=115 True
7 3 128 63+11=074 63+11=074 True
7 3 129 42+92=134 42+92=134 True
7 3 130 99+16=115 99+16=115 True
7 3 131 50+31=081 50+31=081 True
7 3 132 23+46=069 23+46=079 False
7 3 133 45+73=118 45+73=118 True
7 3 134 89+77=166 89+77=166 True
7 3 135 45+78=123 45+78=123 True
7 3 136 96+60=156 96+60=156 True
7 3 137 74+61=135 74+61=135 True
7 3 138 87+01=088 87+01=098 False
7 3 139 63+88=151 63+88=151 True
7 3 140 59+72=131 59+72=131 True
7 3 141 17+96=113 17+96=113 True
7 3 142 89+77=166 89+77=166 True
7 3 143 24+69=093 24+69=093 True
7 3 144 75+83=158 75+83=158 True
7 3 145 50+54=104 50+54=104 True
7 3 146 93+47=140 93+47=140 True
7 3 147 20+55=075 20+55=075 True
7 3 148 91+79=170 91+79=170 True
7 3 149 15+13=028 15+13=028 True
7 3 150 86+09=095 86+09=095 True
7 3 151 29+58=087 29+58=087 True
7 3 152 01+29=030 01+29=030 True
7 3 153 65+48=113 65+48=113 True
7 3 154 96+45=141 96+45=141 True
7 3 155 58+69=127 58+69=127 True
7 3 156 84+43=127 84+43=137 False
7 3 157 90+38=128 90+38=128 True
7 3 158 39+97=136 39+97=136 True
7 3 159 74+84=158 74+84=158 True
7 3 160 86+22=108 86+22=108 True
7 3 161 01+86=087 01+86=097 False
7 3 162 81+63=144 81+63=144 True
7 3 163 80+94=174 80+94=174 True
7 3 164 44+42=086 44+42=086 True
7 3 165 72+60=132 72+60=132 True
7 3 166 28+07=035 28+07=035 True
7 3 167 69+54=123 69+54=123 True
7 3 168 68+77=145 68+77=145 True
7 3 169 90+16=106 90+16=106 True
7 3 170 64+50=114 64+50=114 True
7 3 171 46+88=134 46+88=134 True
7 3 172 55+99=154 55+99=154 True
7 3 173 31+97=128 31+97=138 False
7 3 174 79+28=107 79+28=107 True
7 3 175 81+43=124 81+43=124 True
7 3 176 41+15=056 41+15=066 False
7 3 177 38+77=115 38+77=115 True
7 3 178 25+06=031 25+06=031 True
7 3 179 01+93=094 01+93=094 True
7 3 180 97+22=119 97+22=119 True
7 3 181 71+84=155 71+84=155 True
7 3 182 26+36=062 26+36=062 True
7 3 183 60+92=152 60+92=152 True
7 3 184 02+94=096 02+94=096 True
7 3 185 31+58=089 31+58=099 False
7 3 186 70+52=122 70+52=122 True
7 3 187 19+42=061 19+42=061 True
7 3 188 95+73=168 95+73=178 False
7 3 189 21+25=046 21+25=056 False
7 3 190 13+58=071 13+58=071 True
7 3 191 62+28=090 62+28=090 True
7 3 192 38+14=052 38+14=052 True
7 3 193 66+75=141 66+75=141 True
7 3 194 24+59=083 24+59=083 True
7 3 195 97+66=163 97+66=163 True
7 3 196 76+70=146 76+70=146 True
7 3 197 08+40=048 08+40=058 False
7 3 198 84+00=084 84+00=094 False
7 3 199 54+73=127 54+73=127 True
7 3 200 16+88=104 16+88=104 True
7 3 201 99+47=146 99+47=146 True
7 3 202 31+95=126 31+95=126 True
7 3 203 01+79=080 01+79=080 True
7 3 204 03+68=071 03+68=071 True
7 3 205 10+05=015 10+05=015 True
7 3 206 98+90=188 98+90=188 True
7 3 207 58+53=111 58+53=111 True
7 3 208 34+87=121 34+87=121 True
7 3 209 07+31=038 07+31=048 False
7 3 210 59+08=067 59+08=067 True
7 3 211 51+38=089 51+38=099 False
7 3 212 62+62=124 62+62=124 True
7 3 213 80+32=112 80+32=112 True
7 3 214 69+16=085 69+16=085 True
7 3 215 01+17=018 01+17=027 False
7 3 216 74+41=115 74+41=115 True
7 3 217 20+89=109 20+89=109 True
7 3 218 53+50=103 53+50=103 True
7 3 219 82+85=167 82+85=167 True
7 3 220 34+47=081 34+47=081 True
7 3 221 34+45=079 34+45=089 False
7 3 222 77+34=111 77+34=111 True
7 3 223 56+33=089 56+33=089 True
7 3 224 97+56=153 97+56=153 True
7 3 225 29+06=035 29+06=035 True
7 3 226 78+96=174 78+96=174 True
7 3 227 28+65=093 28+65=093 True
7 3 228 61+64=125 61+64=125 True
7 3 229 32+64=096 32+64=096 True
7 3 230 98+32=130 98+32=130 True
7 3 231 25+35=060 25+35=060 True
7 3 232 05+08=013 05+08=013 True
7 3 233 05+26=031 05+26=031 True
7 3 234 84+71=155 84+71=155 True
7 3 235 33+10=043 33+10=043 True
7 3 236 98+35=133 98+35=133 True
7 3 237 68+98=166 68+98=166 True
7 3 238 03+63=066 03+63=076 False
7 3 239 12+96=108 12+96=108 True
7 3 240 02+81=083 02+81=083 True
7 3 241 83+13=096 83+13=096 True
7 3 242 55+92=147 55+92=147 True
7 3 243 96+09=105 96+09=105 True
7 3 244 61+08=069 61+08=079 False
7 3 245 39+75=114 39+75=114 True
7 3 246 40+74=114 40+74=114 True
7 3 247 39+80=119 39+80=129 False
7 3 248 57+95=152 57+95=152 True
7 3 249 92+97=189 92+97=189 True
7 3 250 33+03=036 33+03=046 False
7 3 251 74+92=166 74+92=176 False
7 3 252 99+09=108 99+09=108 True
7 3 253 98+10=108 98+10=108 True
7 3 254 46+77=123 46+77=123 True
7 3 255 85+78=163 85+78=163 True
7 4 0 41+21=062 41+21=062 True
7 4 1 49+13=062 49+13=062 True
7 4 2 59+07=066 59+07=066 True
7 4 3 31+11=042 31+11=042 True
7 4 4 74+16=090 74+16=090 True
7 4 5 43+38=081 43+38=081 True
7 4 6 08+67=075 08+67=075 True
7 4 7 31+66=097 31+66=097 True
7 4 8 10+31=041 10+31=041 True
7 4 9 34+59=093 34+59=093 True
7 4 10 78+42=120 78+42=120 True
7 4 11 13+41=054 13+41=054 True
7 4 12 97+89=186 97+89=186 True
7 4 13 15+62=077 15+62=077 True
7 4 14 39+36=075 39+36=075 True
7 4 15 21+25=046 21+25=056 False
7 4 16 74+56=130 74+56=130 True
7 4 17 85+47=132 85+47=132 True
7 4 18 47+32=079 47+32=089 False
7 4 19 37+66=103 37+66=103 True
7 4 20 16+29=045 16+29=045 True
7 4 21 86+77=163 86+77=163 True
7 4 22 80+07=087 80+07=096 False
7 4 23 87+05=092 87+05=092 True
7 4 24 58+16=074 58+16=074 True
7 4 25 52+79=131 52+79=131 True
7 4 26 91+08=099 91+08=099 True
7 4 27 47+78=125 47+78=125 True
7 4 28 86+96=182 86+96=183 False
7 4 29 90+22=112 90+22=112 True
7 4 30 31+18=049 31+18=059 False
7 4 31 86+15=101 86+15=101 True
7 4 32 15+95=110 15+95=100 False
7 4 33 42+11=053 42+11=053 True
7 4 34 65+99=164 65+99=164 True
7 4 35 89+29=118 89+29=118 True
7 4 36 35+11=046 35+11=056 False
7 4 37 71+41=112 71+41=112 True
7 4 38 16+24=040 16+24=040 True
7 4 39 77+82=159 77+82=169 False
7 4 40 55+89=144 55+89=144 True
7 4 41 17+88=105 17+88=105 True
7 4 42 54+72=126 54+72=126 True
7 4 43 34+98=132 34+98=132 True
7 4 44 09+97=106 09+97=106 True
7 4 45 91+07=098 91+07=098 True
7 4 46 55+94=149 55+94=159 False
7 4 47 22+58=080 22+58=080 True
7 4 48 91+37=128 91+37=138 False
7 4 49 16+10=026 16+10=025 False
7 4 50 96+32=128 96+32=138 False
7 4 51 35+75=110 35+75=100 False
7 4 52 88+73=161 88+73=161 True
7 4 53 35+18=053 35+18=053 True
7 4 54 33+10=043 33+10=043 True
7 4 55 08+50=058 08+50=068 False
7 4 56 22+62=084 22+62=084 True
7 4 57 26+37=063 26+37=063 True
7 4 58 80+27=107 80+27=107 True
7 4 59 68+28=096 68+28=096 True
7 4 60 48+03=051 48+03=061 False
7 4 61 40+18=058 40+18=068 False
7 4 62 16+59=075 16+59=075 True
7 4 63 02+19=021 02+19=021 True
7 4 64 01+09=010 01+09=010 True
7 4 65 62+68=130 62+68=130 True
7 4 66 09+71=080 09+71=080 True
7 4 67 00+58=058 00+58=068 False
7 4 68 16+45=061 16+45=061 True
7 4 69 24+98=122 24+98=122 True
7 4 70 47+92=139 47+92=149 False
7 4 71 94+84=178 94+84=178 True
7 4 72 21+32=053 21+32=053 True
7 4 73 29+82=111 29+82=112 False
7 4 74 32+79=111 32+79=111 True
7 4 75 13+98=111 13+98=111 True
7 4 76 41+94=135 41+94=135 True
7 4 77 51+84=135 51+84=135 True
7 4 78 42+05=047 42+05=057 False
7 4 79 39+03=042 39+03=042 True
7 4 80 02+92=094 02+92=094 True
7 4 81 99+81=180 99+81=180 True
7 4 82 32+68=100 32+68=100 True
7 4 83 52+17=069 52+17=079 False
7 4 84 56+58=114 56+58=114 True
7 4 85 21+48=069 21+48=079 False
7 4 86 61+71=132 61+71=132 True
7 4 87 17+01=018 17+01=028 False
7 4 88 68+23=091 68+23=091 True
7 4 89 00+37=037 00+37=047 False
7 4 90 94+88=182 94+88=182 True
7 4 91 06+31=037 06+31=047 False
7 4 92 27+18=045 27+18=045 True
7 4 93 41+81=122 41+81=122 True
7 4 94 15+86=101 15+86=101 True
7 4 95 36+87=123 36+87=123 True
7 4 96 17+37=054 17+37=054 True
7 4 97 13+86=099 13+86=099 True
7 4 98 29+69=098 29+69=098 True
7 4 99 31+99=130 31+99=130 True
7 4 100 47+29=076 47+29=076 True
7 4 101 08+81=089 08+81=099 False
7 4 102 72+82=154 72+82=154 True
7 4 103 46+91=137 46+91=137 True
7 4 104 70+35=105 70+35=105 True
7 4 105 90+55=145 90+55=145 True
7 4 106 99+99=198 99+99=199 False
7 4 107 60+97=157 60+97=157 True
7 4 108 03+40=043 03+40=053 False
7 4 109 35+49=084 35+49=084 True
7 4 110 32+02=034 32+02=044 False
7 4 111 70+18=088 70+18=088 True
7 4 112 99+05=104 99+05=104 True
7 4 113 78+73=151 78+73=151 True
7 4 114 03+02=005 03+02=005 True
7 4 115 50+14=064 50+14=064 True
7 4 116 62+02=064 62+02=064 True
7 4 117 16+74=090 16+74=090 True
7 4 118 68+65=133 68+65=133 True
7 4 119 74+81=155 74+81=155 True
7 4 120 37+48=085 37+48=085 True
7 4 121 63+04=067 63+04=077 False
7 4 122 06+62=068 06+62=078 False
7 4 123 95+75=170 95+75=170 True
7 4 124 92+37=129 92+37=139 False
7 4 125 81+32=113 81+32=113 True
7 4 126 53+28=081 53+28=081 True
7 4 127 52+42=094 52+42=094 True
7 4 128 66+97=163 66+97=163 True
7 4 129 00+48=048 00+48=058 False
7 4 130 65+32=097 65+32=097 True
7 4 131 60+89=149 60+89=159 False
7 4 132 71+61=132 71+61=132 True
7 4 133 98+50=148 98+50=148 True
7 4 134 90+96=186 90+96=186 True
7 4 135 02+96=098 02+96=098 True
7 4 136 62+75=137 62+75=137 True
7 4 137 41+28=069 41+28=079 False
7 4 138 95+79=174 95+79=174 True
7 4 139 48+41=089 48+41=089 True
7 4 140 87+95=182 87+95=182 True
7 4 141 75+38=113 75+38=113 True
7 4 142 31+55=086 31+55=086 True
7 4 143 54+63=117 54+63=117 True
7 4 144 75+82=157 75+82=157 True
7 4 145 46+45=091 46+45=091 True
7 4 146 13+08=021 13+08=021 True
7 4 147 77+97=174 77+97=174 True
7 4 148 37+35=072 37+35=072 True
7 4 149 21+89=110 21+89=110 True
7 4 150 58+51=109 58+51=109 True
7 4 151 91+48=139 91+48=149 False
7 4 152 33+23=056 33+23=056 True
7 4 153 80+96=176 80+96=175 False
7 4 154 78+02=080 78+02=080 True
7 4 155 38+95=133 38+95=133 True
7 4 156 99+25=124 99+25=124 True
7 4 157 30+76=106 30+76=106 True
7 4 158 42+40=082 42+40=082 True
7 4 159 85+58=143 85+58=143 True
7 4 160 44+46=090 44+46=090 True
7 4 161 06+41=047 06+41=057 False
7 4 162 65+90=155 65+90=155 True
7 4 163 43+83=126 43+83=136 False
7 4 164 36+61=097 36+61=097 True
7 4 165 61+51=112 61+51=112 True
7 4 166 38+09=047 38+09=047 True
7 4 167 21+97=118 21+97=117 False
7 4 168 83+30=113 83+30=113 True
7 4 169 11+79=090 11+79=090 True
7 4 170 14+29=043 14+29=043 True
7 4 171 21+11=032 21+11=032 True
7 4 172 43+53=096 43+53=096 True
7 4 173 02+58=060 02+58=060 True
7 4 174 78+82=160 78+82=160 True
7 4 175 91+11=102 91+11=102 True
7 4 176 58+54=112 58+54=112 True
7 4 177 00+15=015 00+15=025 False
7 4 178 83+51=134 83+51=134 True
7 4 179 44+72=116 44+72=116 True
7 4 180 71+20=091 71+20=091 True
7 4 181 24+99=123 24+99=123 True
7 4 182 46+30=076 46+30=075 False
7 4 183 08+67=075 08+67=075 True
7 4 184 47+42=089 47+42=089 True
7 4 185 95+67=162 95+67=162 True
7 4 186 40+56=096 40+56=095 False
7 4 187 17+95=112 17+95=112 True
7 4 188 94+66=160 94+66=160 True
7 4 189 14+58=072 14+58=072 True
7 4 190 56+05=061 56+05=061 True
7 4 191 70+01=071 70+01=071 True
7 4 192 97+59=156 97+59=156 True
7 4 193 94+67=161 94+67=161 True
7 4 194 13+41=054 13+41=054 True
7 4 195 85+15=100 85+15=100 True
7 4 196 48+53=101 48+53=101 True
7 4 197 62+75=137 62+75=137 True
7 4 198 87+47=134 87+47=134 True
7 4 199 31+88=119 31+88=129 False
7 4 200 97+16=113 97+16=113 True
7 4 201 48+45=093 48+45=093 True
7 4 202 99+00=099 99+00=099 True
7 4 203 15+01=016 15+01=026 False
7 4 204 28+96=124 28+96=124 True
7 4 205 20+11=031 20+11=031 True
7 4 206 07+56=063 07+56=063 True
7 4 207 06+08=014 06+08=014 True
7 4 208 45+46=091 45+46=091 True
7 4 209 48+85=133 48+85=133 True
7 4 210 62+14=076 62+14=076 True
7 4 211 82+31=113 82+31=113 True
7 4 212 85+88=173 85+88=173 True
7 4 213 77+08=085 77+08=085 True
7 4 214 16+64=080 16+64=080 True
7 4 215 00+27=027 00+27=036 False
7 4 216 36+75=111 36+75=111 True
7 4 217 38+38=076 38+38=076 True
7 4 218 88+32=120 88+32=120 True
7 4 219 09+88=097 09+88=097 True
7 4 220 96+87=183 96+87=183 True
7 4 221 71+29=100 71+29=090 False
7 4 222 99+13=112 99+13=112 True
7 4 223 03+13=016 03+13=026 False
7 4 224 67+23=090 67+23=090 True
7 4 225 15+98=113 15+98=113 True
7 4 226 10+08=018 10+08=028 False
7 4 227 46+24=070 46+24=070 True
7 4 228 55+63=118 55+63=118 True
7 4 229 28+06=034 28+06=034 True
7 4 230 43+87=130 43+87=130 True
7 4 231 34+05=039 34+05=049 False
2024-12-17 09:44:18,305 - root - WARNING - The method `test_one_epoch` is not implemented. Please override this method in a subclass or use a custom callback.
2024-12-17 09:44:18,307 - root - INFO - ====================================================== Starting Train Epoch: 8/9 ======================================================
2024-12-17 09:44:18,307 - root - INFO - Learning rates for each parameter group: 0.00125948180464969628, 0.00125948180464969628
0%| | 0/28 [00:00<?, ?it/s]
Epoch: 8, Step: 1: 0%| | 0/28 [00:00<?, ?it/s]
Epoch: 8, Step: 1: 0%| | 0/28 [00:00<?, ?it/s, total_batch_loss=70.02212, average_batch_loss=0.27352, average_batch_perplexity=1.31459, lr=0.001259482, 0.001259482]
Epoch: 8, Step: 2: 0%| | 0/28 [00:00<?, ?it/s, total_batch_loss=70.02212, average_batch_loss=0.27352, average_batch_perplexity=1.31459, lr=0.001259482, 0.001259482]
Epoch: 8, Step: 2: 0%| | 0/28 [00:00<?, ?it/s, total_batch_loss=64.04005, average_batch_loss=0.25016, average_batch_perplexity=1.28423, lr=0.001256297, 0.001256297]
Epoch: 8, Step: 2: 7%|▋ | 2/28 [00:00<00:02, 11.43it/s, total_batch_loss=64.04005, average_batch_loss=0.25016, average_batch_perplexity=1.28423, lr=0.001256297, 0.001256297]
Epoch: 8, Step: 3: 7%|▋ | 2/28 [00:00<00:02, 11.43it/s, total_batch_loss=64.04005, average_batch_loss=0.25016, average_batch_perplexity=1.28423, lr=0.001256297, 0.001256297]
Epoch: 8, Step: 3: 7%|▋ | 2/28 [00:00<00:02, 11.43it/s, total_batch_loss=69.81609, average_batch_loss=0.27272, average_batch_perplexity=1.31353, lr=0.001253137, 0.001253137]
2024-12-17 09:44:18,651 - root - INFO - Epoch: 8, Step: 200, Avg Batch Loss: 0.23207, Avg Batch Perplexity: 1.26121, LR: 0.001250000
Epoch: 8, Step: 4: 7%|▋ | 2/28 [00:00<00:02, 11.43it/s, total_batch_loss=69.81609, average_batch_loss=0.27272, average_batch_perplexity=1.31353, lr=0.001253137, 0.001253137]
Epoch: 8, Step: 4: 7%|▋ | 2/28 [00:00<00:02, 11.43it/s, total_batch_loss=59.41085, average_batch_loss=0.23207, average_batch_perplexity=1.26121, lr=0.001250000, 0.001250000]
Epoch: 8, Step: 4: 14%|█▍ | 4/28 [00:00<00:02, 11.67it/s, total_batch_loss=59.41085, average_batch_loss=0.23207, average_batch_perplexity=1.26121, lr=0.001250000, 0.001250000]
Epoch: 8, Step: 5: 14%|█▍ | 4/28 [00:00<00:02, 11.67it/s, total_batch_loss=59.41085, average_batch_loss=0.23207, average_batch_perplexity=1.26121, lr=0.001250000, 0.001250000]
Epoch: 8, Step: 5: 14%|█▍ | 4/28 [00:00<00:02, 11.67it/s, total_batch_loss=65.20641, average_batch_loss=0.25471, average_batch_perplexity=1.29009, lr=0.001246887, 0.001246887]
Epoch: 8, Step: 6: 14%|█▍ | 4/28 [00:00<00:02, 11.67it/s, total_batch_loss=65.20641, average_batch_loss=0.25471, average_batch_perplexity=1.29009, lr=0.001246887, 0.001246887]
Epoch: 8, Step: 6: 14%|█▍ | 4/28 [00:00<00:02, 11.67it/s, total_batch_loss=58.38728, average_batch_loss=0.22808, average_batch_perplexity=1.25618, lr=0.001243796, 0.001243796]
Epoch: 8, Step: 6: 21%|██▏ | 6/28 [00:00<00:01, 12.00it/s, total_batch_loss=58.38728, average_batch_loss=0.22808, average_batch_perplexity=1.25618, lr=0.001243796, 0.001243796]
Epoch: 8, Step: 7: 21%|██▏ | 6/28 [00:00<00:01, 12.00it/s, total_batch_loss=58.38728, average_batch_loss=0.22808, average_batch_perplexity=1.25618, lr=0.001243796, 0.001243796]
Epoch: 8, Step: 7: 21%|██▏ | 6/28 [00:00<00:01, 12.00it/s, total_batch_loss=65.68538, average_batch_loss=0.25658, average_batch_perplexity=1.29251, lr=0.001240729, 0.001240729]
Epoch: 8, Step: 8: 21%|██▏ | 6/28 [00:00<00:01, 12.00it/s, total_batch_loss=65.68538, average_batch_loss=0.25658, average_batch_perplexity=1.29251, lr=0.001240729, 0.001240729]
Epoch: 8, Step: 8: 21%|██▏ | 6/28 [00:00<00:01, 12.00it/s, total_batch_loss=64.49298, average_batch_loss=0.25193, average_batch_perplexity=1.28650, lr=0.001237684, 0.001237684]
Epoch: 8, Step: 8: 29%|██▊ | 8/28 [00:00<00:01, 12.13it/s, total_batch_loss=64.49298, average_batch_loss=0.25193, average_batch_perplexity=1.28650, lr=0.001237684, 0.001237684]
Epoch: 8, Step: 9: 29%|██▊ | 8/28 [00:00<00:01, 12.13it/s, total_batch_loss=64.49298, average_batch_loss=0.25193, average_batch_perplexity=1.28650, lr=0.001237684, 0.001237684]
Epoch: 8, Step: 9: 29%|██▊ | 8/28 [00:00<00:01, 12.13it/s, total_batch_loss=56.49179, average_batch_loss=0.22067, average_batch_perplexity=1.24691, lr=0.001234662, 0.001234662]
Epoch: 8, Step: 10: 29%|██▊ | 8/28 [00:00<00:01, 12.13it/s, total_batch_loss=56.49179, average_batch_loss=0.22067, average_batch_perplexity=1.24691, lr=0.001234662, 0.001234662]
Epoch: 8, Step: 10: 29%|██▊ | 8/28 [00:00<00:01, 12.13it/s, total_batch_loss=63.72670, average_batch_loss=0.24893, average_batch_perplexity=1.28266, lr=0.001231662, 0.001231662]
Epoch: 8, Step: 10: 36%|███▌ | 10/28 [00:00<00:01, 12.12it/s, total_batch_loss=63.72670, average_batch_loss=0.24893, average_batch_perplexity=1.28266, lr=0.001231662, 0.001231662]
Epoch: 8, Step: 11: 36%|███▌ | 10/28 [00:00<00:01, 12.12it/s, total_batch_loss=63.72670, average_batch_loss=0.24893, average_batch_perplexity=1.28266, lr=0.001231662, 0.001231662]
Epoch: 8, Step: 11: 36%|███▌ | 10/28 [00:00<00:01, 12.12it/s, total_batch_loss=53.56535, average_batch_loss=0.20924, average_batch_perplexity=1.23274, lr=0.001228683, 0.001228683]
Epoch: 8, Step: 12: 36%|███▌ | 10/28 [00:00<00:01, 12.12it/s, total_batch_loss=53.56535, average_batch_loss=0.20924, average_batch_perplexity=1.23274, lr=0.001228683, 0.001228683]
Epoch: 8, Step: 12: 36%|███▌ | 10/28 [00:00<00:01, 12.12it/s, total_batch_loss=56.57499, average_batch_loss=0.22100, average_batch_perplexity=1.24732, lr=0.001225726, 0.001225726]
Epoch: 8, Step: 12: 43%|████▎ | 12/28 [00:00<00:01, 12.20it/s, total_batch_loss=56.57499, average_batch_loss=0.22100, average_batch_perplexity=1.24732, lr=0.001225726, 0.001225726]
Epoch: 8, Step: 13: 43%|████▎ | 12/28 [00:01<00:01, 12.20it/s, total_batch_loss=56.57499, average_batch_loss=0.22100, average_batch_perplexity=1.24732, lr=0.001225726, 0.001225726]
Epoch: 8, Step: 13: 43%|████▎ | 12/28 [00:01<00:01, 12.20it/s, total_batch_loss=54.72008, average_batch_loss=0.21375, average_batch_perplexity=1.23831, lr=0.001222790, 0.001222790]
Epoch: 8, Step: 14: 43%|████▎ | 12/28 [00:01<00:01, 12.20it/s, total_batch_loss=54.72008, average_batch_loss=0.21375, average_batch_perplexity=1.23831, lr=0.001222790, 0.001222790]
Epoch: 8, Step: 14: 43%|████▎ | 12/28 [00:01<00:01, 12.20it/s, total_batch_loss=49.66970, average_batch_loss=0.19402, average_batch_perplexity=1.21412, lr=0.001219875, 0.001219875]
Epoch: 8, Step: 14: 50%|█████ | 14/28 [00:01<00:01, 12.15it/s, total_batch_loss=49.66970, average_batch_loss=0.19402, average_batch_perplexity=1.21412, lr=0.001219875, 0.001219875]
Epoch: 8, Step: 15: 50%|█████ | 14/28 [00:01<00:01, 12.15it/s, total_batch_loss=49.66970, average_batch_loss=0.19402, average_batch_perplexity=1.21412, lr=0.001219875, 0.001219875]
Epoch: 8, Step: 15: 50%|█████ | 14/28 [00:01<00:01, 12.15it/s, total_batch_loss=50.02804, average_batch_loss=0.19542, average_batch_perplexity=1.21582, lr=0.001216981, 0.001216981]
Epoch: 8, Step: 16: 50%|█████ | 14/28 [00:01<00:01, 12.15it/s, total_batch_loss=50.02804, average_batch_loss=0.19542, average_batch_perplexity=1.21582, lr=0.001216981, 0.001216981]
Epoch: 8, Step: 16: 50%|█████ | 14/28 [00:01<00:01, 12.15it/s, total_batch_loss=50.38107, average_batch_loss=0.19680, average_batch_perplexity=1.21750, lr=0.001214107, 0.001214107]
Epoch: 8, Step: 16: 57%|█████▋ | 16/28 [00:01<00:00, 12.35it/s, total_batch_loss=50.38107, average_batch_loss=0.19680, average_batch_perplexity=1.21750, lr=0.001214107, 0.001214107]
Epoch: 8, Step: 17: 57%|█████▋ | 16/28 [00:01<00:00, 12.35it/s, total_batch_loss=50.38107, average_batch_loss=0.19680, average_batch_perplexity=1.21750, lr=0.001214107, 0.001214107]
Epoch: 8, Step: 17: 57%|█████▋ | 16/28 [00:01<00:00, 12.35it/s, total_batch_loss=52.12938, average_batch_loss=0.20363, average_batch_perplexity=1.22584, lr=0.001211254, 0.001211254]
Epoch: 8, Step: 18: 57%|█████▋ | 16/28 [00:01<00:00, 12.35it/s, total_batch_loss=52.12938, average_batch_loss=0.20363, average_batch_perplexity=1.22584, lr=0.001211254, 0.001211254]
Epoch: 8, Step: 18: 57%|█████▋ | 16/28 [00:01<00:00, 12.35it/s, total_batch_loss=51.27622, average_batch_loss=0.20030, average_batch_perplexity=1.22177, lr=0.001208421, 0.001208421]
Epoch: 8, Step: 18: 64%|██████▍ | 18/28 [00:01<00:00, 12.39it/s, total_batch_loss=51.27622, average_batch_loss=0.20030, average_batch_perplexity=1.22177, lr=0.001208421, 0.001208421]
Epoch: 8, Step: 19: 64%|██████▍ | 18/28 [00:01<00:00, 12.39it/s, total_batch_loss=51.27622, average_batch_loss=0.20030, average_batch_perplexity=1.22177, lr=0.001208421, 0.001208421]
Epoch: 8, Step: 19: 64%|██████▍ | 18/28 [00:01<00:00, 12.39it/s, total_batch_loss=53.21026, average_batch_loss=0.20785, average_batch_perplexity=1.23103, lr=0.001205607, 0.001205607]
Epoch: 8, Step: 20: 64%|██████▍ | 18/28 [00:01<00:00, 12.39it/s, total_batch_loss=53.21026, average_batch_loss=0.20785, average_batch_perplexity=1.23103, lr=0.001205607, 0.001205607]
Epoch: 8, Step: 20: 64%|██████▍ | 18/28 [00:01<00:00, 12.39it/s, total_batch_loss=50.26188, average_batch_loss=0.19634, average_batch_perplexity=1.21694, lr=0.001202813, 0.001202813]
Epoch: 8, Step: 20: 71%|███████▏ | 20/28 [00:01<00:00, 12.27it/s, total_batch_loss=50.26188, average_batch_loss=0.19634, average_batch_perplexity=1.21694, lr=0.001202813, 0.001202813]
Epoch: 8, Step: 21: 71%|███████▏ | 20/28 [00:01<00:00, 12.27it/s, total_batch_loss=50.26188, average_batch_loss=0.19634, average_batch_perplexity=1.21694, lr=0.001202813, 0.001202813]
Epoch: 8, Step: 21: 71%|███████▏ | 20/28 [00:01<00:00, 12.27it/s, total_batch_loss=50.20437, average_batch_loss=0.19611, average_batch_perplexity=1.21666, lr=0.001200038, 0.001200038]
Epoch: 8, Step: 22: 71%|███████▏ | 20/28 [00:01<00:00, 12.27it/s, total_batch_loss=50.20437, average_batch_loss=0.19611, average_batch_perplexity=1.21666, lr=0.001200038, 0.001200038]
Epoch: 8, Step: 22: 71%|███████▏ | 20/28 [00:01<00:00, 12.27it/s, total_batch_loss=51.38136, average_batch_loss=0.20071, average_batch_perplexity=1.22227, lr=0.001197283, 0.001197283]
Epoch: 8, Step: 22: 79%|███████▊ | 22/28 [00:01<00:00, 12.25it/s, total_batch_loss=51.38136, average_batch_loss=0.20071, average_batch_perplexity=1.22227, lr=0.001197283, 0.001197283]
Epoch: 8, Step: 23: 79%|███████▊ | 22/28 [00:01<00:00, 12.25it/s, total_batch_loss=51.38136, average_batch_loss=0.20071, average_batch_perplexity=1.22227, lr=0.001197283, 0.001197283]
Epoch: 8, Step: 23: 79%|███████▊ | 22/28 [00:01<00:00, 12.25it/s, total_batch_loss=45.50115, average_batch_loss=0.17774, average_batch_perplexity=1.19451, lr=0.001194546, 0.001194546]
Epoch: 8, Step: 24: 79%|███████▊ | 22/28 [00:01<00:00, 12.25it/s, total_batch_loss=45.50115, average_batch_loss=0.17774, average_batch_perplexity=1.19451, lr=0.001194546, 0.001194546]
Epoch: 8, Step: 24: 79%|███████▊ | 22/28 [00:01<00:00, 12.25it/s, total_batch_loss=51.44242, average_batch_loss=0.20095, average_batch_perplexity=1.22256, lr=0.001191828, 0.001191828]
Epoch: 8, Step: 24: 86%|████████▌ | 24/28 [00:01<00:00, 12.22it/s, total_batch_loss=51.44242, average_batch_loss=0.20095, average_batch_perplexity=1.22256, lr=0.001191828, 0.001191828]
Epoch: 8, Step: 25: 86%|████████▌ | 24/28 [00:02<00:00, 12.22it/s, total_batch_loss=51.44242, average_batch_loss=0.20095, average_batch_perplexity=1.22256, lr=0.001191828, 0.001191828]
Epoch: 8, Step: 25: 86%|████████▌ | 24/28 [00:02<00:00, 12.22it/s, total_batch_loss=47.85750, average_batch_loss=0.18694, average_batch_perplexity=1.20556, lr=0.001189129, 0.001189129]
Epoch: 8, Step: 26: 86%|████████▌ | 24/28 [00:02<00:00, 12.22it/s, total_batch_loss=47.85750, average_batch_loss=0.18694, average_batch_perplexity=1.20556, lr=0.001189129, 0.001189129]
Epoch: 8, Step: 26: 86%|████████▌ | 24/28 [00:02<00:00, 12.22it/s, total_batch_loss=40.43978, average_batch_loss=0.15797, average_batch_perplexity=1.17113, lr=0.001186447, 0.001186447]
Epoch: 8, Step: 26: 93%|█████████▎| 26/28 [00:02<00:00, 12.40it/s, total_batch_loss=40.43978, average_batch_loss=0.15797, average_batch_perplexity=1.17113, lr=0.001186447, 0.001186447]
Epoch: 8, Step: 27: 93%|█████████▎| 26/28 [00:02<00:00, 12.40it/s, total_batch_loss=40.43978, average_batch_loss=0.15797, average_batch_perplexity=1.17113, lr=0.001186447, 0.001186447]
Epoch: 8, Step: 27: 93%|█████████▎| 26/28 [00:02<00:00, 12.40it/s, total_batch_loss=50.69247, average_batch_loss=0.19802, average_batch_perplexity=1.21898, lr=0.001183784, 0.001183784]
Epoch: 8, Step: 28: 93%|█████████▎| 26/28 [00:02<00:00, 12.40it/s, total_batch_loss=50.69247, average_batch_loss=0.19802, average_batch_perplexity=1.21898, lr=0.001183784, 0.001183784]
Epoch: 8, Step: 28: 93%|█████████▎| 26/28 [00:02<00:00, 12.40it/s, total_batch_loss=18.43602, average_batch_loss=0.20950, average_batch_perplexity=1.23306, lr=0.001181139, 0.001181139]
Epoch: 8, Step: 28: 100%|██████████| 28/28 [00:02<00:00, 13.72it/s, total_batch_loss=18.43602, average_batch_loss=0.20950, average_batch_perplexity=1.23306, lr=0.001181139, 0.001181139]
2024-12-17 09:44:20,548 - root - INFO - Total Samples: 7000
2024-12-17 09:44:20,548 - root - INFO - Total Batches: 28
2024-12-17 09:44:20,549 - root - INFO - Average Epoch Train Loss: 0.21644
2024-12-17 09:44:20,550 - root - INFO - Average Epoch Train Perplexity: 1.24164
2024-12-17 09:44:20,551 - root - INFO -
2024-12-17 09:44:20,552 - root - INFO - ====================================================== Starting Valid Epoch: 8/9 ======================================================
0%| | 0/8 [00:00<?, ?it/s]
Epoch: 8, Step: 1: 0%| | 0/8 [00:00<?, ?it/s]
Epoch: 8, Step: 1: 0%| | 0/8 [00:00<?, ?it/s, total_batch_loss=29.76483, average_batch_loss=0.11627, average_batch_perplexity=1.12330]
Epoch: 8, Step: 2: 0%| | 0/8 [00:00<?, ?it/s, total_batch_loss=29.76483, average_batch_loss=0.11627, average_batch_perplexity=1.12330]
Epoch: 8, Step: 2: 0%| | 0/8 [00:00<?, ?it/s, total_batch_loss=29.46812, average_batch_loss=0.11511, average_batch_perplexity=1.12200]
Epoch: 8, Step: 3: 0%| | 0/8 [00:00<?, ?it/s, total_batch_loss=29.46812, average_batch_loss=0.11511, average_batch_perplexity=1.12200]
Epoch: 8, Step: 3: 0%| | 0/8 [00:00<?, ?it/s, total_batch_loss=29.96622, average_batch_loss=0.11706, average_batch_perplexity=1.12418]
Epoch: 8, Step: 3: 38%|███▊ | 3/8 [00:00<00:00, 23.38it/s, total_batch_loss=29.96622, average_batch_loss=0.11706, average_batch_perplexity=1.12418]
Epoch: 8, Step: 4: 38%|███▊ | 3/8 [00:00<00:00, 23.38it/s, total_batch_loss=29.96622, average_batch_loss=0.11706, average_batch_perplexity=1.12418]
Epoch: 8, Step: 4: 38%|███▊ | 3/8 [00:00<00:00, 23.38it/s, total_batch_loss=33.13523, average_batch_loss=0.12943, average_batch_perplexity=1.13818]
Epoch: 8, Step: 5: 38%|███▊ | 3/8 [00:00<00:00, 23.38it/s, total_batch_loss=33.13523, average_batch_loss=0.12943, average_batch_perplexity=1.13818]
Epoch: 8, Step: 5: 38%|███▊ | 3/8 [00:00<00:00, 23.38it/s, total_batch_loss=29.03102, average_batch_loss=0.11340, average_batch_perplexity=1.12008]
Epoch: 8, Step: 6: 38%|███▊ | 3/8 [00:00<00:00, 23.38it/s, total_batch_loss=29.03102, average_batch_loss=0.11340, average_batch_perplexity=1.12008]
Epoch: 8, Step: 6: 38%|███▊ | 3/8 [00:00<00:00, 23.38it/s, total_batch_loss=30.31429, average_batch_loss=0.11842, average_batch_perplexity=1.12571]
Epoch: 8, Step: 6: 75%|███████▌ | 6/8 [00:00<00:00, 23.96it/s, total_batch_loss=30.31429, average_batch_loss=0.11842, average_batch_perplexity=1.12571]
Epoch: 8, Step: 7: 75%|███████▌ | 6/8 [00:00<00:00, 23.96it/s, total_batch_loss=30.31429, average_batch_loss=0.11842, average_batch_perplexity=1.12571]
Epoch: 8, Step: 7: 75%|███████▌ | 6/8 [00:00<00:00, 23.96it/s, total_batch_loss=31.22097, average_batch_loss=0.12196, average_batch_perplexity=1.12971]
Epoch: 8, Step: 8: 75%|███████▌ | 6/8 [00:00<00:00, 23.96it/s, total_batch_loss=31.22097, average_batch_loss=0.12196, average_batch_perplexity=1.12971]
Epoch: 8, Step: 8: 75%|███████▌ | 6/8 [00:00<00:00, 23.96it/s, total_batch_loss=21.50961, average_batch_loss=0.10341, average_batch_perplexity=1.10895]
2024-12-17 09:44:20,882 - root - INFO - Total Samples: 2000
2024-12-17 09:44:20,883 - root - INFO - Total Batches: 8
2024-12-17 09:44:20,884 - root - INFO - Average Epoch Valid Loss: 0.11721
2024-12-17 09:44:20,884 - root - INFO - Average Epoch Valid Perplexity: 1.12435
2024-12-17 09:44:20,885 - root - INFO -
Evaluating and Generation.: 0%| | 0/4 [00:00<?, ?it/s]
Evaluating and Generation.: 0%| | 0/4 [00:00<?, ?it/s, accuracy: 0.8789]
Evaluating and Generation.: 25%|██▌ | 1/4 [00:00<00:00, 9.75it/s, accuracy: 0.8789]
Evaluating and Generation.: 25%|██▌ | 1/4 [00:00<00:00, 9.75it/s, accuracy: 0.8594]
Evaluating and Generation.: 25%|██▌ | 1/4 [00:00<00:00, 9.75it/s, accuracy: 0.9258]
Evaluating and Generation.: 75%|███████▌ | 3/4 [00:00<00:00, 10.40it/s, accuracy: 0.9258]
Evaluating and Generation.: 75%|███████▌ | 3/4 [00:00<00:00, 10.40it/s, accuracy: 0.8793]
2024-12-17 09:44:21,266 - root - INFO - Correct/Total Samples: 886/1000
2024-12-17 09:44:21,266 - root - INFO - Eval Accuracy: 0.886
2024-12-17 09:44:21,284 - root - INFO -
epoch batch_index sample_index equation generated correct
8 1 0 13+48=061 13+48=061 True
8 1 1 16+55=071 16+55=071 True
8 1 2 79+34=113 79+34=103 False
8 1 3 35+44=079 35+44=079 True
8 1 4 16+50=066 16+50=066 True
8 1 5 28+47=075 28+47=075 True
8 1 6 00+74=074 00+74=064 False
8 1 7 15+20=035 15+20=035 True
8 1 8 72+60=132 72+60=122 False
8 1 9 63+68=131 63+68=131 True
8 1 10 29+45=074 29+45=074 True
8 1 11 34+60=094 34+60=094 True
8 1 12 53+70=123 53+70=123 True
8 1 13 70+50=120 70+50=110 False
8 1 14 11+84=095 11+84=095 True
8 1 15 42+71=113 42+71=113 True
8 1 16 98+22=120 98+22=120 True
8 1 17 02+02=004 02+02=014 False
8 1 18 15+85=100 15+85=100 True
8 1 19 21+78=099 21+78=099 True
8 1 20 61+79=140 61+79=140 True
8 1 21 25+99=124 25+99=124 True
8 1 22 09+85=094 09+85=094 True
8 1 23 60+91=151 60+91=151 True
8 1 24 35+30=065 35+30=065 True
8 1 25 24+51=075 24+51=075 True
8 1 26 93+91=184 93+91=184 True
8 1 27 39+96=135 39+96=135 True
8 1 28 64+35=099 64+35=099 True
8 1 29 36+22=058 36+22=058 True
8 1 30 68+45=113 68+45=113 True
8 1 31 16+84=100 16+84=100 True
8 1 32 91+52=143 91+52=143 True
8 1 33 97+36=133 97+36=133 True
8 1 34 27+37=064 27+37=063 False
8 1 35 99+82=181 99+82=181 True
8 1 36 03+42=045 03+42=045 True
8 1 37 18+38=056 18+38=056 True
8 1 38 32+20=052 32+20=052 True
8 1 39 38+13=051 38+13=051 True
8 1 40 68+42=110 68+42=100 False
8 1 41 64+00=064 64+00=054 False
8 1 42 48+94=142 48+94=142 True
8 1 43 58+36=094 58+36=094 True
8 1 44 41+22=063 41+22=053 False
8 1 45 23+58=081 23+58=081 True
8 1 46 67+46=113 67+46=113 True
8 1 47 40+78=118 40+78=118 True
8 1 48 90+38=128 90+38=128 True
8 1 49 89+52=141 89+52=141 True
8 1 50 37+77=114 37+77=113 False
8 1 51 29+76=105 29+76=105 True
8 1 52 42+90=132 42+90=132 True
8 1 53 45+82=127 45+82=127 True
8 1 54 35+95=130 35+95=130 True
8 1 55 92+98=190 92+98=190 True
8 1 56 73+91=164 73+91=164 True
8 1 57 53+97=150 53+97=150 True
8 1 58 98+69=167 98+69=167 True
8 1 59 20+46=066 20+46=056 False
8 1 60 48+69=117 48+69=117 True
8 1 61 62+31=093 62+31=093 True
8 1 62 80+59=139 80+59=139 True
8 1 63 58+12=070 58+12=070 True
8 1 64 08+96=104 08+96=104 True
8 1 65 67+06=073 67+06=063 False
8 1 66 22+04=026 22+04=026 True
8 1 67 61+87=148 61+87=148 True
8 1 68 95+27=122 95+27=122 True
8 1 69 49+83=132 49+83=132 True
8 1 70 43+00=043 43+00=043 True
8 1 71 01+85=086 01+85=086 True
8 1 72 11+68=079 11+68=079 True
8 1 73 80+03=083 80+03=083 True
8 1 74 54+83=137 54+83=137 True
8 1 75 73+47=120 73+47=110 False
8 1 76 99+93=192 99+93=192 True
8 1 77 99+13=112 99+13=112 True
8 1 78 92+66=158 92+66=158 True
8 1 79 90+31=121 90+31=121 True
8 1 80 25+69=094 25+69=094 True
8 1 81 25+44=069 25+44=069 True
8 1 82 00+93=093 00+93=093 True
8 1 83 88+87=175 88+87=175 True
8 1 84 47+56=103 47+56=103 True
8 1 85 43+59=102 43+59=102 True
8 1 86 22+00=022 22+00=022 True
8 1 87 34+04=038 34+04=038 True
8 1 88 65+13=078 65+13=078 True
8 1 89 39+82=121 39+82=121 True
8 1 90 66+83=149 66+83=149 True
8 1 91 51+69=120 51+69=110 False
8 1 92 80+21=101 80+21=101 True
8 1 93 36+79=115 36+79=115 True
8 1 94 21+68=089 21+68=089 True
8 1 95 11+66=077 11+66=077 True
8 1 96 55+19=074 55+19=074 True
8 1 97 51+61=112 51+61=102 False
8 1 98 38+88=126 38+88=126 True
8 1 99 37+27=064 37+27=063 False
8 1 100 18+63=081 18+63=081 True
8 1 101 48+11=059 48+11=059 True
8 1 102 72+68=140 72+68=140 True
8 1 103 37+39=076 37+39=076 True
8 1 104 64+95=159 64+95=159 True
8 1 105 49+75=124 49+75=124 True
8 1 106 45+66=111 45+66=111 True
8 1 107 34+87=121 34+87=121 True
8 1 108 02+84=086 02+84=086 True
8 1 109 95+00=095 95+00=095 True
8 1 110 09+56=065 09+56=065 True
8 1 111 22+66=088 22+66=088 True
8 1 112 43+18=061 43+18=061 True
8 1 113 61+35=096 61+35=096 True
8 1 114 13+73=086 13+73=086 True
8 1 115 25+95=120 25+95=110 False
8 1 116 73+96=169 73+96=169 True
8 1 117 03+96=099 03+96=099 True
8 1 118 97+82=179 97+82=179 True
8 1 119 18+42=060 18+42=060 True
8 1 120 29+98=127 29+98=127 True
8 1 121 61+00=061 61+00=051 False
8 1 122 22+98=120 22+98=120 True
8 1 123 12+50=062 12+50=062 True
8 1 124 02+58=060 02+58=060 True
8 1 125 75+86=161 75+86=161 True
8 1 126 31+57=088 31+57=088 True
8 1 127 49+82=131 49+82=131 True
8 1 128 15+33=048 15+33=048 True
8 1 129 49+57=106 49+57=106 True
8 1 130 61+70=131 61+70=121 False
8 1 131 91+51=142 91+51=142 True
8 1 132 50+05=055 50+05=055 True
8 1 133 44+16=060 44+16=060 True
8 1 134 92+01=093 92+01=093 True
8 1 135 85+82=167 85+82=167 True
8 1 136 07+41=048 07+41=048 True
8 1 137 40+06=046 40+06=046 True
8 1 138 79+62=141 79+62=141 True
8 1 139 95+62=157 95+62=157 True
8 1 140 42+93=135 42+93=135 True
8 1 141 32+73=105 32+73=105 True
8 1 142 47+09=056 47+09=056 True
8 1 143 59+50=109 59+50=109 True
8 1 144 61+77=138 61+77=138 True
8 1 145 64+06=070 64+06=060 False
8 1 146 35+10=045 35+10=045 True
8 1 147 32+88=120 32+88=120 True
8 1 148 03+95=098 03+95=098 True
8 1 149 11+38=049 11+38=049 True
8 1 150 21+67=088 21+67=088 True
8 1 151 33+25=058 33+25=058 True
8 1 152 63+45=108 63+45=108 True
8 1 153 56+12=068 56+12=068 True
8 1 154 19+79=098 19+79=098 True
8 1 155 60+43=103 60+43=103 True
8 1 156 07+61=068 07+61=068 True
8 1 157 58+03=061 58+03=061 True
8 1 158 11+10=021 11+10=021 True
8 1 159 49+89=138 49+89=138 True
8 1 160 37+58=095 37+58=095 True
8 1 161 59+78=137 59+78=137 True
8 1 162 11+21=032 11+21=032 True
8 1 163 37+43=080 37+43=070 False
8 1 164 44+21=065 44+21=065 True
8 1 165 22+97=119 22+97=119 True
8 1 166 65+35=100 65+35=090 False
8 1 167 06+51=057 06+51=057 True
8 1 168 65+25=090 65+25=080 False
8 1 169 74+94=168 74+94=168 True
8 1 170 87+55=142 87+55=142 True
8 1 171 90+67=157 90+67=157 True
8 1 172 11+02=013 11+02=013 True
8 1 173 01+66=067 01+66=057 False
8 1 174 56+00=056 56+00=056 True
8 1 175 58+52=110 58+52=110 True
8 1 176 24+99=123 24+99=123 True
8 1 177 97+13=110 97+13=100 False
8 1 178 42+94=136 42+94=136 True
8 1 179 60+15=075 60+15=075 True
8 1 180 20+46=066 20+46=056 False
8 1 181 40+70=110 40+70=110 True
8 1 182 95+45=140 95+45=140 True
8 1 183 96+95=191 96+95=191 True
8 1 184 98+20=118 98+20=118 True
8 1 185 43+19=062 43+19=062 True
8 1 186 50+69=119 50+69=119 True
8 1 187 27+53=080 27+53=070 False
8 1 188 24+25=049 24+25=049 True
8 1 189 65+92=157 65+92=157 True
8 1 190 28+14=042 28+14=042 True
8 1 191 20+57=077 20+57=077 True
8 1 192 59+97=156 59+97=156 True
8 1 193 98+32=130 98+32=130 True
8 1 194 55+84=139 55+84=139 True
8 1 195 20+39=059 20+39=059 True
8 1 196 86+47=133 86+47=133 True
8 1 197 92+36=128 92+36=128 True
8 1 198 05+38=043 05+38=043 True
8 1 199 77+36=113 77+36=103 False
8 1 200 41+64=105 41+64=105 True
8 1 201 74+51=125 74+51=115 False
8 1 202 74+55=129 74+55=129 True
8 1 203 64+64=128 64+64=128 True
8 1 204 60+19=079 60+19=079 True
8 1 205 77+96=173 77+96=173 True
8 1 206 22+30=052 22+30=052 True
8 1 207 82+49=131 82+49=131 True
8 1 208 39+67=106 39+67=106 True
8 1 209 62+40=102 62+40=102 True
8 1 210 28+71=099 28+71=099 True
8 1 211 47+26=073 47+26=073 True
8 1 212 98+54=152 98+54=152 True
8 1 213 38+70=108 38+70=108 True
8 1 214 63+40=103 63+40=103 True
8 1 215 86+62=148 86+62=148 True
8 1 216 22+65=087 22+65=087 True
8 1 217 41+17=058 41+17=058 True
8 1 218 68+88=156 68+88=156 True
8 1 219 96+70=166 96+70=166 True
8 1 220 99+29=128 99+29=128 True
8 1 221 83+39=122 83+39=122 True
8 1 222 26+55=081 26+55=081 True
8 1 223 53+70=123 53+70=123 True
8 1 224 94+12=106 94+12=106 True
8 1 225 00+37=037 00+37=037 True
8 1 226 36+94=130 36+94=130 True
8 1 227 40+58=098 40+58=098 True
8 1 228 19+80=099 19+80=099 True
8 1 229 49+44=093 49+44=093 True
8 1 230 70+27=097 70+27=097 True
8 1 231 52+80=132 52+80=132 True
8 1 232 77+90=167 77+90=167 True
8 1 233 13+92=105 13+92=105 True
8 1 234 59+09=068 59+09=068 True
8 1 235 33+55=088 33+55=088 True
8 1 236 85+16=101 85+16=101 True
8 1 237 25+65=090 25+65=080 False
8 1 238 46+20=066 46+20=056 False
8 1 239 29+52=081 29+52=081 True
8 1 240 32+36=068 32+36=068 True
8 1 241 47+08=055 47+08=055 True
8 1 242 21+84=105 21+84=105 True
8 1 243 24+45=069 24+45=069 True
8 1 244 29+15=044 29+15=044 True
8 1 245 83+03=086 83+03=086 True
8 1 246 83+36=119 83+36=119 True
8 1 247 58+95=153 58+95=153 True
8 1 248 76+79=155 76+79=155 True
8 1 249 63+30=093 63+30=093 True
8 1 250 38+24=062 38+24=062 True
8 1 251 19+46=065 19+46=065 True
8 1 252 99+66=165 99+66=165 True
8 1 253 95+73=168 95+73=168 True
8 1 254 65+27=092 65+27=092 True
8 1 255 91+83=174 91+83=174 True
8 2 0 65+49=114 65+49=104 False
8 2 1 03+08=011 03+08=021 False
8 2 2 67+81=148 67+81=148 True
8 2 3 47+23=070 47+23=060 False
8 2 4 43+91=134 43+91=134 True
8 2 5 41+67=108 41+67=108 True
8 2 6 02+33=035 02+33=035 True
8 2 7 64+84=148 64+84=148 True
8 2 8 81+64=145 81+64=145 True
8 2 9 80+11=091 80+11=091 True
8 2 10 78+01=079 78+01=079 True
8 2 11 89+18=107 89+18=107 True
8 2 12 45+52=097 45+52=097 True
8 2 13 35+30=065 35+30=065 True
8 2 14 53+32=085 53+32=085 True
8 2 15 49+90=139 49+90=149 False
8 2 16 41+37=078 41+37=078 True
8 2 17 35+14=049 35+14=049 True
8 2 18 92+50=142 92+50=142 True
8 2 19 37+60=097 37+60=097 True
8 2 20 91+61=152 91+61=152 True
8 2 21 80+77=157 80+77=157 True
8 2 22 66+24=090 66+24=080 False
8 2 23 81+07=088 81+07=088 True
8 2 24 85+59=144 85+59=144 True
8 2 25 19+69=088 19+69=088 True
8 2 26 91+44=135 91+44=135 True
8 2 27 25+29=054 25+29=054 True
8 2 28 27+08=035 27+08=035 True
8 2 29 66+14=080 66+14=080 True
8 2 30 95+11=106 95+11=106 True
8 2 31 13+97=110 13+97=100 False
8 2 32 94+40=134 94+40=134 True
8 2 33 74+31=105 74+31=105 True
8 2 34 49+00=049 49+00=049 True
8 2 35 59+18=077 59+18=077 True
8 2 36 07+65=072 07+65=062 False
8 2 37 83+55=138 83+55=138 True
8 2 38 49+80=129 49+80=139 False
8 2 39 64+17=081 64+17=081 True
8 2 40 48+83=131 48+83=131 True
8 2 41 95+44=139 95+44=149 False
8 2 42 71+26=097 71+26=097 True
8 2 43 06+74=080 06+74=070 False
8 2 44 34+24=058 34+24=058 True
8 2 45 59+71=130 59+71=120 False
8 2 46 68+32=100 68+32=090 False
8 2 47 38+81=119 38+81=119 True
8 2 48 29+56=085 29+56=085 True
8 2 49 54+55=109 54+55=109 True
8 2 50 31+27=058 31+27=058 True
8 2 51 97+89=186 97+89=186 True
8 2 52 48+09=057 48+09=057 True
8 2 53 86+76=162 86+76=162 True
8 2 54 82+59=141 82+59=141 True
8 2 55 01+67=068 01+67=068 True
8 2 56 26+06=032 26+06=032 True
8 2 57 22+46=068 22+46=068 True
8 2 58 85+16=101 85+16=101 True
8 2 59 29+08=037 29+08=037 True
8 2 60 73+94=167 73+94=167 True
8 2 61 19+62=081 19+62=081 True
8 2 62 86+62=148 86+62=148 True
8 2 63 38+99=137 38+99=137 True
8 2 64 64+25=089 64+25=089 True
8 2 65 61+72=133 61+72=123 False
8 2 66 78+88=166 78+88=166 True
8 2 67 43+66=109 43+66=109 True
8 2 68 69+35=104 69+35=104 True
8 2 69 33+77=110 33+77=100 False
8 2 70 37+37=074 37+37=073 False
8 2 71 87+54=141 87+54=141 True
8 2 72 68+90=158 68+90=158 True
8 2 73 83+44=127 83+44=127 True
8 2 74 41+09=050 41+09=050 True
8 2 75 13+48=061 13+48=061 True
8 2 76 01+41=042 01+41=042 True
8 2 77 19+74=093 19+74=093 True
8 2 78 15+05=020 15+05=020 True
8 2 79 55+46=101 55+46=101 True
8 2 80 68+33=101 68+33=101 True
8 2 81 44+40=084 44+40=084 True
8 2 82 88+03=091 88+03=091 True
8 2 83 81+79=160 81+79=160 True
8 2 84 18+98=116 18+98=116 True
8 2 85 70+64=134 70+64=134 True
8 2 86 26+44=070 26+44=060 False
8 2 87 98+87=185 98+87=185 True
8 2 88 18+74=092 18+74=092 True
8 2 89 50+68=118 50+68=118 True
8 2 90 13+51=064 13+51=064 True
8 2 91 90+89=179 90+89=189 False
8 2 92 47+78=125 47+78=125 True
8 2 93 81+57=138 81+57=138 True
8 2 94 34+47=081 34+47=081 True
8 2 95 94+23=117 94+23=117 True
8 2 96 07+70=077 07+70=077 True
8 2 97 56+33=089 56+33=089 True
8 2 98 33+04=037 33+04=037 True
8 2 99 26+09=035 26+09=035 True
8 2 100 14+92=106 14+92=106 True
8 2 101 78+54=132 78+54=132 True
8 2 102 36+76=112 36+76=112 True
8 2 103 17+47=064 17+47=063 False
8 2 104 28+18=046 28+18=046 True
8 2 105 78+54=132 78+54=132 True
8 2 106 84+72=156 84+72=156 True
8 2 107 00+44=044 00+44=044 True
8 2 108 50+41=091 50+41=081 False
8 2 109 87+88=175 87+88=175 True
8 2 110 11+66=077 11+66=077 True
8 2 111 80+60=140 80+60=140 True
8 2 112 78+76=154 78+76=154 True
8 2 113 24+74=098 24+74=098 True
8 2 114 88+48=136 88+48=136 True
8 2 115 38+31=069 38+31=069 True
8 2 116 29+27=056 29+27=056 True
8 2 117 08+45=053 08+45=053 True
8 2 118 28+13=041 28+13=041 True
8 2 119 53+99=152 53+99=152 True
8 2 120 47+92=139 47+92=139 True
8 2 121 76+21=097 76+21=097 True
8 2 122 53+96=149 53+96=149 True
8 2 123 93+91=184 93+91=184 True
8 2 124 97+33=130 97+33=120 False
8 2 125 67+78=145 67+78=145 True
8 2 126 58+05=063 58+05=063 True
8 2 127 00+16=016 00+16=016 True
8 2 128 80+19=099 80+19=099 True
8 2 129 98+22=120 98+22=120 True
8 2 130 09+62=071 09+62=071 True
8 2 131 06+23=029 06+23=029 True
8 2 132 32+99=131 32+99=131 True
8 2 133 17+02=019 17+02=019 True
8 2 134 64+35=099 64+35=099 True
8 2 135 35+83=118 35+83=118 True
8 2 136 71+36=107 71+36=107 True
8 2 137 75+06=081 75+06=071 False
8 2 138 88+95=183 88+95=183 True
8 2 139 19+98=117 19+98=107 False
8 2 140 28+89=117 28+89=117 True
8 2 141 33+11=044 33+11=044 True
8 2 142 34+49=083 34+49=083 True
8 2 143 90+35=125 90+35=125 True
8 2 144 22+90=112 22+90=112 True
8 2 145 98+89=187 98+89=187 True
8 2 146 88+47=135 88+47=135 True
8 2 147 30+86=116 30+86=116 True
8 2 148 31+48=079 31+48=079 True
8 2 149 39+21=060 39+21=060 True
8 2 150 19+17=036 19+17=036 True
8 2 151 27+60=087 27+60=087 True
8 2 152 12+16=028 12+16=028 True
8 2 153 51+75=126 51+75=116 False
8 2 154 10+74=084 10+74=084 True
8 2 155 42+63=105 42+63=105 True
8 2 156 40+14=054 40+14=054 True
8 2 157 23+93=116 23+93=116 True
8 2 158 85+26=111 85+26=111 True
8 2 159 28+46=074 28+46=074 True
8 2 160 28+33=061 28+33=061 True
8 2 161 43+30=073 43+30=073 True
8 2 162 89+72=161 89+72=161 True
8 2 163 52+21=073 52+21=063 False
8 2 164 21+54=075 21+54=075 True
8 2 165 69+13=082 69+13=082 True
8 2 166 07+60=067 07+60=067 True
8 2 167 63+83=146 63+83=146 True
8 2 168 80+69=149 80+69=159 False
8 2 169 27+28=055 27+28=055 True
8 2 170 42+31=073 42+31=063 False
8 2 171 51+99=150 51+99=150 True
8 2 172 28+75=103 28+75=103 True
8 2 173 38+57=095 38+57=095 True
8 2 174 83+16=099 83+16=099 True
8 2 175 92+94=186 92+94=186 True
8 2 176 55+75=130 55+75=120 False
8 2 177 59+51=110 59+51=100 False
8 2 178 33+09=042 33+09=042 True
8 2 179 53+13=066 53+13=066 True
8 2 180 05+70=075 05+70=075 True
8 2 181 12+20=032 12+20=033 False
8 2 182 11+49=060 11+49=060 True
8 2 183 63+45=108 63+45=108 True
8 2 184 92+23=115 92+23=115 True
8 2 185 82+45=127 82+45=127 True
8 2 186 23+41=064 23+41=054 False
8 2 187 64+26=090 64+26=080 False
8 2 188 91+24=115 91+24=115 True
8 2 189 20+32=052 20+32=052 True
8 2 190 83+21=104 83+21=104 True
8 2 191 07+20=027 07+20=027 True
8 2 192 94+14=108 94+14=108 True
8 2 193 96+89=185 96+89=185 True
8 2 194 13+08=021 13+08=021 True
8 2 195 32+05=037 32+05=037 True
8 2 196 09+51=060 09+51=060 True
8 2 197 26+29=055 26+29=055 True
8 2 198 49+65=114 49+65=114 True
8 2 199 32+66=098 32+66=098 True
8 2 200 41+08=049 41+08=049 True
8 2 201 26+79=105 26+79=105 True
8 2 202 29+91=120 29+91=120 True
8 2 203 51+00=051 51+00=051 True
8 2 204 61+60=121 61+60=111 False
8 2 205 45+78=123 45+78=123 True
8 2 206 56+16=072 56+16=072 True
8 2 207 66+68=134 66+68=134 True
8 2 208 32+16=048 32+16=048 True
8 2 209 84+49=133 84+49=133 True
8 2 210 45+09=054 45+09=054 True
8 2 211 96+78=174 96+78=174 True
8 2 212 10+02=012 10+02=012 True
8 2 213 36+60=096 36+60=096 True
8 2 214 44+36=080 44+36=080 True
8 2 215 12+86=098 12+86=098 True
8 2 216 94+54=148 94+54=148 True
8 2 217 64+73=137 64+73=137 True
8 2 218 73+10=083 73+10=083 True
8 2 219 14+62=076 14+62=076 True
8 2 220 25+22=047 25+22=047 True
8 2 221 94+22=116 94+22=116 True
8 2 222 41+76=117 41+76=117 True
8 2 223 38+46=084 38+46=084 True
8 2 224 71+72=143 71+72=143 True
8 2 225 74+79=153 74+79=153 True
8 2 226 99+67=166 99+67=166 True
8 2 227 78+71=149 78+71=149 True
8 2 228 23+19=042 23+19=042 True
8 2 229 51+65=116 51+65=116 True
8 2 230 94+86=180 94+86=180 True
8 2 231 09+79=088 09+79=088 True
8 2 232 69+39=108 69+39=108 True
8 2 233 84+13=097 84+13=097 True
8 2 234 36+59=095 36+59=095 True
8 2 235 87+47=134 87+47=133 False
8 2 236 50+00=050 50+00=050 True
8 2 237 76+96=172 76+96=172 True
8 2 238 12+18=030 12+18=030 True
8 2 239 99+95=194 99+95=184 False
8 2 240 22+00=022 22+00=022 True
8 2 241 96+18=114 96+18=114 True
8 2 242 51+20=071 51+20=071 True
8 2 243 66+81=147 66+81=147 True
8 2 244 78+18=096 78+18=096 True
8 2 245 09+78=087 09+78=087 True
8 2 246 24+20=044 24+20=044 True
8 2 247 76+13=089 76+13=089 True
8 2 248 05+10=015 05+10=015 True
8 2 249 97+14=111 97+14=111 True
8 2 250 92+38=130 92+38=130 True
8 2 251 77+13=090 77+13=080 False
8 2 252 70+19=089 70+19=089 True
8 2 253 90+45=135 90+45=135 True
8 2 254 50+09=059 50+09=059 True
8 2 255 78+06=084 78+06=074 False
8 3 0 03+25=028 03+25=028 True
8 3 1 48+43=091 48+43=091 True
8 3 2 39+47=086 39+47=086 True
8 3 3 48+19=067 48+19=067 True
8 3 4 07+22=029 07+22=029 True
8 3 5 73+68=141 73+68=141 True
8 3 6 14+56=070 14+56=070 True
8 3 7 96+95=191 96+95=191 True
8 3 8 96+28=124 96+28=124 True
8 3 9 82+05=087 82+05=087 True
8 3 10 27+94=121 27+94=121 True
8 3 11 87+86=173 87+86=173 True
8 3 12 00+68=068 00+68=068 True
8 3 13 11+37=048 11+37=048 True
8 3 14 95+93=188 95+93=188 True
8 3 15 75+82=157 75+82=157 True
8 3 16 41+71=112 41+71=112 True
8 3 17 60+14=074 60+14=074 True
8 3 18 77+77=154 77+77=143 False
8 3 19 31+84=115 31+84=115 True
8 3 20 31+57=088 31+57=088 True
8 3 21 27+87=114 27+87=113 False
8 3 22 31+89=120 31+89=120 True
8 3 23 22+18=040 22+18=040 True
8 3 24 38+25=063 38+25=063 True
8 3 25 64+54=118 64+54=118 True
8 3 26 85+60=145 85+60=145 True
8 3 27 14+71=085 14+71=085 True
8 3 28 06+16=022 06+16=022 True
8 3 29 78+61=139 78+61=139 True
8 3 30 65+75=140 65+75=130 False
8 3 31 13+83=096 13+83=096 True
8 3 32 75+49=124 75+49=114 False
8 3 33 05+78=083 05+78=083 True
8 3 34 66+55=121 66+55=121 True
8 3 35 03+05=008 03+05=008 True
8 3 36 69+99=168 69+99=168 True
8 3 37 52+82=134 52+82=134 True
8 3 38 45+97=142 45+97=142 True
8 3 39 66+17=083 66+17=083 True
8 3 40 36+17=053 36+17=053 True
8 3 41 92+74=166 92+74=166 True
8 3 42 48+44=092 48+44=092 True
8 3 43 34+17=051 34+17=051 True
8 3 44 56+11=067 56+11=067 True
8 3 45 77+23=100 77+23=090 False
8 3 46 10+11=021 10+11=021 True
8 3 47 32+65=097 32+65=097 True
8 3 48 53+49=102 53+49=102 True
8 3 49 68+86=154 68+86=154 True
8 3 50 52+94=146 52+94=146 True
8 3 51 97+71=168 97+71=168 True
8 3 52 05+37=042 05+37=042 True
8 3 53 58+75=133 58+75=133 True
8 3 54 06+24=030 06+24=030 True
8 3 55 15+44=059 15+44=059 True
8 3 56 90+49=139 90+49=139 True
8 3 57 50+37=087 50+37=087 True
8 3 58 88+61=149 88+61=159 False
8 3 59 21+57=078 21+57=078 True
8 3 60 24+85=109 24+85=109 True
8 3 61 01+66=067 01+66=057 False
8 3 62 50+46=096 50+46=096 True
8 3 63 76+65=141 76+65=141 True
8 3 64 23+74=097 23+74=097 True
8 3 65 76+16=092 76+16=092 True
8 3 66 06+08=014 06+08=014 True
8 3 67 69+25=094 69+25=094 True
8 3 68 15+23=038 15+23=038 True
8 3 69 41+02=043 41+02=043 True
8 3 70 16+66=082 16+66=082 True
8 3 71 59+94=153 59+94=153 True
8 3 72 32+88=120 32+88=120 True
8 3 73 46+21=067 46+21=067 True
8 3 74 57+28=085 57+28=085 True
8 3 75 00+31=031 00+31=031 True
8 3 76 77+07=084 77+07=073 False
8 3 77 28+70=098 28+70=098 True
8 3 78 05+61=066 05+61=056 False
8 3 79 22+09=031 22+09=031 True
8 3 80 08+94=102 08+94=102 True
8 3 81 40+11=051 40+11=051 True
8 3 82 10+48=058 10+48=058 True
8 3 83 27+56=083 27+56=083 True
8 3 84 42+16=058 42+16=058 True
8 3 85 69+43=112 69+43=112 True
8 3 86 57+69=126 57+69=126 True
8 3 87 18+86=104 18+86=104 True
8 3 88 86+80=166 86+80=166 True
8 3 89 30+85=115 30+85=115 True
8 3 90 77+66=143 77+66=143 True
8 3 91 39+64=103 39+64=103 True
8 3 92 76+61=137 76+61=137 True
8 3 93 42+61=103 42+61=103 True
8 3 94 07+30=037 07+30=037 True
8 3 95 35+93=128 35+93=128 True
8 3 96 40+90=130 40+90=130 True
8 3 97 08+91=099 08+91=099 True
8 3 98 62+34=096 62+34=096 True
8 3 99 86+49=135 86+49=135 True
8 3 100 73+23=096 73+23=096 True
8 3 101 87+35=122 87+35=122 True
8 3 102 35+31=066 35+31=066 True
8 3 103 07+13=020 07+13=020 True
8 3 104 39+41=080 39+41=080 True
8 3 105 44+63=107 44+63=107 True
8 3 106 94+66=160 94+66=160 True
8 3 107 49+54=103 49+54=103 True
8 3 108 79+46=125 79+46=125 True
8 3 109 53+12=065 53+12=065 True
8 3 110 60+92=152 60+92=152 True
8 3 111 25+60=085 25+60=085 True
8 3 112 64+53=117 64+53=117 True
8 3 113 41+02=043 41+02=043 True
8 3 114 00+97=097 00+97=097 True
8 3 115 12+52=064 12+52=064 True
8 3 116 39+50=089 39+50=089 True
8 3 117 87+21=108 87+21=108 True
8 3 118 04+99=103 04+99=103 True
8 3 119 19+75=094 19+75=094 True
8 3 120 90+05=095 90+05=095 True
8 3 121 54+39=093 54+39=093 True
8 3 122 29+26=055 29+26=055 True
8 3 123 82+95=177 82+95=177 True
8 3 124 55+09=064 55+09=064 True
8 3 125 02+62=064 02+62=054 False
8 3 126 68+30=098 68+30=098 True
8 3 127 99+16=115 99+16=105 False
8 3 128 63+11=074 63+11=074 True
8 3 129 42+92=134 42+92=134 True
8 3 130 99+16=115 99+16=105 False
8 3 131 50+31=081 50+31=081 True
8 3 132 23+46=069 23+46=069 True
8 3 133 45+73=118 45+73=118 True
8 3 134 89+77=166 89+77=166 True
8 3 135 45+78=123 45+78=123 True
8 3 136 96+60=156 96+60=156 True
8 3 137 74+61=135 74+61=135 True
8 3 138 87+01=088 87+01=088 True
8 3 139 63+88=151 63+88=151 True
8 3 140 59+72=131 59+72=131 True
8 3 141 17+96=113 17+96=113 True
8 3 142 89+77=166 89+77=166 True
8 3 143 24+69=093 24+69=093 True
8 3 144 75+83=158 75+83=158 True
8 3 145 50+54=104 50+54=104 True
8 3 146 93+47=140 93+47=140 True
8 3 147 20+55=075 20+55=075 True
8 3 148 91+79=170 91+79=170 True
8 3 149 15+13=028 15+13=028 True
8 3 150 86+09=095 86+09=095 True
8 3 151 29+58=087 29+58=087 True
8 3 152 01+29=030 01+29=030 True
8 3 153 65+48=113 65+48=113 True
8 3 154 96+45=141 96+45=141 True
8 3 155 58+69=127 58+69=127 True
8 3 156 84+43=127 84+43=127 True
8 3 157 90+38=128 90+38=128 True
8 3 158 39+97=136 39+97=136 True
8 3 159 74+84=158 74+84=158 True
8 3 160 86+22=108 86+22=108 True
8 3 161 01+86=087 01+86=087 True
8 3 162 81+63=144 81+63=144 True
8 3 163 80+94=174 80+94=174 True
8 3 164 44+42=086 44+42=086 True
8 3 165 72+60=132 72+60=122 False
8 3 166 28+07=035 28+07=035 True
8 3 167 69+54=123 69+54=123 True
8 3 168 68+77=145 68+77=145 True
8 3 169 90+16=106 90+16=106 True
8 3 170 64+50=114 64+50=114 True
8 3 171 46+88=134 46+88=134 True
8 3 172 55+99=154 55+99=154 True
8 3 173 31+97=128 31+97=128 True
8 3 174 79+28=107 79+28=107 True
8 3 175 81+43=124 81+43=124 True
8 3 176 41+15=056 41+15=056 True
8 3 177 38+77=115 38+77=115 True
8 3 178 25+06=031 25+06=031 True
8 3 179 01+93=094 01+93=094 True
8 3 180 97+22=119 97+22=109 False
8 3 181 71+84=155 71+84=155 True
8 3 182 26+36=062 26+36=062 True
8 3 183 60+92=152 60+92=152 True
8 3 184 02+94=096 02+94=096 True
8 3 185 31+58=089 31+58=089 True
8 3 186 70+52=122 70+52=112 False
8 3 187 19+42=061 19+42=061 True
8 3 188 95+73=168 95+73=168 True
8 3 189 21+25=046 21+25=046 True
8 3 190 13+58=071 13+58=071 True
8 3 191 62+28=090 62+28=090 True
8 3 192 38+14=052 38+14=052 True
8 3 193 66+75=141 66+75=141 True
8 3 194 24+59=083 24+59=083 True
8 3 195 97+66=163 97+66=163 True
8 3 196 76+70=146 76+70=146 True
8 3 197 08+40=048 08+40=048 True
8 3 198 84+00=084 84+00=084 True
8 3 199 54+73=127 54+73=127 True
8 3 200 16+88=104 16+88=104 True
8 3 201 99+47=146 99+47=146 True
8 3 202 31+95=126 31+95=126 True
8 3 203 01+79=080 01+79=080 True
8 3 204 03+68=071 03+68=061 False
8 3 205 10+05=015 10+05=015 True
8 3 206 98+90=188 98+90=188 True
8 3 207 58+53=111 58+53=111 True
8 3 208 34+87=121 34+87=121 True
8 3 209 07+31=038 07+31=038 True
8 3 210 59+08=067 59+08=067 True
8 3 211 51+38=089 51+38=089 True
8 3 212 62+62=124 62+62=114 False
8 3 213 80+32=112 80+32=112 True
8 3 214 69+16=085 69+16=085 True
8 3 215 01+17=018 01+17=018 True
8 3 216 74+41=115 74+41=115 True
8 3 217 20+89=109 20+89=109 True
8 3 218 53+50=103 53+50=103 True
8 3 219 82+85=167 82+85=167 True
8 3 220 34+47=081 34+47=081 True
8 3 221 34+45=079 34+45=079 True
8 3 222 77+34=111 77+34=111 True
8 3 223 56+33=089 56+33=089 True
8 3 224 97+56=153 97+56=153 True
8 3 225 29+06=035 29+06=035 True
8 3 226 78+96=174 78+96=174 True
8 3 227 28+65=093 28+65=093 True
8 3 228 61+64=125 61+64=115 False
8 3 229 32+64=096 32+64=096 True
8 3 230 98+32=130 98+32=130 True
8 3 231 25+35=060 25+35=060 True
8 3 232 05+08=013 05+08=013 True
8 3 233 05+26=031 05+26=031 True
8 3 234 84+71=155 84+71=155 True
8 3 235 33+10=043 33+10=043 True
8 3 236 98+35=133 98+35=133 True
8 3 237 68+98=166 68+98=166 True
8 3 238 03+63=066 03+63=056 False
8 3 239 12+96=108 12+96=108 True
8 3 240 02+81=083 02+81=083 True
8 3 241 83+13=096 83+13=096 True
8 3 242 55+92=147 55+92=147 True
8 3 243 96+09=105 96+09=105 True
8 3 244 61+08=069 61+08=069 True
8 3 245 39+75=114 39+75=114 True
8 3 246 40+74=114 40+74=114 True
8 3 247 39+80=119 39+80=119 True
8 3 248 57+95=152 57+95=152 True
8 3 249 92+97=189 92+97=189 True
8 3 250 33+03=036 33+03=036 True
8 3 251 74+92=166 74+92=166 True
8 3 252 99+09=108 99+09=108 True
8 3 253 98+10=108 98+10=108 True
8 3 254 46+77=123 46+77=123 True
8 3 255 85+78=163 85+78=163 True
8 4 0 41+21=062 41+21=052 False
8 4 1 49+13=062 49+13=062 True
8 4 2 59+07=066 59+07=066 True
8 4 3 31+11=042 31+11=042 True
8 4 4 74+16=090 74+16=090 True
8 4 5 43+38=081 43+38=081 True
8 4 6 08+67=075 08+67=075 True
8 4 7 31+66=097 31+66=097 True
8 4 8 10+31=041 10+31=041 True
8 4 9 34+59=093 34+59=093 True
8 4 10 78+42=120 78+42=110 False
8 4 11 13+41=054 13+41=054 True
8 4 12 97+89=186 97+89=186 True
8 4 13 15+62=077 15+62=077 True
8 4 14 39+36=075 39+36=075 True
8 4 15 21+25=046 21+25=046 True
8 4 16 74+56=130 74+56=120 False
8 4 17 85+47=132 85+47=132 True
8 4 18 47+32=079 47+32=079 True
8 4 19 37+66=103 37+66=103 True
8 4 20 16+29=045 16+29=045 True
8 4 21 86+77=163 86+77=163 True
8 4 22 80+07=087 80+07=087 True
8 4 23 87+05=092 87+05=092 True
8 4 24 58+16=074 58+16=074 True
8 4 25 52+79=131 52+79=131 True
8 4 26 91+08=099 91+08=099 True
8 4 27 47+78=125 47+78=125 True
8 4 28 86+96=182 86+96=182 True
8 4 29 90+22=112 90+22=112 True
8 4 30 31+18=049 31+18=049 True
8 4 31 86+15=101 86+15=101 True
8 4 32 15+95=110 15+95=100 False
8 4 33 42+11=053 42+11=053 True
8 4 34 65+99=164 65+99=164 True
8 4 35 89+29=118 89+29=118 True
8 4 36 35+11=046 35+11=046 True
8 4 37 71+41=112 71+41=112 True
8 4 38 16+24=040 16+24=040 True
8 4 39 77+82=159 77+82=159 True
8 4 40 55+89=144 55+89=144 True
8 4 41 17+88=105 17+88=105 True
8 4 42 54+72=126 54+72=126 True
8 4 43 34+98=132 34+98=132 True
8 4 44 09+97=106 09+97=106 True
8 4 45 91+07=098 91+07=098 True
8 4 46 55+94=149 55+94=149 True
8 4 47 22+58=080 22+58=080 True
8 4 48 91+37=128 91+37=128 True
8 4 49 16+10=026 16+10=026 True
8 4 50 96+32=128 96+32=128 True
8 4 51 35+75=110 35+75=100 False
8 4 52 88+73=161 88+73=161 True
8 4 53 35+18=053 35+18=053 True
8 4 54 33+10=043 33+10=043 True
8 4 55 08+50=058 08+50=058 True
8 4 56 22+62=084 22+62=084 True
8 4 57 26+37=063 26+37=063 True
8 4 58 80+27=107 80+27=108 False
8 4 59 68+28=096 68+28=096 True
8 4 60 48+03=051 48+03=051 True
8 4 61 40+18=058 40+18=058 True
8 4 62 16+59=075 16+59=075 True
8 4 63 02+19=021 02+19=031 False
8 4 64 01+09=010 01+09=010 True
8 4 65 62+68=130 62+68=130 True
8 4 66 09+71=080 09+71=070 False
8 4 67 00+58=058 00+58=058 True
8 4 68 16+45=061 16+45=061 True
8 4 69 24+98=122 24+98=122 True
8 4 70 47+92=139 47+92=139 True
8 4 71 94+84=178 94+84=178 True
8 4 72 21+32=053 21+32=053 True
8 4 73 29+82=111 29+82=111 True
8 4 74 32+79=111 32+79=111 True
8 4 75 13+98=111 13+98=111 True
8 4 76 41+94=135 41+94=135 True
8 4 77 51+84=135 51+84=135 True
8 4 78 42+05=047 42+05=047 True
8 4 79 39+03=042 39+03=042 True
8 4 80 02+92=094 02+92=094 True
8 4 81 99+81=180 99+81=180 True
8 4 82 32+68=100 32+68=100 True
8 4 83 52+17=069 52+17=069 True
8 4 84 56+58=114 56+58=114 True
8 4 85 21+48=069 21+48=069 True
8 4 86 61+71=132 61+71=122 False
8 4 87 17+01=018 17+01=018 True
8 4 88 68+23=091 68+23=091 True
8 4 89 00+37=037 00+37=037 True
8 4 90 94+88=182 94+88=182 True
8 4 91 06+31=037 06+31=037 True
8 4 92 27+18=045 27+18=045 True
8 4 93 41+81=122 41+81=122 True
8 4 94 15+86=101 15+86=101 True
8 4 95 36+87=123 36+87=123 True
8 4 96 17+37=054 17+37=053 False
8 4 97 13+86=099 13+86=099 True
8 4 98 29+69=098 29+69=098 True
8 4 99 31+99=130 31+99=130 True
8 4 100 47+29=076 47+29=076 True
8 4 101 08+81=089 08+81=089 True
8 4 102 72+82=154 72+82=154 True
8 4 103 46+91=137 46+91=137 True
8 4 104 70+35=105 70+35=105 True
8 4 105 90+55=145 90+55=145 True
8 4 106 99+99=198 99+99=198 True
8 4 107 60+97=157 60+97=157 True
8 4 108 03+40=043 03+40=043 True
8 4 109 35+49=084 35+49=084 True
8 4 110 32+02=034 32+02=034 True
8 4 111 70+18=088 70+18=088 True
8 4 112 99+05=104 99+05=104 True
8 4 113 78+73=151 78+73=151 True
8 4 114 03+02=005 03+02=005 True
8 4 115 50+14=064 50+14=064 True
8 4 116 62+02=064 62+02=054 False
8 4 117 16+74=090 16+74=090 True
8 4 118 68+65=133 68+65=133 True
8 4 119 74+81=155 74+81=155 True
8 4 120 37+48=085 37+48=085 True
8 4 121 63+04=067 63+04=057 False
8 4 122 06+62=068 06+62=068 True
8 4 123 95+75=170 95+75=160 False
8 4 124 92+37=129 92+37=129 True
8 4 125 81+32=113 81+32=113 True
8 4 126 53+28=081 53+28=081 True
8 4 127 52+42=094 52+42=094 True
8 4 128 66+97=163 66+97=163 True
8 4 129 00+48=048 00+48=048 True
8 4 130 65+32=097 65+32=097 True
8 4 131 60+89=149 60+89=159 False
8 4 132 71+61=132 71+61=122 False
8 4 133 98+50=148 98+50=148 True
8 4 134 90+96=186 90+96=186 True
8 4 135 02+96=098 02+96=098 True
8 4 136 62+75=137 62+75=137 True
8 4 137 41+28=069 41+28=069 True
8 4 138 95+79=174 95+79=174 True
8 4 139 48+41=089 48+41=089 True
8 4 140 87+95=182 87+95=182 True
8 4 141 75+38=113 75+38=103 False
8 4 142 31+55=086 31+55=086 True
8 4 143 54+63=117 54+63=117 True
8 4 144 75+82=157 75+82=157 True
8 4 145 46+45=091 46+45=091 True
8 4 146 13+08=021 13+08=021 True
8 4 147 77+97=174 77+97=173 False
8 4 148 37+35=072 37+35=072 True
8 4 149 21+89=110 21+89=110 True
8 4 150 58+51=109 58+51=109 True
8 4 151 91+48=139 91+48=139 True
8 4 152 33+23=056 33+23=056 True
8 4 153 80+96=176 80+96=176 True
8 4 154 78+02=080 78+02=070 False
8 4 155 38+95=133 38+95=133 True
8 4 156 99+25=124 99+25=124 True
8 4 157 30+76=106 30+76=106 True
8 4 158 42+40=082 42+40=082 True
8 4 159 85+58=143 85+58=143 True
8 4 160 44+46=090 44+46=090 True
8 4 161 06+41=047 06+41=047 True
8 4 162 65+90=155 65+90=155 True
8 4 163 43+83=126 43+83=126 True
8 4 164 36+61=097 36+61=097 True
8 4 165 61+51=112 61+51=102 False
8 4 166 38+09=047 38+09=047 True
8 4 167 21+97=118 21+97=118 True
8 4 168 83+30=113 83+30=113 True
8 4 169 11+79=090 11+79=090 True
8 4 170 14+29=043 14+29=043 True
8 4 171 21+11=032 21+11=032 True
8 4 172 43+53=096 43+53=096 True
8 4 173 02+58=060 02+58=060 True
8 4 174 78+82=160 78+82=160 True
8 4 175 91+11=102 91+11=102 True
8 4 176 58+54=112 58+54=112 True
8 4 177 00+15=015 00+15=015 True
8 4 178 83+51=134 83+51=134 True
8 4 179 44+72=116 44+72=116 True
8 4 180 71+20=091 71+20=091 True
8 4 181 24+99=123 24+99=123 True
8 4 182 46+30=076 46+30=076 True
8 4 183 08+67=075 08+67=075 True
8 4 184 47+42=089 47+42=089 True
8 4 185 95+67=162 95+67=162 True
8 4 186 40+56=096 40+56=096 True
8 4 187 17+95=112 17+95=112 True
8 4 188 94+66=160 94+66=160 True
8 4 189 14+58=072 14+58=072 True
8 4 190 56+05=061 56+05=061 True
8 4 191 70+01=071 70+01=061 False
8 4 192 97+59=156 97+59=156 True
8 4 193 94+67=161 94+67=161 True
8 4 194 13+41=054 13+41=054 True
8 4 195 85+15=100 85+15=090 False
8 4 196 48+53=101 48+53=101 True
8 4 197 62+75=137 62+75=137 True
8 4 198 87+47=134 87+47=133 False
8 4 199 31+88=119 31+88=119 True
8 4 200 97+16=113 97+16=113 True
8 4 201 48+45=093 48+45=093 True
8 4 202 99+00=099 99+00=099 True
8 4 203 15+01=016 15+01=016 True
8 4 204 28+96=124 28+96=124 True
8 4 205 20+11=031 20+11=031 True
8 4 206 07+56=063 07+56=063 True
8 4 207 06+08=014 06+08=014 True
8 4 208 45+46=091 45+46=091 True
8 4 209 48+85=133 48+85=133 True
8 4 210 62+14=076 62+14=076 True
8 4 211 82+31=113 82+31=113 True
8 4 212 85+88=173 85+88=173 True
8 4 213 77+08=085 77+08=075 False
8 4 214 16+64=080 16+64=080 True
8 4 215 00+27=027 00+27=027 True
8 4 216 36+75=111 36+75=111 True
8 4 217 38+38=076 38+38=076 True
8 4 218 88+32=120 88+32=110 False
8 4 219 09+88=097 09+88=097 True
8 4 220 96+87=183 96+87=183 True
8 4 221 71+29=100 71+29=090 False
8 4 222 99+13=112 99+13=112 True
8 4 223 03+13=016 03+13=016 True
8 4 224 67+23=090 67+23=080 False
8 4 225 15+98=113 15+98=113 True
8 4 226 10+08=018 10+08=018 True
8 4 227 46+24=070 46+24=060 False
8 4 228 55+63=118 55+63=118 True
8 4 229 28+06=034 28+06=034 True
8 4 230 43+87=130 43+87=130 True
8 4 231 34+05=039 34+05=049 False
2024-12-17 09:44:21,285 - root - WARNING - The method `test_one_epoch` is not implemented. Please override this method in a subclass or use a custom callback.
2024-12-17 09:44:21,287 - root - INFO - ====================================================== Starting Train Epoch: 9/9 ======================================================
2024-12-17 09:44:21,287 - root - INFO - Learning rates for each parameter group: 0.00117851130197757937, 0.00117851130197757937
0%| | 0/28 [00:00<?, ?it/s]
Epoch: 9, Step: 1: 0%| | 0/28 [00:00<?, ?it/s]
Epoch: 9, Step: 1: 0%| | 0/28 [00:00<?, ?it/s, total_batch_loss=50.79201, average_batch_loss=0.19841, average_batch_perplexity=1.21946, lr=0.001178511, 0.001178511]
Epoch: 9, Step: 2: 0%| | 0/28 [00:00<?, ?it/s, total_batch_loss=50.79201, average_batch_loss=0.19841, average_batch_perplexity=1.21946, lr=0.001178511, 0.001178511]
Epoch: 9, Step: 2: 0%| | 0/28 [00:00<?, ?it/s, total_batch_loss=48.12430, average_batch_loss=0.18799, average_batch_perplexity=1.20682, lr=0.001175901, 0.001175901]
Epoch: 9, Step: 2: 7%|▋ | 2/28 [00:00<00:02, 11.67it/s, total_batch_loss=48.12430, average_batch_loss=0.18799, average_batch_perplexity=1.20682, lr=0.001175901, 0.001175901]
Epoch: 9, Step: 3: 7%|▋ | 2/28 [00:00<00:02, 11.67it/s, total_batch_loss=48.12430, average_batch_loss=0.18799, average_batch_perplexity=1.20682, lr=0.001175901, 0.001175901]
Epoch: 9, Step: 3: 7%|▋ | 2/28 [00:00<00:02, 11.67it/s, total_batch_loss=49.34882, average_batch_loss=0.19277, average_batch_perplexity=1.21260, lr=0.001173308, 0.001173308]
Epoch: 9, Step: 4: 7%|▋ | 2/28 [00:00<00:02, 11.67it/s, total_batch_loss=49.34882, average_batch_loss=0.19277, average_batch_perplexity=1.21260, lr=0.001173308, 0.001173308]
Epoch: 9, Step: 4: 7%|▋ | 2/28 [00:00<00:02, 11.67it/s, total_batch_loss=46.44347, average_batch_loss=0.18142, average_batch_perplexity=1.19892, lr=0.001170732, 0.001170732]
Epoch: 9, Step: 4: 14%|█▍ | 4/28 [00:00<00:02, 11.26it/s, total_batch_loss=46.44347, average_batch_loss=0.18142, average_batch_perplexity=1.19892, lr=0.001170732, 0.001170732]
Epoch: 9, Step: 5: 14%|█▍ | 4/28 [00:00<00:02, 11.26it/s, total_batch_loss=46.44347, average_batch_loss=0.18142, average_batch_perplexity=1.19892, lr=0.001170732, 0.001170732]
Epoch: 9, Step: 5: 14%|█▍ | 4/28 [00:00<00:02, 11.26it/s, total_batch_loss=45.92714, average_batch_loss=0.17940, average_batch_perplexity=1.19650, lr=0.001168173, 0.001168173]
Epoch: 9, Step: 6: 14%|█▍ | 4/28 [00:00<00:02, 11.26it/s, total_batch_loss=45.92714, average_batch_loss=0.17940, average_batch_perplexity=1.19650, lr=0.001168173, 0.001168173]
Epoch: 9, Step: 6: 14%|█▍ | 4/28 [00:00<00:02, 11.26it/s, total_batch_loss=50.60395, average_batch_loss=0.19767, average_batch_perplexity=1.21856, lr=0.001165631, 0.001165631]
Epoch: 9, Step: 6: 21%|██▏ | 6/28 [00:00<00:01, 11.64it/s, total_batch_loss=50.60395, average_batch_loss=0.19767, average_batch_perplexity=1.21856, lr=0.001165631, 0.001165631]
Epoch: 9, Step: 7: 21%|██▏ | 6/28 [00:00<00:01, 11.64it/s, total_batch_loss=50.60395, average_batch_loss=0.19767, average_batch_perplexity=1.21856, lr=0.001165631, 0.001165631]
Epoch: 9, Step: 7: 21%|██▏ | 6/28 [00:00<00:01, 11.64it/s, total_batch_loss=44.93650, average_batch_loss=0.17553, average_batch_perplexity=1.19188, lr=0.001163105, 0.001163105]
Epoch: 9, Step: 8: 21%|██▏ | 6/28 [00:00<00:01, 11.64it/s, total_batch_loss=44.93650, average_batch_loss=0.17553, average_batch_perplexity=1.19188, lr=0.001163105, 0.001163105]
Epoch: 9, Step: 8: 21%|██▏ | 6/28 [00:00<00:01, 11.64it/s, total_batch_loss=49.44500, average_batch_loss=0.19314, average_batch_perplexity=1.21306, lr=0.001160596, 0.001160596]
Epoch: 9, Step: 8: 29%|██▊ | 8/28 [00:00<00:01, 11.37it/s, total_batch_loss=49.44500, average_batch_loss=0.19314, average_batch_perplexity=1.21306, lr=0.001160596, 0.001160596]
Epoch: 9, Step: 9: 29%|██▊ | 8/28 [00:00<00:01, 11.37it/s, total_batch_loss=49.44500, average_batch_loss=0.19314, average_batch_perplexity=1.21306, lr=0.001160596, 0.001160596]
Epoch: 9, Step: 9: 29%|██▊ | 8/28 [00:00<00:01, 11.37it/s, total_batch_loss=50.19176, average_batch_loss=0.19606, average_batch_perplexity=1.21660, lr=0.001158103, 0.001158103]
Epoch: 9, Step: 10: 29%|██▊ | 8/28 [00:00<00:01, 11.37it/s, total_batch_loss=50.19176, average_batch_loss=0.19606, average_batch_perplexity=1.21660, lr=0.001158103, 0.001158103]
Epoch: 9, Step: 10: 29%|██▊ | 8/28 [00:00<00:01, 11.37it/s, total_batch_loss=49.01073, average_batch_loss=0.19145, average_batch_perplexity=1.21100, lr=0.001155625, 0.001155625]
Epoch: 9, Step: 10: 36%|███▌ | 10/28 [00:00<00:01, 11.60it/s, total_batch_loss=49.01073, average_batch_loss=0.19145, average_batch_perplexity=1.21100, lr=0.001155625, 0.001155625]
Epoch: 9, Step: 11: 36%|███▌ | 10/28 [00:00<00:01, 11.60it/s, total_batch_loss=49.01073, average_batch_loss=0.19145, average_batch_perplexity=1.21100, lr=0.001155625, 0.001155625]
Epoch: 9, Step: 11: 36%|███▌ | 10/28 [00:00<00:01, 11.60it/s, total_batch_loss=51.62689, average_batch_loss=0.20167, average_batch_perplexity=1.22344, lr=0.001153164, 0.001153164]
Epoch: 9, Step: 12: 36%|███▌ | 10/28 [00:01<00:01, 11.60it/s, total_batch_loss=51.62689, average_batch_loss=0.20167, average_batch_perplexity=1.22344, lr=0.001153164, 0.001153164]
Epoch: 9, Step: 12: 36%|███▌ | 10/28 [00:01<00:01, 11.60it/s, total_batch_loss=46.63403, average_batch_loss=0.18216, average_batch_perplexity=1.19981, lr=0.001150718, 0.001150718]
Epoch: 9, Step: 12: 43%|████▎ | 12/28 [00:01<00:01, 11.81it/s, total_batch_loss=46.63403, average_batch_loss=0.18216, average_batch_perplexity=1.19981, lr=0.001150718, 0.001150718]
Epoch: 9, Step: 13: 43%|████▎ | 12/28 [00:01<00:01, 11.81it/s, total_batch_loss=46.63403, average_batch_loss=0.18216, average_batch_perplexity=1.19981, lr=0.001150718, 0.001150718]
Epoch: 9, Step: 13: 43%|████▎ | 12/28 [00:01<00:01, 11.81it/s, total_batch_loss=41.82396, average_batch_loss=0.16337, average_batch_perplexity=1.17748, lr=0.001148288, 0.001148288]
Epoch: 9, Step: 14: 43%|████▎ | 12/28 [00:01<00:01, 11.81it/s, total_batch_loss=41.82396, average_batch_loss=0.16337, average_batch_perplexity=1.17748, lr=0.001148288, 0.001148288]
Epoch: 9, Step: 14: 43%|████▎ | 12/28 [00:01<00:01, 11.81it/s, total_batch_loss=51.75311, average_batch_loss=0.20216, average_batch_perplexity=1.22404, lr=0.001145873, 0.001145873]
Epoch: 9, Step: 14: 50%|█████ | 14/28 [00:01<00:01, 12.09it/s, total_batch_loss=51.75311, average_batch_loss=0.20216, average_batch_perplexity=1.22404, lr=0.001145873, 0.001145873]
Epoch: 9, Step: 15: 50%|█████ | 14/28 [00:01<00:01, 12.09it/s, total_batch_loss=51.75311, average_batch_loss=0.20216, average_batch_perplexity=1.22404, lr=0.001145873, 0.001145873]
Epoch: 9, Step: 15: 50%|█████ | 14/28 [00:01<00:01, 12.09it/s, total_batch_loss=44.71657, average_batch_loss=0.17467, average_batch_perplexity=1.19086, lr=0.001143473, 0.001143473]
Epoch: 9, Step: 16: 50%|█████ | 14/28 [00:01<00:01, 12.09it/s, total_batch_loss=44.71657, average_batch_loss=0.17467, average_batch_perplexity=1.19086, lr=0.001143473, 0.001143473]
Epoch: 9, Step: 16: 50%|█████ | 14/28 [00:01<00:01, 12.09it/s, total_batch_loss=39.45398, average_batch_loss=0.15412, average_batch_perplexity=1.16663, lr=0.001141089, 0.001141089]
Epoch: 9, Step: 16: 57%|█████▋ | 16/28 [00:01<00:00, 12.16it/s, total_batch_loss=39.45398, average_batch_loss=0.15412, average_batch_perplexity=1.16663, lr=0.001141089, 0.001141089]
Epoch: 9, Step: 17: 57%|█████▋ | 16/28 [00:01<00:00, 12.16it/s, total_batch_loss=39.45398, average_batch_loss=0.15412, average_batch_perplexity=1.16663, lr=0.001141089, 0.001141089]
Epoch: 9, Step: 17: 57%|█████▋ | 16/28 [00:01<00:00, 12.16it/s, total_batch_loss=47.38599, average_batch_loss=0.18510, average_batch_perplexity=1.20334, lr=0.001138719, 0.001138719]
Epoch: 9, Step: 18: 57%|█████▋ | 16/28 [00:01<00:00, 12.16it/s, total_batch_loss=47.38599, average_batch_loss=0.18510, average_batch_perplexity=1.20334, lr=0.001138719, 0.001138719]
Epoch: 9, Step: 18: 57%|█████▋ | 16/28 [00:01<00:00, 12.16it/s, total_batch_loss=45.27081, average_batch_loss=0.17684, average_batch_perplexity=1.19344, lr=0.001136364, 0.001136364]
Epoch: 9, Step: 18: 64%|██████▍ | 18/28 [00:01<00:00, 12.16it/s, total_batch_loss=45.27081, average_batch_loss=0.17684, average_batch_perplexity=1.19344, lr=0.001136364, 0.001136364]
Epoch: 9, Step: 19: 64%|██████▍ | 18/28 [00:01<00:00, 12.16it/s, total_batch_loss=45.27081, average_batch_loss=0.17684, average_batch_perplexity=1.19344, lr=0.001136364, 0.001136364]
Epoch: 9, Step: 19: 64%|██████▍ | 18/28 [00:01<00:00, 12.16it/s, total_batch_loss=44.93793, average_batch_loss=0.17554, average_batch_perplexity=1.19189, lr=0.001134023, 0.001134023]
Epoch: 9, Step: 20: 64%|██████▍ | 18/28 [00:01<00:00, 12.16it/s, total_batch_loss=44.93793, average_batch_loss=0.17554, average_batch_perplexity=1.19189, lr=0.001134023, 0.001134023]
Epoch: 9, Step: 20: 64%|██████▍ | 18/28 [00:01<00:00, 12.16it/s, total_batch_loss=42.98513, average_batch_loss=0.16791, average_batch_perplexity=1.18283, lr=0.001131697, 0.001131697]
Epoch: 9, Step: 20: 71%|███████▏ | 20/28 [00:01<00:00, 12.23it/s, total_batch_loss=42.98513, average_batch_loss=0.16791, average_batch_perplexity=1.18283, lr=0.001131697, 0.001131697]
Epoch: 9, Step: 21: 71%|███████▏ | 20/28 [00:01<00:00, 12.23it/s, total_batch_loss=42.98513, average_batch_loss=0.16791, average_batch_perplexity=1.18283, lr=0.001131697, 0.001131697]
Epoch: 9, Step: 21: 71%|███████▏ | 20/28 [00:01<00:00, 12.23it/s, total_batch_loss=39.07924, average_batch_loss=0.15265, average_batch_perplexity=1.16492, lr=0.001129385, 0.001129385]
Epoch: 9, Step: 22: 71%|███████▏ | 20/28 [00:01<00:00, 12.23it/s, total_batch_loss=39.07924, average_batch_loss=0.15265, average_batch_perplexity=1.16492, lr=0.001129385, 0.001129385]
Epoch: 9, Step: 22: 71%|███████▏ | 20/28 [00:01<00:00, 12.23it/s, total_batch_loss=35.62823, average_batch_loss=0.13917, average_batch_perplexity=1.14932, lr=0.001127087, 0.001127087]
Epoch: 9, Step: 22: 79%|███████▊ | 22/28 [00:01<00:00, 12.23it/s, total_batch_loss=35.62823, average_batch_loss=0.13917, average_batch_perplexity=1.14932, lr=0.001127087, 0.001127087]
Epoch: 9, Step: 23: 79%|███████▊ | 22/28 [00:01<00:00, 12.23it/s, total_batch_loss=35.62823, average_batch_loss=0.13917, average_batch_perplexity=1.14932, lr=0.001127087, 0.001127087]
Epoch: 9, Step: 23: 79%|███████▊ | 22/28 [00:01<00:00, 12.23it/s, total_batch_loss=42.13190, average_batch_loss=0.16458, average_batch_perplexity=1.17890, lr=0.001124803, 0.001124803]
Epoch: 9, Step: 24: 79%|███████▊ | 22/28 [00:01<00:00, 12.23it/s, total_batch_loss=42.13190, average_batch_loss=0.16458, average_batch_perplexity=1.17890, lr=0.001124803, 0.001124803]
Epoch: 9, Step: 24: 79%|███████▊ | 22/28 [00:01<00:00, 12.23it/s, total_batch_loss=42.47777, average_batch_loss=0.16593, average_batch_perplexity=1.18049, lr=0.001122533, 0.001122533]
Epoch: 9, Step: 24: 86%|████████▌ | 24/28 [00:01<00:00, 12.42it/s, total_batch_loss=42.47777, average_batch_loss=0.16593, average_batch_perplexity=1.18049, lr=0.001122533, 0.001122533]
Epoch: 9, Step: 25: 86%|████████▌ | 24/28 [00:02<00:00, 12.42it/s, total_batch_loss=42.47777, average_batch_loss=0.16593, average_batch_perplexity=1.18049, lr=0.001122533, 0.001122533]
Epoch: 9, Step: 25: 86%|████████▌ | 24/28 [00:02<00:00, 12.42it/s, total_batch_loss=38.05074, average_batch_loss=0.14864, average_batch_perplexity=1.16025, lr=0.001120277, 0.001120277]
Epoch: 9, Step: 26: 86%|████████▌ | 24/28 [00:02<00:00, 12.42it/s, total_batch_loss=38.05074, average_batch_loss=0.14864, average_batch_perplexity=1.16025, lr=0.001120277, 0.001120277]
Epoch: 9, Step: 26: 86%|████████▌ | 24/28 [00:02<00:00, 12.42it/s, total_batch_loss=37.62466, average_batch_loss=0.14697, average_batch_perplexity=1.15832, lr=0.001118034, 0.001118034]
Epoch: 9, Step: 26: 93%|█████████▎| 26/28 [00:02<00:00, 12.45it/s, total_batch_loss=37.62466, average_batch_loss=0.14697, average_batch_perplexity=1.15832, lr=0.001118034, 0.001118034]
Epoch: 9, Step: 27: 93%|█████████▎| 26/28 [00:02<00:00, 12.45it/s, total_batch_loss=37.62466, average_batch_loss=0.14697, average_batch_perplexity=1.15832, lr=0.001118034, 0.001118034]
Epoch: 9, Step: 27: 93%|█████████▎| 26/28 [00:02<00:00, 12.45it/s, total_batch_loss=42.60037, average_batch_loss=0.16641, average_batch_perplexity=1.18105, lr=0.001115805, 0.001115805]
Epoch: 9, Step: 28: 93%|█████████▎| 26/28 [00:02<00:00, 12.45it/s, total_batch_loss=42.60037, average_batch_loss=0.16641, average_batch_perplexity=1.18105, lr=0.001115805, 0.001115805]
Epoch: 9, Step: 28: 93%|█████████▎| 26/28 [00:02<00:00, 12.45it/s, total_batch_loss=17.79465, average_batch_loss=0.20221, average_batch_perplexity=1.22411, lr=0.001113589, 0.001113589]
Epoch: 9, Step: 28: 100%|██████████| 28/28 [00:02<00:00, 13.65it/s, total_batch_loss=17.79465, average_batch_loss=0.20221, average_batch_perplexity=1.22411, lr=0.001113589, 0.001113589]
2024-12-17 09:44:23,559 - root - INFO - Total Samples: 7000
2024-12-17 09:44:23,560 - root - INFO - Total Batches: 28
2024-12-17 09:44:23,560 - root - INFO - Average Epoch Train Loss: 0.17669
2024-12-17 09:44:23,561 - root - INFO - Average Epoch Train Perplexity: 1.19326
2024-12-17 09:44:23,561 - root - INFO -
2024-12-17 09:44:23,562 - root - INFO - ====================================================== Starting Valid Epoch: 9/9 ======================================================
0%| | 0/8 [00:00<?, ?it/s]
Epoch: 9, Step: 1: 0%| | 0/8 [00:00<?, ?it/s]
Epoch: 9, Step: 1: 0%| | 0/8 [00:00<?, ?it/s, total_batch_loss=40.87537, average_batch_loss=0.15967, average_batch_perplexity=1.17312]
Epoch: 9, Step: 2: 0%| | 0/8 [00:00<?, ?it/s, total_batch_loss=40.87537, average_batch_loss=0.15967, average_batch_perplexity=1.17312]
Epoch: 9, Step: 2: 0%| | 0/8 [00:00<?, ?it/s, total_batch_loss=42.97948, average_batch_loss=0.16789, average_batch_perplexity=1.18280]
Epoch: 9, Step: 3: 0%| | 0/8 [00:00<?, ?it/s, total_batch_loss=42.97948, average_batch_loss=0.16789, average_batch_perplexity=1.18280]
Epoch: 9, Step: 3: 0%| | 0/8 [00:00<?, ?it/s, total_batch_loss=48.15429, average_batch_loss=0.18810, average_batch_perplexity=1.20696]
Epoch: 9, Step: 3: 38%|███▊ | 3/8 [00:00<00:00, 23.61it/s, total_batch_loss=48.15429, average_batch_loss=0.18810, average_batch_perplexity=1.20696]
Epoch: 9, Step: 4: 38%|███▊ | 3/8 [00:00<00:00, 23.61it/s, total_batch_loss=48.15429, average_batch_loss=0.18810, average_batch_perplexity=1.20696]
Epoch: 9, Step: 4: 38%|███▊ | 3/8 [00:00<00:00, 23.61it/s, total_batch_loss=39.25602, average_batch_loss=0.15334, average_batch_perplexity=1.16573]
Epoch: 9, Step: 5: 38%|███▊ | 3/8 [00:00<00:00, 23.61it/s, total_batch_loss=39.25602, average_batch_loss=0.15334, average_batch_perplexity=1.16573]
Epoch: 9, Step: 5: 38%|███▊ | 3/8 [00:00<00:00, 23.61it/s, total_batch_loss=41.24788, average_batch_loss=0.16112, average_batch_perplexity=1.17483]
Epoch: 9, Step: 6: 38%|███▊ | 3/8 [00:00<00:00, 23.61it/s, total_batch_loss=41.24788, average_batch_loss=0.16112, average_batch_perplexity=1.17483]
Epoch: 9, Step: 6: 38%|███▊ | 3/8 [00:00<00:00, 23.61it/s, total_batch_loss=45.03639, average_batch_loss=0.17592, average_batch_perplexity=1.19235]
Epoch: 9, Step: 6: 75%|███████▌ | 6/8 [00:00<00:00, 23.85it/s, total_batch_loss=45.03639, average_batch_loss=0.17592, average_batch_perplexity=1.19235]
Epoch: 9, Step: 7: 75%|███████▌ | 6/8 [00:00<00:00, 23.85it/s, total_batch_loss=45.03639, average_batch_loss=0.17592, average_batch_perplexity=1.19235]
Epoch: 9, Step: 7: 75%|███████▌ | 6/8 [00:00<00:00, 23.85it/s, total_batch_loss=41.77565, average_batch_loss=0.16319, average_batch_perplexity=1.17726]
Epoch: 9, Step: 8: 75%|███████▌ | 6/8 [00:00<00:00, 23.85it/s, total_batch_loss=41.77565, average_batch_loss=0.16319, average_batch_perplexity=1.17726]
Epoch: 9, Step: 8: 75%|███████▌ | 6/8 [00:00<00:00, 23.85it/s, total_batch_loss=27.56857, average_batch_loss=0.13254, average_batch_perplexity=1.14173]
2024-12-17 09:44:23,894 - root - INFO - Total Samples: 2000
2024-12-17 09:44:23,895 - root - INFO - Total Batches: 8
2024-12-17 09:44:23,895 - root - INFO - Average Epoch Valid Loss: 0.16345
2024-12-17 09:44:23,896 - root - INFO - Average Epoch Valid Perplexity: 1.17756
2024-12-17 09:44:23,896 - root - INFO -
Evaluating and Generation.: 0%| | 0/4 [00:00<?, ?it/s]
Evaluating and Generation.: 0%| | 0/4 [00:00<?, ?it/s, accuracy: 0.7500]
Evaluating and Generation.: 0%| | 0/4 [00:00<?, ?it/s, accuracy: 0.7578]
Evaluating and Generation.: 50%|█████ | 2/4 [00:00<00:00, 10.45it/s, accuracy: 0.7578]
Evaluating and Generation.: 50%|█████ | 2/4 [00:00<00:00, 10.45it/s, accuracy: 0.7930]
Evaluating and Generation.: 50%|█████ | 2/4 [00:00<00:00, 10.45it/s, accuracy: 0.7457]
Evaluating and Generation.: 100%|██████████| 4/4 [00:00<00:00, 10.80it/s, accuracy: 0.7457]
2024-12-17 09:44:24,274 - root - INFO - Correct/Total Samples: 762/1000
2024-12-17 09:44:24,275 - root - INFO - Eval Accuracy: 0.762
2024-12-17 09:44:24,294 - root - INFO -
epoch batch_index sample_index equation generated correct
9 1 0 13+48=061 13+48=071 False
9 1 1 16+55=071 16+55=071 True
9 1 2 79+34=113 79+34=113 True
9 1 3 35+44=079 35+44=089 False
9 1 4 16+50=066 16+50=066 True
9 1 5 28+47=075 28+47=075 True
9 1 6 00+74=074 00+74=074 True
9 1 7 15+20=035 15+20=035 True
9 1 8 72+60=132 72+60=132 True
9 1 9 63+68=131 63+68=131 True
9 1 10 29+45=074 29+45=074 True
9 1 11 34+60=094 34+60=094 True
9 1 12 53+70=123 53+70=123 True
9 1 13 70+50=120 70+50=120 True
9 1 14 11+84=095 11+84=096 False
9 1 15 42+71=113 42+71=113 True
9 1 16 98+22=120 98+22=110 False
9 1 17 02+02=004 02+02=005 False
9 1 18 15+85=100 15+85=100 True
9 1 19 21+78=099 21+78=099 True
9 1 20 61+79=140 61+79=140 True
9 1 21 25+99=124 25+99=124 True
9 1 22 09+85=094 09+85=094 True
9 1 23 60+91=151 60+91=151 True
9 1 24 35+30=065 35+30=075 False
9 1 25 24+51=075 24+51=075 True
9 1 26 93+91=184 93+91=185 False
9 1 27 39+96=135 39+96=135 True
9 1 28 64+35=099 64+35=099 True
9 1 29 36+22=058 36+22=068 False
9 1 30 68+45=113 68+45=113 True
9 1 31 16+84=100 16+84=100 True
9 1 32 91+52=143 91+52=144 False
9 1 33 97+36=133 97+36=133 True
9 1 34 27+37=064 27+37=074 False
9 1 35 99+82=181 99+82=181 True
9 1 36 03+42=045 03+42=056 False
9 1 37 18+38=056 18+38=066 False
9 1 38 32+20=052 32+20=052 True
9 1 39 38+13=051 38+13=061 False
9 1 40 68+42=110 68+42=110 True
9 1 41 64+00=064 64+00=064 True
9 1 42 48+94=142 48+94=142 True
9 1 43 58+36=094 58+36=094 True
9 1 44 41+22=063 41+22=064 False
9 1 45 23+58=081 23+58=081 True
9 1 46 67+46=113 67+46=113 True
9 1 47 40+78=118 40+78=118 True
9 1 48 90+38=128 90+38=128 True
9 1 49 89+52=141 89+52=141 True
9 1 50 37+77=114 37+77=114 True
9 1 51 29+76=105 29+76=105 True
9 1 52 42+90=132 42+90=132 True
9 1 53 45+82=127 45+82=127 True
9 1 54 35+95=130 35+95=120 False
9 1 55 92+98=190 92+98=190 True
9 1 56 73+91=164 73+91=164 True
9 1 57 53+97=150 53+97=150 True
9 1 58 98+69=167 98+69=167 True
9 1 59 20+46=066 20+46=066 True
9 1 60 48+69=117 48+69=117 True
9 1 61 62+31=093 62+31=093 True
9 1 62 80+59=139 80+59=139 True
9 1 63 58+12=070 58+12=070 True
9 1 64 08+96=104 08+96=104 True
9 1 65 67+06=073 67+06=073 True
9 1 66 22+04=026 22+04=027 False
9 1 67 61+87=148 61+87=148 True
9 1 68 95+27=122 95+27=122 True
9 1 69 49+83=132 49+83=132 True
9 1 70 43+00=043 43+00=053 False
9 1 71 01+85=086 01+85=086 True
9 1 72 11+68=079 11+68=079 True
9 1 73 80+03=083 80+03=083 True
9 1 74 54+83=137 54+83=137 True
9 1 75 73+47=120 73+47=110 False
9 1 76 99+93=192 99+93=192 True
9 1 77 99+13=112 99+13=112 True
9 1 78 92+66=158 92+66=158 True
9 1 79 90+31=121 90+31=121 True
9 1 80 25+69=094 25+69=094 True
9 1 81 25+44=069 25+44=079 False
9 1 82 00+93=093 00+93=093 True
9 1 83 88+87=175 88+87=175 True
9 1 84 47+56=103 47+56=103 True
9 1 85 43+59=102 43+59=102 True
9 1 86 22+00=022 22+00=022 True
9 1 87 34+04=038 34+04=038 True
9 1 88 65+13=078 65+13=078 True
9 1 89 39+82=121 39+82=121 True
9 1 90 66+83=149 66+83=149 True
9 1 91 51+69=120 51+69=110 False
9 1 92 80+21=101 80+21=101 True
9 1 93 36+79=115 36+79=115 True
9 1 94 21+68=089 21+68=089 True
9 1 95 11+66=077 11+66=077 True
9 1 96 55+19=074 55+19=074 True
9 1 97 51+61=112 51+61=112 True
9 1 98 38+88=126 38+88=126 True
9 1 99 37+27=064 37+27=074 False
9 1 100 18+63=081 18+63=081 True
9 1 101 48+11=059 48+11=069 False
9 1 102 72+68=140 72+68=140 True
9 1 103 37+39=076 37+39=076 True
9 1 104 64+95=159 64+95=159 True
9 1 105 49+75=124 49+75=124 True
9 1 106 45+66=111 45+66=111 True
9 1 107 34+87=121 34+87=121 True
9 1 108 02+84=086 02+84=087 False
9 1 109 95+00=095 95+00=095 True
9 1 110 09+56=065 09+56=065 True
9 1 111 22+66=088 22+66=088 True
9 1 112 43+18=061 43+18=071 False
9 1 113 61+35=096 61+35=096 True
9 1 114 13+73=086 13+73=087 False
9 1 115 25+95=120 25+95=110 False
9 1 116 73+96=169 73+96=169 True
9 1 117 03+96=099 03+96=099 True
9 1 118 97+82=179 97+82=179 True
9 1 119 18+42=060 18+42=070 False
9 1 120 29+98=127 29+98=127 True
9 1 121 61+00=061 61+00=061 True
9 1 122 22+98=120 22+98=120 True
9 1 123 12+50=062 12+50=062 True
9 1 124 02+58=060 02+58=060 True
9 1 125 75+86=161 75+86=161 True
9 1 126 31+57=088 31+57=088 True
9 1 127 49+82=131 49+82=131 True
9 1 128 15+33=048 15+33=058 False
9 1 129 49+57=106 49+57=106 True
9 1 130 61+70=131 61+70=131 True
9 1 131 91+51=142 91+51=142 True
9 1 132 50+05=055 50+05=055 True
9 1 133 44+16=060 44+16=070 False
9 1 134 92+01=093 92+01=093 True
9 1 135 85+82=167 85+82=167 True
9 1 136 07+41=048 07+41=058 False
9 1 137 40+06=046 40+06=056 False
9 1 138 79+62=141 79+62=141 True
9 1 139 95+62=157 95+62=157 True
9 1 140 42+93=135 42+93=136 False
9 1 141 32+73=105 32+73=106 False
9 1 142 47+09=056 47+09=056 True
9 1 143 59+50=109 59+50=109 True
9 1 144 61+77=138 61+77=138 True
9 1 145 64+06=070 64+06=070 True
9 1 146 35+10=045 35+10=055 False
9 1 147 32+88=120 32+88=120 True
9 1 148 03+95=098 03+95=098 True
9 1 149 11+38=049 11+38=059 False
9 1 150 21+67=088 21+67=088 True
9 1 151 33+25=058 33+25=068 False
9 1 152 63+45=108 63+45=108 True
9 1 153 56+12=068 56+12=078 False
9 1 154 19+79=098 19+79=097 False
9 1 155 60+43=103 60+43=104 False
9 1 156 07+61=068 07+61=068 True
9 1 157 58+03=061 58+03=071 False
9 1 158 11+10=021 11+10=011 False
9 1 159 49+89=138 49+89=138 True
9 1 160 37+58=095 37+58=095 True
9 1 161 59+78=137 59+78=137 True
9 1 162 11+21=032 11+21=032 True
9 1 163 37+43=080 37+43=080 True
9 1 164 44+21=065 44+21=065 True
9 1 165 22+97=119 22+97=119 True
9 1 166 65+35=100 65+35=100 True
9 1 167 06+51=057 06+51=057 True
9 1 168 65+25=090 65+25=090 True
9 1 169 74+94=168 74+94=168 True
9 1 170 87+55=142 87+55=142 True
9 1 171 90+67=157 90+67=157 True
9 1 172 11+02=013 11+02=014 False
9 1 173 01+66=067 01+66=067 True
9 1 174 56+00=056 56+00=056 True
9 1 175 58+52=110 58+52=110 True
9 1 176 24+99=123 24+99=123 True
9 1 177 97+13=110 97+13=100 False
9 1 178 42+94=136 42+94=137 False
9 1 179 60+15=075 60+15=075 True
9 1 180 20+46=066 20+46=066 True
9 1 181 40+70=110 40+70=110 True
9 1 182 95+45=140 95+45=140 True
9 1 183 96+95=191 96+95=191 True
9 1 184 98+20=118 98+20=118 True
9 1 185 43+19=062 43+19=072 False
9 1 186 50+69=119 50+69=119 True
9 1 187 27+53=080 27+53=080 True
9 1 188 24+25=049 24+25=059 False
9 1 189 65+92=157 65+92=157 True
9 1 190 28+14=042 28+14=052 False
9 1 191 20+57=077 20+57=077 True
9 1 192 59+97=156 59+97=156 True
9 1 193 98+32=130 98+32=130 True
9 1 194 55+84=139 55+84=139 True
9 1 195 20+39=059 20+39=069 False
9 1 196 86+47=133 86+47=133 True
9 1 197 92+36=128 92+36=128 True
9 1 198 05+38=043 05+38=053 False
9 1 199 77+36=113 77+36=113 True
9 1 200 41+64=105 41+64=105 True
9 1 201 74+51=125 74+51=125 True
9 1 202 74+55=129 74+55=129 True
9 1 203 64+64=128 64+64=128 True
9 1 204 60+19=079 60+19=079 True
9 1 205 77+96=173 77+96=173 True
9 1 206 22+30=052 22+30=062 False
9 1 207 82+49=131 82+49=131 True
9 1 208 39+67=106 39+67=106 True
9 1 209 62+40=102 62+40=102 True
9 1 210 28+71=099 28+71=099 True
9 1 211 47+26=073 47+26=073 True
9 1 212 98+54=152 98+54=152 True
9 1 213 38+70=108 38+70=108 True
9 1 214 63+40=103 63+40=103 True
9 1 215 86+62=148 86+62=148 True
9 1 216 22+65=087 22+65=087 True
9 1 217 41+17=058 41+17=068 False
9 1 218 68+88=156 68+88=156 True
9 1 219 96+70=166 96+70=166 True
9 1 220 99+29=128 99+29=127 False
9 1 221 83+39=122 83+39=122 True
9 1 222 26+55=081 26+55=081 True
9 1 223 53+70=123 53+70=123 True
9 1 224 94+12=106 94+12=107 False
9 1 225 00+37=037 00+37=037 True
9 1 226 36+94=130 36+94=120 False
9 1 227 40+58=098 40+58=098 True
9 1 228 19+80=099 19+80=099 True
9 1 229 49+44=093 49+44=093 True
9 1 230 70+27=097 70+27=097 True
9 1 231 52+80=132 52+80=132 True
9 1 232 77+90=167 77+90=167 True
9 1 233 13+92=105 13+92=106 False
9 1 234 59+09=068 59+09=067 False
9 1 235 33+55=088 33+55=088 True
9 1 236 85+16=101 85+16=101 True
9 1 237 25+65=090 25+65=090 True
9 1 238 46+20=066 46+20=076 False
9 1 239 29+52=081 29+52=081 True
9 1 240 32+36=068 32+36=078 False
9 1 241 47+08=055 47+08=065 False
9 1 242 21+84=105 21+84=106 False
9 1 243 24+45=069 24+45=079 False
9 1 244 29+15=044 29+15=044 True
9 1 245 83+03=086 83+03=087 False
9 1 246 83+36=119 83+36=119 True
9 1 247 58+95=153 58+95=153 True
9 1 248 76+79=155 76+79=155 True
9 1 249 63+30=093 63+30=093 True
9 1 250 38+24=062 38+24=072 False
9 1 251 19+46=065 19+46=065 True
9 1 252 99+66=165 99+66=165 True
9 1 253 95+73=168 95+73=168 True
9 1 254 65+27=092 65+27=092 True
9 1 255 91+83=174 91+83=175 False
9 2 0 65+49=114 65+49=114 True
9 2 1 03+08=011 03+08=011 True
9 2 2 67+81=148 67+81=148 True
9 2 3 47+23=070 47+23=070 True
9 2 4 43+91=134 43+91=135 False
9 2 5 41+67=108 41+67=108 True
9 2 6 02+33=035 02+33=046 False
9 2 7 64+84=148 64+84=148 True
9 2 8 81+64=145 81+64=145 True
9 2 9 80+11=091 80+11=091 True
9 2 10 78+01=079 78+01=079 True
9 2 11 89+18=107 89+18=107 True
9 2 12 45+52=097 45+52=097 True
9 2 13 35+30=065 35+30=075 False
9 2 14 53+32=085 53+32=086 False
9 2 15 49+90=139 49+90=139 True
9 2 16 41+37=078 41+37=078 True
9 2 17 35+14=049 35+14=059 False
9 2 18 92+50=142 92+50=142 True
9 2 19 37+60=097 37+60=097 True
9 2 20 91+61=152 91+61=152 True
9 2 21 80+77=157 80+77=157 True
9 2 22 66+24=090 66+24=090 True
9 2 23 81+07=088 81+07=088 True
9 2 24 85+59=144 85+59=144 True
9 2 25 19+69=088 19+69=087 False
9 2 26 91+44=135 91+44=135 True
9 2 27 25+29=054 25+29=064 False
9 2 28 27+08=035 27+08=035 True
9 2 29 66+14=080 66+14=080 True
9 2 30 95+11=106 95+11=106 True
9 2 31 13+97=110 13+97=100 False
9 2 32 94+40=134 94+40=134 True
9 2 33 74+31=105 74+31=105 True
9 2 34 49+00=049 49+00=059 False
9 2 35 59+18=077 59+18=077 True
9 2 36 07+65=072 07+65=072 True
9 2 37 83+55=138 83+55=138 True
9 2 38 49+80=129 49+80=129 True
9 2 39 64+17=081 64+17=081 True
9 2 40 48+83=131 48+83=131 True
9 2 41 95+44=139 95+44=139 True
9 2 42 71+26=097 71+26=097 True
9 2 43 06+74=080 06+74=080 True
9 2 44 34+24=058 34+24=068 False
9 2 45 59+71=130 59+71=120 False
9 2 46 68+32=100 68+32=100 True
9 2 47 38+81=119 38+81=119 True
9 2 48 29+56=085 29+56=085 True
9 2 49 54+55=109 54+55=109 True
9 2 50 31+27=058 31+27=068 False
9 2 51 97+89=186 97+89=186 True
9 2 52 48+09=057 48+09=057 True
9 2 53 86+76=162 86+76=162 True
9 2 54 82+59=141 82+59=141 True
9 2 55 01+67=068 01+67=068 True
9 2 56 26+06=032 26+06=032 True
9 2 57 22+46=068 22+46=078 False
9 2 58 85+16=101 85+16=101 True
9 2 59 29+08=037 29+08=037 True
9 2 60 73+94=167 73+94=167 True
9 2 61 19+62=081 19+62=081 True
9 2 62 86+62=148 86+62=148 True
9 2 63 38+99=137 38+99=137 True
9 2 64 64+25=089 64+25=089 True
9 2 65 61+72=133 61+72=134 False
9 2 66 78+88=166 78+88=166 True
9 2 67 43+66=109 43+66=109 True
9 2 68 69+35=104 69+35=104 True
9 2 69 33+77=110 33+77=110 True
9 2 70 37+37=074 37+37=084 False
9 2 71 87+54=141 87+54=141 True
9 2 72 68+90=158 68+90=158 True
9 2 73 83+44=127 83+44=127 True
9 2 74 41+09=050 41+09=050 True
9 2 75 13+48=061 13+48=071 False
9 2 76 01+41=042 01+41=052 False
9 2 77 19+74=093 19+74=093 True
9 2 78 15+05=020 15+05=010 False
9 2 79 55+46=101 55+46=101 True
9 2 80 68+33=101 68+33=101 True
9 2 81 44+40=084 44+40=084 True
9 2 82 88+03=091 88+03=091 True
9 2 83 81+79=160 81+79=160 True
9 2 84 18+98=116 18+98=116 True
9 2 85 70+64=134 70+64=134 True
9 2 86 26+44=070 26+44=070 True
9 2 87 98+87=185 98+87=185 True
9 2 88 18+74=092 18+74=092 True
9 2 89 50+68=118 50+68=118 True
9 2 90 13+51=064 13+51=065 False
9 2 91 90+89=179 90+89=179 True
9 2 92 47+78=125 47+78=125 True
9 2 93 81+57=138 81+57=138 True
9 2 94 34+47=081 34+47=081 True
9 2 95 94+23=117 94+23=117 True
9 2 96 07+70=077 07+70=077 True
9 2 97 56+33=089 56+33=089 True
9 2 98 33+04=037 33+04=037 True
9 2 99 26+09=035 26+09=035 True
9 2 100 14+92=106 14+92=107 False
9 2 101 78+54=132 78+54=132 True
9 2 102 36+76=112 36+76=112 True
9 2 103 17+47=064 17+47=074 False
9 2 104 28+18=046 28+18=046 True
9 2 105 78+54=132 78+54=132 True
9 2 106 84+72=156 84+72=157 False
9 2 107 00+44=044 00+44=054 False
9 2 108 50+41=091 50+41=091 True
9 2 109 87+88=175 87+88=175 True
9 2 110 11+66=077 11+66=077 True
9 2 111 80+60=140 80+60=140 True
9 2 112 78+76=154 78+76=154 True
9 2 113 24+74=098 24+74=098 True
9 2 114 88+48=136 88+48=136 True
9 2 115 38+31=069 38+31=079 False
9 2 116 29+27=056 29+27=066 False
9 2 117 08+45=053 08+45=063 False
9 2 118 28+13=041 28+13=051 False
9 2 119 53+99=152 53+99=152 True
9 2 120 47+92=139 47+92=139 True
9 2 121 76+21=097 76+21=097 True
9 2 122 53+96=149 53+96=149 True
9 2 123 93+91=184 93+91=185 False
9 2 124 97+33=130 97+33=120 False
9 2 125 67+78=145 67+78=145 True
9 2 126 58+05=063 58+05=073 False
9 2 127 00+16=016 00+16=016 True
9 2 128 80+19=099 80+19=099 True
9 2 129 98+22=120 98+22=110 False
9 2 130 09+62=071 09+62=071 True
9 2 131 06+23=029 06+23=029 True
9 2 132 32+99=131 32+99=131 True
9 2 133 17+02=019 17+02=019 True
9 2 134 64+35=099 64+35=099 True
9 2 135 35+83=118 35+83=118 True
9 2 136 71+36=107 71+36=107 True
9 2 137 75+06=081 75+06=081 True
9 2 138 88+95=183 88+95=183 True
9 2 139 19+98=117 19+98=117 True
9 2 140 28+89=117 28+89=117 True
9 2 141 33+11=044 33+11=055 False
9 2 142 34+49=083 34+49=083 True
9 2 143 90+35=125 90+35=125 True
9 2 144 22+90=112 22+90=112 True
9 2 145 98+89=187 98+89=187 True
9 2 146 88+47=135 88+47=135 True
9 2 147 30+86=116 30+86=116 True
9 2 148 31+48=079 31+48=089 False
9 2 149 39+21=060 39+21=060 True
9 2 150 19+17=036 19+17=036 True
9 2 151 27+60=087 27+60=087 True
9 2 152 12+16=028 12+16=028 True
9 2 153 51+75=126 51+75=126 True
9 2 154 10+74=084 10+74=084 True
9 2 155 42+63=105 42+63=106 False
9 2 156 40+14=054 40+14=064 False
9 2 157 23+93=116 23+93=117 False
9 2 158 85+26=111 85+26=111 True
9 2 159 28+46=074 28+46=074 True
9 2 160 28+33=061 28+33=071 False
9 2 161 43+30=073 43+30=073 True
9 2 162 89+72=161 89+72=161 True
9 2 163 52+21=073 52+21=073 True
9 2 164 21+54=075 21+54=075 True
9 2 165 69+13=082 69+13=082 True
9 2 166 07+60=067 07+60=067 True
9 2 167 63+83=146 63+83=147 False
9 2 168 80+69=149 80+69=149 True
9 2 169 27+28=055 27+28=065 False
9 2 170 42+31=073 42+31=073 True
9 2 171 51+99=150 51+99=150 True
9 2 172 28+75=103 28+75=103 True
9 2 173 38+57=095 38+57=095 True
9 2 174 83+16=099 83+16=099 True
9 2 175 92+94=186 92+94=187 False
9 2 176 55+75=130 55+75=120 False
9 2 177 59+51=110 59+51=100 False
9 2 178 33+09=042 33+09=052 False
9 2 179 53+13=066 53+13=067 False
9 2 180 05+70=075 05+70=075 True
9 2 181 12+20=032 12+20=032 True
9 2 182 11+49=060 11+49=070 False
9 2 183 63+45=108 63+45=108 True
9 2 184 92+23=115 92+23=116 False
9 2 185 82+45=127 82+45=127 True
9 2 186 23+41=064 23+41=065 False
9 2 187 64+26=090 64+26=090 True
9 2 188 91+24=115 91+24=115 True
9 2 189 20+32=052 20+32=062 False
9 2 190 83+21=104 83+21=105 False
9 2 191 07+20=027 07+20=027 True
9 2 192 94+14=108 94+14=108 True
9 2 193 96+89=185 96+89=185 True
9 2 194 13+08=021 13+08=021 True
9 2 195 32+05=037 32+05=037 True
9 2 196 09+51=060 09+51=060 True
9 2 197 26+29=055 26+29=065 False
9 2 198 49+65=114 49+65=114 True
9 2 199 32+66=098 32+66=098 True
9 2 200 41+08=049 41+08=059 False
9 2 201 26+79=105 26+79=105 True
9 2 202 29+91=120 29+91=110 False
9 2 203 51+00=051 51+00=051 True
9 2 204 61+60=121 61+60=111 False
9 2 205 45+78=123 45+78=123 True
9 2 206 56+16=072 56+16=072 True
9 2 207 66+68=134 66+68=134 True
9 2 208 32+16=048 32+16=058 False
9 2 209 84+49=133 84+49=133 True
9 2 210 45+09=054 45+09=064 False
9 2 211 96+78=174 96+78=174 True
9 2 212 10+02=012 10+02=012 True
9 2 213 36+60=096 36+60=096 True
9 2 214 44+36=080 44+36=080 True
9 2 215 12+86=098 12+86=098 True
9 2 216 94+54=148 94+54=148 True
9 2 217 64+73=137 64+73=137 True
9 2 218 73+10=083 73+10=083 True
9 2 219 14+62=076 14+62=076 True
9 2 220 25+22=047 25+22=057 False
9 2 221 94+22=116 94+22=116 True
9 2 222 41+76=117 41+76=117 True
9 2 223 38+46=084 38+46=084 True
9 2 224 71+72=143 71+72=144 False
9 2 225 74+79=153 74+79=153 True
9 2 226 99+67=166 99+67=166 True
9 2 227 78+71=149 78+71=149 True
9 2 228 23+19=042 23+19=052 False
9 2 229 51+65=116 51+65=116 True
9 2 230 94+86=180 94+86=180 True
9 2 231 09+79=088 09+79=087 False
9 2 232 69+39=108 69+39=107 False
9 2 233 84+13=097 84+13=097 True
9 2 234 36+59=095 36+59=095 True
9 2 235 87+47=134 87+47=134 True
9 2 236 50+00=050 50+00=050 True
9 2 237 76+96=172 76+96=172 True
9 2 238 12+18=030 12+18=030 True
9 2 239 99+95=194 99+95=194 True
9 2 240 22+00=022 22+00=022 True
9 2 241 96+18=114 96+18=114 True
9 2 242 51+20=071 51+20=071 True
9 2 243 66+81=147 66+81=147 True
9 2 244 78+18=096 78+18=096 True
9 2 245 09+78=087 09+78=087 True
9 2 246 24+20=044 24+20=054 False
9 2 247 76+13=089 76+13=089 True
9 2 248 05+10=015 05+10=015 True
9 2 249 97+14=111 97+14=111 True
9 2 250 92+38=130 92+38=130 True
9 2 251 77+13=090 77+13=090 True
9 2 252 70+19=089 70+19=089 True
9 2 253 90+45=135 90+45=135 True
9 2 254 50+09=059 50+09=069 False
9 2 255 78+06=084 78+06=084 True
9 3 0 03+25=028 03+25=028 True
9 3 1 48+43=091 48+43=091 True
9 3 2 39+47=086 39+47=086 True
9 3 3 48+19=067 48+19=067 True
9 3 4 07+22=029 07+22=029 True
9 3 5 73+68=141 73+68=141 True
9 3 6 14+56=070 14+56=070 True
9 3 7 96+95=191 96+95=191 True
9 3 8 96+28=124 96+28=124 True
9 3 9 82+05=087 82+05=087 True
9 3 10 27+94=121 27+94=121 True
9 3 11 87+86=173 87+86=173 True
9 3 12 00+68=068 00+68=068 True
9 3 13 11+37=048 11+37=058 False
9 3 14 95+93=188 95+93=188 True
9 3 15 75+82=157 75+82=157 True
9 3 16 41+71=112 41+71=112 True
9 3 17 60+14=074 60+14=074 True
9 3 18 77+77=154 77+77=154 True
9 3 19 31+84=115 31+84=116 False
9 3 20 31+57=088 31+57=088 True
9 3 21 27+87=114 27+87=114 True
9 3 22 31+89=120 31+89=120 True
9 3 23 22+18=040 22+18=040 True
9 3 24 38+25=063 38+25=073 False
9 3 25 64+54=118 64+54=118 True
9 3 26 85+60=145 85+60=145 True
9 3 27 14+71=085 14+71=085 True
9 3 28 06+16=022 06+16=022 True
9 3 29 78+61=139 78+61=139 True
9 3 30 65+75=140 65+75=140 True
9 3 31 13+83=096 13+83=097 False
9 3 32 75+49=124 75+49=114 False
9 3 33 05+78=083 05+78=083 True
9 3 34 66+55=121 66+55=121 True
9 3 35 03+05=008 03+05=008 True
9 3 36 69+99=168 69+99=167 False
9 3 37 52+82=134 52+82=135 False
9 3 38 45+97=142 45+97=142 True
9 3 39 66+17=083 66+17=083 True
9 3 40 36+17=053 36+17=063 False
9 3 41 92+74=166 92+74=167 False
9 3 42 48+44=092 48+44=092 True
9 3 43 34+17=051 34+17=061 False
9 3 44 56+11=067 56+11=077 False
9 3 45 77+23=100 77+23=100 True
9 3 46 10+11=021 10+11=011 False
9 3 47 32+65=097 32+65=097 True
9 3 48 53+49=102 53+49=102 True
9 3 49 68+86=154 68+86=154 True
9 3 50 52+94=146 52+94=147 False
9 3 51 97+71=168 97+71=168 True
9 3 52 05+37=042 05+37=052 False
9 3 53 58+75=133 58+75=133 True
9 3 54 06+24=030 06+24=030 True
9 3 55 15+44=059 15+44=069 False
9 3 56 90+49=139 90+49=139 True
9 3 57 50+37=087 50+37=087 True
9 3 58 88+61=149 88+61=149 True
9 3 59 21+57=078 21+57=078 True
9 3 60 24+85=109 24+85=109 True
9 3 61 01+66=067 01+66=067 True
9 3 62 50+46=096 50+46=096 True
9 3 63 76+65=141 76+65=141 True
9 3 64 23+74=097 23+74=097 True
9 3 65 76+16=092 76+16=092 True
9 3 66 06+08=014 06+08=014 True
9 3 67 69+25=094 69+25=094 True
9 3 68 15+23=038 15+23=038 True
9 3 69 41+02=043 41+02=054 False
9 3 70 16+66=082 16+66=082 True
9 3 71 59+94=153 59+94=153 True
9 3 72 32+88=120 32+88=120 True
9 3 73 46+21=067 46+21=077 False
9 3 74 57+28=085 57+28=085 True
9 3 75 00+31=031 00+31=031 True
9 3 76 77+07=084 77+07=084 True
9 3 77 28+70=098 28+70=098 True
9 3 78 05+61=066 05+61=066 True
9 3 79 22+09=031 22+09=031 True
9 3 80 08+94=102 08+94=102 True
9 3 81 40+11=051 40+11=061 False
9 3 82 10+48=058 10+48=068 False
9 3 83 27+56=083 27+56=083 True
9 3 84 42+16=058 42+16=068 False
9 3 85 69+43=112 69+43=112 True
9 3 86 57+69=126 57+69=126 True
9 3 87 18+86=104 18+86=104 True
9 3 88 86+80=166 86+80=166 True
9 3 89 30+85=115 30+85=115 True
9 3 90 77+66=143 77+66=143 True
9 3 91 39+64=103 39+64=103 True
9 3 92 76+61=137 76+61=137 True
9 3 93 42+61=103 42+61=103 True
9 3 94 07+30=037 07+30=037 True
9 3 95 35+93=128 35+93=128 True
9 3 96 40+90=130 40+90=130 True
9 3 97 08+91=099 08+91=099 True
9 3 98 62+34=096 62+34=097 False
9 3 99 86+49=135 86+49=135 True
9 3 100 73+23=096 73+23=097 False
9 3 101 87+35=122 87+35=122 True
9 3 102 35+31=066 35+31=076 False
9 3 103 07+13=020 07+13=010 False
9 3 104 39+41=080 39+41=080 True
9 3 105 44+63=107 44+63=107 True
9 3 106 94+66=160 94+66=160 True
9 3 107 49+54=103 49+54=103 True
9 3 108 79+46=125 79+46=125 True
9 3 109 53+12=065 53+12=066 False
9 3 110 60+92=152 60+92=152 True
9 3 111 25+60=085 25+60=085 True
9 3 112 64+53=117 64+53=117 True
9 3 113 41+02=043 41+02=054 False
9 3 114 00+97=097 00+97=097 True
9 3 115 12+52=064 12+52=065 False
9 3 116 39+50=089 39+50=089 True
9 3 117 87+21=108 87+21=108 True
9 3 118 04+99=103 04+99=103 True
9 3 119 19+75=094 19+75=094 True
9 3 120 90+05=095 90+05=095 True
9 3 121 54+39=093 54+39=093 True
9 3 122 29+26=055 29+26=065 False
9 3 123 82+95=177 82+95=177 True
9 3 124 55+09=064 55+09=064 True
9 3 125 02+62=064 02+62=065 False
9 3 126 68+30=098 68+30=098 True
9 3 127 99+16=115 99+16=115 True
9 3 128 63+11=074 63+11=075 False
9 3 129 42+92=134 42+92=135 False
9 3 130 99+16=115 99+16=115 True
9 3 131 50+31=081 50+31=081 True
9 3 132 23+46=069 23+46=079 False
9 3 133 45+73=118 45+73=118 True
9 3 134 89+77=166 89+77=166 True
9 3 135 45+78=123 45+78=123 True
9 3 136 96+60=156 96+60=156 True
9 3 137 74+61=135 74+61=135 True
9 3 138 87+01=088 87+01=088 True
9 3 139 63+88=151 63+88=151 True
9 3 140 59+72=131 59+72=131 True
9 3 141 17+96=113 17+96=113 True
9 3 142 89+77=166 89+77=166 True
9 3 143 24+69=093 24+69=093 True
9 3 144 75+83=158 75+83=158 True
9 3 145 50+54=104 50+54=104 True
9 3 146 93+47=140 93+47=140 True
9 3 147 20+55=075 20+55=075 True
9 3 148 91+79=170 91+79=170 True
9 3 149 15+13=028 15+13=028 True
9 3 150 86+09=095 86+09=095 True
9 3 151 29+58=087 29+58=087 True
9 3 152 01+29=030 01+29=030 True
9 3 153 65+48=113 65+48=113 True
9 3 154 96+45=141 96+45=141 True
9 3 155 58+69=127 58+69=127 True
9 3 156 84+43=127 84+43=127 True
9 3 157 90+38=128 90+38=128 True
9 3 158 39+97=136 39+97=136 True
9 3 159 74+84=158 74+84=158 True
9 3 160 86+22=108 86+22=108 True
9 3 161 01+86=087 01+86=087 True
9 3 162 81+63=144 81+63=145 False
9 3 163 80+94=174 80+94=174 True
9 3 164 44+42=086 44+42=086 True
9 3 165 72+60=132 72+60=132 True
9 3 166 28+07=035 28+07=035 True
9 3 167 69+54=123 69+54=123 True
9 3 168 68+77=145 68+77=145 True
9 3 169 90+16=106 90+16=106 True
9 3 170 64+50=114 64+50=114 True
9 3 171 46+88=134 46+88=134 True
9 3 172 55+99=154 55+99=154 True
9 3 173 31+97=128 31+97=128 True
9 3 174 79+28=107 79+28=107 True
9 3 175 81+43=124 81+43=125 False
9 3 176 41+15=056 41+15=066 False
9 3 177 38+77=115 38+77=115 True
9 3 178 25+06=031 25+06=031 True
9 3 179 01+93=094 01+93=095 False
9 3 180 97+22=119 97+22=109 False
9 3 181 71+84=155 71+84=155 True
9 3 182 26+36=062 26+36=072 False
9 3 183 60+92=152 60+92=152 True
9 3 184 02+94=096 02+94=097 False
9 3 185 31+58=089 31+58=089 True
9 3 186 70+52=122 70+52=122 True
9 3 187 19+42=061 19+42=071 False
9 3 188 95+73=168 95+73=168 True
9 3 189 21+25=046 21+25=056 False
9 3 190 13+58=071 13+58=071 True
9 3 191 62+28=090 62+28=090 True
9 3 192 38+14=052 38+14=062 False
9 3 193 66+75=141 66+75=141 True
9 3 194 24+59=083 24+59=083 True
9 3 195 97+66=163 97+66=163 True
9 3 196 76+70=146 76+70=146 True
9 3 197 08+40=048 08+40=058 False
9 3 198 84+00=084 84+00=084 True
9 3 199 54+73=127 54+73=127 True
9 3 200 16+88=104 16+88=104 True
9 3 201 99+47=146 99+47=146 True
9 3 202 31+95=126 31+95=126 True
9 3 203 01+79=080 01+79=080 True
9 3 204 03+68=071 03+68=071 True
9 3 205 10+05=015 10+05=015 True
9 3 206 98+90=188 98+90=188 True
9 3 207 58+53=111 58+53=111 True
9 3 208 34+87=121 34+87=121 True
9 3 209 07+31=038 07+31=048 False
9 3 210 59+08=067 59+08=067 True
9 3 211 51+38=089 51+38=089 True
9 3 212 62+62=124 62+62=125 False
9 3 213 80+32=112 80+32=112 True
9 3 214 69+16=085 69+16=085 True
9 3 215 01+17=018 01+17=018 True
9 3 216 74+41=115 74+41=115 True
9 3 217 20+89=109 20+89=109 True
9 3 218 53+50=103 53+50=103 True
9 3 219 82+85=167 82+85=167 True
9 3 220 34+47=081 34+47=081 True
9 3 221 34+45=079 34+45=089 False
9 3 222 77+34=111 77+34=111 True
9 3 223 56+33=089 56+33=089 True
9 3 224 97+56=153 97+56=153 True
9 3 225 29+06=035 29+06=035 True
9 3 226 78+96=174 78+96=174 True
9 3 227 28+65=093 28+65=093 True
9 3 228 61+64=125 61+64=126 False
9 3 229 32+64=096 32+64=097 False
9 3 230 98+32=130 98+32=130 True
9 3 231 25+35=060 25+35=060 True
9 3 232 05+08=013 05+08=013 True
9 3 233 05+26=031 05+26=031 True
9 3 234 84+71=155 84+71=155 True
9 3 235 33+10=043 33+10=053 False
9 3 236 98+35=133 98+35=133 True
9 3 237 68+98=166 68+98=166 True
9 3 238 03+63=066 03+63=067 False
9 3 239 12+96=108 12+96=108 True
9 3 240 02+81=083 02+81=083 True
9 3 241 83+13=096 83+13=097 False
9 3 242 55+92=147 55+92=147 True
9 3 243 96+09=105 96+09=105 True
9 3 244 61+08=069 61+08=069 True
9 3 245 39+75=114 39+75=114 True
9 3 246 40+74=114 40+74=114 True
9 3 247 39+80=119 39+80=119 True
9 3 248 57+95=152 57+95=152 True
9 3 249 92+97=189 92+97=189 True
9 3 250 33+03=036 33+03=037 False
9 3 251 74+92=166 74+92=166 True
9 3 252 99+09=108 99+09=107 False
9 3 253 98+10=108 98+10=108 True
9 3 254 46+77=123 46+77=123 True
9 3 255 85+78=163 85+78=163 True
9 4 0 41+21=062 41+21=062 True
9 4 1 49+13=062 49+13=072 False
9 4 2 59+07=066 59+07=066 True
9 4 3 31+11=042 31+11=052 False
9 4 4 74+16=090 74+16=090 True
9 4 5 43+38=081 43+38=081 True
9 4 6 08+67=075 08+67=075 True
9 4 7 31+66=097 31+66=097 True
9 4 8 10+31=041 10+31=051 False
9 4 9 34+59=093 34+59=093 True
9 4 10 78+42=120 78+42=110 False
9 4 11 13+41=054 13+41=065 False
9 4 12 97+89=186 97+89=186 True
9 4 13 15+62=077 15+62=077 True
9 4 14 39+36=075 39+36=075 True
9 4 15 21+25=046 21+25=056 False
9 4 16 74+56=130 74+56=120 False
9 4 17 85+47=132 85+47=132 True
9 4 18 47+32=079 47+32=079 True
9 4 19 37+66=103 37+66=103 True
9 4 20 16+29=045 16+29=045 True
9 4 21 86+77=163 86+77=163 True
9 4 22 80+07=087 80+07=087 True
9 4 23 87+05=092 87+05=092 True
9 4 24 58+16=074 58+16=074 True
9 4 25 52+79=131 52+79=131 True
9 4 26 91+08=099 91+08=099 True
9 4 27 47+78=125 47+78=125 True
9 4 28 86+96=182 86+96=182 True
9 4 29 90+22=112 90+22=112 True
9 4 30 31+18=049 31+18=059 False
9 4 31 86+15=101 86+15=101 True
9 4 32 15+95=110 15+95=100 False
9 4 33 42+11=053 42+11=063 False
9 4 34 65+99=164 65+99=164 True
9 4 35 89+29=118 89+29=117 False
9 4 36 35+11=046 35+11=056 False
9 4 37 71+41=112 71+41=112 True
9 4 38 16+24=040 16+24=040 True
9 4 39 77+82=159 77+82=159 True
9 4 40 55+89=144 55+89=144 True
9 4 41 17+88=105 17+88=105 True
9 4 42 54+72=126 54+72=127 False
9 4 43 34+98=132 34+98=132 True
9 4 44 09+97=106 09+97=106 True
9 4 45 91+07=098 91+07=098 True
9 4 46 55+94=149 55+94=149 True
9 4 47 22+58=080 22+58=080 True
9 4 48 91+37=128 91+37=128 True
9 4 49 16+10=026 16+10=026 True
9 4 50 96+32=128 96+32=128 True
9 4 51 35+75=110 35+75=110 True
9 4 52 88+73=161 88+73=161 True
9 4 53 35+18=053 35+18=063 False
9 4 54 33+10=043 33+10=053 False
9 4 55 08+50=058 08+50=068 False
9 4 56 22+62=084 22+62=085 False
9 4 57 26+37=063 26+37=073 False
9 4 58 80+27=107 80+27=107 True
9 4 59 68+28=096 68+28=096 True
9 4 60 48+03=051 48+03=061 False
9 4 61 40+18=058 40+18=068 False
9 4 62 16+59=075 16+59=075 True
9 4 63 02+19=021 02+19=021 True
9 4 64 01+09=010 01+09=010 True
9 4 65 62+68=130 62+68=130 True
9 4 66 09+71=080 09+71=080 True
9 4 67 00+58=058 00+58=068 False
9 4 68 16+45=061 16+45=071 False
9 4 69 24+98=122 24+98=122 True
9 4 70 47+92=139 47+92=139 True
9 4 71 94+84=178 94+84=178 True
9 4 72 21+32=053 21+32=064 False
9 4 73 29+82=111 29+82=111 True
9 4 74 32+79=111 32+79=111 True
9 4 75 13+98=111 13+98=111 True
9 4 76 41+94=135 41+94=136 False
9 4 77 51+84=135 51+84=136 False
9 4 78 42+05=047 42+05=057 False
9 4 79 39+03=042 39+03=052 False
9 4 80 02+92=094 02+92=095 False
9 4 81 99+81=180 99+81=180 True
9 4 82 32+68=100 32+68=100 True
9 4 83 52+17=069 52+17=079 False
9 4 84 56+58=114 56+58=114 True
9 4 85 21+48=069 21+48=079 False
9 4 86 61+71=132 61+71=132 True
9 4 87 17+01=018 17+01=018 True
9 4 88 68+23=091 68+23=091 True
9 4 89 00+37=037 00+37=037 True
9 4 90 94+88=182 94+88=182 True
9 4 91 06+31=037 06+31=037 True
9 4 92 27+18=045 27+18=055 False
9 4 93 41+81=122 41+81=122 True
9 4 94 15+86=101 15+86=101 True
9 4 95 36+87=123 36+87=123 True
9 4 96 17+37=054 17+37=064 False
9 4 97 13+86=099 13+86=099 True
9 4 98 29+69=098 29+69=097 False
9 4 99 31+99=130 31+99=130 True
9 4 100 47+29=076 47+29=076 True
9 4 101 08+81=089 08+81=089 True
9 4 102 72+82=154 72+82=155 False
9 4 103 46+91=137 46+91=137 True
9 4 104 70+35=105 70+35=105 True
9 4 105 90+55=145 90+55=145 True
9 4 106 99+99=198 99+99=197 False
9 4 107 60+97=157 60+97=157 True
9 4 108 03+40=043 03+40=053 False
9 4 109 35+49=084 35+49=084 True
9 4 110 32+02=034 32+02=035 False
9 4 111 70+18=088 70+18=088 True
9 4 112 99+05=104 99+05=104 True
9 4 113 78+73=151 78+73=151 True
9 4 114 03+02=005 03+02=006 False
9 4 115 50+14=064 50+14=064 True
9 4 116 62+02=064 62+02=065 False
9 4 117 16+74=090 16+74=090 True
9 4 118 68+65=133 68+65=133 True
9 4 119 74+81=155 74+81=155 True
9 4 120 37+48=085 37+48=085 True
9 4 121 63+04=067 63+04=067 True
9 4 122 06+62=068 06+62=068 True
9 4 123 95+75=170 95+75=170 True
9 4 124 92+37=129 92+37=129 True
9 4 125 81+32=113 81+32=113 True
9 4 126 53+28=081 53+28=081 True
9 4 127 52+42=094 52+42=095 False
9 4 128 66+97=163 66+97=163 True
9 4 129 00+48=048 00+48=058 False
9 4 130 65+32=097 65+32=097 True
9 4 131 60+89=149 60+89=149 True
9 4 132 71+61=132 71+61=122 False
9 4 133 98+50=148 98+50=148 True
9 4 134 90+96=186 90+96=186 True
9 4 135 02+96=098 02+96=098 True
9 4 136 62+75=137 62+75=137 True
9 4 137 41+28=069 41+28=079 False
9 4 138 95+79=174 95+79=174 True
9 4 139 48+41=089 48+41=089 True
9 4 140 87+95=182 87+95=182 True
9 4 141 75+38=113 75+38=113 True
9 4 142 31+55=086 31+55=086 True
9 4 143 54+63=117 54+63=117 True
9 4 144 75+82=157 75+82=157 True
9 4 145 46+45=091 46+45=091 True
9 4 146 13+08=021 13+08=021 True
9 4 147 77+97=174 77+97=174 True
9 4 148 37+35=072 37+35=082 False
9 4 149 21+89=110 21+89=110 True
9 4 150 58+51=109 58+51=109 True
9 4 151 91+48=139 91+48=139 True
9 4 152 33+23=056 33+23=067 False
9 4 153 80+96=176 80+96=176 True
9 4 154 78+02=080 78+02=080 True
9 4 155 38+95=133 38+95=133 True
9 4 156 99+25=124 99+25=124 True
9 4 157 30+76=106 30+76=106 True
9 4 158 42+40=082 42+40=082 True
9 4 159 85+58=143 85+58=143 True
9 4 160 44+46=090 44+46=090 True
9 4 161 06+41=047 06+41=057 False
9 4 162 65+90=155 65+90=155 True
9 4 163 43+83=126 43+83=127 False
9 4 164 36+61=097 36+61=097 True
9 4 165 61+51=112 61+51=112 True
9 4 166 38+09=047 38+09=047 True
9 4 167 21+97=118 21+97=118 True
9 4 168 83+30=113 83+30=113 True
9 4 169 11+79=090 11+79=090 True
9 4 170 14+29=043 14+29=053 False
9 4 171 21+11=032 21+11=032 True
9 4 172 43+53=096 43+53=097 False
9 4 173 02+58=060 02+58=060 True
9 4 174 78+82=160 78+82=160 True
9 4 175 91+11=102 91+11=102 True
9 4 176 58+54=112 58+54=112 True
9 4 177 00+15=015 00+15=015 True
9 4 178 83+51=134 83+51=135 False
9 4 179 44+72=116 44+72=116 True
9 4 180 71+20=091 71+20=091 True
9 4 181 24+99=123 24+99=123 True
9 4 182 46+30=076 46+30=076 True
9 4 183 08+67=075 08+67=075 True
9 4 184 47+42=089 47+42=089 True
9 4 185 95+67=162 95+67=162 True
9 4 186 40+56=096 40+56=096 True
9 4 187 17+95=112 17+95=112 True
9 4 188 94+66=160 94+66=160 True
9 4 189 14+58=072 14+58=072 True
9 4 190 56+05=061 56+05=071 False
9 4 191 70+01=071 70+01=071 True
9 4 192 97+59=156 97+59=156 True
9 4 193 94+67=161 94+67=161 True
9 4 194 13+41=054 13+41=065 False
9 4 195 85+15=100 85+15=090 False
9 4 196 48+53=101 48+53=101 True
9 4 197 62+75=137 62+75=137 True
9 4 198 87+47=134 87+47=134 True
9 4 199 31+88=119 31+88=119 True
9 4 200 97+16=113 97+16=113 True
9 4 201 48+45=093 48+45=093 True
9 4 202 99+00=099 99+00=099 True
9 4 203 15+01=016 15+01=016 True
9 4 204 28+96=124 28+96=124 True
9 4 205 20+11=031 20+11=031 True
9 4 206 07+56=063 07+56=073 False
9 4 207 06+08=014 06+08=014 True
9 4 208 45+46=091 45+46=091 True
9 4 209 48+85=133 48+85=133 True
9 4 210 62+14=076 62+14=077 False
9 4 211 82+31=113 82+31=113 True
9 4 212 85+88=173 85+88=173 True
9 4 213 77+08=085 77+08=085 True
9 4 214 16+64=080 16+64=080 True
9 4 215 00+27=027 00+27=027 True
9 4 216 36+75=111 36+75=111 True
9 4 217 38+38=076 38+38=076 True
9 4 218 88+32=120 88+32=110 False
9 4 219 09+88=097 09+88=097 True
9 4 220 96+87=183 96+87=183 True
9 4 221 71+29=100 71+29=100 True
9 4 222 99+13=112 99+13=112 True
9 4 223 03+13=016 03+13=017 False
9 4 224 67+23=090 67+23=090 True
9 4 225 15+98=113 15+98=113 True
9 4 226 10+08=018 10+08=018 True
9 4 227 46+24=070 46+24=070 True
9 4 228 55+63=118 55+63=118 True
9 4 229 28+06=034 28+06=034 True
9 4 230 43+87=130 43+87=120 False
9 4 231 34+05=039 34+05=049 False
2024-12-17 09:44:24,295 - root - WARNING - The method `test_one_epoch` is not implemented. Please override this method in a subclass or use a custom callback.
Trained Attention Heatmaps#
torch.manual_seed(composer.global_.seed)
batch = next(iter(train_loader))
inputs, targets, target_padding_masks, future_masks = batch
trained_model = _trained_state.model
trained_model.eval()
with torch.no_grad():
logits = model(inputs, target_padding_masks=target_padding_masks, future_masks=future_masks)
We zoom into just 1 example in the batch, note that the predicted values are jibberish for the first few tokens (before equal sign) because recall we told the model to practically “don’t care” about any tokens before the answer by padding them with masks. As long as our answer is correct, then it is expected.
example_input = inputs[0]
pprint(example_input)
decoded_input = decode_equation(vocabulary, example_input, show_special_tokens=True)
pprint(decoded_input)
example_target = targets[0]
pprint(example_target)
decoded_target = decode_equation(vocabulary, example_target, show_special_tokens=True)
pprint(decoded_target)
example_logits = logits[0]
example_prediction = torch.argmax(example_logits, dim=-1)
pprint(example_prediction)
pprint(decode_equation(vocabulary, example_prediction, show_special_tokens=True))
tensor([14, 3, 1, 10, 0, 4, 13, 0, 3, 5])
'<BOS>31+04=035'
tensor([16, 16, 16, 16, 16, 16, 0, 3, 5, 15])
'<PAD><PAD><PAD><PAD><PAD><PAD>035<EOS>'
tensor([ 1, 4, 0, 1, 5, 0, 0, 3, 5, 15])
'140150035<EOS>'
Indeed we get a correct answer 35.
We take last decoder block’s attention weights to visualize, we can take any decoder block’s attention weights to visualize, but we take the last one for simplicity and hinging on the fact that the last decoder block is the one that contains the most information about the input sequence.
last_decoder_block = trained_model.decoder_blocks[-1]
masked_self_attention_mha = last_decoder_block.masked_self_attention_mha
context_vector, attention_weights = masked_self_attention_mha.context_vector, masked_self_attention_mha.attention_weights
pprint(attention_weights.shape)
torch.Size([256, 4, 10, 10])
We want to select the example earlier, which is conveniently the first example.
# take first sample
example_attention_weights = attention_weights[0, :, :, :].unsqueeze(0)
pprint(example_attention_weights.shape)
torch.Size([1, 4, 10, 10])
So for the xtick and ytick, the attention weight matrix is \(T \times T\), and
first row is first token, seonc row is second token etc. And note that the x
axis is keys and y axis is queries. This convention is easy to see from the
attention scores formula of Q @ K.T
where Q
is the query and K
is the key.
The resulting attention scores matrix has dimensions (num_queries
,
num_keys
), where each row corresponds to a query and each column corresponds
to a key. In our case is just TxT
since both query and keys have hame length.
When visualizing the attention scores as a heatmap, the x-axis corresponds to
the keys dimension (num_keys), and the y-axis corresponds to the queries
dimension (num_queries). This alignment matches the mathematical formulation of
the attention computation.
xticks = ['<BOS>'] + list(decoded_input[5:])
yticks = xticks
fig = show_attention_heatmaps(
attention_weights=example_attention_weights,
xlabel="Keys",
ylabel="Queries",
xticks=xticks,
yticks=yticks,
show_title=True,
show_values=True,
value_dp=2,
figure_kwargs={"figsize": (40, 15)},
)
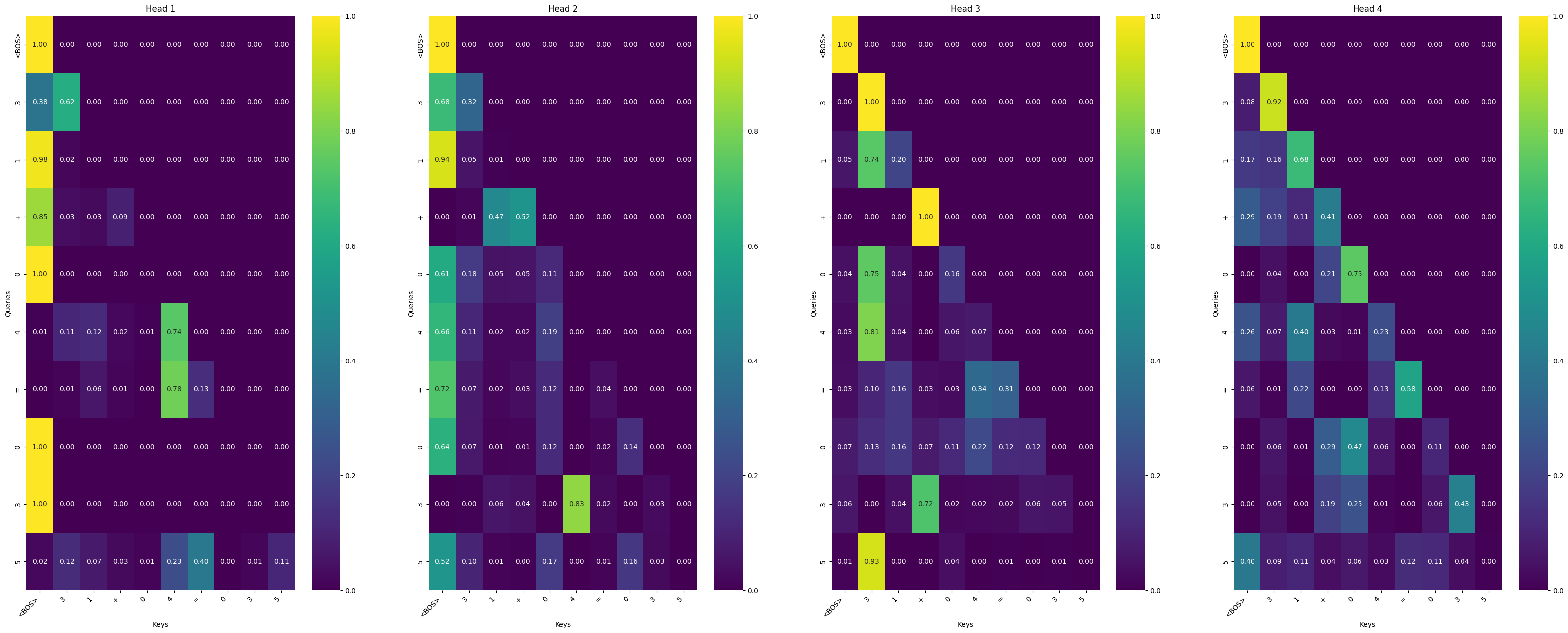
If we want two samples.
_ = show_attention_heatmaps(attention_weights[0:2], show_title=True, show_values=False)
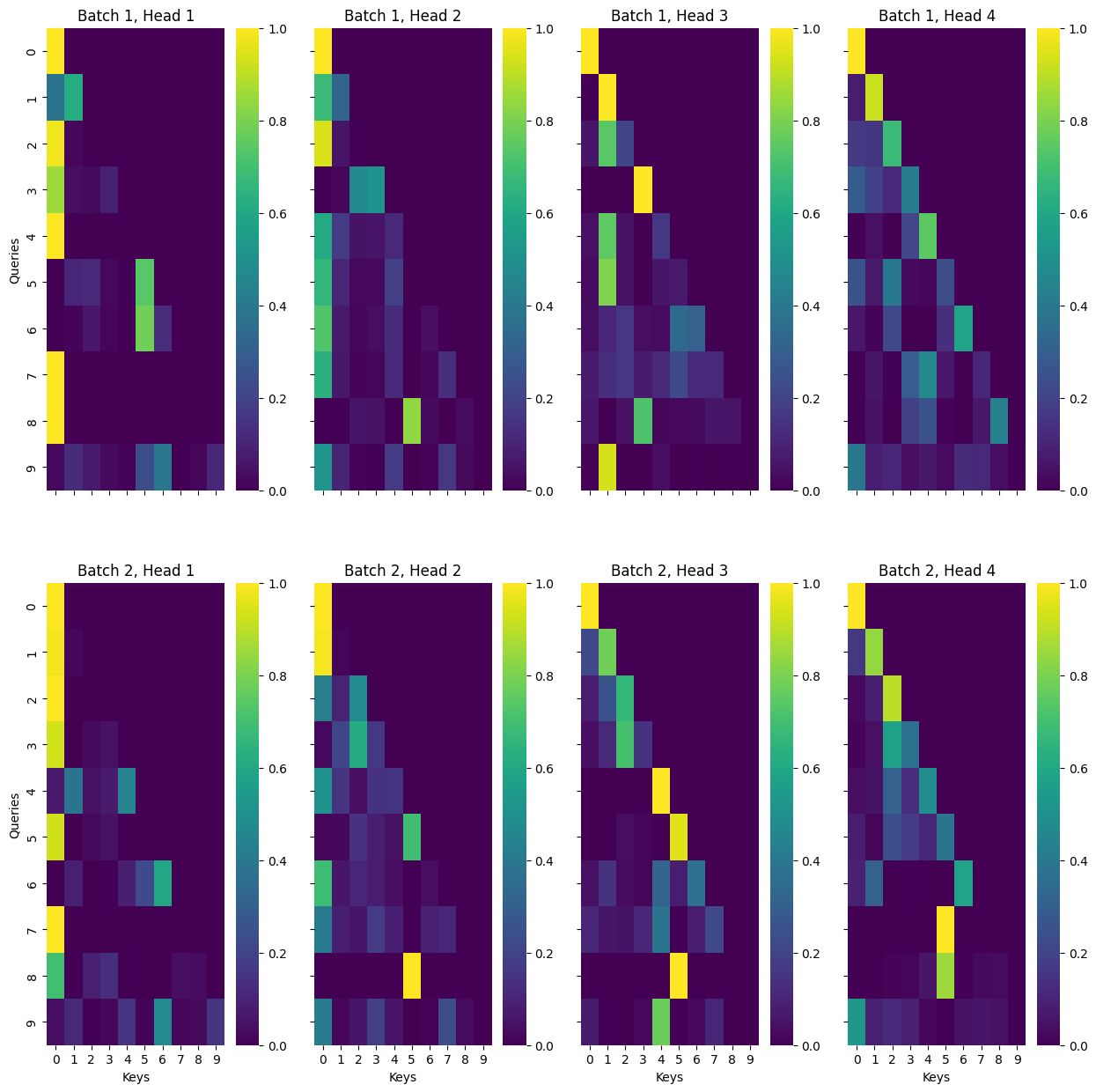
the xy axis is keys and queries, which is correct Q @ K.T
last_decoder_block = trained_model.decoder_blocks[-1]
masked_self_attention_mha = last_decoder_block.masked_self_attention_mha
context_vector, attention_weights = masked_self_attention_mha.context_vector, masked_self_attention_mha.attention_weights
num_heads = attention_weights.size(1)
# Labels for each character in the sequence, including BOS
labels = ['<BOS>'] + list('59+14=073')
# Loop over each head and plot its heatmap
for head in range(num_heads):
plt.figure(figsize=(10, 10))
# Extract attention weights for the last sample in the last batch for this head
attention_matrix = attention_weights[-1, head, :, :].detach().numpy()
sns.heatmap(attention_matrix, annot=True, cmap='viridis', xticklabels=labels, yticklabels=labels)
plt.title(f"Attention Weights Heatmap for '<BOS>59+14=073' - Head {head+1}")
plt.xlabel("Keys")
plt.ylabel("Queries")
plt.show()
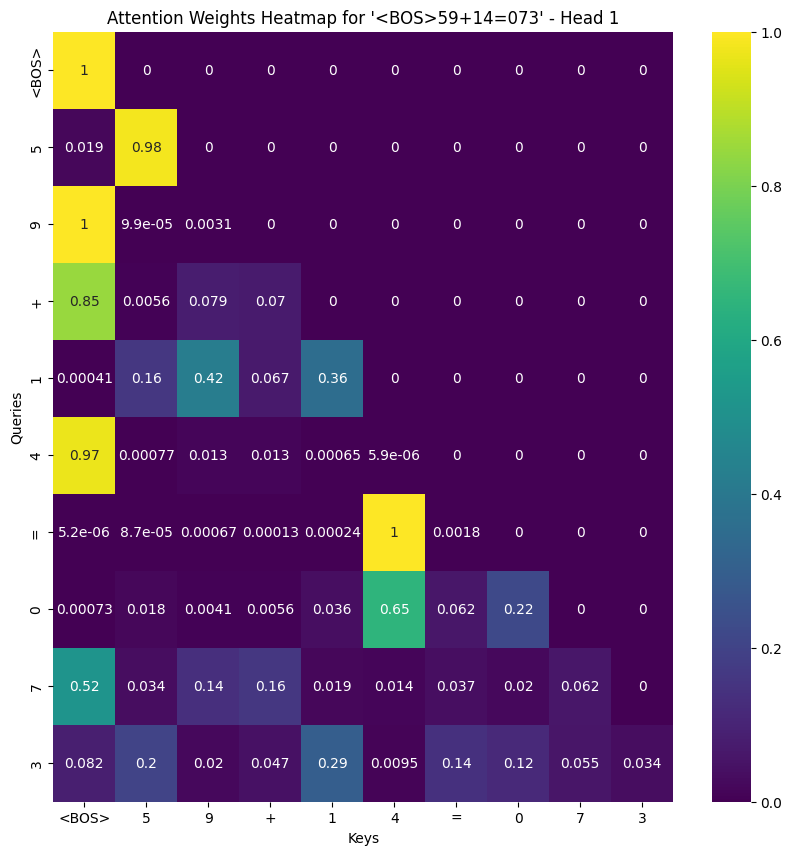
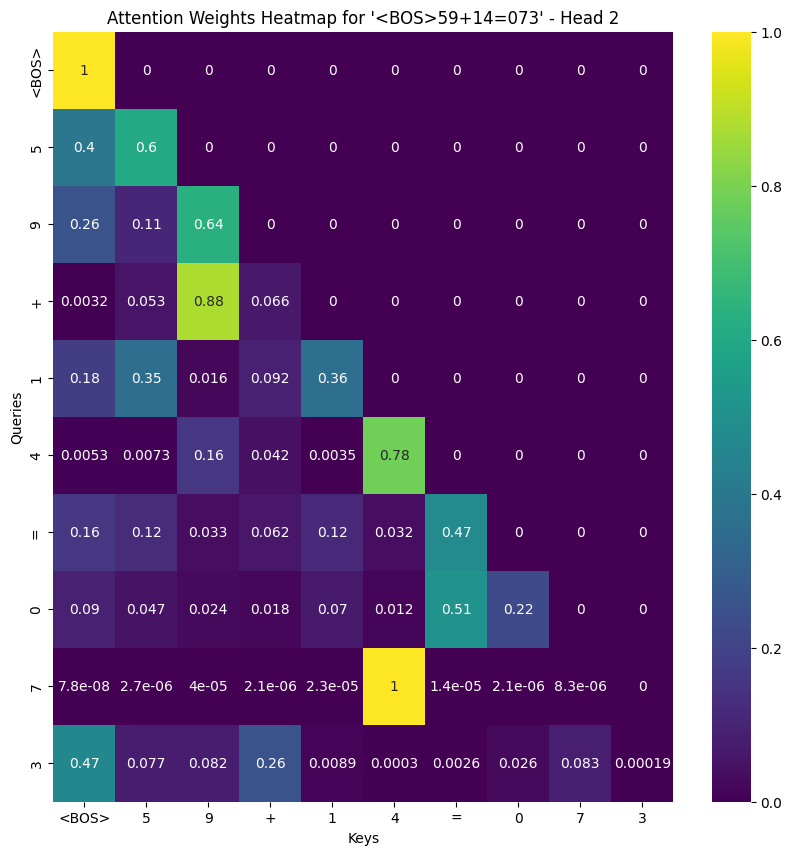
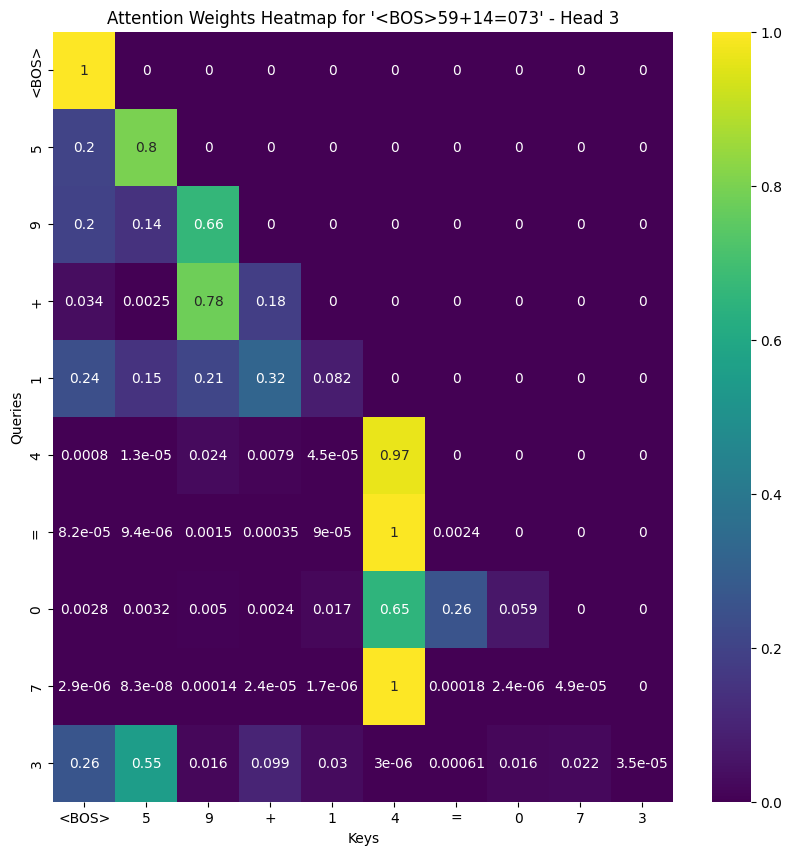
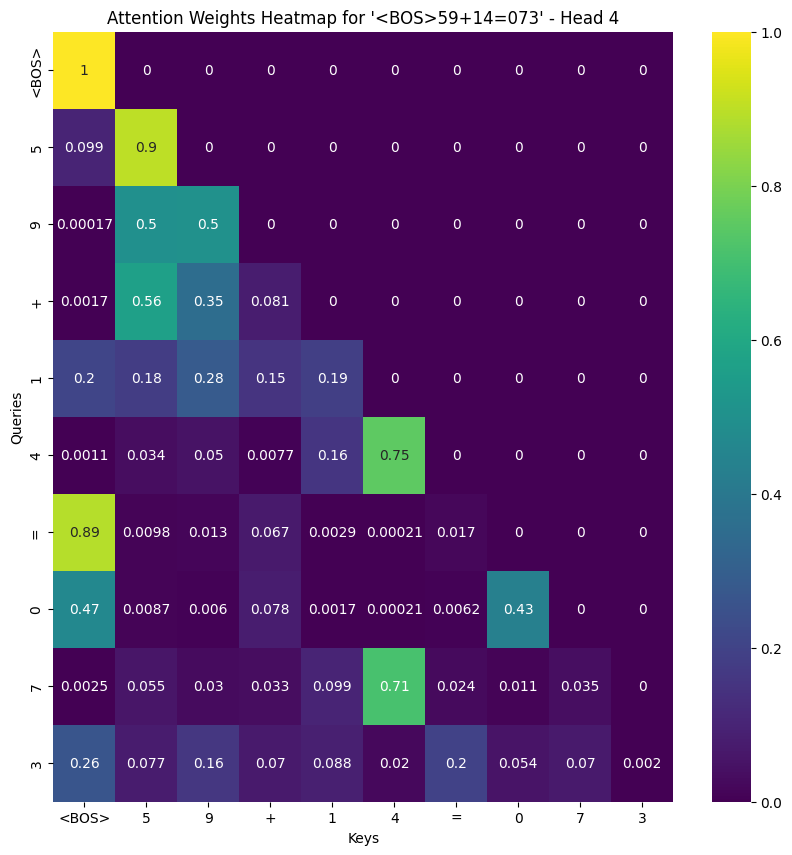
Generation#
Deprecated To Redo
logits.argmax(dim=-1)
basically compress 1x7x18 to 1x7 where for each row of
the 7 rows, find the index that is maximum for example, first row 7.8 is max of
all 18 elements, so index 0 is returned. tensor([[0, 8, 8, 1, 6, 14, 1]])
There is some meaning here too, remember our input [15, 9, 8, 10, 3, 5, 13]
this is basically the BOS (15) up till the equal sign, then
[ 0, 8, 8, 1, 6, 14, 1]
is basically the prediction of each token what comes
next.
Input Sequence: Your input sequence is
[15, 9, 8, 10, 3, 5, 13]
. In this context,15
could be a special token like BOS (Beginning of Sentence) or something else depending on your encoding scheme.Output Tensor Interpretation: The output tensor
tensor([[ 0, 8, 8, 1, 6, 14, 1]])
represents the model’s sequential predictions for each step of the input:The first element
0
is the prediction following the first element15
of the input.The second element
8
is the prediction after seeing the first two elements15, 9
of the input.The third element
8
is predicted after seeing15, 9, 8
.The fourth element
1
follows after15, 9, 8, 10
.The sequence continues in this manner, with each new prediction based on an increasingly longer prefix of the input sequence.
Sequential Predictions: This output suggests that the model is working in an autoregressive manner. It generates predictions one token at a time, and each prediction is based on the sequence of tokens it has seen up to that point.
Specific Meanings of Output Tokens: The actual meaning of each token in your output tensor (
0
,8
,1
,6
,14
, etc.) depends on your specific encoding and task. In a language model, these would correspond to specific words or characters. In a numerical context, they could represent numbers or operations.
In summary, the output tensor reflects the model’s predictions for what comes next in the sequence, based on the current and all previous input tokens. Each element in the output is the model’s guess for the next token, considering the sequence of tokens it has seen up to that point.
Then we move on to the concat operation:
In our model, after processing the input
[15, 9, 8, 10, 3, 5, 13]
, it predicts the next token to be1
. This prediction is based on the entire sequence seen so far.The process of extending the input sequence with this new token (
1
) and then feeding this extended sequence back into the model for further predictions is indeed an example of greedy decoding. The model is iteratively building a longer sequence, one token at a time, always choosing the most likely next token at each step.This process would continue until a stopping condition is met, which might be the prediction of an EOS (End of Sentence) token or reaching a maximum sequence length.
for i in range(num_digits + 2): now you know why loop over 4 times in total if num digits is 2. This is because, after equal sign, we will have answer of 3 digits (xyz) and an EOS token, our stop condition!
Lastly: tensor([[15, 9, 8, 10, 3, 5, 13, 1, 3, 3, 14]])
is the full predicted
after EOS is met.
def what_is(question: str, model: torch.nn.Module, tokenizer: AdderTokenizer, vocabulary: AdderVocabulary, device: torch.device) -> str:
"""
Function for computing the sum of two numbers with input in literal string format.
Args:
question (str): The question string containing the two numbers to add.
model (torch.nn.Module): The trained model for addition.
tokenizer (AdderTokenizer): The tokenizer for encoding and decoding.
vocabulary (AdderVocabulary): The vocabulary used by the tokenizer.
device (torch.device): The device to run the computation on.
Returns:
str: The question with the computed answer appended.
"""
question_tokens = tokenizer.encode(question)
question_tokens = torch.tensor(question_tokens, dtype=torch.long, device=device).unsqueeze(0)
EQUAL = vocabulary.token_to_index[vocabulary.EQUAL]
equal_index = torch.where(question_tokens == EQUAL)[1].item()
starting_tokens = question_tokens[:, :equal_index + 1]
with torch.no_grad():
generated_tokens = model.generate(
starting_tokens=starting_tokens,
max_tokens=4,
greedy=True,
)
generated_equation = tokenizer.decode(generated_tokens.squeeze().tolist(), remove_special_tokens=True)
answer = generated_equation[generated_equation.index("=") + 1:]
return question + answer
what_is("98+35=", model=trained_model, tokenizer=tokenizer, vocabulary=vocabulary, device=composer.trainer.device)
'98+35=133'